How Law Firms Use RAG to Boost Legal Research RAG...
Read MoreAI-Based Fraud Detection in Banks: The Key Role of papAI Solution
Table of Contents
ToggleFraud in the banking industry has grown to be a major worry in today’s digital age. Banks now have to contend with more sophisticated and intricate fraud schemes than ever before due to the growth of Internet banking and mobile payment systems. Many banks are using artificial intelligence (AI) for fraud detection to counter these risks. Massive volumes of data may be analyzed in real-time by AI-based fraud detection tools to spot fraudulent transactions and stop them from happening.
Find out how papAI can improve the deployment of AI projects in the Banking Sector here.
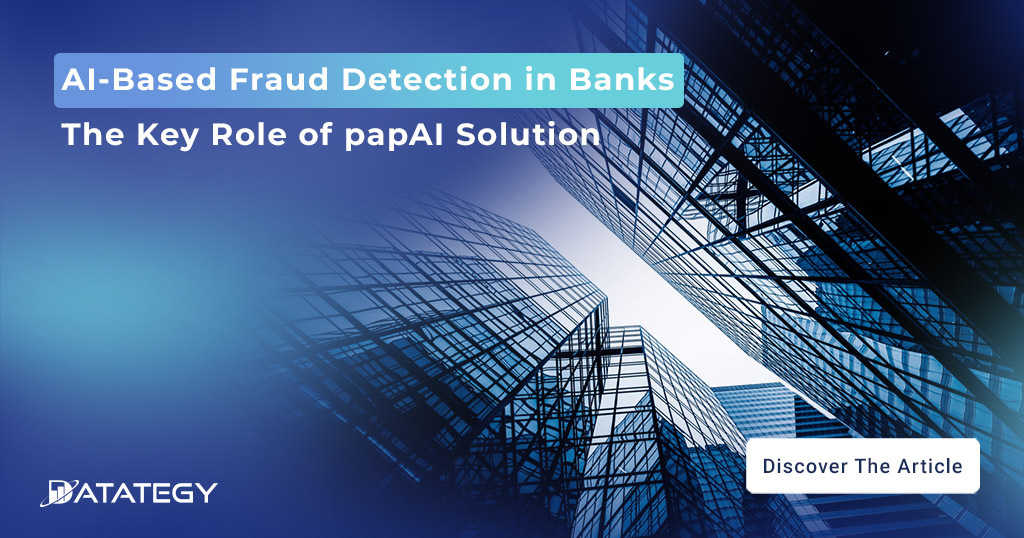
In this article, we’ll examine papAI Solution’s crucial contribution to banks’ AI-based fraud detection. We will go into the advantages of adopting papAI Solution and how it improves the effectiveness and accuracy of fraud detection.
What Is Fraud Detection?
The process of identifying and stopping fraudulent activity within a system or organization is known as fraud detection. Fraud detection, as applied in the banking industry, refers to the methods and tools used to spot fraudulent financial activities such as credit card fraud, identity theft, and money laundering. Methods for detecting fraud can range from simple rule-based systems that alert users to fraudulent transactions based on established criteria to more sophisticated machine learning algorithms that can instantly analyze huge volumes of data to find unusual patterns and behaviors. In order to preserve the organization’s finances and the interests of its clients, fraud detection must be successful.
What Are the Different Types of Fraud and How Do Fraudsters Operate?
Depending on the kind of fraud they are perpetrating, fraudsters employ a variety of tactics. In order to acquire private information or money, fraudsters typically utilize deceit and cunning. They frequently abuse weak points in computer systems or prey on people’s trust. Typical forms of fraud include:
The Limitations of Traditional Fraud Detection Methods
One of their major disadvantages is that traditional fraud detection techniques mainly rely on human procedures and rules-based systems. Staying on top of changing fraud risks can be challenging since these systems might be rigid and sluggish to react to new fraud strategies. Additionally, because of the huge number of false positives that these systems may produce, a lot of time and money is wasted looking into non-fraudulent activity. In the end, this may lead to dissatisfied customers and even missed commercial prospects.
Another drawback of conventional fraud detection techniques is that they frequently only look for well-known fraud patterns. Traditional approaches might not be able to spot new or sophisticated fraud schemes since fraudsters are continually changing their strategies. As a result, financial institutions may be exposed to new fraud schemes, some of which may go undetected until after substantial losses have been incurred.
The benefits of AI-Based Fraud Detection
1- Improved Accuracy
Improved accuracy is one of the most important benefits of AI-based fraud detection in banks. A survey conducted by PwC revealed that 84% of financial institutions reported improved accuracy in fraud detection after adopting AI and machine learning technologies. The foundation of conventional fraud detection techniques is rule-based technology, which can only detect fraud in accordance with pre-established rules and patterns. This implies that scammers will find it simple to circumvent these laws and avoid detection.
2- Greater Detection and Response Speeds
AI-based fraud detection systems may continually monitor transactions, consumer behaviors, and other pertinent data points in real-time by utilizing machine learning algorithms. This gives the system the ability to spot suspicious trends, abnormalities, or departures from the norm that could point to fraud. Banks can as a result quickly identify fraudulent transactions or activity and take action right away to stop future losses.
Either way, having rapid reactions is essential. When possible fraud is identified, AI-based fraud detection systems may produce real-time warnings and notifications. Then, banks may analyze the suspicious activity right once and start the necessary precautions to lessen the effects of fraud. By being proactive, the bank and its clients are both protected and financial losses are reduced.
- 3- Reducing Coast
The first benefit of AI-based fraud detection is that it requires less time and effort to spot probable fraudulent actions. Machine learning algorithms are capable of quickly and accurately analyzing large volumes of data, including transaction logs, client profiles, and historical trends.
According to a report by Accenture, financial institutions that adopted AI technologies for fraud detection and prevention achieved an average cost reduction of 14% compared to traditional methods. Artificial intelligence technologies minimize the need for manual inspection and intervention by automatically reporting questionable transactions and activity. This results in significant time savings for fraud detection teams, enabling them to concentrate their efforts on looking into real risks instead of sorting through a large number of false alarms.
Banking industry : Lead your Business teams to embrace AI
The banking services industry has long been a leader in AI implementation, discover in this free White Paper the power of “Explainable Artificial Intelligence”.
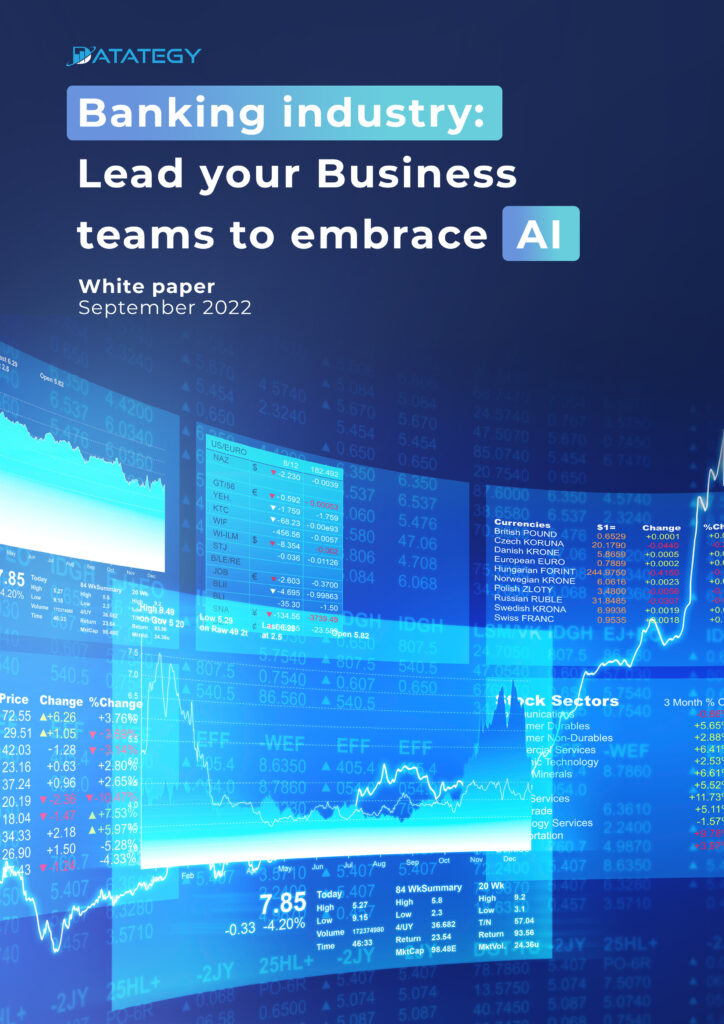
Case Study: Fraud Detection using papAI Solution
Data set presentation
For this use case, we will use the Banksim dataset to detect fraudulent transactions. This synthetically generated dataset consists of payments from various customers made at different times and with different amounts.
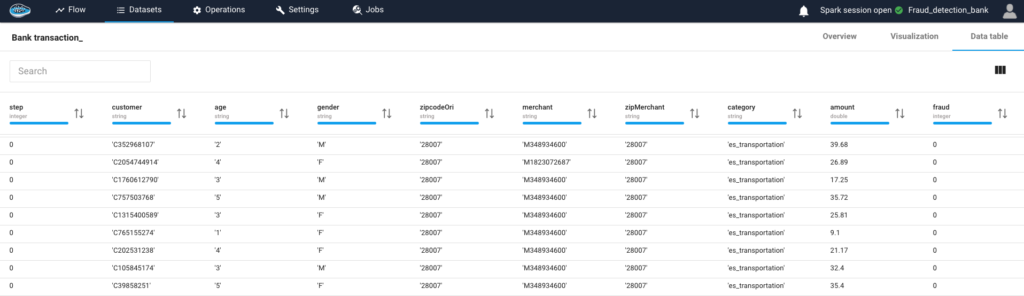
1- Data analysis and preparation
a- Data analysis
Before launching machine learning to predict fraud cases, we start by analyzing the input data. papAI solution is an AI tool, and also a BI (business intelligence) tool. When importing a database, papAI solution detects the type of data for each column and automatically calculates statistics that give an overview of the database.
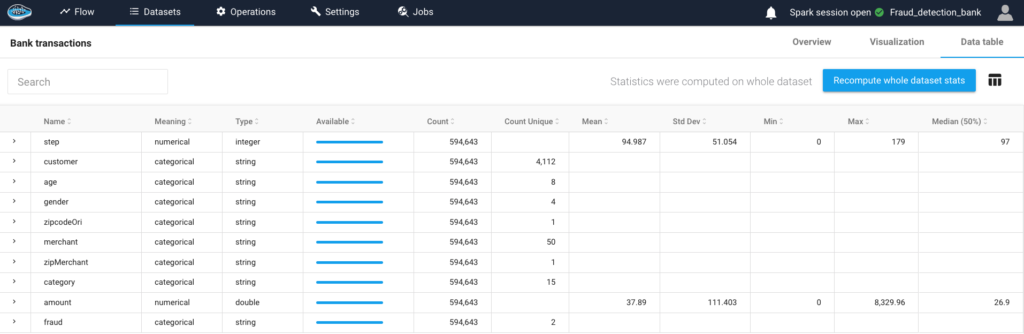
In the picture below, we can see that the number of transactions in our dataset is 594,643. 98.79% are non-fraudulent cases, and only 1.21% of our data represent fraud cases.
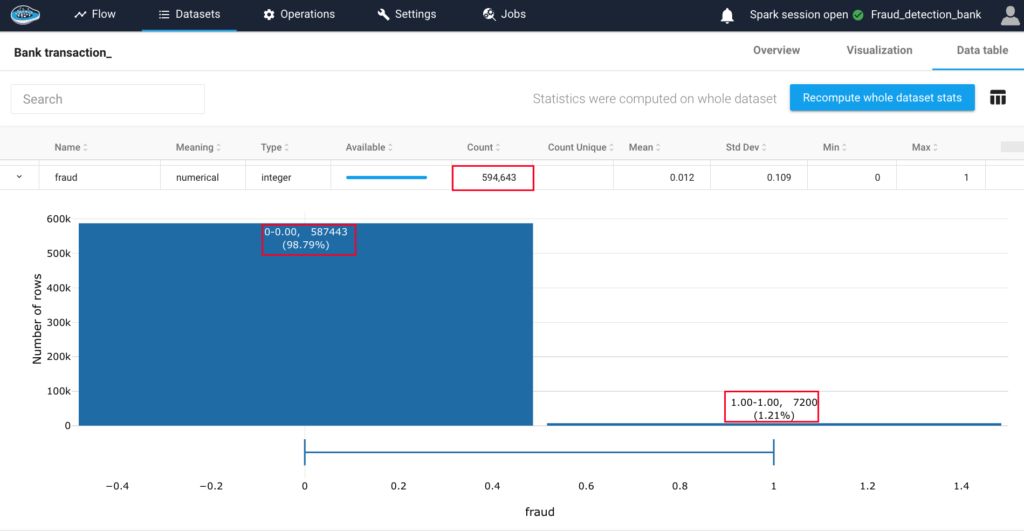
We note that there is a huge unbalance in our dataset, which may lead to a biased model, as the model may learn to “always” predict the non-fraud cases that are the majority class, and will fail to predict the fraud cases. In this case, if the model has a high accuracy rate, this may be due to the fact that it always predicts the majority class, which is not necessarily very useful.
b- Data preparation
After analyzing our data, we will prepare it for prediction. To do this, we divide our database, taking 80% of the data as a training dataset, and 20% as a test dataset. Balancing the percentage of fraud classes on the two datasets. This is done using the “Split rows” operation, with the percentage.
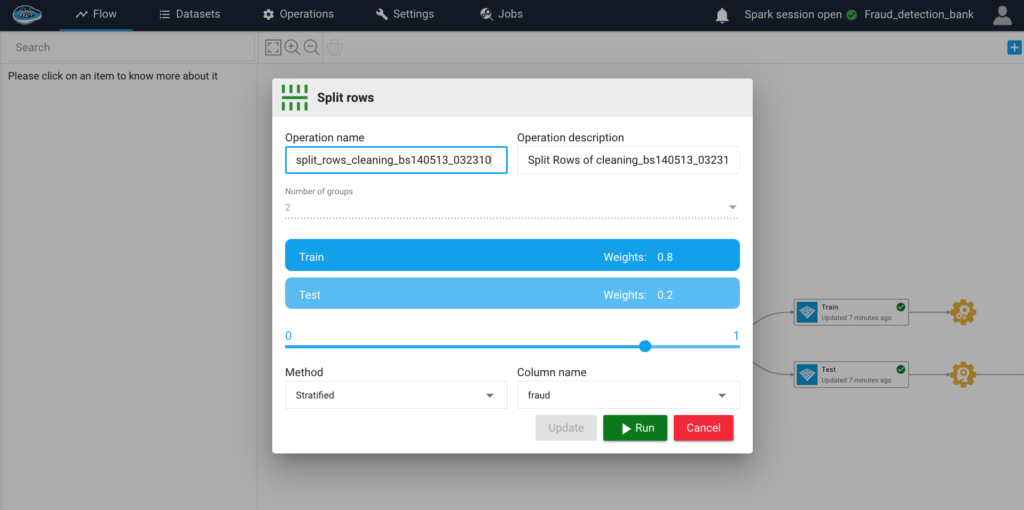
2-Data training
a-Creating the prototype
After preparing the training data and the test data, we will create our training prototype. First, we will select the features to be used in the training, we saw in the analysis that the zipCodeOrigin and zipMerchant columns both contain a single value, which means that they do not need to be added in the training.
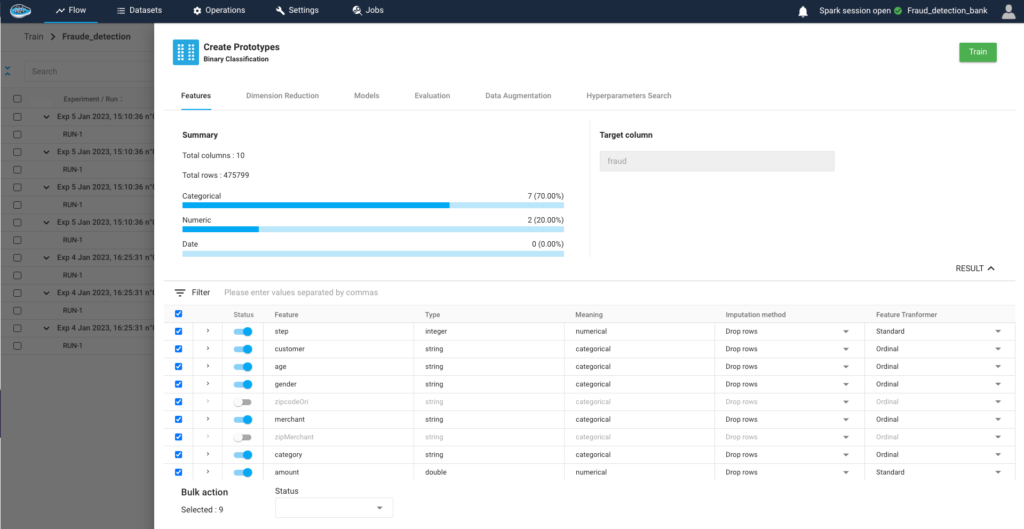
Then we choose the machine learning models to use. We choose all the models, in order to compare the results and choose the model that gives the best result for the prediction.
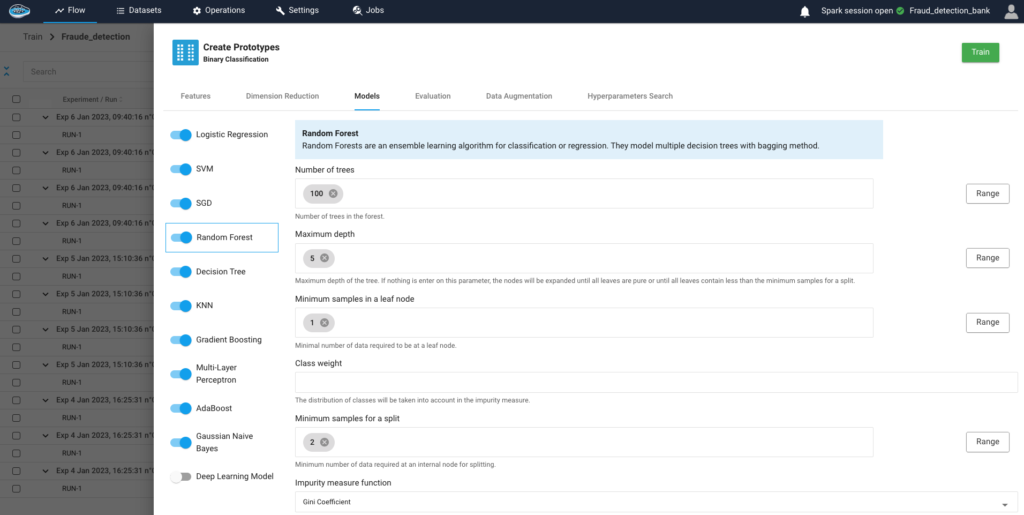
Thirdly, we choose to balance and mix our data.
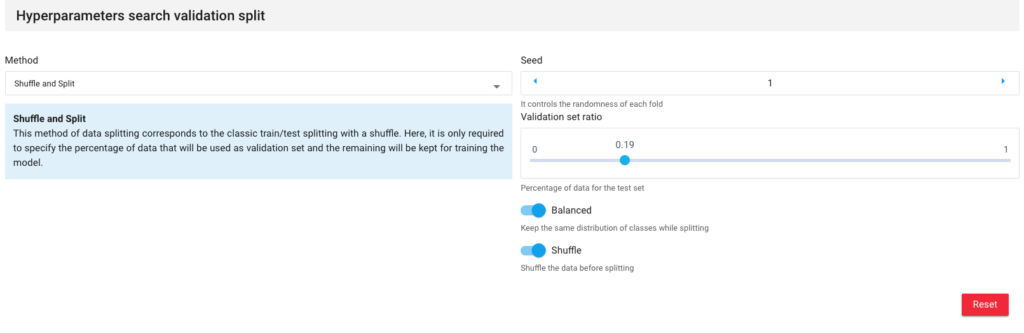
Fourth, and this is a very important step when we have data with an unbalanced target class, we choose a data augmentation model. For example, SMOTE allows you to generate fraud data from existing data, so that you have the same number of fraud and non-fraud cases.
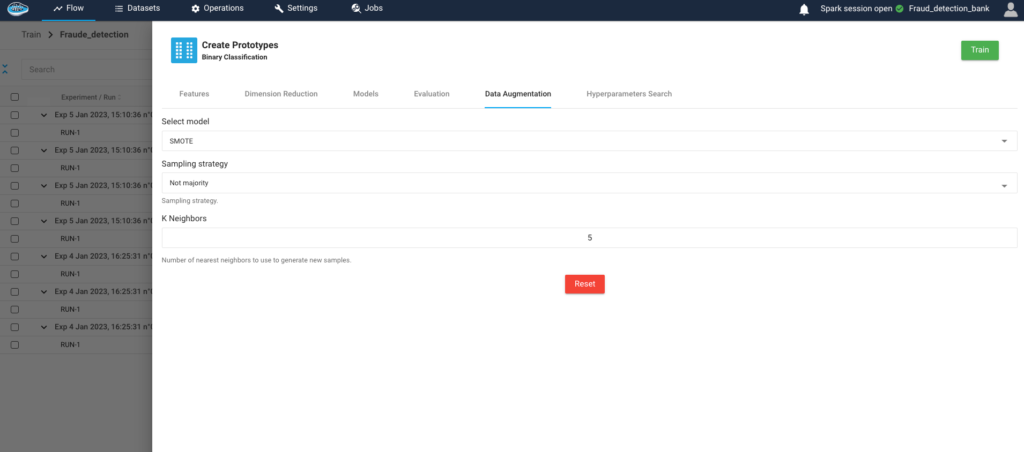
After running several models at the same time, we have a ranking of the results obtained, on which we can notice that the best result was obtained with Random Forest.
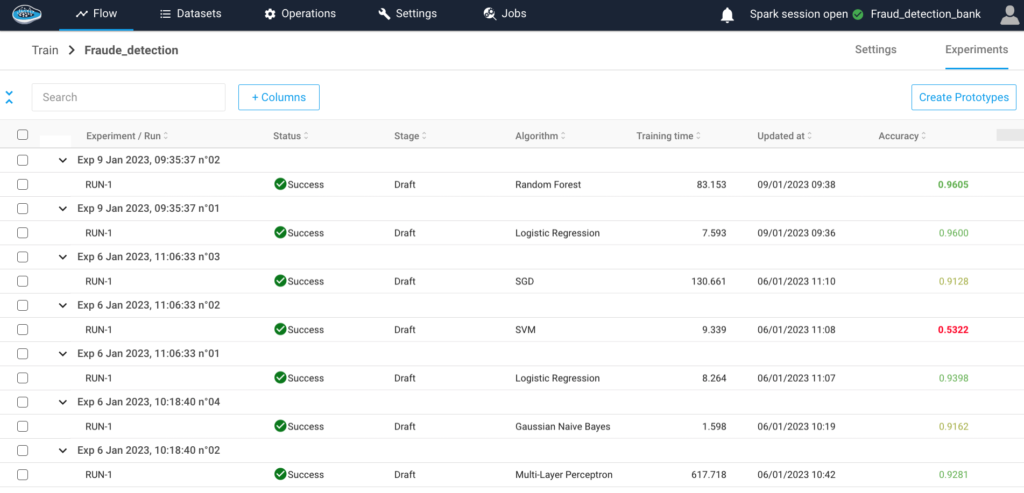
3-Analysis and understanding of the model
a-Evaluation
In order to evaluate the quality of the trained model, papAI solution includes an interpretability and evaluation module for each experiment created. In our case, we ran the Random Forest with which we obtained an accuracy of 96.17%.
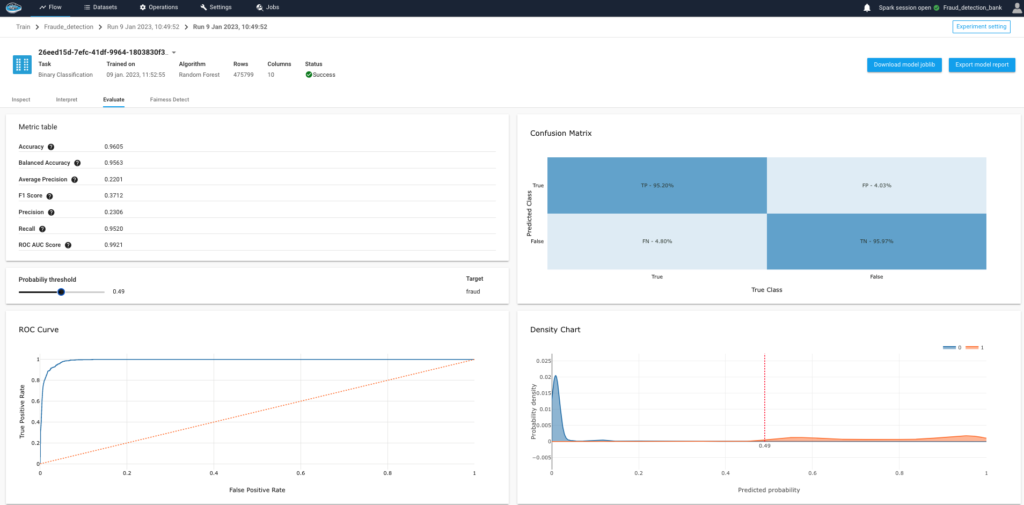
b-Explainability of the model
We can see that the most important feature in determining the value of the target ‘fraud’ is the feature ‘amount’, this feature represents the amount spent in each transaction. the model shows us that fraudsters spend large amounts of money. so the higher the value of the amount, the more suspicious the model is of the transaction
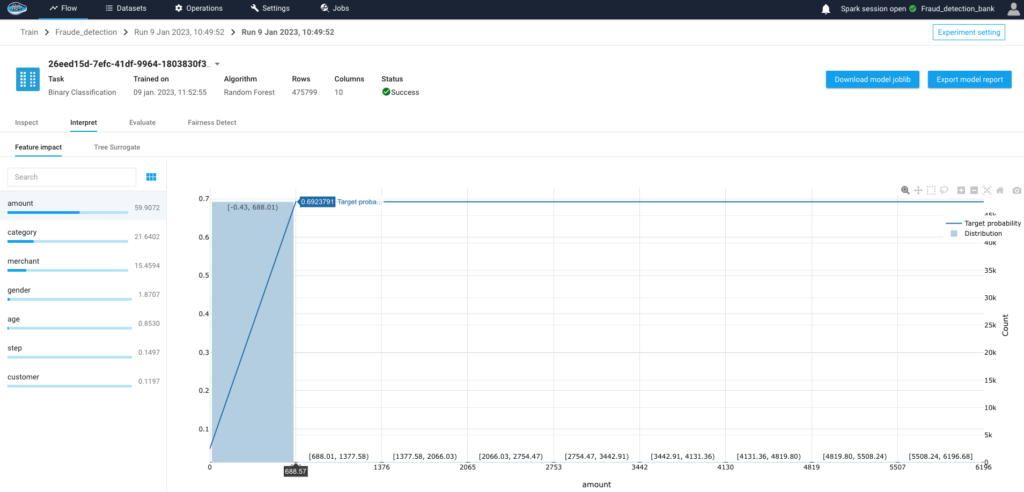
4- Predicting the training set
a-Prediction
The result of the prediction is an output of the test data set with three columns added:
‘proba_0′ the probability that the transaction is a non-fraud case.
‘proba_1′ is the probability that the transaction is a fraud case.
predicted_fraud’ is the value predicted by the model based on the probabilities ‘proba_0’ and ‘proba_1
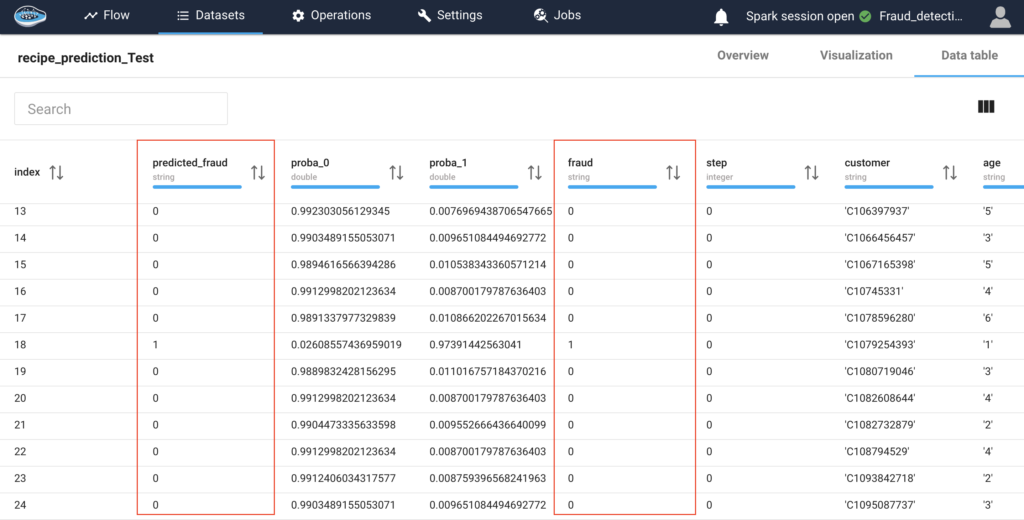
b-Local interpretability
Local interpretability means choosing a specific transaction and seeing the contribution of the characteristics positively or negatively in determining a case of fraud or non-fraud. The image below is a local interpretability of an original fraudulent transaction, we can see that our model chose to classify this transaction as fraudulent because it is a woman who spent $1304.93 in the travel category at a suspicious merchant. and age, which is 5 years old, contributed negatively to the fraud prediction, but with little influence.
In the end, for this transaction, papAI Solution is 94.51% sure that it is a fraud.
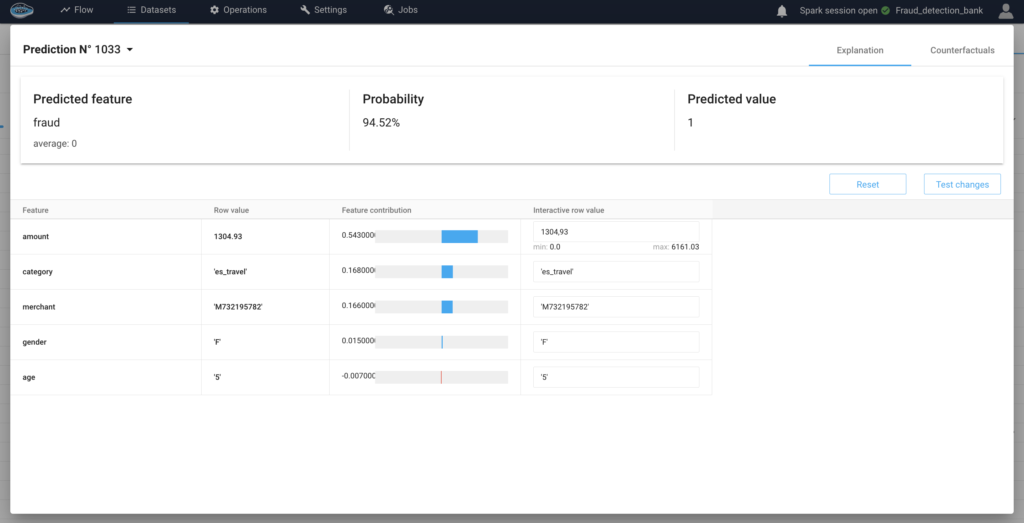
Create your own AI-based tool with papAI solution to improve fraud detection
With papAI solution, you can use AI to improve fraud detection inside your company. You have the chance to design your own AI-based tool using papAI that is suited to your particular requirements. Utilizing cutting-edge machine learning techniques will greatly increase the precision and effectiveness of your fraud detection procedures.
papAI solution helps to explain and make transparent the results through a process of data mining, cleaning, and visualization that can accelerate the deployment of AI projects. Book your demo now. Our team of experts can help you create a custom AI-based tool that meets the unique needs of your organization.
Interested in discovering papAI?
Our commercial team is at your disposal for any questions
How RAG Systems Improve Public Sector Management
How RAG Systems Improve Public Sector Management The most important...
Read MoreScaling RAG Systems in Financial Organizations
Scaling RAG Systems in Financial Organizations Artificial intelligence has emerged...
Read MoreHow AgenticAI is Transforming Sales and Marketing Strategies
How AgenticAI is Transforming Sales and Marketing Strategies Agentic AI...
Read More