How Artificial Intelligence Simplifies Spare Part Searches Artificial intelligence is...
Read MoreAI in Action:
Real-World Applications of AI in Sales
Table of Contents
ToggleArtificial Intelligence (AI) is changing the way sales teams interact, anticipate, and execute their work. It is no longer just a trendy term. AI has the power to improve every consumer interaction and use predictive analytics to identify patterns early.
A Dialpad survey found that 79% of sales teams report improved job performance when using AI tools. AI provides valuable assistance with tasks, allowing reps to focus on strategic selling.
Find out how papAI can improve the deployment of AI projects in Sales Teams.
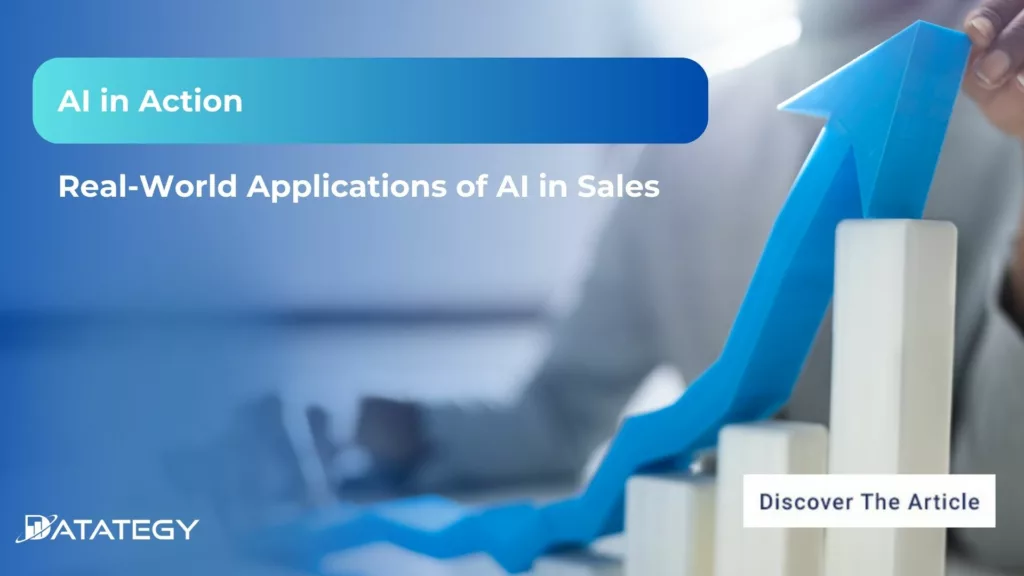
In this article, we will examine how AI can elevate your team’s sales efforts and become its MVP.
What does Artificial Intelligence Mean?
The term artificial intelligence (AI) describes the process of creating computer systems that are capable of carrying out operations that normally call for human intelligence. This covers an extensive variety of skills, such as learning, problem-solving, comprehending natural language, and situational adaptation. AI has the ability to significantly improve and expand upon a number of sales-related processes, including lead generation, customer engagement, and more.
How did AI Emerge in the Sales Sector?
The notion of artificial intelligence (AI) in sales was mainly theoretical at the beginning of the 1980s; it was more of a concept than a useful tool. Sales teams had little access to technology other than rudimentary CRM systems, and they mainly depended on conventional techniques. Although some progressive businesses experimented with basic AI applications for customer segmentation or lead scoring, widespread adoption remained elusive because of technological limitations and doubts about AI’s ability to improve sales.
The sales industry had a transformation in the late 20th and early 21st centuries due to developments in data analytics and processing power. CRM systems advanced in sophistication, improving data gathering and analysis. In the meantime, machine learning and other AI technologies gained popularity, opening up new avenues for task automation and consumer behavior analysis. Despite these developments, AI’s place in sales has remained relatively niche, and many businesses are still hesitant to fully adopt AI-driven solutions because of worries about reliability, cost, and complexity.
But by 2023, artificial intelligence (AI) had revolutionized every step of the sales process and was a mainstay of contemporary sales tactics. AI-powered platforms and tools are now essential resources for sales teams around the globe, helping with everything from lead generation to customer retention. Sales professionals can now make data-driven decisions, personalize interactions at scale, and anticipate customer needs with never-before-seen accuracy thanks to advanced predictive analytics and machine learning algorithms.
What are the Benefits of Using AI in Sales?
Enhanced Efficiency: AI automation handles repetitive tasks like data entry, email scheduling, and follow-up reminders, which streamlines sales processes. AI allows sales representatives to focus on higher-value tasks, like developing relationships and closing deals, by automating these repetitive tasks.
In addition to increasing productivity, this enhanced efficiency makes it possible for sales teams to handle higher lead and opportunity volumes, which in turn propels revenue growth and business success.
Predictive Analytics: AI-driven predictive analytics forecasts future sales trends and anticipates customer behavior by utilizing machine learning algorithms and historical data. Artificial intelligence models can find trends and correlations that provide predictive insights by examining historical performance and market dynamics.
With the help of these projections, sales teams are better equipped to take data-driven decisions, modify their plans on the fly, and seize new opportunities. Sales organizations may stay ahead of the curve, spot possible hazards, and take proactive measures to resolve problems with the help of predictive analytics. This leads to long-term growth and a competitive edge.
Better Customer Insights: AI systems examine data from various sources about customers to learn more about their preferences, habits, and problems. Artificial Intelligence (AI) helps sales teams better understand their customers and adjust their approach by gathering and evaluating data from multiple touchpoints.
Sales representatives can foresee client needs, proactively address issues, and present tailored solutions that appeal to customers by utilising these insights. Sales teams can establish more solid relationships, encourage greater engagement, and eventually boost revenue and sales with the help of this deeper understanding of their customers.
Competitive Advantage: Organisations can become more successful in the market by utilizing AI in sales. Sales teams can take advantage of new opportunities, recognize emerging trends, and adjust to shifting market conditions with the help of AI. Businesses can increase productivity, optimize sales processes, and achieve superior outcomes by utilizing AI-driven insights and automation.
This helps companies stand out from rivals, provide outstanding value to clients, and promote long-term development and success. In today’s cutthroat market, companies can stay ahead of the curve, outperform rivals, and achieve long-term success by implementing AI in sales.
Real-World Use Cases in Sales Field
Sales Forecasting
Predictive analytics, market trends, and historical data are used in AI-driven sales forecasting to project future sales performance. Sales teams can make data-driven decisions, allocate resources wisely, and modify their tactics in response to shifting market conditions when they are given precise forecasts. Sales teams can predict demand, spot opportunities, and reduce risks with the help of AI-driven sales forecasting, which eventually leads to increased revenue and successful business operations.
Customer Segmentation and Targeting
Using customer data, AI-powered customer segmentation creates discrete groups according to characteristics like interests, behaviours, and demographics. Sales teams can better target their audience and increase conversion rates by segmenting their customer base and adjusting their marketing and sales strategies to suit the needs and preferences of each group. Sales teams can better understand their customers, spot relevant opportunities, and create individualised experiences that appeal to each segment by using AI-driven customer segmentation.
Sales Performance Analytics
Using artificial intelligence (AI), sales performance analytics can track key performance indicators (KPIs), spot trends, and deliver useful information to boost sales. Through the examination of metrics like conversion rates, average deal size, and sales velocity, sales teams can pinpoint opportunities for enhancement and refine their approaches to yield superior outcomes. Sales teams can better understand their performance, pinpoint areas for development, and take proactive steps to improve outcomes with the help of AI-driven sales performance analytics.
Route Optimization and Sales Territory Mapping
To optimise sales territories and routes, AI algorithms examine market potential, customer demographics, and geographic data. Sales teams may increase their productivity and efficiency by determining the most profitable regions and planning the best travel routes. This will eventually result in more sales and income. Sales teams can optimize their territories and routes, boost productivity, and achieve better outcomes with the help of AI-driven sales territory mapping and route optimization.
AI and Machine Learning for Sales:
The Key to Unlocking Growth Potential
The development of artificial intelligence (AI) has significantly changed the sales industry, which has witnessed a remarkable evolution throughout time. The way companies approach sales processes and strategies has been completely transformed by AI. In this white paper, we will examine the concept of AI in sales, its historical evolution, and the advantages it provides for companies.
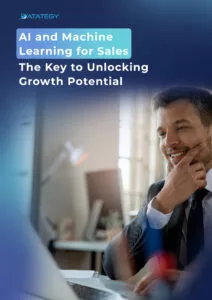
How you can Implement AI in your Sales Teams?
1- Evaluation of Needs: Before integrating AI into your sales teams, it’s critical to conduct a comprehensive evaluation of the requirements and problems facing your company. Determine which particular domains, like lead generation, customer segmentation, or sales forecasting processes, AI can have a big impact on.
Gain a better understanding of the challenges and goals of your sales team so that you can customize AI solutions to meet their specific needs and optimize implementation benefits.
2- Examine AI Solutions: Look into the different AI options on the market that fit your goals and needs. Seek out systems or resources that provide functions like natural language processing, sales automation, or predictive analytics. Consider features, scalability, and ease of integration with your current systems when weighing your options.
3- Pilot Testing Phase: To determine whether implementing AI in the real world is effective, begin with a phase of pilot testing. To test the AI solution and get user feedback on its usability, functionality, and impact on workflows, choose a small team or a particular use case. Use the knowledge gathered from the pilot test to improve the implementation strategy and deal with any problems or obstacles that crop up.
4- Measurement and Analysis: To determine how implementing AI will affect sales performance, set up specific KPIs. To evaluate the success of AI-driven initiatives, monitor metrics like lead conversion rates, customer acquisition costs, and sales pipeline velocity.
To assess performance trends, pinpoint areas for success, and identify areas for improvement, use data analytics and reporting tools. Review and assess performance metrics on a regular basis to make sure AI implementation is producing the desired effects and producing observable business outcomes.
5- Expanding Up: Extend the use of AI in your sales teams after you’ve conducted pilot testing to confirm its efficacy in your processes. Provide resources and assistance to ensure a smooth expansion process, as well as instruction and direction to ensure new users are successfully onboarded.
Keep an eye on an AI solution’s scalability to make sure it can handle expanding demands and changing business needs. As you grow, keep improving and fine-tuning the AI implementation to guarantee optimal productivity and efficacy throughout your sales team.
Use Case: Car Sales Predictions with papAI Solution's TSForecasting Models
Context
We will see how papAI solution can be used in this use case to forecast the number of cars that each car dealer will require, and consequently, the volume of cars that will be sold.
1- Dataset
We are going to use an open-data database. This database includes the number of individual car orders, and it contains 79 weeks of daily data
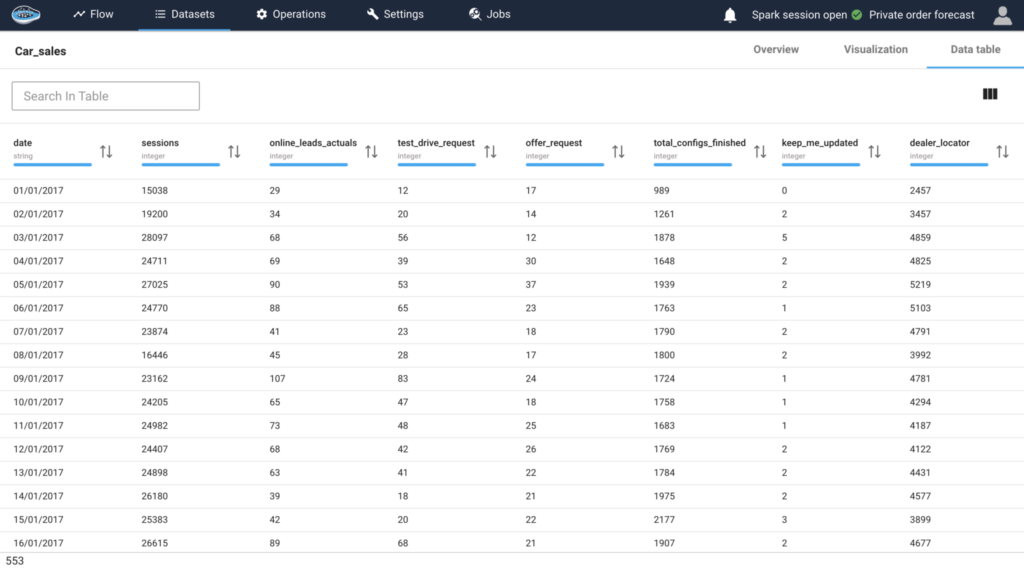
As we can see in the first few rows of the dataset above, the dataset has 8 columns which are as follows:
- Date : Datetime (DD/MM/YYYY)
- Sessions : Number of website visits on a specific day
- Online_leads_actuals : Number of visits to online shop (order online)
- Test_drive_request : Number of test drive requested
- Offer_request : Number of offers requested
- Total_configs_finished : Number of car configuration completed
- Keep_me_updated : Number of users asked to be kept updated
- Dealer_locator : Number of users who looked for a dealer location
- Private_orders : Number of times a private order has been made. This represents the total number of offline sales received. We should try to predict this field.
2- Data Preparation
Without requiring the manipulation of code, papAI solution enables the execution of general processing operations, thereby facilitating the processing and preparation of data. A distinct module is created specifically for processing time series data, should you wish to work with such information.
The date column in the database (previous image) is of type “string,” as you can see. Since this column contains dates, the first action to take in papAI is to convert the type of this column to the proper format of “date” by using the general processing cleaning.
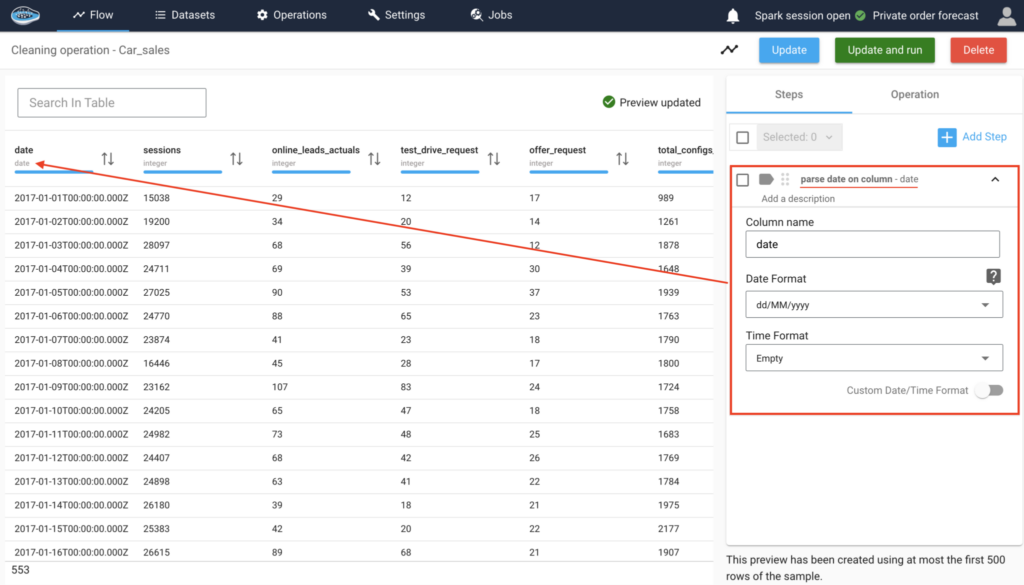
The datetime feature generation found in the TS Cleaning module of papAI Solution will be the second processing we use. Additional information from the date, such as the day of the week, month, weekday, and year, is extracted by this operation. This will enable us to have more features and improve the accuracy of our forecasting.
3-Machine Learning
Model Training
With papAI platform, multiple machine learning models can be run concurrently without the need for coding. The user can alter these settings if desired. These models are initially shown with Scikit-Learn’s default settings. papAI Solution gives us the option to select between Single series (Univariate) and Multiple series (Multivariate) when building a machine learning use case.
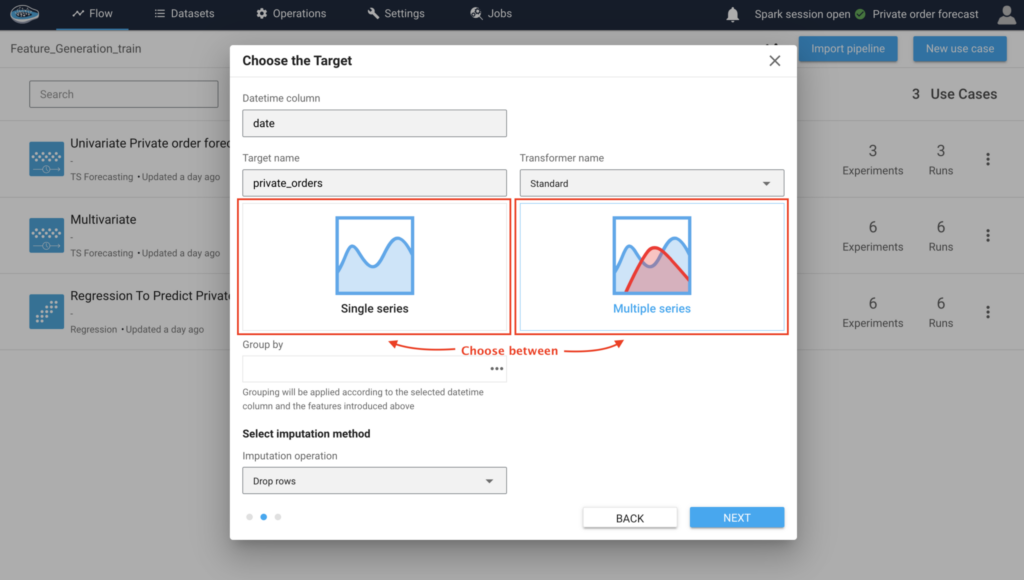
3.a) Univariate:
The TsForecasting univariate is to train a machine learning model by taking into account the target variable on the time series, so in this method, we will use only two columns of our dataset (Date and Private Order).
3.a.1) Data analysis:
After choosing Private Order as a target, and univariate as a series type, papAI automatically displays a visualization of the evolution of ‘Private order’ on the Temp.
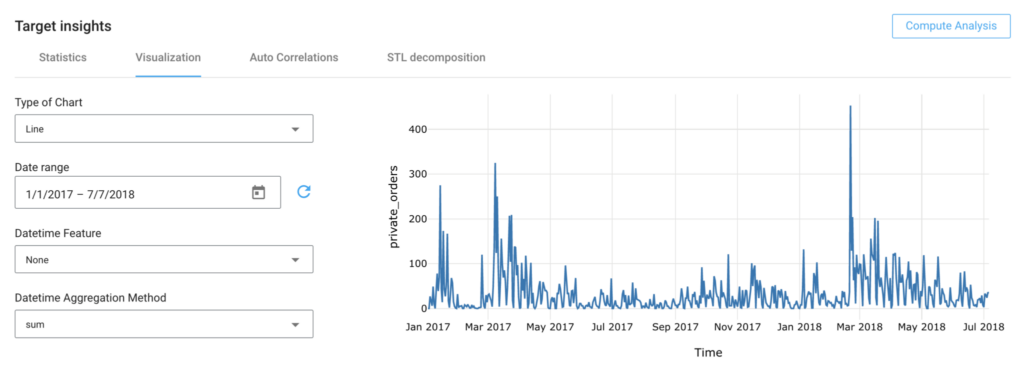
In the tab next to it, we can see an analysis of our database.
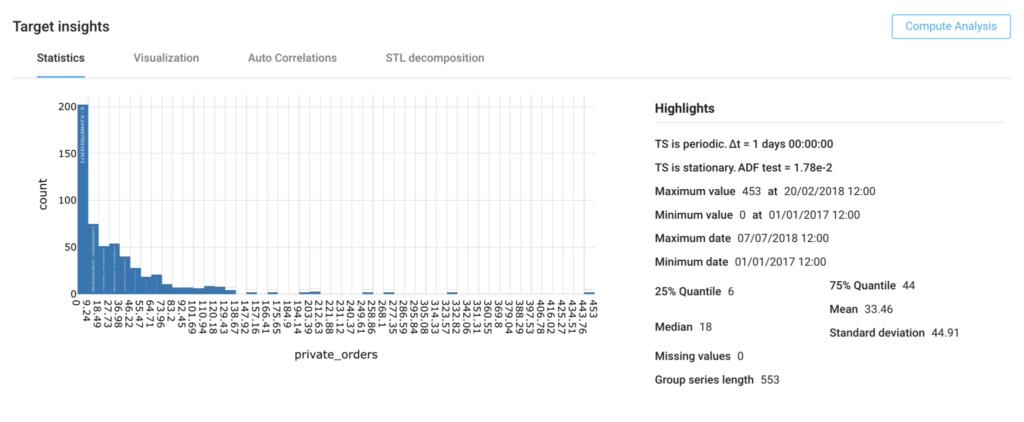
The statistics generated give us very useful information that could help us to become more familiar with our database.
The observations contain :
- The periodicity of the database is 1 day
- The data were collected between 01/01/2017 and 07/07/2018
- The minimum value was 0 on 01/01/2017
- The maximum value was 453 on 07/07/2018
- The Median was 18, the Mean was 33.46.
- No missing values.
- The size of the dataset is 553
3.a.2) Create Prototype :
When creating the univariate prototype we must first choose the ‘Data history to consider’, and the number of ‘Future values to forecast.
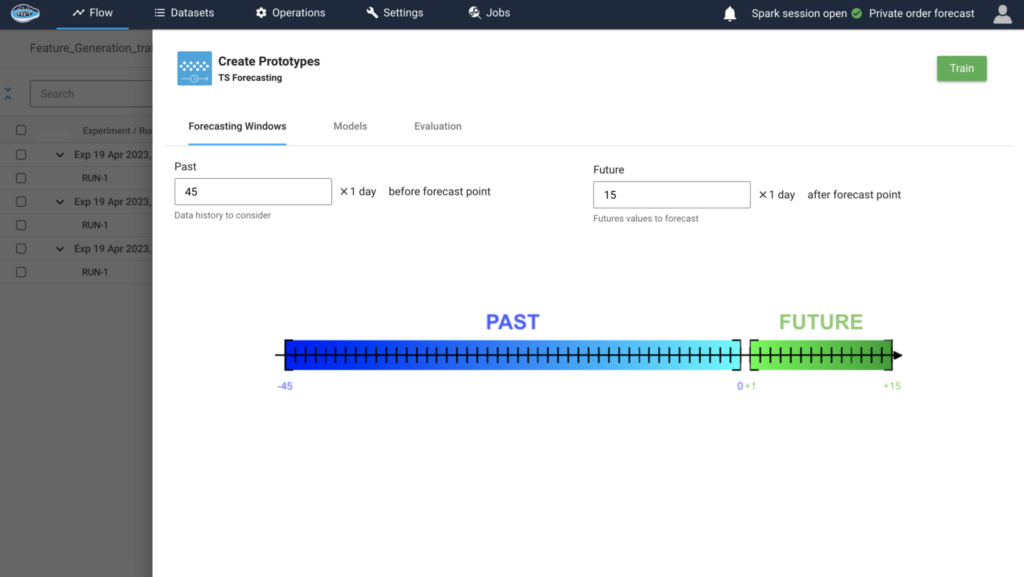
After defining the Forecasting windows, we have to choose the models we would like to train, in multivariate, there are 12 models which are the following
- ARIMA forecaster
- Block RNN
- LSTM
- GRU
- FFT
- LightGBM
- NBEATS
- Prophet
- Regular ML Models (Linear regression)
- Regular ML Models (Random Forest)
- SES
- TCN
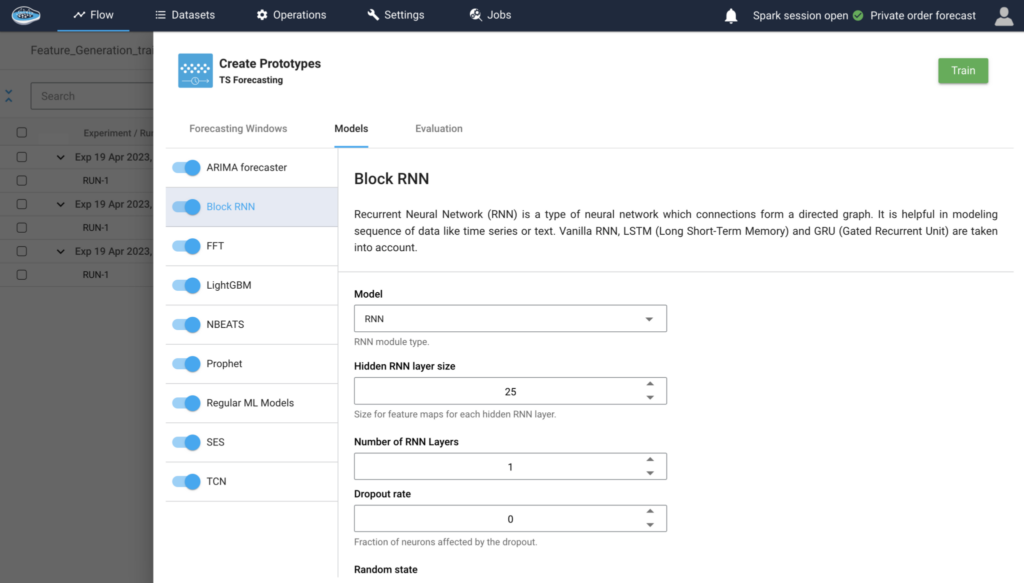
papAI solution guarantees an evaluation of each machine learning model. In order to set up the evaluation of our models.
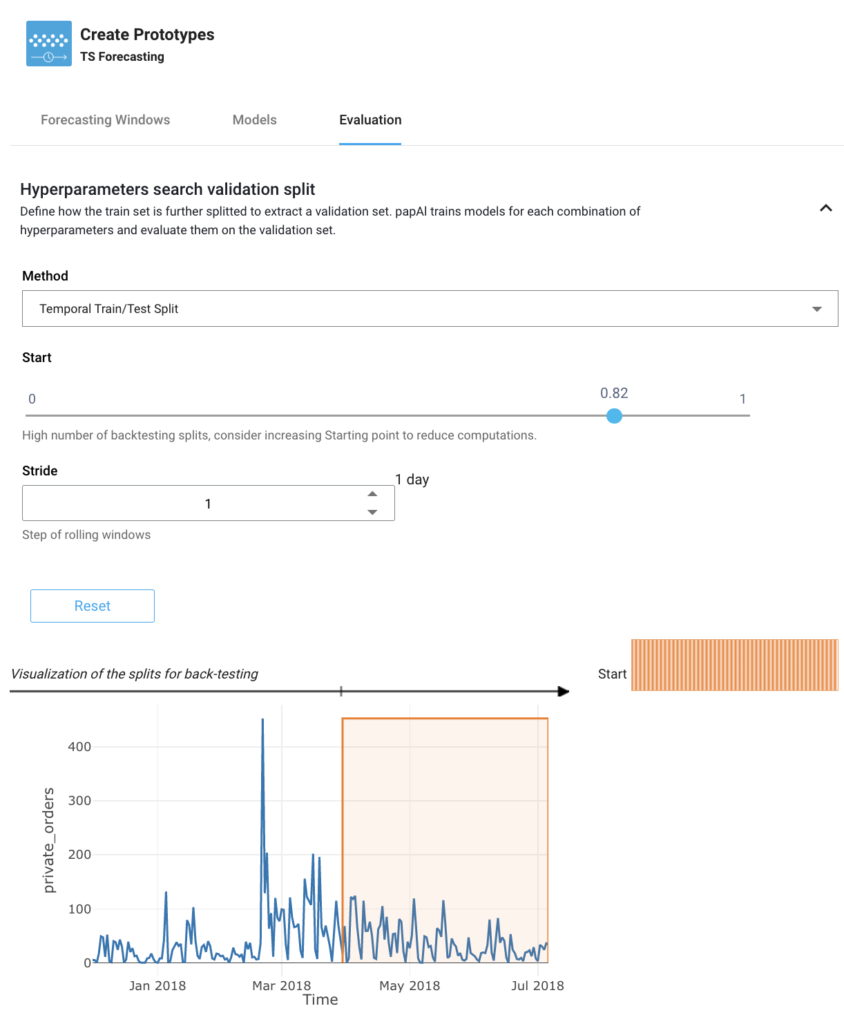
We receive an evaluation of every model once the training process has commenced.
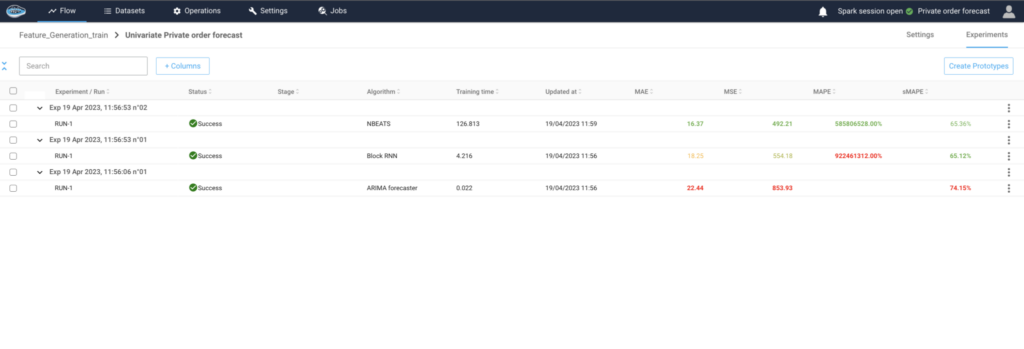
The NBEATS model has the most evaluations.
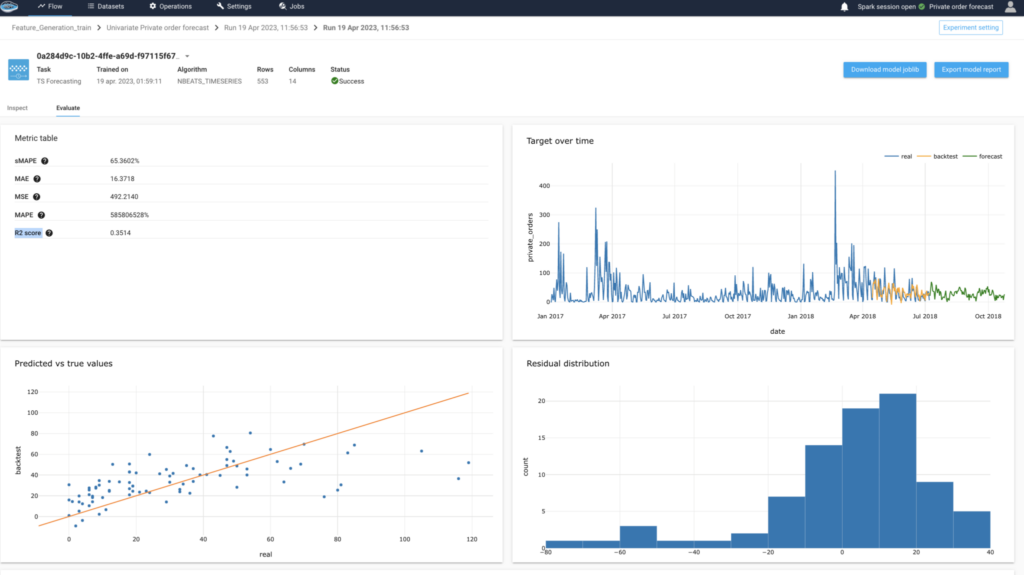
We can see in the evaluation which metrics have been taken into account:
- Metric table with the (sMAPE, MAE, MSE, MAPE, R2 score)
- Plot of the Target over time
- Plot of predicted value vs true value on a backtest
- Risidual distribution and risidual hilight
3.b) Multivariate:
When creating the Multivariate prototype we must first choose the features that can contribute to a better result.
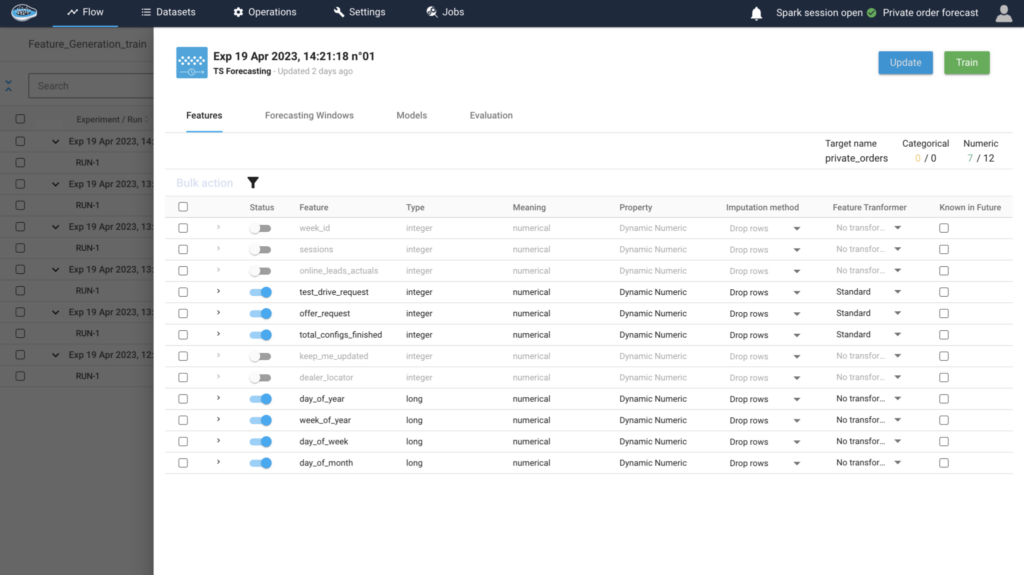
The second step would be to define the forecasting window with the number of points to be considered in the past and in the future.
The third step is to choose the models on which we would like to train the forecast.
In multivariate, papAI Solution has 16 models, which are the following
- Block RNN(RNN)
- Block RNN (LSTM)
- Block RNN (GRU)
- LightGBM
- NBEATS
- Regular ML Models ( Linear Regression)
- Regular ML Models ( Random Forest)
- TCN
- DeepAR (LSTM)
- DeepAR(GRU)
- Temporal Fusion Transformer
- MQ-CNN
- DeepState (LSTM)
- DeepState (GRU)
- DeepFactor (LSTM)
- DeepFactor(GRU)
4- Prediction
We will use the NBEATS model (univariate) to forecast the number of sales per day for the next four months after analysing and comparing the models we ran.
The prediction’s output is a data frame containing the private order values for the four months that follow the dataset’s expiration date.
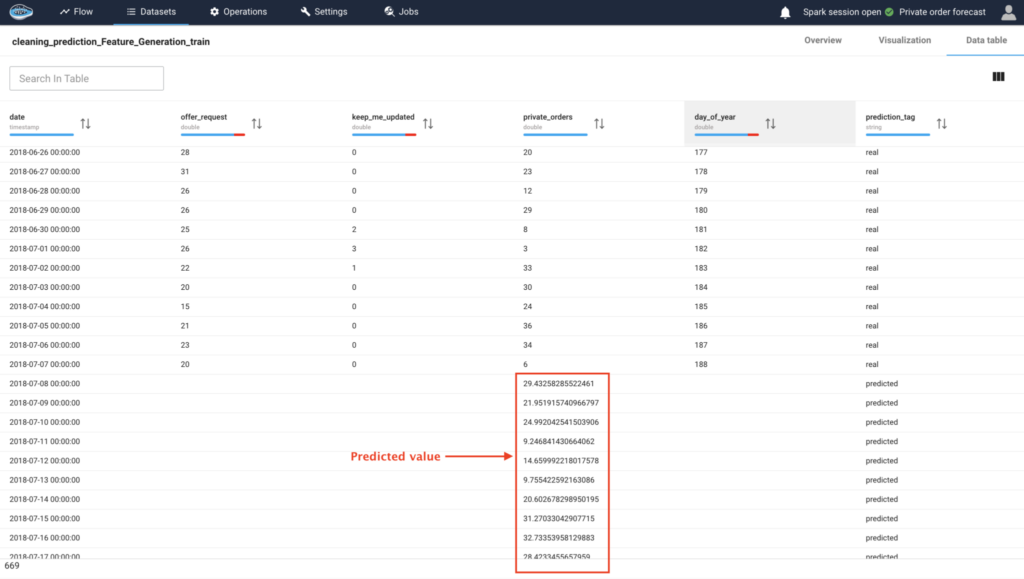
To Increase the Precision of your Sales Forecast, Use papAI Solution to Build your Own AI-based tool
In summary, the use of AI-based technologies for sales forecasting is increasingly essential for organizations of all sizes. By leveraging papAI solution, businesses have the opportunity to develop tailored AI tools that precisely align with their requirements. This can result in more precise and reliable sales predictions, thereby improving decision-making processes and ultimately driving profitability.
papAI solution facilitates the interpretation and transparency of outcomes by employing data mining, cleansing, and visualization techniques. This streamlined approach expedites the implementation of AI initiatives, whether in sales forecasting or drug discovery, while simultaneously reducing the time and expenses associated with bringing new therapies to market.
Schedule your demo today. Our team of AI specialists is poised to assist you in crafting a bespoke AI-based solution perfectly tailored to your organization’s unique needs.
Interested in discovering papAI?
Our AI expert team is at your disposal for any questions
Life at Datategy : Miloud Damene
Life at Datategy : Miloud Damene Hello everyone, my name...
Read MoreAdvanced Artificial Intelligence PDF 2025
Advanced Artificial Intelligence PDF 2025 Artificial intelligence has rapidly transformed...
Read MoreAI-Powered Process Optimization: Boosting Efficiency in Manufacturing
AI-Powered Process Optimization: Boosting Efficiency in Manufacturing In the manufacturing...
Read More