“DATATEGY EARLY CAREERS PROGRAM” With Julien Courteville Hello, my name...
Read MoreHow Artificial Intelligence Simplifies Spare Part Searches
Table of Contents
ToggleArtificial intelligence is becoming a powerful tool for businesses across industries, including manufacturing. One area where AI is making a significant impact is in the search for spare parts. By leveraging machine learning algorithms, AI systems can quickly analyze vast amounts of data to identify the correct spare part for a particular machine or equipment. This not only saves time and reduces downtime but also improves overall efficiency and productivity.
A McKinsey report shows that companies using AI have seen a 10-15% boost in production. They also report a 4-5% rise in EBITA, which stands for Earnings Before Interest, Taxes, and Amortization.
Find out how papAI can improve the deployment of AI projects in the Manufacturing Industry.
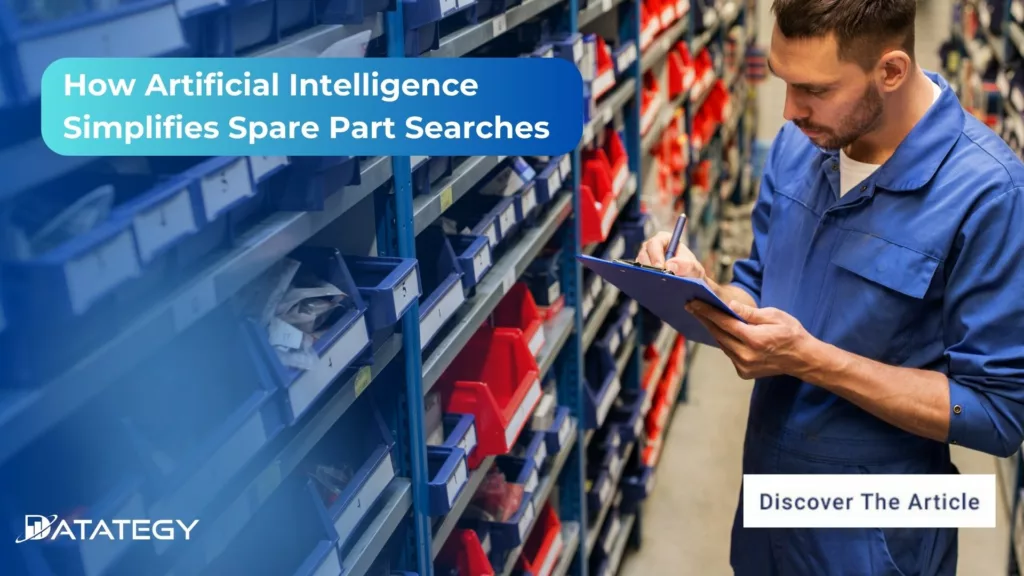
We’ll examine specific AI technologies that support advanced spare part search engines in this post. We’ll look at how these innovative technologies are transforming how companies find, acquire, and handle replacement components.
What does Spare Part Search Powered by AI Mean?
The term “AI-powered spare part search” means using artificial intelligence to improve finding spare parts in a database. Traditional methods for searching spare parts often involve slow lookups, manual data entry, and complex catalogs. These methods can lead to errors and inefficiencies.
AI revolutionizes this procedure by using machine learning, natural language processing, and sophisticated algorithms to deliver fast, precise, and understandable search results. Even with confusing or insufficient data, AI makes it simpler to find the correct part with capabilities like picture recognition, contextual search, and predictive predictions.
AI-powered spare part search improves customer happiness and efficiency while reducing search time and mistakes.
Why is the Demand for Intelligent Search Engines increasing?
In the past, companies used manual techniques, including card catalogs, handwritten records, or basic spreadsheets, to manage and retrieve information. These techniques were effective for smaller inventories, but as industries became larger, they became more difficult to use.
Advancement happened because of digital databases that appeared in the late 20th century. These databases made data storage easier and better organized. It was hard to find specific things without exact keywords or categories. These systems still needed a lot of manual input and had limited search options.
In the 21st century, the expansion of worldwide supply chains, online commerce, and extensive industrial operations amplified the demand for more sophisticated search capabilities. The challenges that businesses began to encounter included vast product catalogs, complex inventory control systems, and the necessity for instantaneous results. Traditional search methods fell short, leading to frustration and inefficiency.
This development opened the door for intelligent search systems driven by machine learning and artificial intelligence. These technologies addressed the shortcomings of previous systems and revolutionized how organizations access and manage their data by introducing dynamic features like picture recognition, natural language processing, and predictive search.
What are the Key Challenges in n Spare Part Search Powered by AI?
The availability and quality of data are two of the main obstacles to AI-powered spare part search. For AI systems to work successfully, enormous volumes of precise and organized data are required. However, a lot of businesses have trouble with old, inconsistent, or incomplete inventory data. Inaccurate search results, misidentifications, or a dearth of pertinent matches might come from poor data.
Furthermore, it might be difficult and time-consuming to integrate many data sources, including unstructured forms like scanned documents, external vendors, and older systems. AI search technologies’ efficacy is greatly reduced in the absence of appropriate data standardization and enrichment, which creates a barrier to smooth operations.
The requirement for AI systems to be flexible and scalable is another significant obstacle. Organisations’ operational needs and inventory data rise as they develop or grow. It is crucial to make sure the AI system can grow to accommodate more datasets and adjust to shifting business requirements. Additionally, for AI models to continue to work, they need to be continuously trained and adjusted.
This calls for constant investment in resources and technological know-how, which might be prohibitive for smaller companies. Additionally, employees may oppose the adoption of AI-powered solutions because they are unfamiliar with them or fear losing their jobs. To achieve effective adoption, businesses must engage in training and change management.
What are the main Advantages of Artificial Intelligence (AI) in Search for Spare Parts?
Increased Precision in Searches:
AI-powered spare part search engines are great at analyzing large amounts of data quickly. They can give accurate results with little effort. Unlike traditional methods, AI can understand vague or unclear questions. Traditional methods often require users to enter specific details or search through big catalogs. Advanced algorithms analyze contextual data to help users find the exact part they need fast.
In sectors where time-sensitive processes depend on the prompt availability of particular parts, this speed and precision are essential. To further improve the search experience, AI also makes it possible to cross-reference related goods, offering substitutes in the event that the requested part is not accessible.
Time and Money Savings:
Saving money and time is a direct result of AI systems’ increased efficiency. Businesses minimize downtime and operational interruptions by promptly locating the relevant part and removing pointless searches. This is particularly important in sectors where delays can lead to large financial losses, such as manufacturing or aviation. Additionally, by automating search procedures, a lot less human labor is required, allowing employees to concentrate on higher-value work.
Better Inventory Control:
Businesses may automate inventory tracking and classification by using AI in spare part management, which will remove manual mistakes and inefficiencies. Real-time stock level monitoring and updates on the availability of particular parts are both possible with AI systems. By doing this, businesses may avoid overstocking or understocking and maintain ideal inventory levels. By anticipating demand trends, AI systems may also expedite the restocking process, assisting businesses in planning their purchases and cutting storage expenses.
Predictive Insight:
Artificial intelligence (AI) solutions offer strong predictive analytics capabilities, forecasting spare part demand based on previous data. By anticipating future demands and making appropriate preparations, these insights help businesses lower the risk of stockouts or excess inventory. Additionally, predictive analytics support maintenance planning, enabling businesses to proactively handle possible problems before they become more serious.
Reduced Errors and Misidentifications:
The possibility of mistakes and misidentifications that frequently beset conventional techniques is greatly decreased by AI-powered spare part search systems. A frequent cause of part identification and ordering errors, human mistakes can result in expensive delays, returns, and interruptions to business operations.
AI systems are capable of precisely identifying parts and cross-referencing data from many sources by utilizing sophisticated algorithms and machine learning. This streamlines procedures and reduces waste by guaranteeing that consumers receive the proper item on their first try.
What are the Main Technologies Behind the use of AI in Spare Part Search?
Natural Language Processing (NLP)
AI-driven spare part search relies heavily on Natural Language Processing (NLP). It enables the machine to replicate human comprehension in the interpretation and processing of text-based requests. Using methods like tokenization, lemmatization, and semantic analysis, NLP dissects questions into their constituent parts so that the AI can comprehend the purpose of the query—even if it contains misspellings or unusual language.
A user looking for a “pump for cooling system,” for instance, doesn’t have to provide the precise component number; NLP may deduce the context and find pertinent results. Furthermore, NLP makes it possible to handle a variety of languages and dialects, which makes it an adaptable option for international enterprises. NLP greatly improves the user experience by bridging the gap between human input and machine comprehension.
Machine Learning Models
The foundation of AI systems is machine learning (ML), which allows them to analyze data and gradually improve performance. To forecast outcomes like part relevance or categorization, supervised learning algorithms such as decision trees and support vector machines train on labeled data.
To improve search recommendations, unsupervised models such as clustering techniques group related objects. By identifying trends in user behavior and past queries, deep learning in particular, neural networks improve search capabilities.
For example, the system can anticipate future requirements and provide proactive recommendations if a user often looks for comparable parts. With continued use, machine learning guarantees that the system gets smarter and more effective.
Computer Vision
Computer vision, powered by advanced image recognition techniques like convolutional neural networks (CNNs), revolutionizes spare part identification. By simply uploading an image of a part, users can leverage AI to analyze its shape, texture, and color. This eliminates the need for manual descriptions, reducing errors caused by incomplete or ambiguous inputs. In industries with visually similar components, computer vision accurately identifies the right part, streamlining the search process and saving valuable time.
Predictive Analytics and Data Mining
Machine learning and statistical models are used in predictive analytics to project future spare component needs. The system forecasts which components are likely to be required and when by examining maintenance data, seasonal trends, and historical usage patterns. Businesses may better predict inventory levels and prevent overstocking and stockouts by using techniques like regression analysis and time-series forecasting. Predictive analytics, when paired with data mining, reveals hidden insights from massive datasets, improving spare part management and guaranteeing improved decision-making.
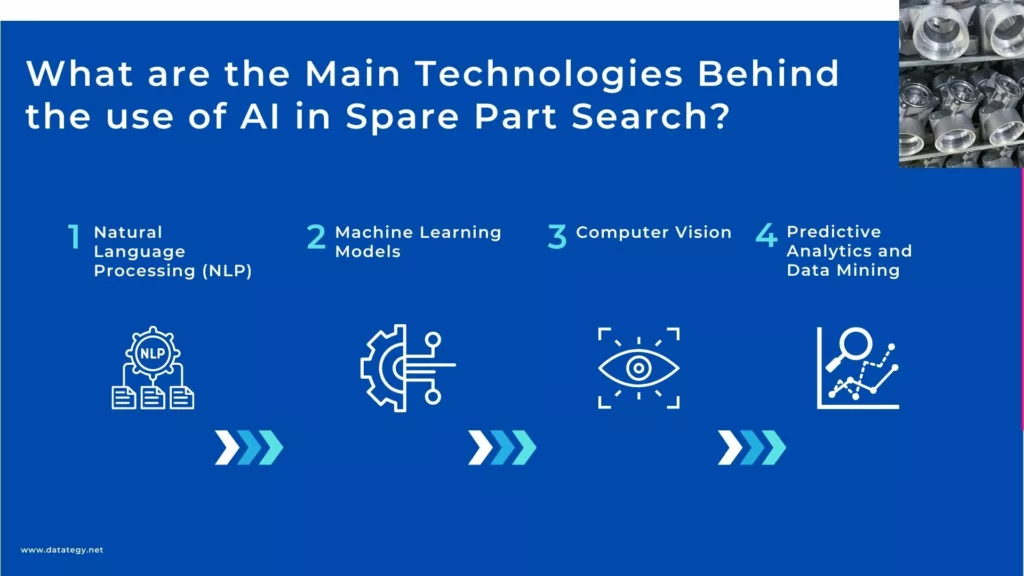
How Spare Part Search is Implemented AI-powered within your companies?
Data Preparation and Standardization
Start by compiling all pertinent inventory data from many sources, including databases, spreadsheets, and ERP systems. Utilise methods such as format alignment, deduplication, and normalization to clean and standardize the data. Make sure the data is comprehensive by filling in the blanks and adding pertinent metadata, including part specs, pictures, and compatibility information, to entries.
Feature Selection and Algorithm Design
Determine which important characteristics, such as part dimensions, material kinds, or operational environment, the AI system should take advantage of. Select the right algorithms according to the requirements of the company; for instance, use natural language processing (NLP) models for textual inquiries or convolutional neural networks (CNNs) for picture recognition. For future scalability, build the system architecture to accommodate modular functionality.
Integration with Existing Systems
Create middleware or APIs to link the AI system to already-existing technologies like warehouse management systems, ERP platforms, and Internet of Things gadgets. Establish real-time data pipelines so that the AI-powered search instantly refreshes in response to inventory changes or equipment telemetry updates.
Model Training and Validation
Train machine learning models using historical data, labeled datasets, or synthetic data. For instance, train an NLP model on common query patterns or an image recognition model on labeled images of spare parts. Validate the model performance through metrics like precision, recall, and F1 score to ensure its reliability in real-world scenarios.
Deployment and Scalability
Install the AI system in a scalable, secure on-premises or cloud-based environment. For flexible deployment, use containerization solutions like Docker or Kubernetes to make sure the system can manage growing feature requirements or data demands.
Real-world Examples of Process Optimization using Artificial Intelligence
Optimizing Spare Part Identification with AI at Deutsche Bahn
Deutsche Bahn (DB), Germany’s national railway operator, faced a significant challenge in efficiently identifying spare parts across its vast network. Technicians were spending an average of 15–20 minutes manually searching for parts, which was both time-consuming and inefficient. To address this, DB implemented an AI-powered spare part search solution designed to streamline and expedite the parts identification process.
This AI-driven solution is now a standard tool used by over 10,000 technicians within DB’s repair and maintenance teams, enabling them to find the required spare parts in just a few seconds. By automating the search process, DB has significantly reduced search times, leading to an extraordinary time savings of 16,800 person-days annually.
This optimization has greatly improved productivity, allowing technicians to focus on more critical tasks and enhancing overall efficiency in DB’s maintenance operations.Source.
Enhancing Spare Part Search Efficiency with AI at ÖBB
Austria’s national railway corporation, ÖBB, aimed to enhance its operational efficiency and service capabilities after being inspired by the success of AI-powered spare part search solutions at Deutsche Bahn (DB). ÖBB chose to include an AI-driven spare part search solution into their maintenance operations in order to expedite parts identification in response to issues including long search times, an aging workforce, and a rising number of materials.
This AI-powered solution is currently being used by about 800 ÖBB personnel, greatly cutting down on the amount of time spent looking for replacement components. The system has been warmly accepted, and preparations are now underway to expand its use across more teams due to its excellent operational impact. Source.
Simplifying Spare Parts Management with AI at ConMoto Consulting
ConMoto Consulting has successfully leveraged AI to streamline decision-making processes in spare parts management. By integrating AI technology, they have optimized critical tasks such as determining which parts to stock and ensuring accurate identification of parts. This advanced system supports smarter, data-driven decisions by analyzing historical data, usage trends, and inventory patterns, reducing human error and improving overall inventory management efficiency.
With AI’s assistance, ConMoto Consulting can predict demand more accurately, minimize overstocking, and ensure the availability of the right parts at the right time. The integration of this technology has significantly enhanced the efficiency of spare parts management, providing more informed decision-making processes and improving operational performance. Source.
How to choose the best AI solution for your data project?
In this white paper, we provide an overview of AI solutions on the market. We give you concrete guidelines to choose the solution that reinforces the collaboration between your teams.
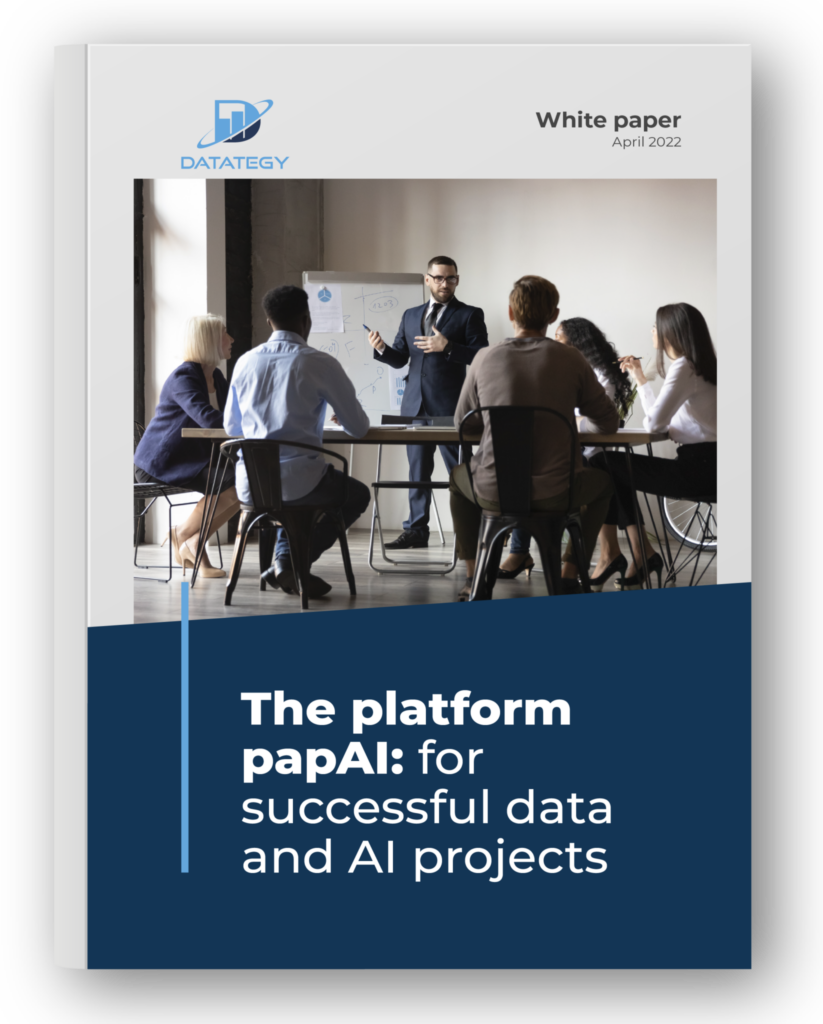
How papAI Helps to build Spare Part Search Powered by AI?
papAI is a comprehensive artificial intelligence solution made to optimize and simplify processes in a variety of sectors. It offers strong data analysis, predictive insights, and process automation capabilities by fusing state-of-the-art machine learning and artificial intelligence technology.
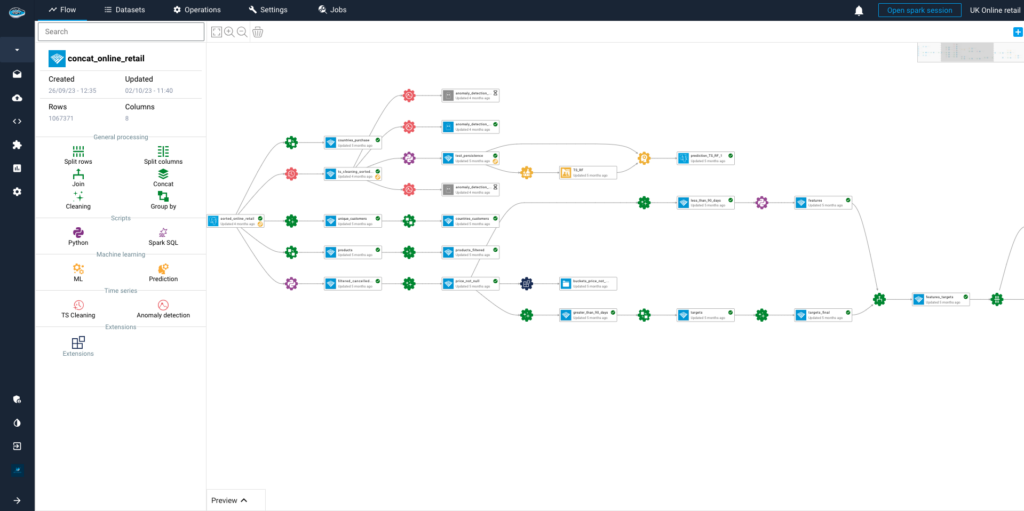
Here’s an in-depth look at the key features and advantages of this innovative solution:
Customizable AI Models
The capacity of papAI to offer very adaptable AI models suited to the particular requirements of an organization’s spare part search is one of its main advantages. The system starts by gaining knowledge from your current data, such as maintenance trends, part specs, and historical records. PapAI can create machine learning models that are exactly in line with your company’s spare parts inventory by evaluating this data.
Even in intricate settings with substantial inventory, this customization guarantees that the AI can recognize parts more accurately. The models get better with time as more data is analyzed, providing more accurate search results. This feature makes the AI system a long-term asset by ensuring that it becomes more effective and efficient as it learns from real-time interactions.
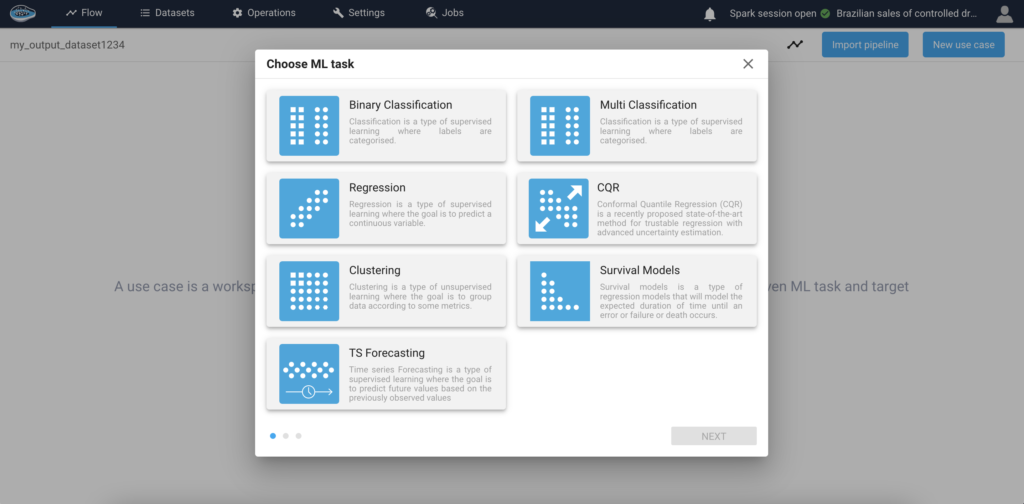
Seamless Data Integration
papAI is excellent in integrating with an organization’s current IT infrastructure smoothly. An ERP system, inventory management software, or other business tools may obtain the most recent spare part information thanks to papAI’s seamless data synchronization. Because of this integration, less manual data entry is required, and mistakes that may occur from disparate systems are reduced.
Through integration with many databases and other systems, papAI offers a consolidated view of spare parts data, simplifying the tracking of order histories, usage, and availability. The real-time data flow guarantees that AI-powered searches consistently represent the current condition of your parts inventory and that your inventory management and maintenance systems stay in sync.
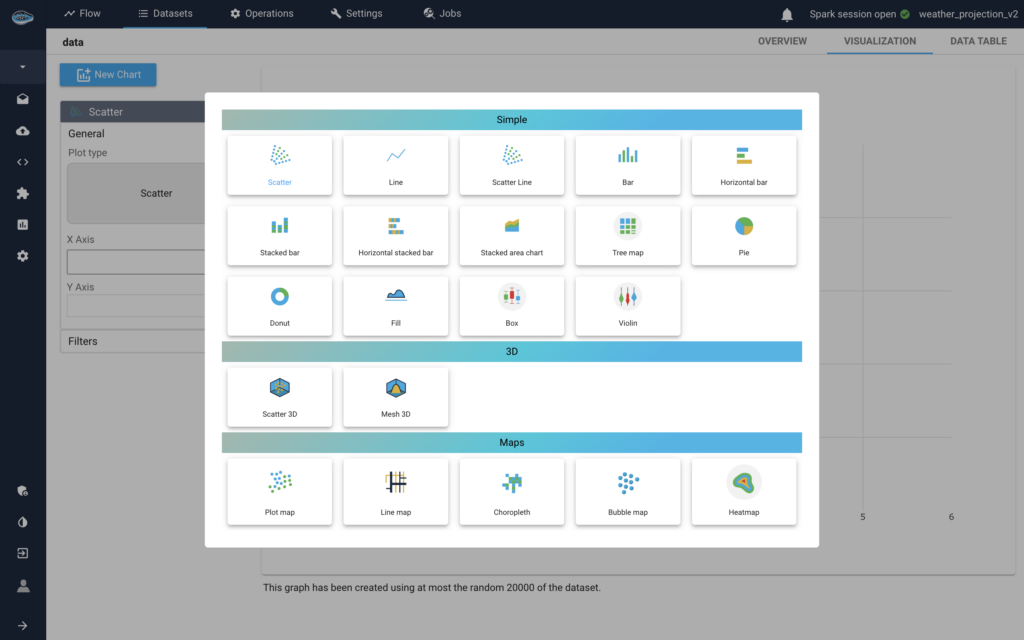
Predictive Analytics
The use of predictive analytics to spare component management is one of papAI’s most notable features. papAI is able to predict the future need for particular components by examining maintenance records, consumption trends, and other pertinent data. By minimizing the chance of overstocking or running out of essential components, this predictive capacity assists businesses in optimizing their inventory.
For example, the system may automatically modify inventory levels to guarantee that there is enough stock on hand when needed if it notices a trend of increasing demand for particular parts during particular maintenance cycles. Predictive analytics also helps businesses manage their spare part needs ahead of time by predicting when components may need to be replaced or serviced. As a result, inventory management becomes more proactive.
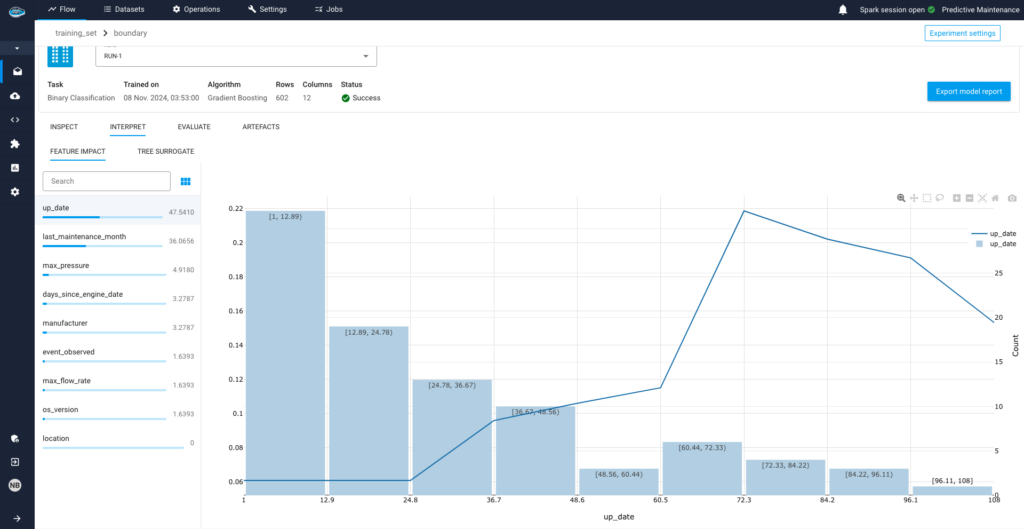
Create Your Own AI-Powered Spare Part Search with papAI
You may improve decision-making, cut down on part search time, and guarantee that your inventory is constantly in line with current demand by putting papAI into practice. papAI provides a customized, scalable solution to match your specific requirements, whether you’re managing intricate component inventories or need to increase maintenance efficiency.
Ready to transform your spare part search process? Book a demo today to see how papAI can streamline your operations and boost productivity. Let us show you how easy and powerful AI can be in managing your spare parts.
AI-powered spare part search systems streamline the identification process by leveraging machine learning algorithms and natural language processing. These technologies enable the system to process large amounts of data quickly and accurately, reducing search time from minutes to seconds
The main challenges include data quality and availability, as AI systems require clean, organized, and comprehensive data to function effectively. Many organizations face difficulties with outdated or inconsistent data, which can lead to inaccurate search results
- Key technologies include machine learning models, natural language processing (NLP), computer vision, and predictive analytics. NLP helps process and understand user queries, while machine learning allows the system to learn and improve over time. Computer vision enables the identification of parts through images, and predictive analytics forecasts future needs, all of which contribute to more accurate and efficient spare part searches.
- As industries grow and supply chains become more complex, traditional manual search methods are no longer sufficient. AI-powered spare part search offers a scalable, fast, and accurate solution to manage increasingly large and intricate inventories.
Interested in discovering papAI?
Our AI expert team is at your disposal for any questions
Datategy + Nedd Technologies: First Center of AI Excellence in USA
Datategy + Nedd Technologies: First Center of AI Excellence in...
Read MoreHow AI Drives the Evolution of Industry 4.0
How AI Drives the Evolution of Industry 4.0 Today, Industry...
Read MoreOptimize RAG Systems Like a Pro with Fine-Tuning
Optimize RAG Systems Like a Pro with Fine-Tuning As artificial...
Read More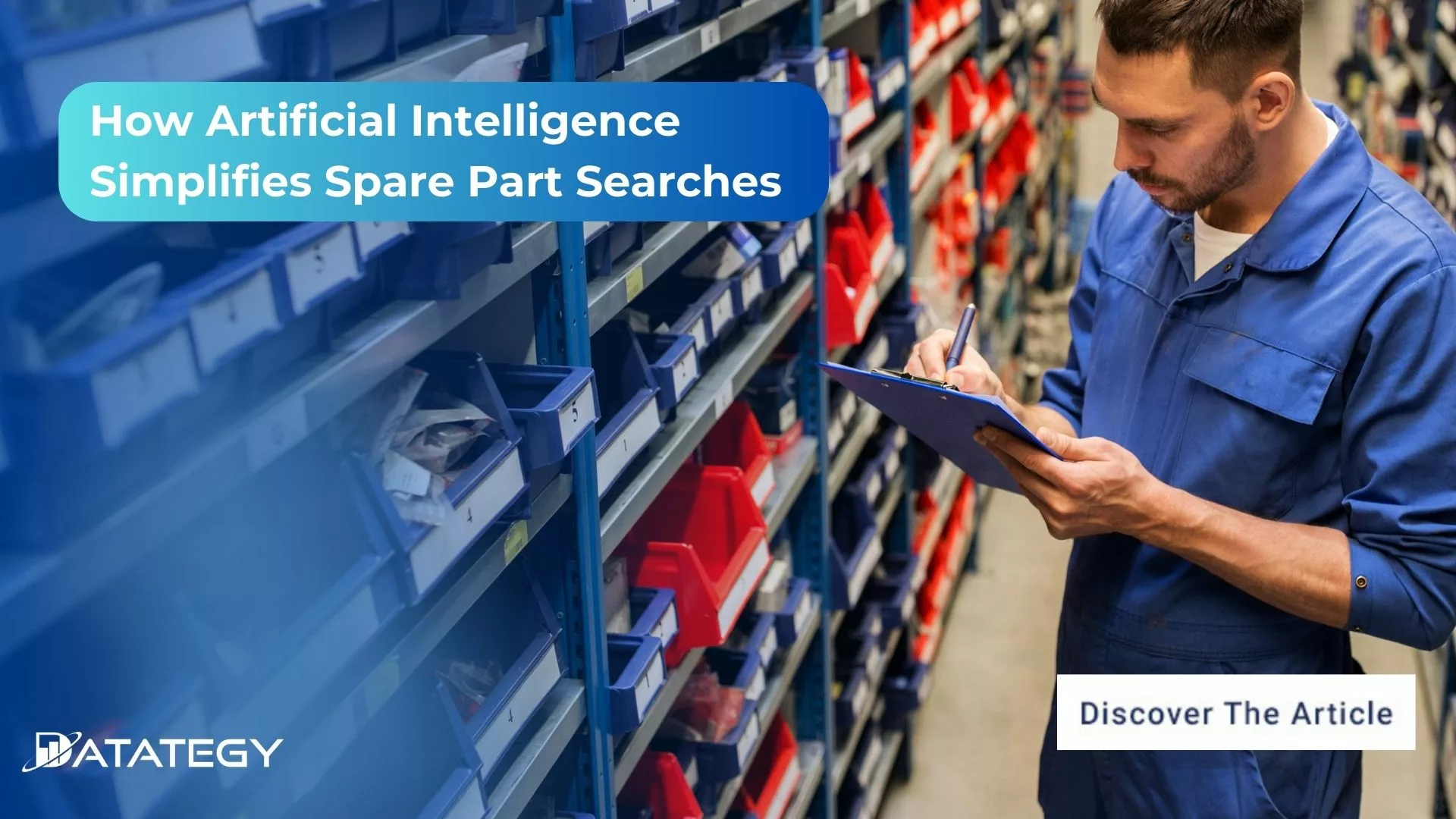
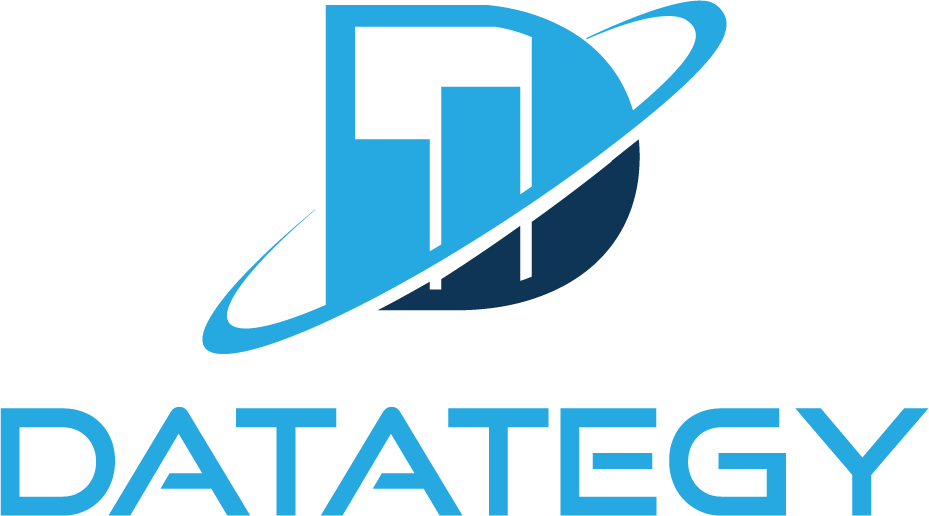