The Next AI Frontier: Jean-Marc Briquet Talks to Datategy’s Future...
Read MoreUnveiling papAI 7:
Navigating the Era of Context-Aware Generative AI
Table of Contents
ToggleFew technical innovations have captured the public’s attention as much as Generative Artificial Intelligence (Gen AI) has in the constantly changing environment of technological progress. This cutting-edge technology has changed sectors over the last two years and opened up new opportunities for innovation, automation, and problem-solving. At Datategy, our dedication to being at the cutting edge of innovation fuels our constant goal of seamlessly integrating Gen AI into our lauded papAI platform.
With the release of papAI 7, we are excited to announce a significant advancement. The inherent power of Generative AI is beautifully linked with the dynamic adaptability of papAI’s powerful capabilities in its most recent edition, which symbolizes a synthesis of innovative technologies. Context-Aware Generative AI, a paradigm-shifting development, is introduced in papAI 7 as part of our commitment to delivering unmatched user experiences.
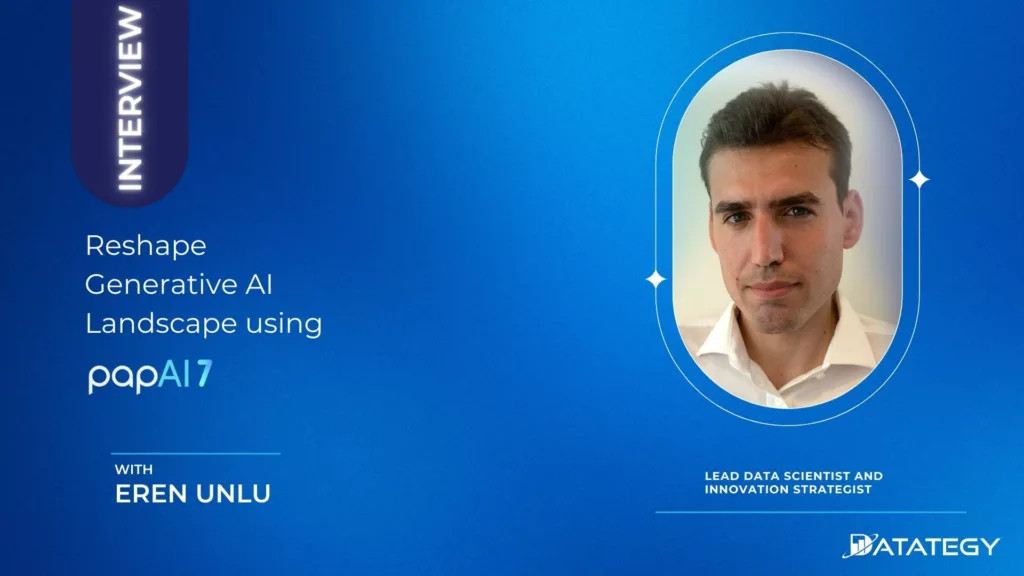
Read Eren UNLU’s interview (Lead Data Scientist and Innovation Strategist) for more information on how Datategy’s teams imagined papAI 7 to address use cases. Explore a future of limitless invention, innovation, and opportunity.
Could you introduce yourself?
Hey. I’m Eren UNLU a data scientist and AI engineer, currently lead data scientist and innovation strategist at Datategy, a company pioneering the development of new solutions based on artificial intelligence and data. My current expertise and professional activities cover a wide spectrum of AI applications such as computer vision, signal processing, resource optimization, and forecasting. I conducted more than 6 years of academic research as a PhD student and post-doc in different institutes in France, before joining Datategy in 2019. I also continue to participate in academic activities as I can find within Datategy, publishing papers, giving lectures, and developing patents.
Can you give us an overview of the context-aware gen ai capabilities of papai?
The emergence of powerful foundational models has set the stage for a new era in business transformation. However, these models, while groundbreaking, only represent a fraction of the vast potential of AI.
While their generic capabilities can achieve much, they often fall short in addressing specific business challenges. Enter papAI 7: your no-code gateway to state-of-the-art AI solutions. Whether you’re an expert or just starting out, our platform empowers you to customize language or image-generative models to fit your unique business needs. Imagine crafting a tailored image generation model for product designs based on specific criteria like type, color, and subtype. Or fine-tuning a linguistic model to answer intricate queries from your extensive legal databases.
Beyond these, papAI 7 seamlessly integrates cutting-edge features like Retrieval Augmented Generation (RAG), LLM-tool interaction, orchestration of multiple LLMs, semantic comprehension, and automated knowledge graph building – all within a user-friendly, no-code environment. With papAI 7, you’re not just adopting another tool; you’re embarking on a comprehensive data science journey. Our pre-configured foundational agents ensure you enjoy a context-rich, holistic data analysis and machine learning experience tailored for your business success. Elevate, innovate, and dominate with papAI 7.
What was the inspiration behind integrating Context-Aware Generative AI into papAI? How do you envision this technology enhancing the content generation process for users?
Inspiration:
The motivation to integrate Context-Aware Generative AI into papAI stemmed from two primary observations:
- Dynamic User Needs: In the vast expanse of the digital world, users are constantly evolving, and so are their requirements. Traditional generative AI models, though revolutionary, often deliver outputs based on generalized training, making them less adaptable to niche or specific contexts.
- The Limitation of Broad Spectrum AI: While foundational models possess extensive knowledge, they lack the finesse to truly understand and generate content tailored to a user’s unique context. We identified this gap as an opportunity to fine-tune and align AI outputs more closely with individual user needs.
Vision:
We envisage the Context-Aware Generative AI as a game-changer in content generation for users in the following ways:
- Personalized Outputs: By being context-aware, the AI can create content that resonates more with a user’s immediate requirements and the environment. Whether it’s creating marketing campaigns for specific demographics or generating reports for a distinct industry, the outputs are more in line with what’s needed.
- Seamless Integration with Existing Workflows: Users no longer need to make extensive modifications to AI-generated content. The context-awareness ensures that the first draft is closer to the final product, saving time and resources.
- Enhanced Creativity: With the AI understanding context better, users can collaborate with the system, using it as a creative partner rather than just a tool. This synergy can lead to novel ideas and content that might not have been possible otherwise.
- Learning and Adaptation: The more users interact with the context-aware system, the better it becomes at predicting and aligning with their needs. This continuous learning cycle ensures that the system stays relevant and useful.
In essence, by embedding Context-Aware Generative AI into papAI, we’re not just enhancing the tool’s capabilities; we’re transforming how users perceive and interact with AI. We’re transitioning from a world where AI is a mere utility to one where it becomes an intuitive collaborator, enhancing creativity, efficiency, and innovation.
Fine-tuning language models to align with specific domains can be complex. Could you share some best practices or guidance for users who want to fine-tune LLM on papAI for their unique requirements?
Finding Language Models (LLMs) is essential for unlocking new levels of sophistication and flexibility in the field of context-aware generative AI. This paradigm refers to the practice of fine-tuning language models to fit them with certain activities, domains, or circumstances. It is the method that bridges the gap between a fundamental command of the language and the nuanced understanding necessary to provide information that is relevant to the situation.
Here are some advice and best practices to fine-tune papAI’s models for a unique requirement:
- Understand Your Objective: Before beginning the fine-tuning process, clearly define what you aim to achieve. Whether it’s answering domain-specific questions, generating tailored content, or understanding niche terminologies, your objective will guide the fine-tuning process.
- Curate High-Quality Data: The quality of your training data is paramount. Gather domain-specific datasets that are clean, accurate, and representative of the nuances of your field. The better your data, the more effective your fine-tuned model will be.
- Start Small, Then Expand: Begin with a subset of your data to test the fine-tuning process. Once you’re confident in the results, you can scale up to larger datasets. This iterative approach allows for quicker troubleshooting and optimization.
- Avoid Overfitting: While it’s tempting to fine-tune extensively to achieve perfection, overfitting can make the model perform poorly on unseen data. Regularly validate the model’s performance on a separate test set to ensure generalization.
- Regularly Evaluate Performance: Use evaluation metrics relevant to your domain and task. For instance, if your task is text classification, accuracy or F1-score might be appropriate. Regular evaluations will provide insights into how the model is improving and when it’s ready for deployment.
- Leverage Transfer Learning: Take advantage of the knowledge the model has already acquired during its initial training. This can reduce the amount of new data needed and speed up the fine-tuning process.
- Iterate and Adjust: Fine-tuning is often an iterative process. Don’t be afraid to go back, adjust parameters, or introduce new data to enhance the model’s performance.
- Stay Updated: AI and machine learning are rapidly evolving fields. Stay informed about the latest techniques, tools, and best practices in model fine-tuning. What works best now might evolve in the near future.
- Ethical Considerations: Ensure that the data you’re using for fine-tuning respects privacy and ethical guidelines. Be aware of potential biases in your data, as these can inadvertently be amplified during the fine-tuning process.
- Seek Expertise When Needed: If you’re venturing into unfamiliar territories, consider collaborating with experts or seeking external guidance. Sometimes, a fresh pair of eyes or expert insight can significantly expedite the fine-tuning process.
mPLUG-Owl architecture for improved image captioning using LLMs
Watch papAI7's Demo and Redefine Your Possibilities
Witnessing generative AI in action on the new version of papAI is an awe-inspiring experience. This live demonstration demonstrates the innovative prowess of the latest iteration of papAI, which promises to revolutionize content creation by harnessing the power of adaptability and scalability.
Interested in discovering papAI?
Our commercial team is at your disposal for any questions
How AI Transforms Production Lines into Smart Manufacturing Systems
How AI Transforms Production Lines into Smart Manufacturing Systems AI...
Read MoreHow Artificial Intelligence Simplifies Spare Part Searches
How Artificial Intelligence Simplifies Spare Part Searches Artificial intelligence is...
Read MoreLife at Datategy : Miloud Damene
Life at Datategy : Miloud Damene Hello everyone, my name...
Read More