Scaling RAG Systems in Financial Organizations Artificial intelligence has emerged...
Read MoreHow AI Transforms Quality Control in Modern Manufacturing?
Table of Contents
ToggleQuality control is a cornerstone of the manufacturing industry, regardless of whether the end product is high-end or budget-friendly. Standardization is synonymous with successful sales and business growth.
Artificial intelligence (AI) has emerged as a powerful tool for enhancing quality control processes. By leveraging various technologies, AI can significantly improve the accuracy and efficiency of quality inspections.
Gartner predicts that by 2025, 50% of manufacturers will rely on AI-driven insights for quality control. This indicates a significant shift towards AI-powered solutions to enhance quality assurance processes.
Find out how papAI can improve the deployment of AI projects in the Manufacturing Industry.
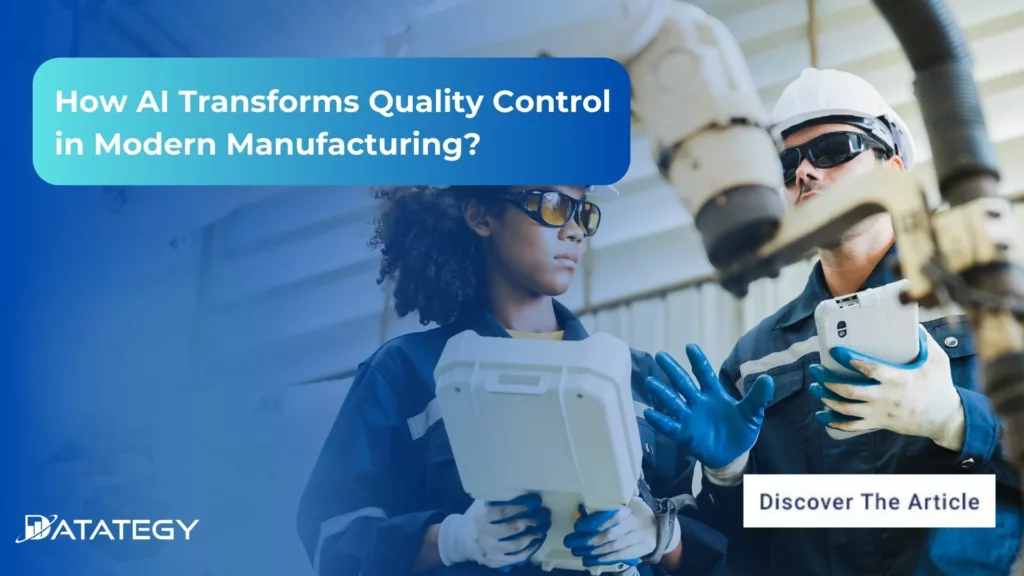
In this article, we will explore how artificial intelligence (AI) transforms Quality Control and examine best practices to implement it.
What Does Quality Control Mean?
The term quality control describes a methodical procedure intended to guarantee that goods and services fulfill predetermined requirements for performance, dependability, and quality. It includes a number of inspections, tests, and assessments carried out at different phases of manufacturing in order to find and get rid of flaws or irregularities. Quality control ensures that the final product meets customer expectations and legal requirements while protecting the integrity of the manufacturing process by strictly adhering to predetermined benchmarks.
Quality control is the foundation of any successful business, reducing waste and production errors while promoting customer satisfaction and trust. It uses both manual inspection and cutting-edge technologies, like artificial intelligence, to evaluate enormous volumes of data and identify possible problems instantly.
How Quality Control Has Changed Over the Time?
As technology has advanced and manufacturing processes have become more complex, quality control has undergone a radical transformation. Initially, quality control was primarily done by hand, using trained inspectors to check products for flaws and irregularities. Although somewhat successful, this time-consuming method was constrained by inefficiencies and human error.
The introduction of digital technologies and automation in recent decades has further transformed quality control. These days, real-time monitoring systems, predictive analytics, and artificial intelligence (AI) are essential for identifying flaws, anticipating possible problems, and guaranteeing continuous improvement. These developments enable a more proactive and effective approach to quality management by enabling manufacturers to analyze enormous volumes of data generated during production processes.
The Key Benefits of Artificial Intelligence (AI ) in Quality Control
Monitoring and Identifying Problems in Real Time: Real-time production line monitoring is one of AI’s most notable advantages. These systems continuously assess the manufacturing process using integrated sensors and AI models, spotting possible problems before they become serious. In addition to aiding in defect detection, real-time monitoring provides information about the underlying causes, enabling prompt remedial action. This proactive strategy reduces downtime, guarantees smooth operations, and stops faulty products from piling up. Manufacturers can maximise production efficiency and uphold high standards by resolving issues as they emerge.
Improved Precision and Effectiveness: The precision and effectiveness of quality control procedures are greatly increased by artificial intelligence (AI). Conventional manual inspection techniques frequently lack consistency and are subject to human error, particularly in large-scale manufacturing. AI systems, on the other hand, use sophisticated algorithms to precisely analyse enormous volumes of data produced during production.
This lessens the possibility that faulty products will make it to market by guaranteeing that even the smallest flaws or irregularities are found. Furthermore, AI-powered tools can perform inspections much more quickly without sacrificing detail because they work at speeds far faster than humans. Quality control becomes a more dependable and efficient operation thanks to the dual benefits of increased accuracy and quick processing.
Predictive Maintenance for Seamless Operations: AI is excellent at predictive maintenance because it can predict equipment failures by evaluating both historical and current data. AI systems anticipate when machinery may need maintenance, preventing unplanned breakdowns rather than depending on reactive repairs or regular schedules. This feature increases equipment longevity and decreases production halts, resulting in more economical and effective operations. Manufacturers can optimize resources and maintain consistent product quality by incorporating predictive maintenance into quality control.
Resource Optimisation and Cost Reduction: Artificial intelligence lowers the need for manual labor by automating quality control procedures, which saves money and time. Early defect detection reduces material waste because defective products are found and fixed before large sums of money are spent. Furthermore, AI-driven efficiency lowers operating costs, including maintenance and energy use. By taking a comprehensive approach to cost reduction, manufacturers can increase profitability without sacrificing quality standards. In the end, AI gives businesses a competitive edge by balancing financial sustainability and quality assurance.
How to implement AI in Quality Control within your Organizations?
Choose the Right AI Tools
Choosing the best AI tools and technologies to meet your unique needs comes next after determining which areas need improvement. You might need to use technologies like computer vision, machine learning, or predictive analytics, depending on your goals. For example, machine learning models can be used to predict failures or optimize workflows, while computer vision is especially helpful for visual inspections and defect detection. Tools for predictive analytics are useful for predicting maintenance requirements. In order to ensure seamless integration and scalability for future requirements, you must select tools that are compatible with your current infrastructure and systems.
Data Gathering and Infrastructure Configuration
Since data is the cornerstone of any AI implementation, collecting pertinent data is essential for training your AI models. This comprises sensor data, historical production data, and any additional manufacturing-related inputs. Accurate, clean, and structured data are essential because AI models depend on high-quality data to work well. Install the infrastructure required to facilitate data gathering and archiving, making sure that it integrates with your current ERP or MES systems. In order to enable real-time monitoring and analysis, it might also be necessary to install IoT sensors on production lines to collect data in real-time on variables like temperature, pressure, or vibration.
Customization and Model Training
Training AI models that are specific to your quality control procedures comes next after the data has been gathered and processed. For computer vision applications, this entails feeding the data into deep learning models or machine learning algorithms. For instance, computer vision systems will need sizable datasets of labeled images to detect product flaws, whereas machine learning models can be trained to identify defects based on historical data. In order to make sure that the AI models are tailored to your manufacturing environment and the particulars of your products, customization is essential. To make sure the models function well in practical settings, testing, validation, and iteration are crucial.
Pilot Testing and Validation
Perform a pilot test to assess the system’s performance in a controlled setting prior to implementing AI systems throughout your whole production line. To validate the AI models and evaluate their effect on quality control, the pilot should concentrate on a small section of your production process. Keep an eye on the AI system’s capacity to identify flaws, streamline processes, and forecast maintenance requirements during this stage. To find any problems or potential areas for improvement, get input from operators and quality control teams. Prior to scaling the solution, make the required modifications to the AI models or system integration.
What are the Main Technologies Behind the use of AI in Quality Control?
Predictive Analytics: Time Series Forecasting and Anomaly Detection
AI quality control predictive analytics uses anomaly detection models and time-series forecasting to anticipate possible production process failures. To examine past production data and forecast future trends, time-series forecasting methods like LSTM (Long Short-Term Memory) networks and ARIMA (AutoRegressive Integrated Moving Average) are used.
Based on factors like temperature, humidity, or equipment wear, these models assist in identifying departures from typical patterns, allowing the system to forecast when a quality problem is likely to arise. Unusual patterns in the production data can also be found using AI algorithms for anomaly detection, such as statistical approaches like clustering (e.g., k-means) or more sophisticated strategies like autoencoders. Predictive analytics, which continuously learns from incoming data, not only detects possible quality problems but also enables manufacturers to optimize maintenance schedules.
Machine Learning Models: Supervised, Unsupervised, and Reinforcement Learning
In AI-driven quality control, machine learning models cover a variety of methods, each with a distinct set of uses. Labeled datasets are necessary for supervised learning, which is commonly used for tasks like identifying defective versus non-defective products based on characteristics like size or color. For this task, algorithms like Random Forests, decision trees, and Support Vector Machines (SVM) are frequently used.
Conversely, when labeled data is limited, unsupervised learning is employed for anomaly detection and clustering. Similar product features can be grouped together and outliers that might point to flaws can be found using algorithms like K-means or DBSCAN (Density-Based Spatial Clustering of Applications with Noise). Although it is less frequently used in quality control.
Computer Vision: Advanced Image Processing and Feature Extraction
Computer vision, which is at the core of AI-driven quality control, uses sophisticated image-processing methods to examine products. The system takes detailed pictures of the products on the production line using high-definition cameras. After that, feature extraction algorithms are used to process these photos in order to find important components like edges, textures, and shapes.
These features are analyzed hierarchically using deep learning models, specifically Convolutional Neural Networks (CNNs), which simulate how people interpret visual information. The system can identify anomalies like cracks, scratches, or alignment problems because these networks are trained on enormous datasets of labeled images. The system can also confirm labels or serial numbers by using methods like optical character recognition (OCR).
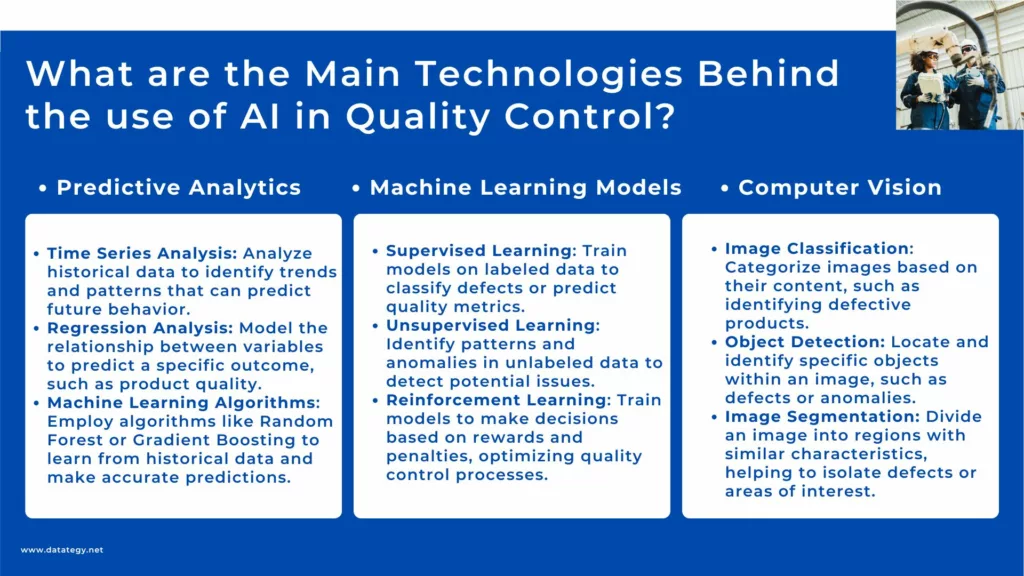
Main Technologies Behind AI in Quality Control
Real-world Examples of AI Enhancing Control Quality by Industry
Manufacturing Industry: Siemens
AI has been incorporated by Siemens into its production procedures, especially in the quality control sector. Siemens performs remarkably accurate product inspections on assembly lines using AI-powered computer vision systems. These systems are trained to find even the smallest flaws and irregularities that human inspectors might miss.
Real-time analysis of high-resolution photos and videos enables the AI to spot imperfections like dents, scratches, and misalignments. In addition to guaranteeing better product quality, this also drastically lowers the quantity of faulty goods that are released onto the market. The ability of the AI systems to work continuously without getting tired allows them to maintain a constant level of scrutiny, which raises overall productivity. Furthermore, patterns and the underlying causes of flaws can be found using the data gathered from these inspections, enabling operations.
Pharmaceuticals and Healthcare: Pfizer
Quality control is crucial in the pharmaceutical sector because of the strict regulations and possible threats to patient safety. Pfizer uses AI to improve the quality of drug packaging by using algorithms to check labels and packaging information. These algorithms can identify and contrast printed information with a database of accurate information.
They can spot inconsistencies that could endanger public safety, like inaccurate labels, missing information, or flaws in the packaging. Pfizer guarantees greater accuracy and efficiency by automating this process as opposed to manual inspections. The AI systems’ ability to process a high volume of products quickly cuts down on the time needed for quality checks and speeds up the production process as a whole. Additionally, it ensures adherence to regulations.
Food Industry: Nestlé
Nestlé improves food safety and quality control by using AI-powered sorting systems. These systems identify contaminants and foreign objects in food products using sensors and machine learning algorithms. AI systems, for instance, can recognise and eliminate materials like plastic, metal, or other contaminants that might have inadvertently made their way onto the production line while making chocolate bars.
Only safe and superior products are delivered to customers thanks to this real-time detection and removal procedure. Additionally, the sorting process is optimised, waste is decreased, and overall efficiency is increased with the aid of AI technology. Nestlé can uphold high standards of food safety and quality by utilising AI, which is essential for retaining customer trust and enhancing the company’s reputation.
Automotive Industry: Toyota
Quality control is essential in the automotive sector to guarantee vehicle performance and safety. Toyota uses machine vision systems and robotic arms to perform highly accurate vehicle component inspections. These AI-powered devices are capable of carrying out thorough examinations of parts like electrical systems, body panels, and engine parts. They are able to identify flaws that might jeopardise the vehicle’s performance or safety, like cracks or misalignments.
By analysing enormous volumes of data from numerous sensors and cameras, the AI systems are able to provide a thorough evaluation of every element. Toyota can increase accuracy and consistency, cut down on inspection time, and boost overall production efficiency by automating these inspections. Additionally, the knowledge gathered from AI-driven quality control aids in pinpointing areas that require improvement in the manufacturing process, leading to continuous enhancements in product quality.
What Challenges need to be Overcome before Implementing AI-Powered Quality Control?
Costs and ROI: The initial investment required for new technologies is a significant barrier, particularly for small and medium-sized businesses. It can be intimidating to invest in sensors, data integration tools, and AI-powered systems. Organizations must, however, consider these expenditures as long-term investments that eventually lower costs and improve operational efficiency.
Ensuring Data Quality for Reliability: Implementing cutting-edge technologies like AI-powered quality control presents additional challenges related to data management and quality. Data inconsistent, lacking, or erroneous can compromise the efficacy of machine learning and artificial intelligence models. To guarantee accuracy, businesses must set up strong procedures for data collection, storage, and analysis. Reliability can be increased by using sophisticated analytics tools and routinely auditing data inputs.
How to choose the best AI solution for your data project?
In this white paper, we provide an overview of AI solutions on the market. We give you concrete guidelines to choose the solution that reinforces the collaboration between your teams.
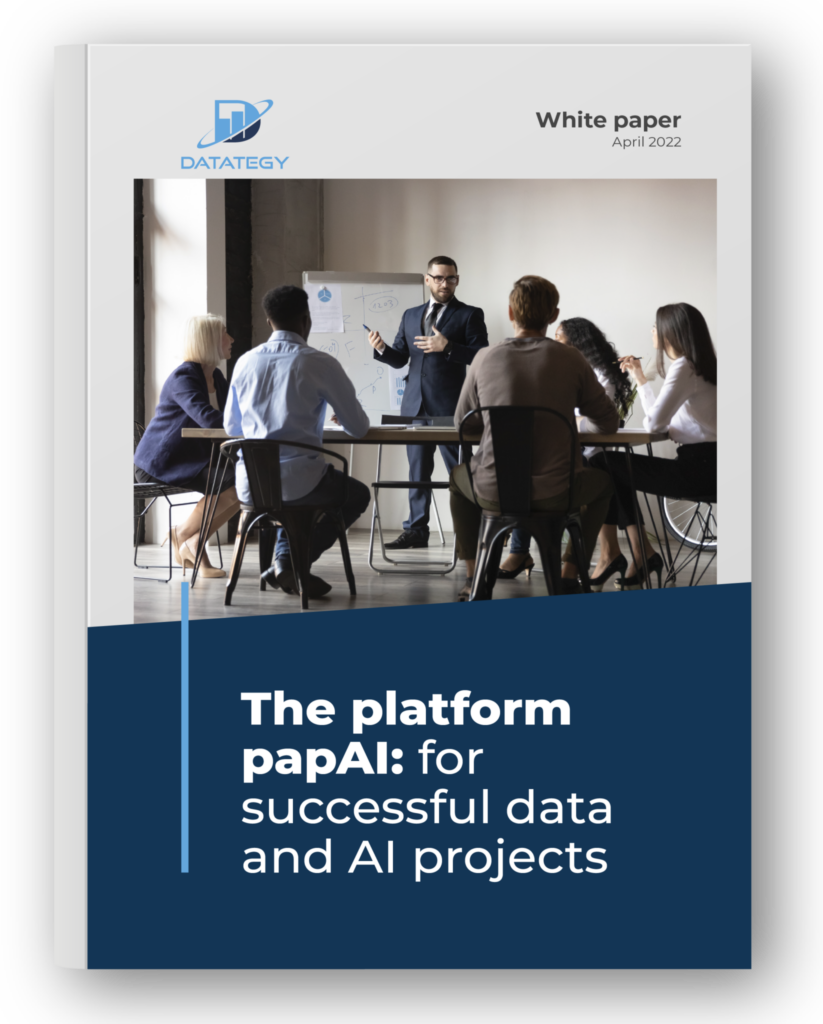
How papAI Helps Build Effective AI-Powered Quality Control?
papAI is an all-in-one artificial intelligence solution designed to streamline and optimize procedures across multiple industries. By combining cutting-edge machine learning and artificial intelligence technologies, it provides powerful capabilities for data analysis, predictive insights, and process automation.
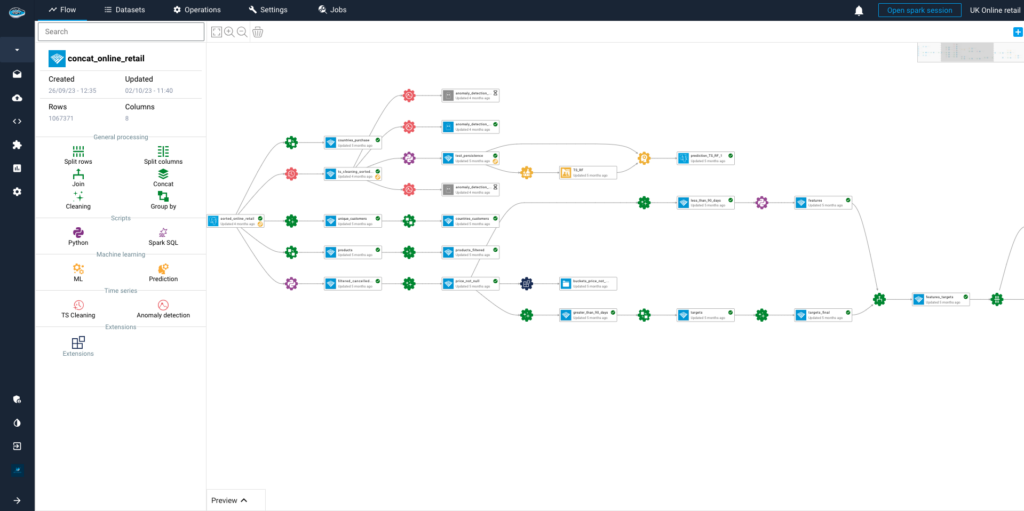
Here’s an in-depth look at the key features and advantages of this innovative solution:
Data Integration and Streamlining
Production logs, sensor data, and old quality control records are just a few of the data sources that papAI first seamlessly integrates with. It gathers enormous volumes of data from multiple systems (such as ERP and MES) and compiles it into a single platform. Accurate analysis and decision-making depend on papAI having real-time access to all pertinent production data, which is ensured by this integration.
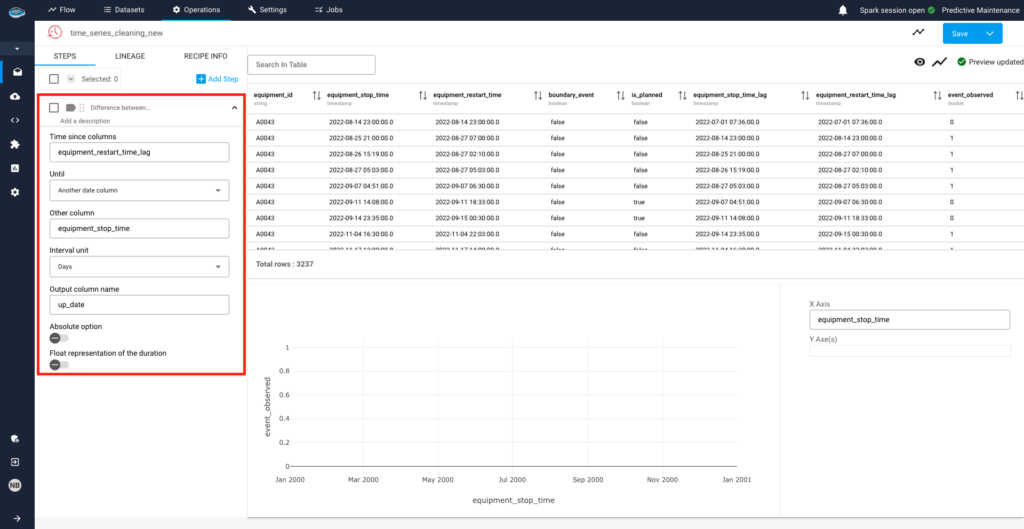
Advanced Predictive Analytics and Real-time Insights
The intelligent predictive analytics capabilities of papAI are among its primary attributes. It makes use of both historical and current data to spot trends and anticipate possible flaws or equipment failures before they happen. papAI forecasts possible problems in the manufacturing process by analyzing production variables like temperature, pressure, and vibration using machine learning algorithms. By using these predictive insights, businesses can avoid quality problems by taking proactive measures like planning maintenance or modifying production parameters.
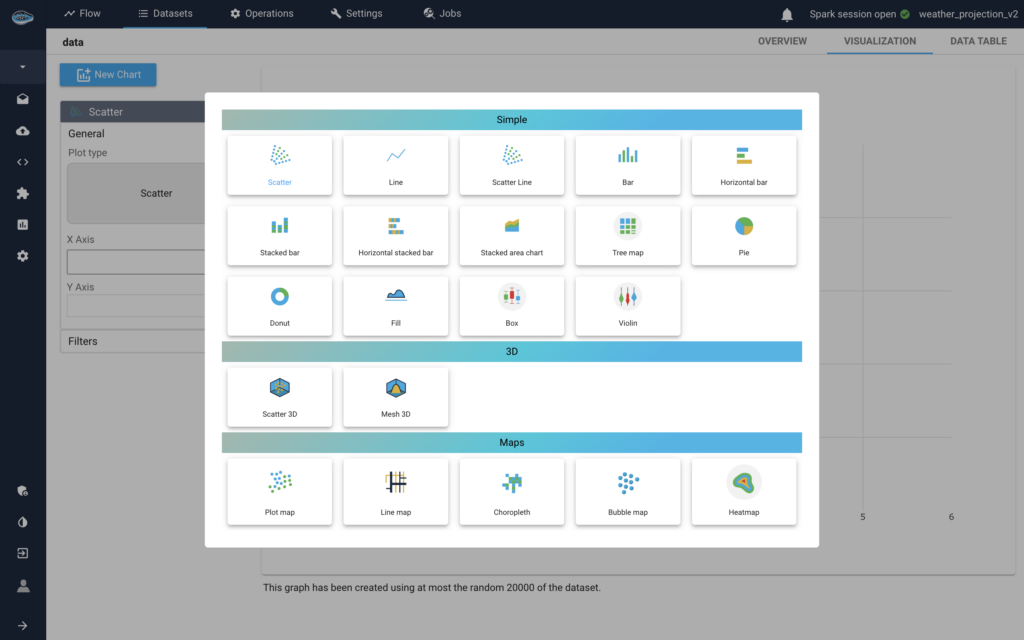
Smooth Scalability in Different Production Lines
papAI is made to easily scale across various manufacturing environments and production lines. PapAI can adjust to a variety of production requirements and configurations, regardless of whether your business runs a single production line or several lines spread across various locations. It makes sure that quality control is always effective, no matter how big or small, by automatically adapting to changes in production volume, product types, or operational procedures. Because it can grow with your operations and continuously provide value across a range of production setups, its scalability makes it the perfect solution for companies of all sizes. Because of its adaptability,
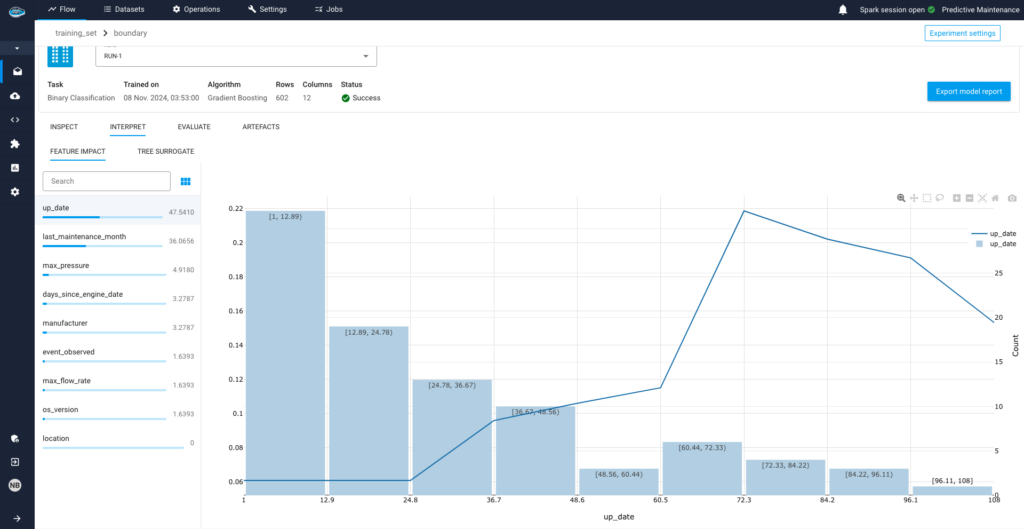
Create your Own AI-Powered Quality Control Model using papAI solution
papAI is transforming quality control by fusing state-of-the-art technologies such as machine learning, computer vision, and predictive analytics to produce a more intelligent and effective manufacturing process. Your quality control is always optimized thanks to papAI’s smooth integration with your current systems, real-time insights, and ongoing data learning. PapAI gives you the intelligence and tools to achieve your goals, whether they are to estimate maintenance requirements, decrease defects, or increase overall production efficiency.
Book a demo today and experience firsthand how our AI-powered solution can elevate your manufacturing operations and drive lasting improvements in product quality.
AI enhances accuracy by analyzing large datasets, detecting minute defects with precision. Unlike manual inspections, AI identifies issues faster and with greater consistency. Through machine learning and computer vision, it ensures high-quality products by minimizing human error, improving product quality, and optimizing manufacturing efficiency over time.
Predictive maintenance driven by AI analyses both historical and current data to predict equipment failures. Proactive repairs are made possible by this method, which lowers unscheduled downtime while preserving production quality. Predictive maintenance prolongs machine lifespans, minimises operational interruptions, and prevents equipment-related defects, all of which contribute to more dependable product output and more economical, efficient manufacturing.
- AI speeds up defect detection and automates inspections, which lowers labour costs. It maximises operational efficiency by reducing material waste and avoiding expensive rework. AI boosts profitability while upholding high standards of quality and guaranteeing sustainable production processes by increasing speed, accuracy, and decreasing downtime.
- By combining data from multiple systems onto a single platform, papAI improves quality control. Its predictive analytics allow for proactive action by anticipating flaws and equipment failures. PapAI is incredibly scalable and flexible enough to work in a variety of production settings. PapAI streamlines processes for efficiency and guarantees consistent quality with real-time insights and sophisticated machine learning.
Interested in discovering papAI?
Our AI expert team is at your disposal for any questions
How AgenticAI is Transforming Sales and Marketing Strategies
How AgenticAI is Transforming Sales and Marketing Strategies Agentic AI...
Read More“DATATEGY EARLY CAREERS PROGRAM” With Abdelmoumen ATMANI
“DATATEGY EARLY CAREERS PROGRAM” With Abdelmoumen ATMANI Hello, my name...
Read MoreIntroducing the Error Analysis Tree: A Smarter Way to Improve AI Models
Introducing the Error Analysis Tree: A Smarter Way to Improve...
Read More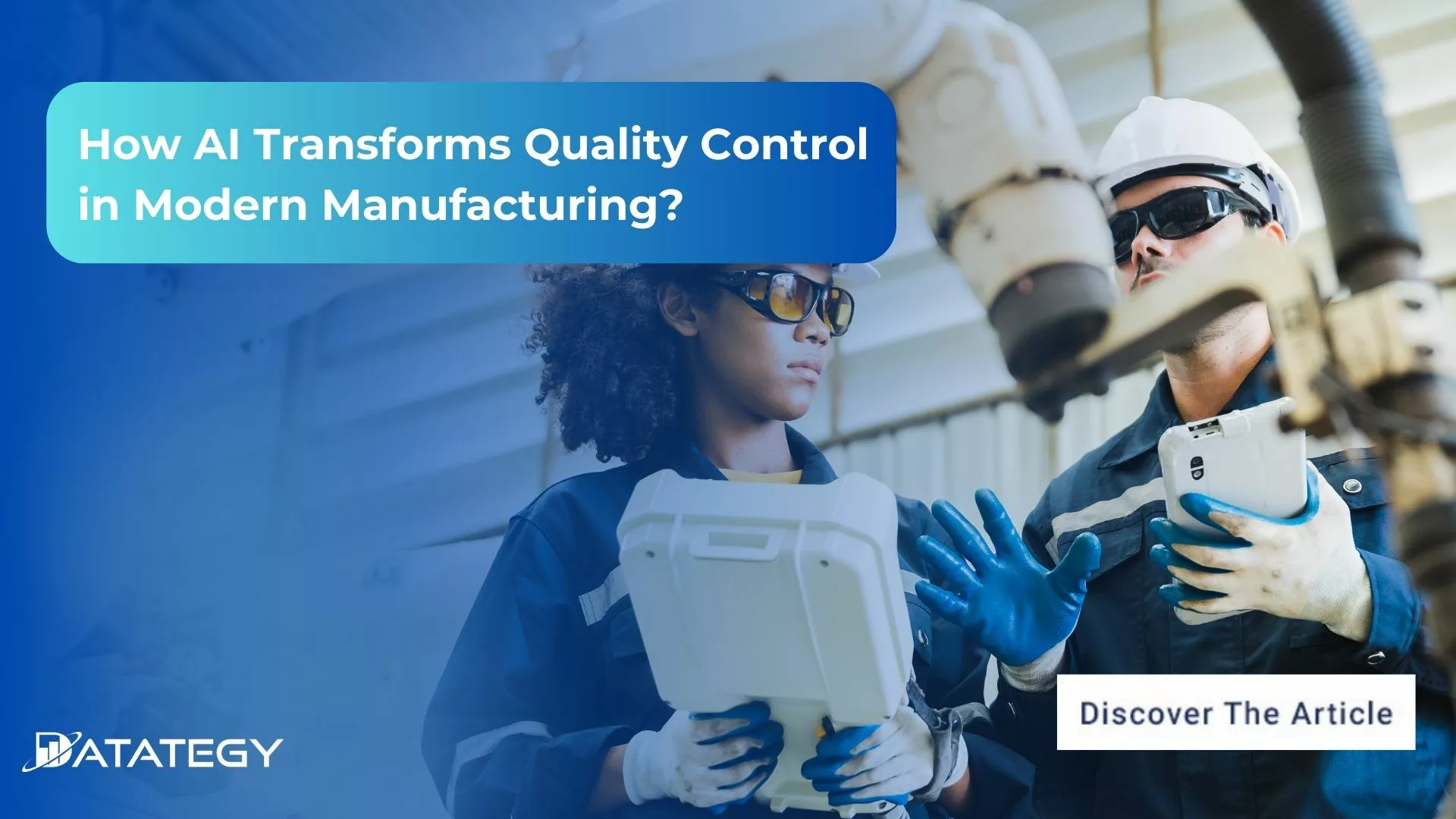
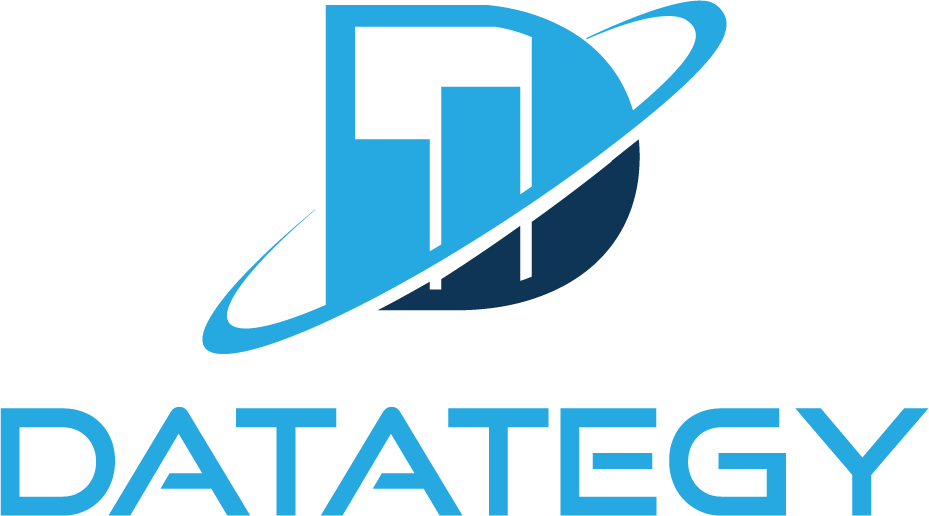