How RAG Systems Improve Public Sector Management The most important...
Read MoreThe landscape of almost every business in today’s world is changing as a result of technology, and the fitness and wellness sector is no different. Fitness clubs that were formerly limited to using weights and computers are increasingly embracing the power of artificial intelligence (AI) to improve member engagement and experiences.
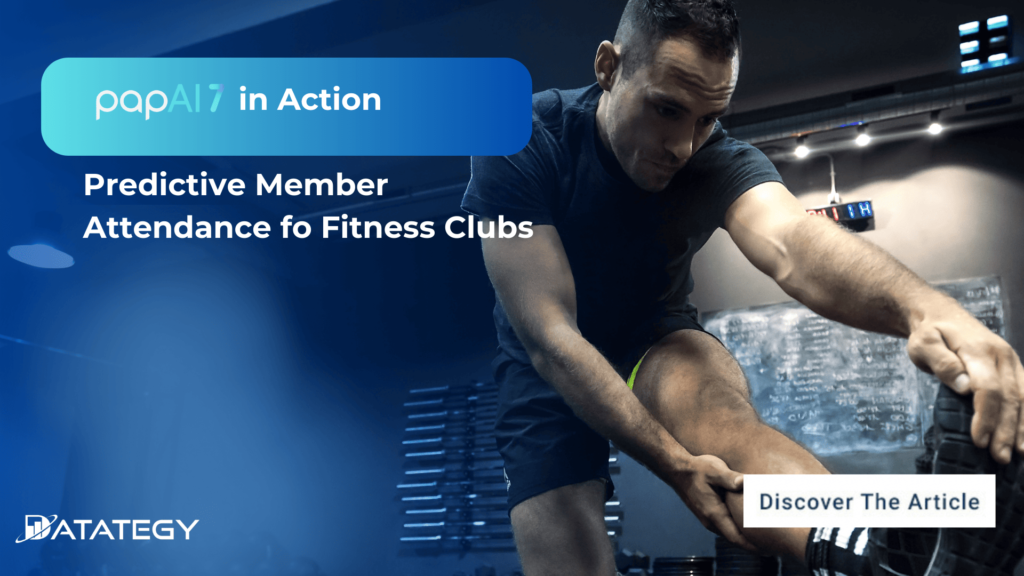
In this article, we will explore the predictive feature of papAI 7 and how it optimizes attendance in the fitness club field to able more customer retention and profits.
AI's Place in Fitness Club Management
AI technologies can be used in a wide range of applications:
1- Personalization of Member Experiences
Personalizing member experiences is one of AI’s important functions in fitness club administration. In order to customize workout programs, offer courses, and advise exercises that are in line with specific demands, AI-powered systems may analyze member data, including workout preferences, attendance trends, and fitness objectives. This degree of personalization encourages member involvement and aids people in more successfully achieving their fitness goals.
2- Resource Allocation Using Predictive Analytics
Fitness clubs can efficiently allocate resources thanks to AI’s predictive powers. For instance, AI algorithms can estimate high attendance hours, assisting clubs in more effectively scheduling workers and allocating resources like equipment and classroom space. This improves member experiences while simultaneously lowering operating expenses.
3- Security and Access Control
The safety and security of fitness club facilities are improved by AI-based security solutions, such as facial recognition and biometric access management. Both members and employees may feel secure knowing that only authorized people can enter restricted areas thanks to these technologies.
4- Making Decisions Based on Data
AI provides managers and owners of fitness clubs with insightful information. AI can find patterns, preferences, and places for improvement by examining member data. Clubs are able to regularly modify their offerings to match the requirements and interests of their members thanks to this data-driven decision-making process.
Understanding Member Engagement
Fitness clubs and wellness centers’ profitability and sustainability depend heavily on member participation. It includes members’ emotional and physical dedication to their workout regimens and club loyalty. In this part, we dig into the complex idea of member engagement, highlighting its significance and the difficulties that health clubs like GoalZone encounter.
Membership Engagement in Fitness Clubs: Its Importance
Engagement extends beyond only showing up. It involves encouraging club members to feel a feeling of commitment, drive, and belonging. Through a number of crucial factors, it is possible to comprehend the importance of member participation in fitness clubs:
Retention: Active members are more likely to renew their subscriptions, which lowers the turnover rate for fitness facilities. Long-term participants help to create a strong and vibrant fitness community.
Increased income: Active members can take part in more activities, buy club goods, or recommend others, all of which increase the club’s income sources.
Achievement of Wellness Goals: Actively involved members are more likely to reach their fitness and wellness objectives, which increases satisfaction and motivation.
How to choose the best AI solution for your data project?
In this white paper, we provide an overview of AI solutions on the market. We give you concrete guidelines to choose the solution that reinforces the collaboration between your teams.
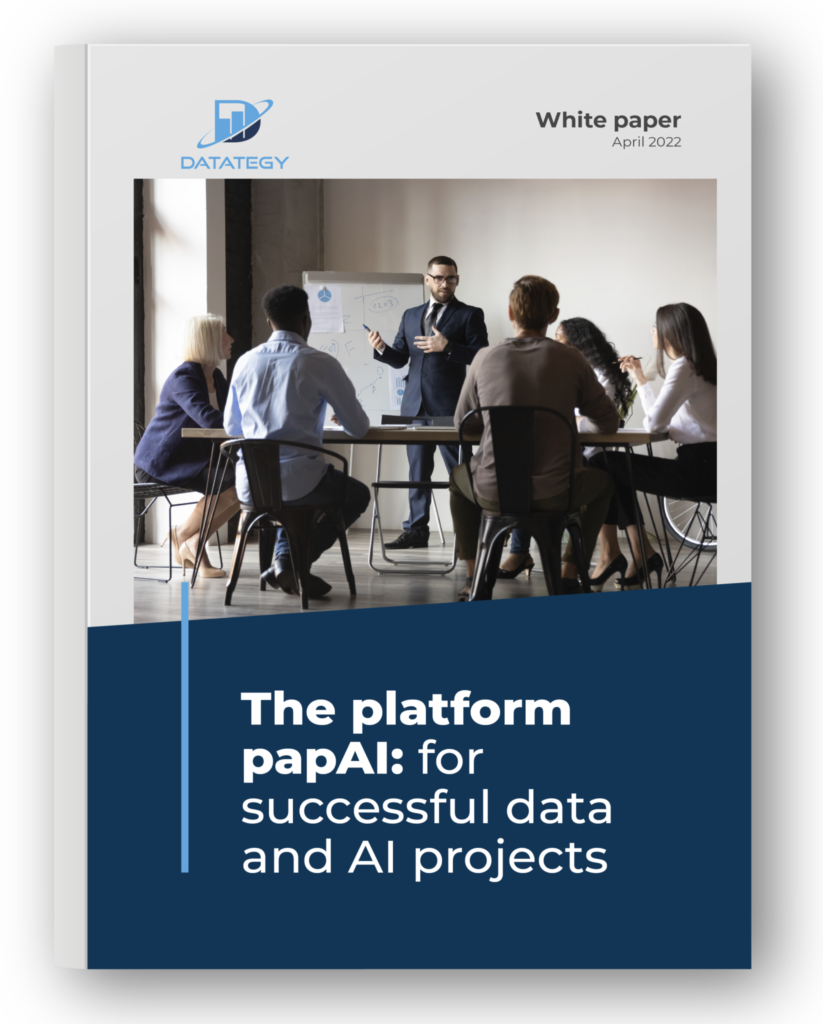
Case study: Predictive Member Attendance for Fitness Clubs using papAI 7
1- Data Presentation
GoalZone, a Canadian fitness club chain, offers fitness classes with varying capacities of 25 and 15 participants. While some classes consistently reach full capacity, others frequently suffer from low attendance rates. To address this challenge and improve class availability, GoalZone’s objective is to predict member attendance. By forecasting whether a member will attend a class or not, GoalZone can strategically open up additional spaces when non-attendance is anticipated, effectively increasing class availability and optimizing their services.
The primary goal of this use case is to develop a machine-learning model for predicting whether a member will attend a class.
The dataset comprises several critical columns. “Booking_id” serves as a unique identifier for each booking, allowing for precise tracking. “Months_as_member” monitors the member’s tenure in months, with a minimum duration of one month. The “Weight” column meticulously records members’ weights in kilograms, rounded to two decimal places. “Days_before” offers insight into the number of days prior to the class that a member registered, while “Day_of_week” specifies the day of the week when the class is scheduled. Furthermore, the “Time” column indicates whether the class falls in the morning (AM) or afternoon (PM). “Category” categorizes the type of fitness class being offered. Finally, the “Attended” column uses binary values, with “1” denoting attendance and “0” indicating non-attendance. Within the context of a fitness club, this dataset provides invaluable information for predictive modeling and analysis.
2- Data Preparation
Data preparation is the backbone of effective data analysis and machine learning. In this section of our article, we’ll explore the critical role data preparation plays in transforming raw data into valuable insights. From cleaning and transformation to handling missing values and outliers, we’ll uncover the essential practices and techniques that ensure your data is ready for analysis and modeling. So, let’s dive into the vital world of data preparation and its significance in extracting meaningful information from your datasets. On our data, we can notice some mistakes on the values of different columns :
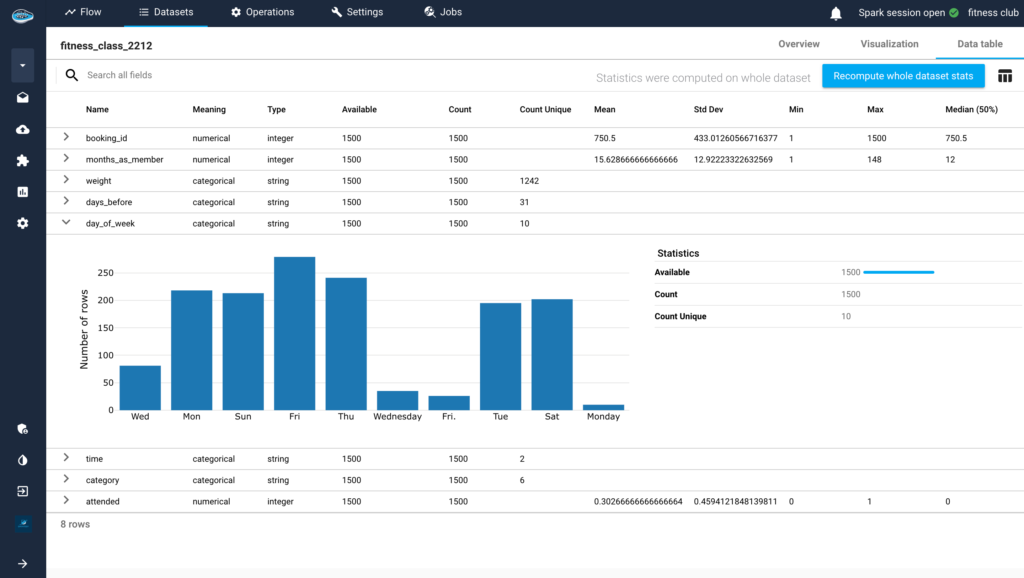
In our dataset, several discrepancies have been identified in various columns. Specifically, we observed inconsistencies in the “day_of_week” column, where values such as “Wednesday” should have been represented as “Wed”, “Fri.” as “Fri ”, and “Monday ” as “Mon ”. Additionally, we encountered typographical errors in the “days_before” column. To rectify these issues and enhance data quality, we employed the preprocessing features provided by papAI. This enabled us to perform data cleaning and standardization.
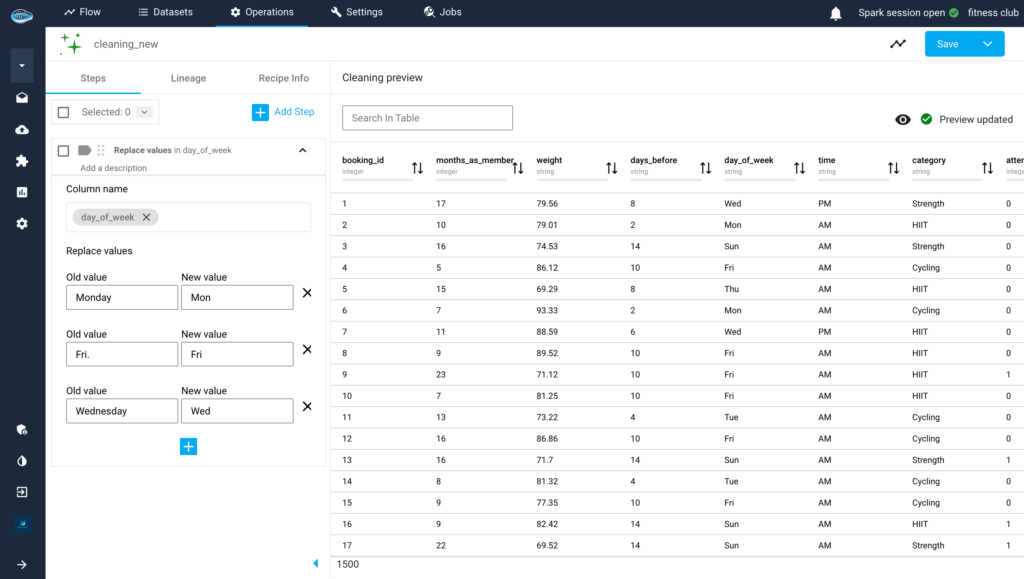
3- Model Training
The pivotal phase is training, where we put our data to work and enable our models to learn and generalize from it. This section of our article is all about training models, where we delve into the intricacies of machine learning and provide insights into the methodologies and techniques that empower your data to make predictions, classifications, and more.
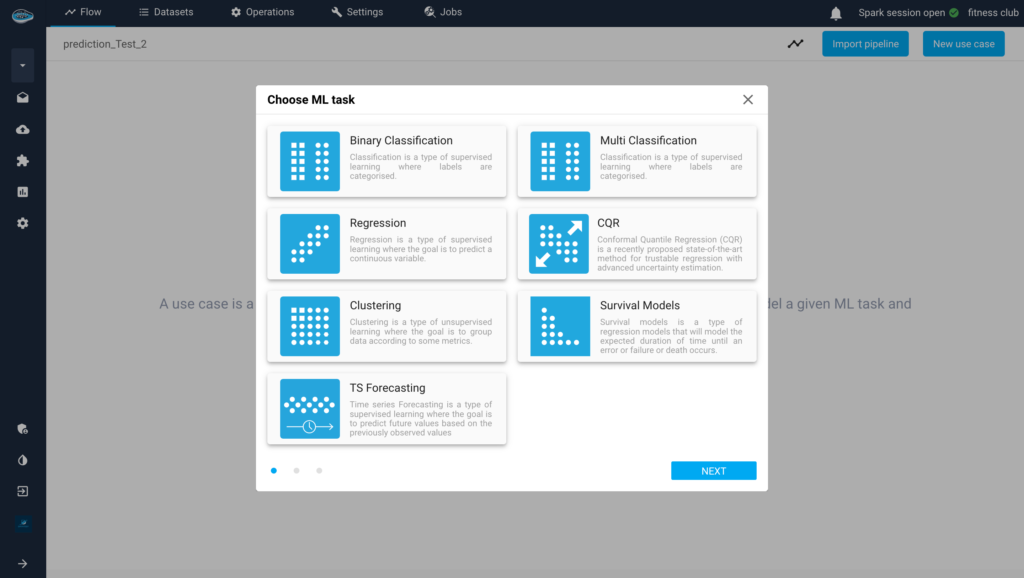
During the training phase, we utilized Papai’s diverse set of machine learning models to build and evaluate prediction models. Papai offers an extensive selection of machine learning algorithms and methodologies tailored specifically for classification tasks. Our approach commenced with the training of numerous models using a training dataset derived from a stratified split. These models encompassed widely recognized classifiers, including logistic regression, decision trees, random forests, and Gradient Boosting, among others.
Each model was trained using input features in conjunction with the corresponding binary target variable. To address the issue of class imbalance, we employed the data augmentation technique known as SMOTE (Synthetic Minority Over-sampling Technique), which generates synthetic samples for the minority class, effectively equalizing the class distribution. papAI also provided a variety of data augmentation methods, including random over-sampling, ADASYN (Adaptive Synthetic Sampling), Borderline SMOTE, SWIM maha (Synthetic With Interpolation of Minority using Mahalanobis distance), and SWIM RBF (Synthetic With Interpolation of Minority using Radial Basis Function).
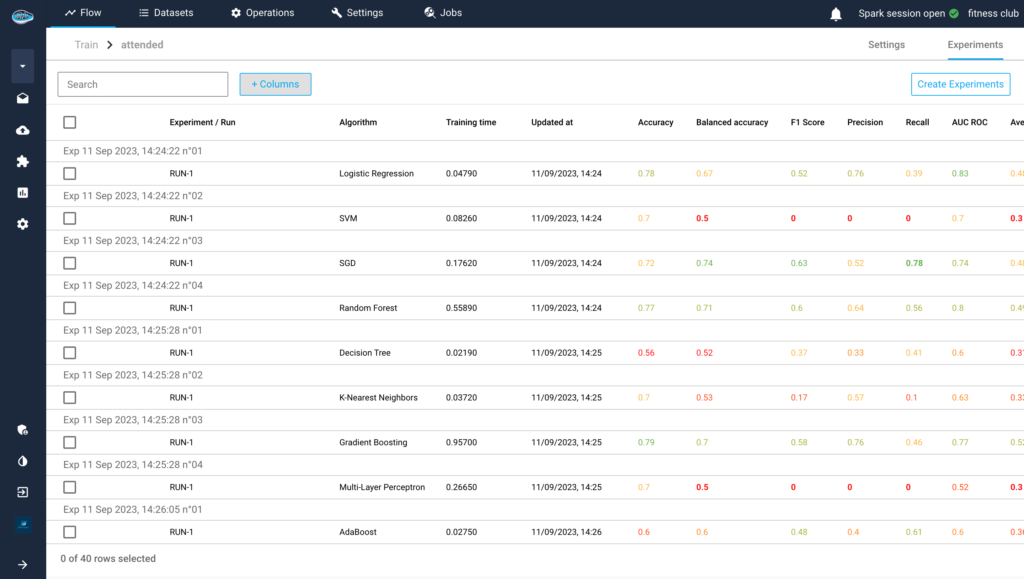
In this scenario, we have chosen to implement the “Decision Tree” model due to its commendable performance metrics. Our decision to utilize the “Decision Tree” model in this context stems from its impressive performance across various key metrics. These metrics, which encompass accuracy, precision, recall, and other pertinent measures, have consistently exceeded our predetermined thresholds. This decision underscores our strong confidence in the model’s capability to provide accurate predictions in a production setting.
4- Results interpretation
a) Roc Curve :
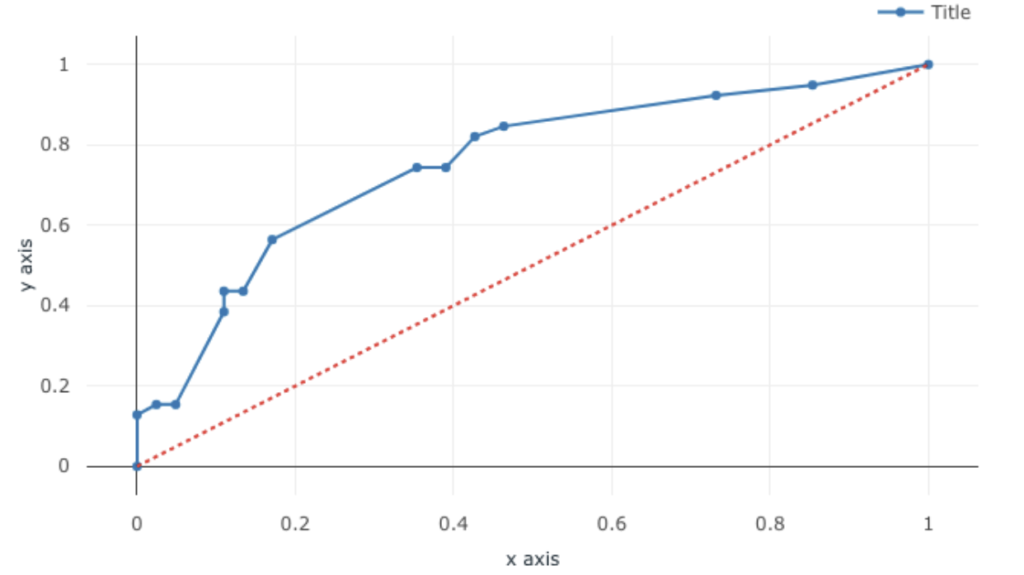
We observe that the blue curve rapidly converges towards 1, residing in close proximity to the upper-left corner. This binary classification effectively distinguishes between positive classes (indicating the presence of a member) and negative classes (indicating the absence of a member). Furthermore, the area under the curve closely approximates 1, affirming the model’s relevance and effectiveness in this particular scenario.
b) Confusion Matrix
A confusion matrix is a vital tool for assessing the performance of a binary classification model. It provides a visual representation of how well the model correctly categorizes data based on their actual class. The matrix includes four key elements:
– True Positives (TP): The number of positive instances correctly identified by the model.
– False Positives (FP): The number of negative instances incorrectly classified as positive.
– True Negatives (TN): The number of negative instances correctly identified as negative.
– False Negatives (FN): The number of positive instances incorrectly classified as negative.
From the confusion matrix, we can calculate important performance metrics:
1. Precision: The proportion of true positives among all positive predictions (TP / (TP + FP)). It measures the accuracy of positive predictions.
2. Recall: The proportion of true positives among all actual positive instances (TP / (TP + FN)). It gauges the model’s ability to identify all positive cases.
3. F1-Score: A combined metric considering both precision and recall, calculated as 2 * (Precision * Recall) / (Precision + Recall). It balances precision and recall when both are critical.
4. False Positive Rate: The ratio of false positives among all actual negatives (FP / (FP + TN)). It indicates the proportion of negative cases incorrectly classified as positive.
In summary, a confusion matrix and its associated metrics are essential tools for evaluating the effectiveness of classification models. They provide a comprehensive view of a model’s performance in distinguishing between two classes.
Here, we get this matrix :
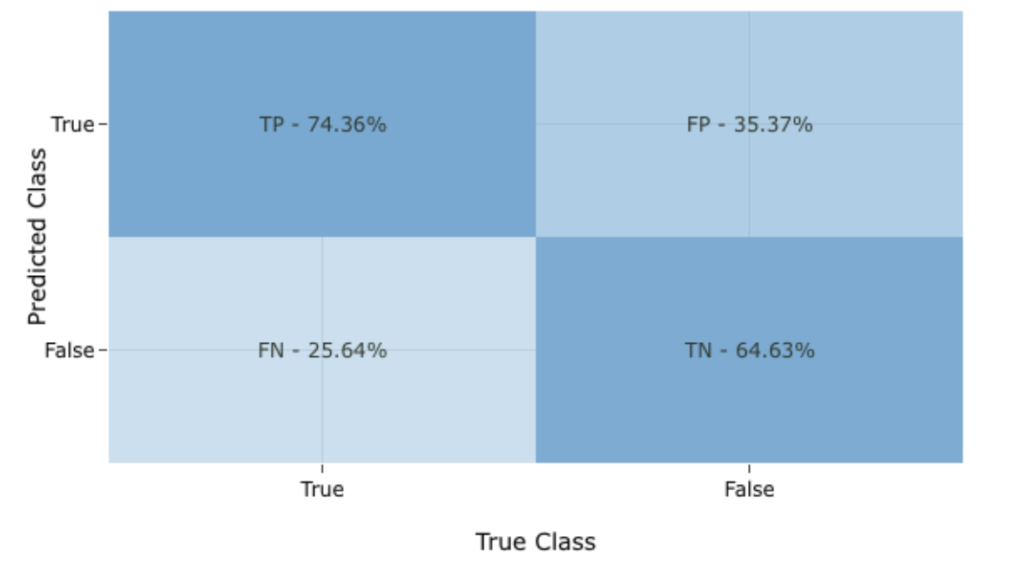
We are highly content with the performance of this matrix. In this specific context, our primary goal revolves around the minimization of false negatives. Our objective is to avert situations where we erroneously predict that a member won’t attend a class when, in reality, they intend to do so. This holds significant importance since an incorrect prediction in this direction could lead to overcrowded classes with limited resources, potentially resulting in member dissatisfaction and their potential migration to alternative fitness facilities. The key concern here is the potential loss of clients, which could adversely affect member retention and overall satisfaction levels. Additionally, we gain valuable insights into the features that exert the most substantial influence on the model’s performance.
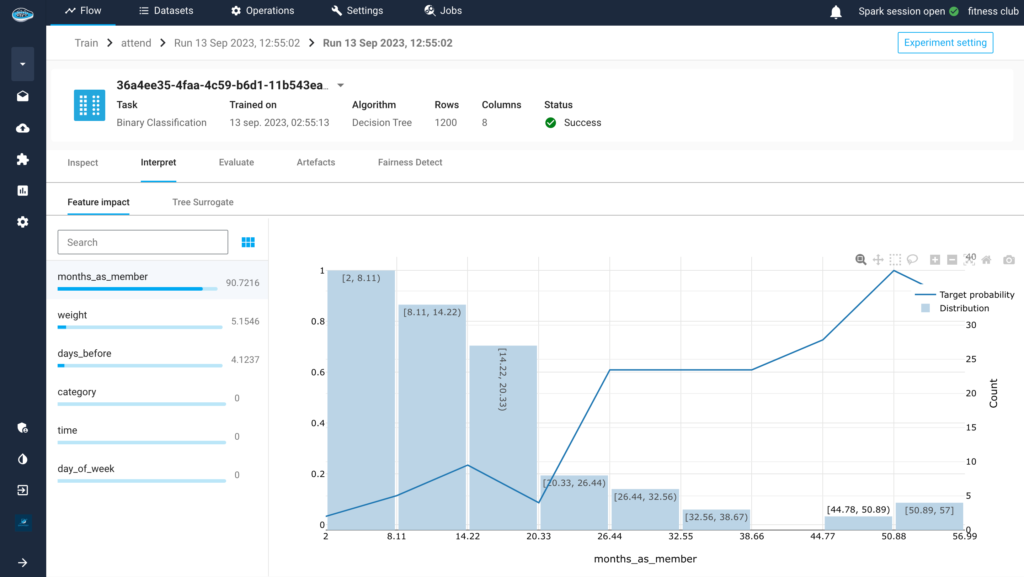
The feature “Months_as_member” emerges as the most influential factor in determining the outcome. This is readily apparent when examining the “target probability” curve, which unequivocally demonstrates that as a member’s tenure in the gym increases, their likelihood of attending classes also rises.
Furthermore, we can explore the impact of altering feature values. For instance, in a specific case, the algorithm predicts a low likelihood of class attendance, estimating a probability of just 93.98%. This particular member has maintained their gym membership for a duration of 7 months.
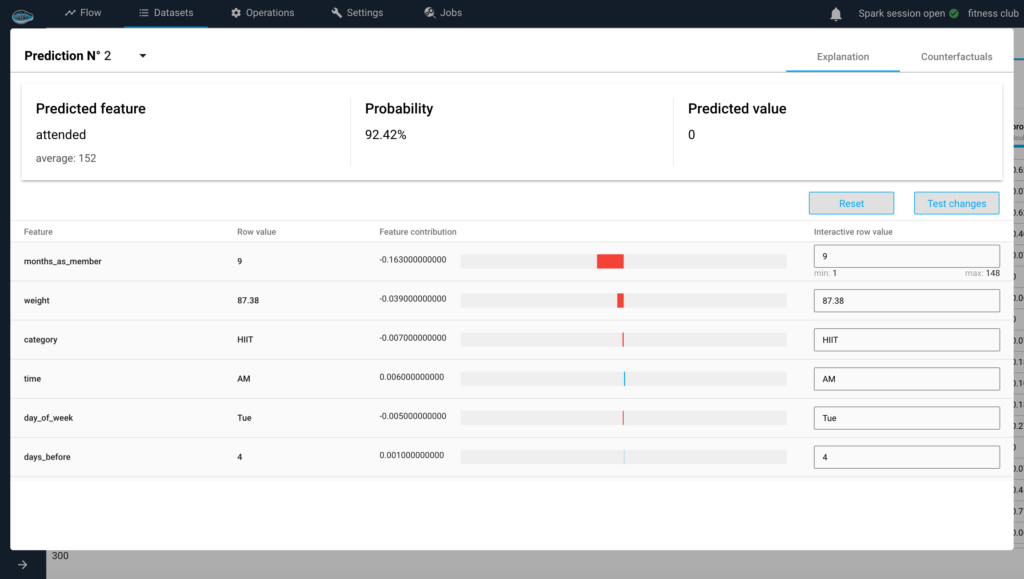
Given that “months_as_member” stands out as the most influential feature, we made the decision to investigate the impact of increasing a member’s seniority in our analysis. For instance, let’s consider a scenario where an individual has maintained their membership for a substantial period, specifically 49 months.
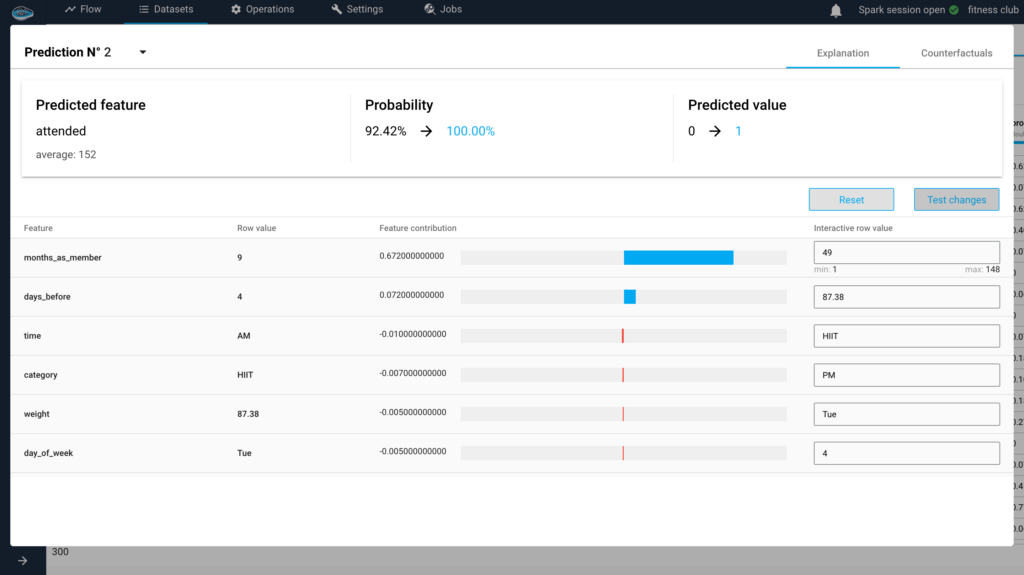
The algorithm predicts that the member will be present with a probability of 100%.
Improve your member attendance forecasts by creating your own AI-based tool with the papAI solution
With papAI solution, you can unleash the potential to completely transform your predictions for member attendance. Our platform gives you the ability to create an AI-driven toolbox that is specifically suited to meet your prediction needs. You can improve the accuracy and effect of your attendance estimates by utilizing cutting-edge machine-learning algorithms.
To see firsthand how papAI solution can drastically improve your member attendance projections, schedule your personalized demo now. Our team of seasoned professionals is prepared to work with you to create a custom AI-powered toolset that is tailored to the unique needs of your organization.
Interested in discovering papAI?
Our AI experts are at your disposal for any questions
Scaling RAG Systems in Financial Organizations
Scaling RAG Systems in Financial Organizations Artificial intelligence has emerged...
Read MoreHow AgenticAI is Transforming Sales and Marketing Strategies
How AgenticAI is Transforming Sales and Marketing Strategies Agentic AI...
Read More“DATATEGY EARLY CAREERS PROGRAM” With Abdelmoumen ATMANI
“DATATEGY EARLY CAREERS PROGRAM” With Abdelmoumen ATMANI Hello, my name...
Read More