The Next AI Frontier: Jean-Marc Briquet Talks to Datategy’s Future...
Read MoreTravel & Tourism : How to predict flight prices from papAI
Table of Contents
ToggleArtificial intelligence (AI) has become a trending word in the travel & tourism industry, with many airlines confident that it will significantly change the way they operate . While some companies are using artificial intelligence to create personalized customer experiences, others are using AI to forecast flight prices and reduce price fluctuations during low-demand periods, which leads to better sales results by reducing price sensitivity during those times of the year.
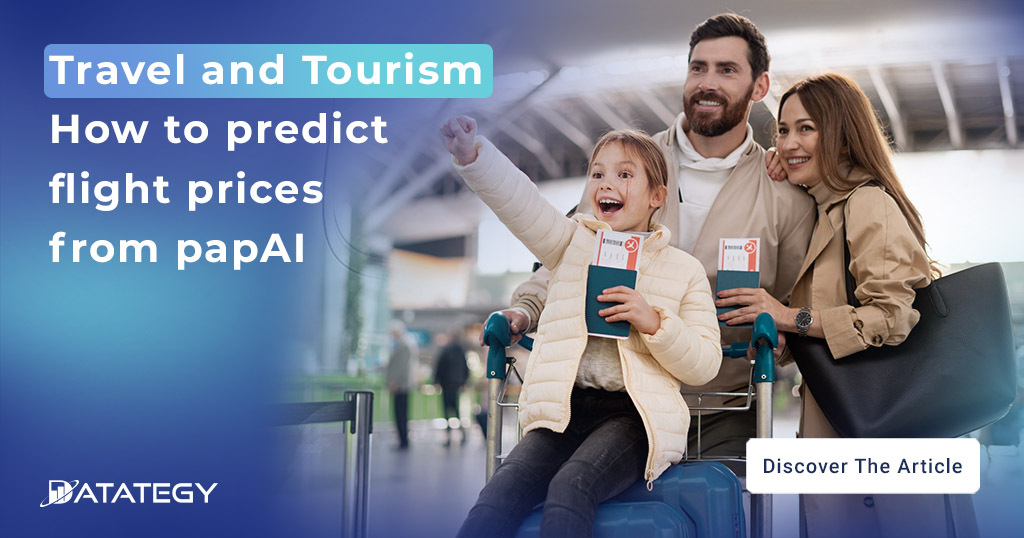
Airlines price forecasting is an important task in the airline industry. In recent years, due to abundant data and advances in computational power, machine learning techniques are becoming more suitable replacements for traditional forecasting methods. In this use case, we propose a new method combining artificial intelligence and machine learning to create an effective price forecasting model using historical airfare data. Our results show that this method can accurately predict future flight price changes with higher accuracy than traditional models.
What are the benefits of flight prices forecasting for airlines ?
Flight prices forecasts are one of the most important pieces of data for airlines, as prices play a crucial role in determining booking decisions and revenues. Airlines that have access to this information can have a significant advantage in several ways:
- Increase revenues: Flight prices forecasting can help airlines maximize revenues by identifying when prices are too high or low and adjusting them accordingly.
- Reduce operations cost: The artificial intelligence based price forecasting can potentially improve the accuracy of flight prices and save airlines time and costs to conduct operations by optimizing their routes while planning.
- Improve the customer experience: With better price prediction, the customer experience becomes more and more optimal by offering travellers to book very long term tickets with the best price reference.
- Meeting government regulatory requirements: The major airlines are required to meet government regulatory requirements which include profitability, revenue forecast, and cost control. The artificial intelligence is applied to flight price forecasting for airlines with the purpose of meeting these regulation requirements.
1 - Context
To determine a flight prices, different vectors come into play such as: the date chosen whether it is in holiday period or not, the airline, the city of departure and the city of arrival, the class chosen ‘economy or business, the number of days remaining until the date of reservation ….. etc.
Many websites or applications provide flight price simulations for their users, these simulations sometimes lack precision, which is why we will use papAI platform to demonstrate its power compared to conventional tools. For this use case we have used the flight booking data set obtained from the website “Ease My Trip” which is an Indian online travel company.
This dataset contains information about air travel between six major cities of India ” Mumbai ” ” Delhi ” ” Bangalor” “Kolkata” ” Hyderabad” ” Chenai “. There are 300 261 data points and 11 features in the dataset.

2 - Data analysis
papAI platform offers an automatic 2D, 3D or cartographic visualization module if we put information on the latitude and longitude of our information. Thanks to this module you can make analyses on your database in order to adapt better. In the following histogram, you can visualize the distribution of flights according to the airline companies.

It can be seen that the company with the most domestic flights is ” Vistara ” with 5616 flights, then in second place you can see ” Ai_india ” with 4425 flights and in third place it is ” Indigo ” with 3557 flights.
3 - Division into learning and test bases
The purpose of papAI platform is to perform data science operations without having to code, so in order to split the database into two tables, you can select the source table, then select the split rows operation. with 88% for training and 12% for testing

In order to have a balanced database, you will need to select the stratified method, and for this case, we have chosen the class column which has two unique values ” Business ” with expensive prices, and ” Economy ” with affordable prices. This will allow the model to train on both high and low values, making it effective with all possible values.
4 - Training Data
a) Choose the model
The problem is to predict flight prices which is a continuous value for each ticket, so the machine learning model that will fit our need will be the application of a ” Regression “. The target variable would be ” Price “. All features could be used in the model except ” Flight ” which represents the flight number and has values that increment from one flight to the next.
papai platform has several regression models, you can choose a few and run them at the same time, to make a comparison on the results afterwards.

b) Validation of the model
After running several models at the same time, we have a ranking of the results obtained, on which we can notice that the best result was obtained with ‘Decision tree’ and ‘Random forest’ in dark green.

5- Evaluation and interpretation of the result
a) Evaluation
In terms of evaluation, papAI platform displays a metric table for each model, a plot of ” Predicted ” vs ” True values ” and other evaluation parameters. In the metric table it is mentioned that the model has 97.65% accuracy, which is a very good result, and on the visualization you can see the blue points which are in majority and which are close to the red line which represents the real values present in our training database.

b) Interpretability
In order to be interpretable, papAI platform exposes for each model a feature importance module (below) and a tree surrogate that shows us the values that will be predicted for each set of values. In the feature impact, you can see that the selected ” Class ” is the feature with the most impact on the ticket price which is logical as there is a grant interval between economy and business class, then the second feature is the ” Duration “, which represents the duration of the journey.

6 - Predict the training set
The result of the prediction is an output of the test dataset with an additional ” Price_prediction ” column that represents the predicted values for each ticket, in the following image you can see the real price of the plane, and the value predicted by papAI platform.

Forecasting offers a major opportunities to airlines, since it can allow them to predict how much revenue they will make based on prices in the future. Accurate (or at least reliable) forecasts would give airlines the ability to adjust their pricing accordingly and reduce losses, on average. This has led to a surge in research into flight price forecasting using artificial intelligence (AI).
Interested in discovering papAI ?
Our commercial team is at your disposal for any question
How AI Transforms Production Lines into Smart Manufacturing Systems
How AI Transforms Production Lines into Smart Manufacturing Systems AI...
Read MoreHow Artificial Intelligence Simplifies Spare Part Searches
How Artificial Intelligence Simplifies Spare Part Searches Artificial intelligence is...
Read More