How Law Firms Use RAG to Boost Legal Research RAG...
Read MoreHow Law Firms Use RAG to Boost Legal Research
Table of Contents
ToggleRAG (Retrieval Augmented Generation) is a key technology that enhances search using proprietary data across all domains, and the legal industry is no longer waiting. The legal industry needs to ride the new wave of AI technology because the sheer volume of legal documents, case files, and regulatory information is becoming overwhelming.
Legal professionals must take advantage of AI’s capacity to effectively sort through this data in order to remain competitive and deliver precise, timely counsel. In an industry where accuracy and dependability are critical, AI and RAG in particular offer a means of firmly establishing AI solutions in internal, trusted knowledge stores.
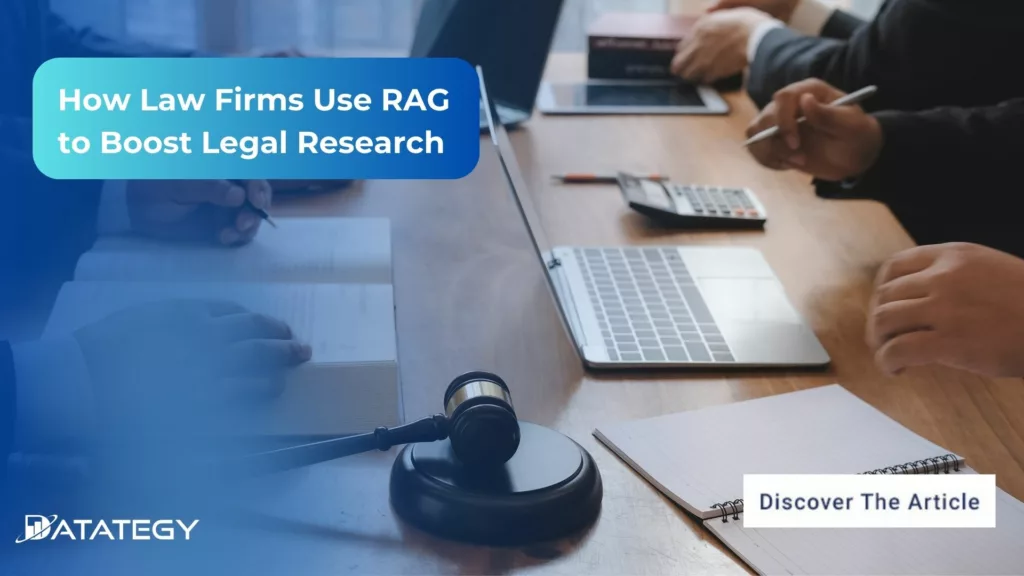
In this article, we will look at how RAG is reshaping legal research and practice. We’ll explore the specific ways it can streamline tasks
What does RAG mean?
RAG, or Retrieval Augmented Generation, essentially describes a way to make AI language models more knowledgeable and reliable by giving them access to external information.
Think of it like this: instead of relying solely on the data they were trained on, which can become outdated, RAG allows them to pull in relevant facts and context from a separate, up-to-date database. T
his means when you ask a question, the AI doesn’t just guess or rely on potentially stale information; it actually goes and finds the answer in real-time, using your own data, or other trusted sources.
What Are a RAG System's Fundamental Elements?
Embedding Models
Embedding models, which transform raw text into dense vector representations that let computers to understand and manipulate word and phrase meaning, are a crucial component of RAG systems. Quick generation and retrieval of relevant information is made possible by these embeddings, which capture the semantic relationships between words. It is essential to select an embedding model that is suitable for the specific task at hand.
For instance, models like MistralAI or GPT are commonly used because of their ability to provide contextual embeddings of the highest caliber. Retrieval accuracy and answer relevance are improved by optimizing these models on task-specific data since they capture the nuances of the field.
Retrievers Systems
In a RAG system, the retriever is the first step of the RAG pipeline and is responsible for locating and obtaining relevant information from a vast amount of data. Sparse retrieval and dense retrieval are the two main methods that retrievers often use. Sparse retrieval, like BM25, is based on keyword matching and is ideal for large document searches where speed is a major consideration.
On the other hand, dense retrieval enhances its capacity to capture query context and complexity by matching learned embeddings with semantic meaning. The ability of the retriever to select relevant, high-quality data is critical to the overall performance of the system since it directly impacts the quality of the response generated by the subsequent generation.
Generators
The generator in a RAG system is responsible for converting the relevant information that the retriever has acquired into text that is grammatically correct, appropriate for the context, and readable by humans. A powerful generator is necessary when dealing with complex queries or large amounts of data in order to ensure that the RAG system produces results that appear authentic.
Generators frequently use large language models, such as GPT or T5, to generate text based on the collected data. Certain output types, such as creating debates, summarising papers, or answering questions, can be better handled by modifying these models.
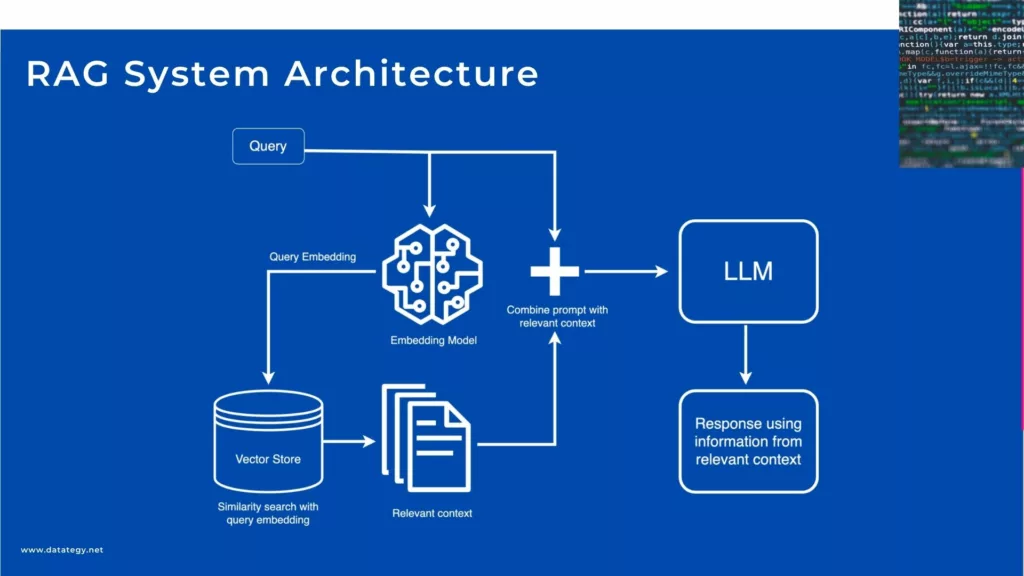
RAG System Architecture
What are the Advantages of Implementing RAG in law firms
Enhanced Legal Research Capabilities
It is always expected of legal professionals to provide well-reasoned opinions, motions, and strategies based on a thorough comprehension of statutes, case law, and precedents unique to their company.
Historically, this requirement has necessitated hours of in-depth, laborious study on several platforms. By combining AI-powered contextual comprehension with real-time document retrieval, RAG systems radically alter this procedure.
RAG is powered by a dual engine that, in response to the user’s inquiry, first gets highly relevant documents from carefully selected sources and then produces natural-language responses that understand those materials.
Lawyers no longer have to search through dozens of documents in search of a single paragraph that can be used. Rather, a well-crafted inquiry can provide succinct responses that include important legal reasoning, citations, and contextual framing.
Time and Cost Efficiency
The increase in time and cost efficiency is among the most obvious and quantifiable effects of RAG implementation in law firms. Legal work involves a lot of paperwork by nature, and many of the everyday tasks such as analyzing contracts, finding terms, and monitoring compliance changes are repetitious but necessary. Numerous hours of human labor are required for these jobs, especially by junior employees.
By automating a large number of these laborious tasks, RAG lessens this load. RAG works quickly and accurately, whether it’s summarizing long contracts, evaluating regulatory terminology, or pointing out discrepancies in client agreements. By enhancing productivity per person, this automation allows legal teams to manage more cases or deliverables without adding more staff.
Accelerated Decision-Making
RAG allows legal teams to quickly obtain synthesized outputs from hundreds of pages of statutes, contracts, and case law.
This shortens the time to insight at crucial points, such as compliance reviews, discussions, or emergency filings. Without assigning research or waiting hours for a memo, a partner preparing a client briefing, for instance, might ask a question and get a structured summary of the jurisprudence or contract consequences.
Additionally, the technology facilitates improved team alignment.
Decisions are made more quickly and unanimously when all parties involved, clients, analysts, and lawyers have access to the same consistent, well-synthesized information. As a result, legal firms can respond more quickly, lower risk, and operate more confidently under duress.
Scalable Knowledge Management
Law firms accumulate a vast quantity of internal knowledge over time—ranging from case strategy notes to specialist legal interpretations and client recommendations.
However, a large portion of this data is still fragmented, kept in disparate systems, or obscured in old records. RAG changes the way that legal organizations handle and apply this information.
RAG makes it possible to uncover information that might otherwise go unnoticed by providing a semantic, context-driven search. By asking natural-language questions, attorneys can obtain pertinent internal precedents, contract templates, or client histories. The algorithm produces accurate and useful results by comprehending firm-specific details as well as legal terms.
How Law Firms Use RAG Technology
1- For Lawyers & Legal Associates
Legal Research & Case Law Retrieval
Retrieving case law and conducting legal research are two of RAG’s most potent applications for lawyers. Lawyers no longer have to spend hours searching through databases like Westlaw or LexisNexis; instead, they can now ask natural language inquiries of an AI-powered assistant.
They could enquire, “What are the key precedents in EU data privacy law concerning biometric data?” for instance, and get a summary together with context and connections to sources.
RAG does more than merely extract data; it eliminates irrelevant information and highlights the most pertinent, referenced content. This enables attorneys to focus more on strategy and less on search and get to the crux of the matter much more quickly.
Drafting Legal Opinions & Memos
The creation of internal memos and legal opinions comes next. RAG can automatically incorporate references to cases or legislation into structured drafts created from a lawyer’s notes or voice recordings. AI should assist in creating a solid foundation rather than writing legal documents from scratch because no one will trust a bot to do that.
The draft can then be reviewed, edited, and polished by attorneys using their knowledge. The advantage is obvious: less time is spent on the preliminary hard work and more time is spent on high-value thinking.
Brief Preparation
Building on the idea of RAG’s impact on legal brief preparation, imagine the potential for increased consistency and quality across a firm’s output. By leveraging a well-maintained RAG system, even junior associates can quickly access and incorporate the firm’s best practices and established arguments into their briefs.
This not only speeds up the process but also helps to ensure that all documents adhere to a high standard of legal reasoning. Furthermore, it facilitates a smoother knowledge transfer within the firm, as experienced lawyers’ insights and strategies are effectively embedded within the system, making them readily available to everyone.
This fosters a collaborative environment where legal expertise is shared and amplified, ultimately leading to more compelling and persuasive legal arguments.
2- For Paralegals & Legal Analysts
Document Review & Summarization
Consider a situation when a policy analyst has to know the background information on a certain rule. An analyst may obtain a thorough understanding in minutes by using a RAG-powered internal search engine to swiftly retrieve all pertinent memoranda, reports, and previous interpretations, saving hours of time spent searching through old records and disjointed databases.
In a similar vein, a caseworker handling a complicated case may quickly consult all pertinent policies, court rulings, and client records, enabling them to make quicker and better choices.
This improved information availability empowers workers, cuts down on research time, minimizes mistakes, and eventually boosts government operations’ overall effectiveness.
Due Diligence Automation
One of the most time-consuming phases of any transactional or litigation-related legal process is due diligence. Legal experts must review a plethora of documents, from contracts and leases to financial reports and governance records, in order to spot liabilities, inconsistencies, and strategic red flags, whether for mergers and acquisitions (M&A), regulatory filings, or litigation assistance.
A paradigm shift in the way legal teams carry out this task is brought about by RAG. RAG is capable of processing and analyzing thousands of documents with exceptional speed and accuracy by combining natural language production with intelligent retrieval technologies.
With the use of the technology, analysts may swiftly identify all pertinent instances within a document set and specify certain due diligence criteria, such as indemnity risks, exclusivity conditions, or change-of-control clauses.
Contract Comparison & Clause Extraction
Standardized contracts are the cornerstone of managing relationships with partners, clients, and suppliers in many legal departments.
However, whether deliberate or unintentional, linguistic errors can pose serious financial and legal consequences. Comparing agreements by hand to find these differences takes a lot of effort and is prone to mistakes, especially when there are many comparable contracts.
Retrieval-Augmented Generation uses intelligence and automation to overcome this difficulty. When a set of papers is provided, RAG can compare each clause to a baseline template or earlier iterations of the agreement. The system draws attention to both structural and semantic differences, such as changed indemnity agreements, changed payment terms, or missing confidentiality clauses.
3- For Legal Operations & IT Teams
Knowledge Management Systems Enhancement
Retrieval-Augmented Generation (RAG) offers a significant upgrade to the internal knowledge management systems. By combining natural language processing with intelligent document retrieval and generation, RAG allows legal operations and IT teams to implement semantic search capabilities that understand the intent behind user queries rather than merely matching keywords.
For example, if a legal professional enters the query: “How has our firm handled similar intellectual property disputes in the past five years?”, a RAG-powered system can retrieve relevant documents from internal archives, summarize previous case strategies, and even highlight trends or outcomes—all within seconds.
The system reads the underlying documents and composes a contextually accurate response based on verified content, reducing the time spent searching and interpreting results.
Compliance Monitoring
In the legal field, maintaining compliance calls for ongoing attention to detail. Law companies must make sure that their internal rules and client advisory are continuously in line with the most recent legal requirements because regulatory frameworks change often, whether as a result of evolving ESG principles, financial reporting standards, or data protection legislation.
RAG makes it possible to check compliance in a more automated and proactive manner. A RAG-based system can regularly check for changes in pertinent legislation, extract and summarise updates, and compare them with the firm’s operational policies by combining internal policy papers with external legal databases and government publications.
Client Onboarding & Chatbot Support
In legal companies, client onboarding is an essential but frequently time-consuming procedure. Obtaining background information, outlining the specifics of the legal issue, responding to procedural enquiries, and making sure the client is directed to the appropriate department are all common tasks of the initial intake. These encounters, which are typically conducted by phone, email, or manual forms, can result in delays and inefficiencies, particularly during busy times.
Law businesses can implement more intelligent chatbot and virtual assistant systems by utilising Retrieval-Augmented Generation. RAG-based systems comprehend context and can produce correct, nuanced responses based on real-time data retrieval from external legal information and internal knowledge bases, in contrast to standard chatbots that rely on strict decision trees or keyword triggers.
What are the Challenges in implementing RAG in Legal Research?
AI Hallucinations
The appearance of “hallucinations” is one of the most urgent issues when implementing AI in the legal field. These happen when an artificial intelligence (AI) system, particularly one that is based on extensive language models, produces content that seems authoritative and logical but is either wholly made up, factually inaccurate, or illegal. Such mistakes can have major repercussions in a field where accuracy and precision are essential.
Hallucinations can cause citations of cases that never happened, misunderstandings of legal doctrine, or improper application of statutes in legal contexts. When AI tools are utilized to create motions, compile case law, or offer compliance advice, this danger is increased. If overlooked, a single hallucinated clause or precedent might undermine a firm’s reputation or even put a client’s position in danger.
Jurisdictional Nuances
In addition to retrieving accurate sources, AI models also need to be aware of the legal environment in which they function. For example, laws in Quebec may disregard or even contradict a precedent that applies in Ontario. A federal decision may not be upheld in a state court in the United States. Ignoring these subtleties could lead to poor strategic choices or advice.
A robust RAG deployment in law firms must include jurisdiction-specific datasets and localized retrieval engines. Additionally, attorneys should make sure their AI assistants are up to date on the latest legal interpretations and pertinent procedural differences for each area they serve.
Law firms can use AI without sacrificing the integrity of legal reasoning across various legal ecosystems by tackling jurisdictional differences head-on.
Data Security Concerns
For RAG systems to work well, access to client files and internal documentation is frequently necessary. As a result, businesses need to be careful about how this data is accessible, where it is kept, and if the systems comply with applicable bar association regulations as well as laws like the CCPA and GDPR. Strict API governance, role-based access rules, and encryption must be commonplace.
Additionally, the security posture of vendors offering AI solutions including audit trails, data segregation policies, and certifications such as ISO 27001 should be assessed. For really sensitive issues, on-premise installations could be the better choice, but secure private-cloud solutions can strike a compromise between control and scalability.
Integration of AI must be viewed by law firms as more than just a technical choice.
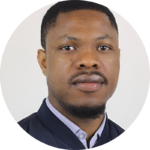
“RAG isn’t just a retrieval method, it’s the bridge between static knowledge and dynamic insight. It empowers AI to reason with relevance, not just recall.”
Kenneth EZUKWOKE
Data Scientist
A to Z of Generative AI: An in-Depth Glossary
This guide will cover the essential terminology that every beginner needs to know. Whether you are a student, a business owner, or simply someone who is interested in AI, this guide will provide you with a solid foundation in AI terminology to help you better understand this exciting field.
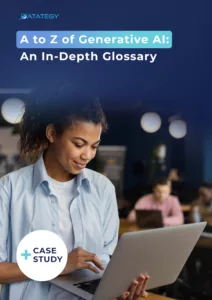
How papAI Helps you to Build Rag Systems ?
papAI is an all-in-one artificial intelligence solution made to optimize and simplify processes in various sectors. It offers strong data analysis, predictive insights, and process automation capabilities.
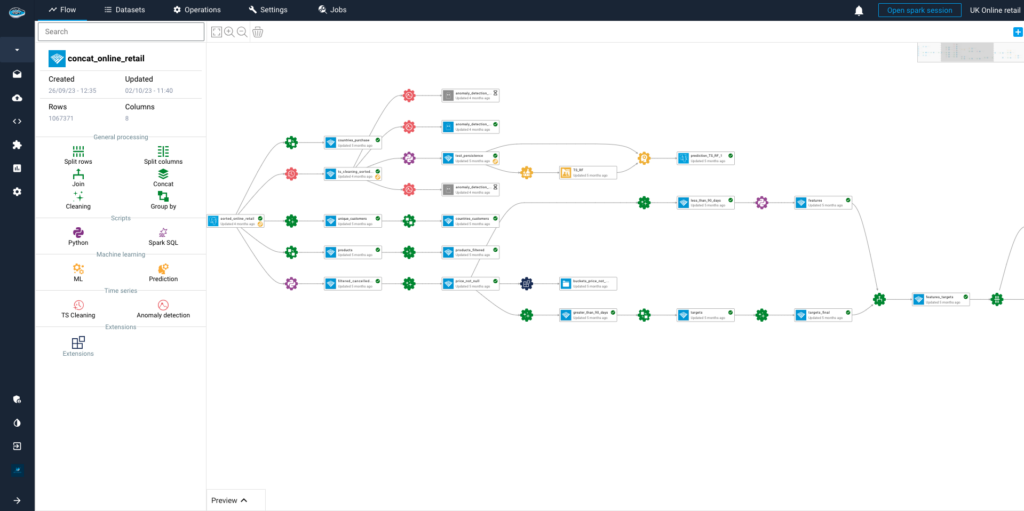
Here’s an in-depth look at the key features and advantages of this innovative solution:
What are the key factors that differentiate Datategy's RAG approach?
Datategy’s approach to building Retrieval-Augmented Generation (RAG) the Hyper-layered Asynchronous Hybrid RAG (HAH-RAG) represents a significant leap in how large-scale legal and enterprise documents are processed, understood, and used to generate high-quality answers. This innovation responds directly to the limitations faced by traditional RAG pipelines, especially when scaling to massive document repositories and maintaining context relevance.
Traditional RAG frameworks often falter when asked to process expansive knowledge bases. They either lose context by overly chunking documents or sacrifice accuracy for speed in their retrieval phase. HAH-RAG avoids this trap by distributing the workload across hyper-layered retrieval tiers. These layers distinguish between high-level topics and granular subcomponents within documents, ensuring that only the most relevant and contextual passages are retrieved.
This intelligent layering helps maintain both global and local document context, allowing the system to be exponentially scaled while preserving precision. For law firms, this means being able to work with massive sets of contracts, case law, or compliance archives without the usual degradation in relevance or response quality.
1- Optimizing Document Retrieval
papAI-RAG combines papAI’s sophisticated capabilities for preprocessing with an improved asynchronous vector database search, enabling a powerful dual-approach system for seamless retrieval. papAI’s modular workflow would handle chunking and indexing while maintaining clear data lineage with its hyper-layered approach that enables multi-granularity search across different data levels.
The key benefits include significantly faster retrieval times (64.5% latency improvement), better data quality through papAI’s preprocessing, and more comprehensive search results through the combined retrieval methods.
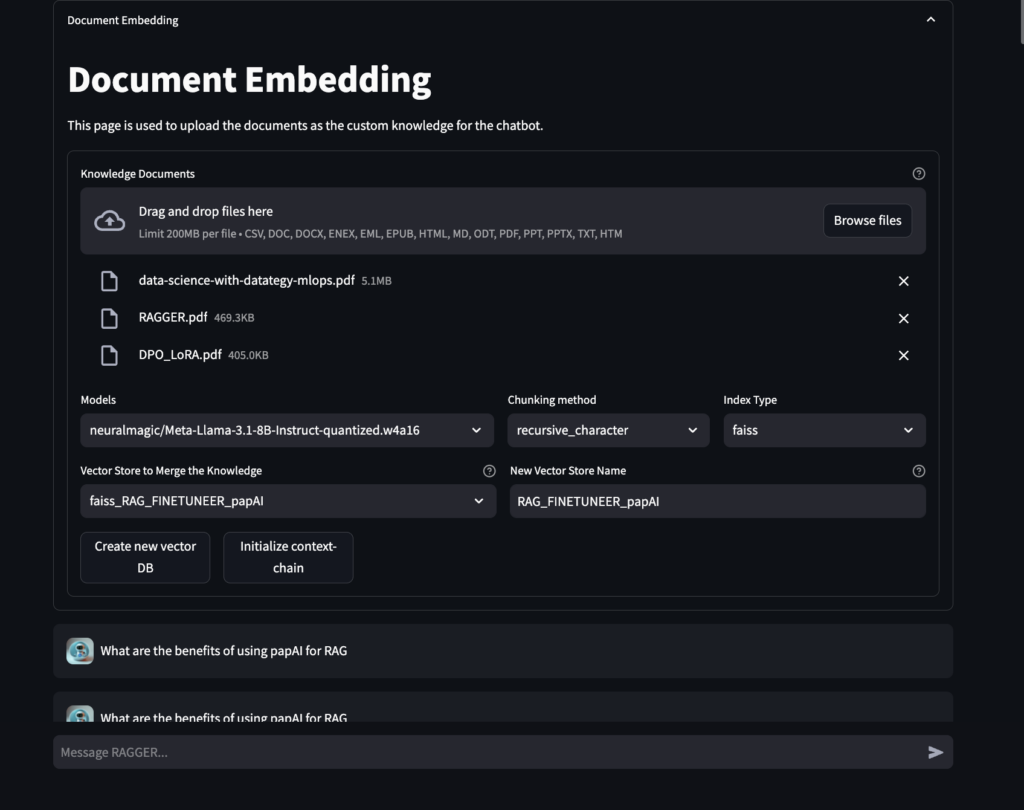
2- Response Generation
the combines papAI-RAG leverages papAI’s data validation and quality control features alongside advanced weighted reciprocal rank fusion and reranking methods for context selection and response generation. Benefits include reduced hallucination rates, improved response relevance, better explainability through visualizations, and a 66.6% reduction in energy consumption compared to traditional RAG approaches.
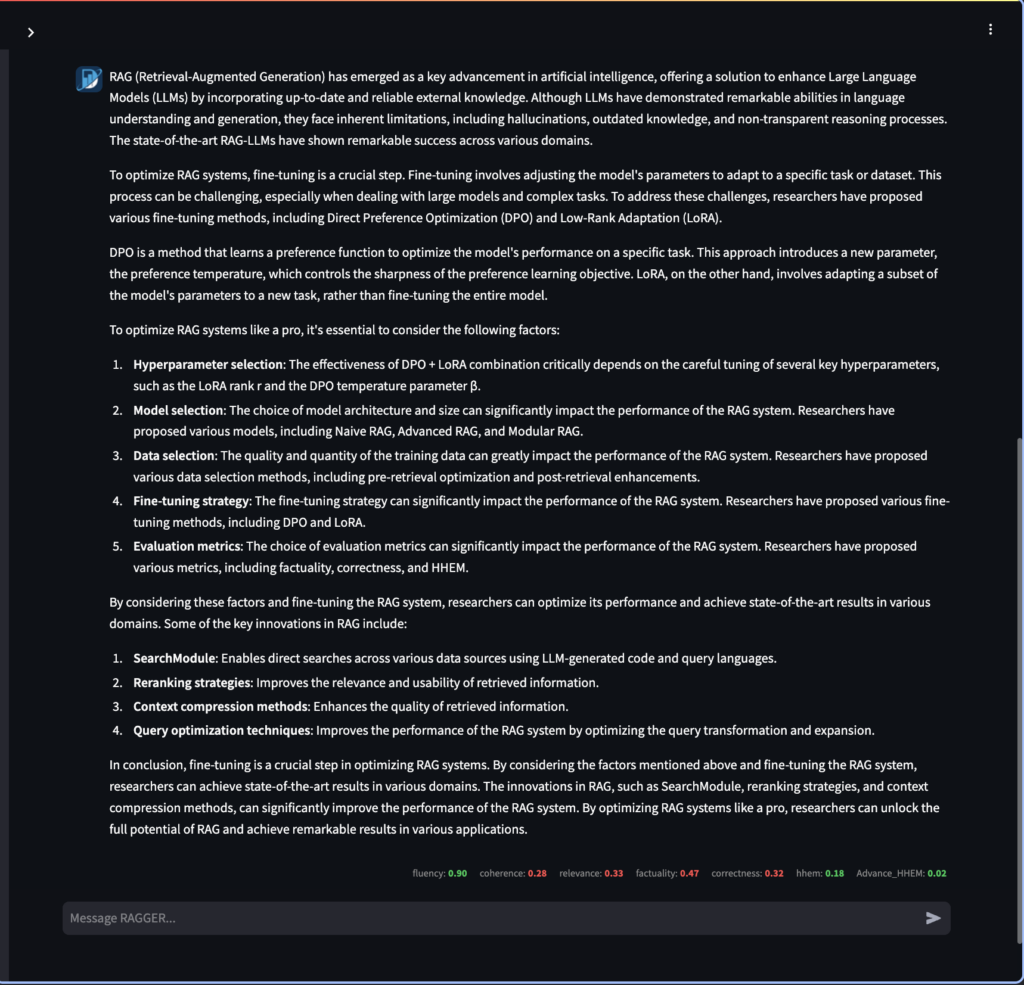
Create Your Own RAG to Leverage Your Data with Datategy
In an era where data is both abundant and essential, law firms and legal departments must go beyond off-the-shelf AI solutions.
With Datategy’s Hyper-layered Asynchronous Hybrid RAG (HAH-RAG), you gain the power to build a Retrieval-Augmented Generation system fully tailored to your internal knowledge, workflows, and regulatory constraints. From enhanced legal research and intelligent contract review to multilingual support and compliance monitoring, our platform offers a robust, scalable foundation designed to transform your firm’s operational intelligence.
Discover how a RAG system, tailored to your organization, can drive the next phase of your operations Schedule a demo to learn more.
The legal industry is overwhelmed by an ever-growing volume of documents, case law, and regulatory updates. Retrieval-Augmented Generation (RAG) enables legal professionals to efficiently navigate this complexity by retrieving and generating accurate , up-to-date information from trusted sources.
- Embedding Models: These transform raw text into dense vector representations, enabling computers to understand the semantic meaning of words and phrases for efficient information retrieval.
- Retrievers Systems: These are responsible for locating and obtaining relevant information from vast amounts of data using methods like sparse retrieval (keyword matching) and dense retrieval (semantic meaning matching).
- Generators: These convert the retrieved relevant information into grammatically correct, contextually appropriate, and human-readable text, often utilizing large language models.
Major challenges include AI hallucinations (inaccurate but plausible-sounding outputs), jurisdictional nuances that require local legal context, and data security concerns around sensitive internal and client information. Addressing these requires localized datasets, robust compliance frameworks, and secure infrastructure often combining cloud and on-premise solutions.
Interested in discovering papAI?
Our AI expert team is at your disposal for any questions
How RAG Systems Improve Public Sector Management
How RAG Systems Improve Public Sector Management The most important...
Read MoreScaling RAG Systems in Financial Organizations
Scaling RAG Systems in Financial Organizations Artificial intelligence has emerged...
Read MoreHow AgenticAI is Transforming Sales and Marketing Strategies
How AgenticAI is Transforming Sales and Marketing Strategies Agentic AI...
Read More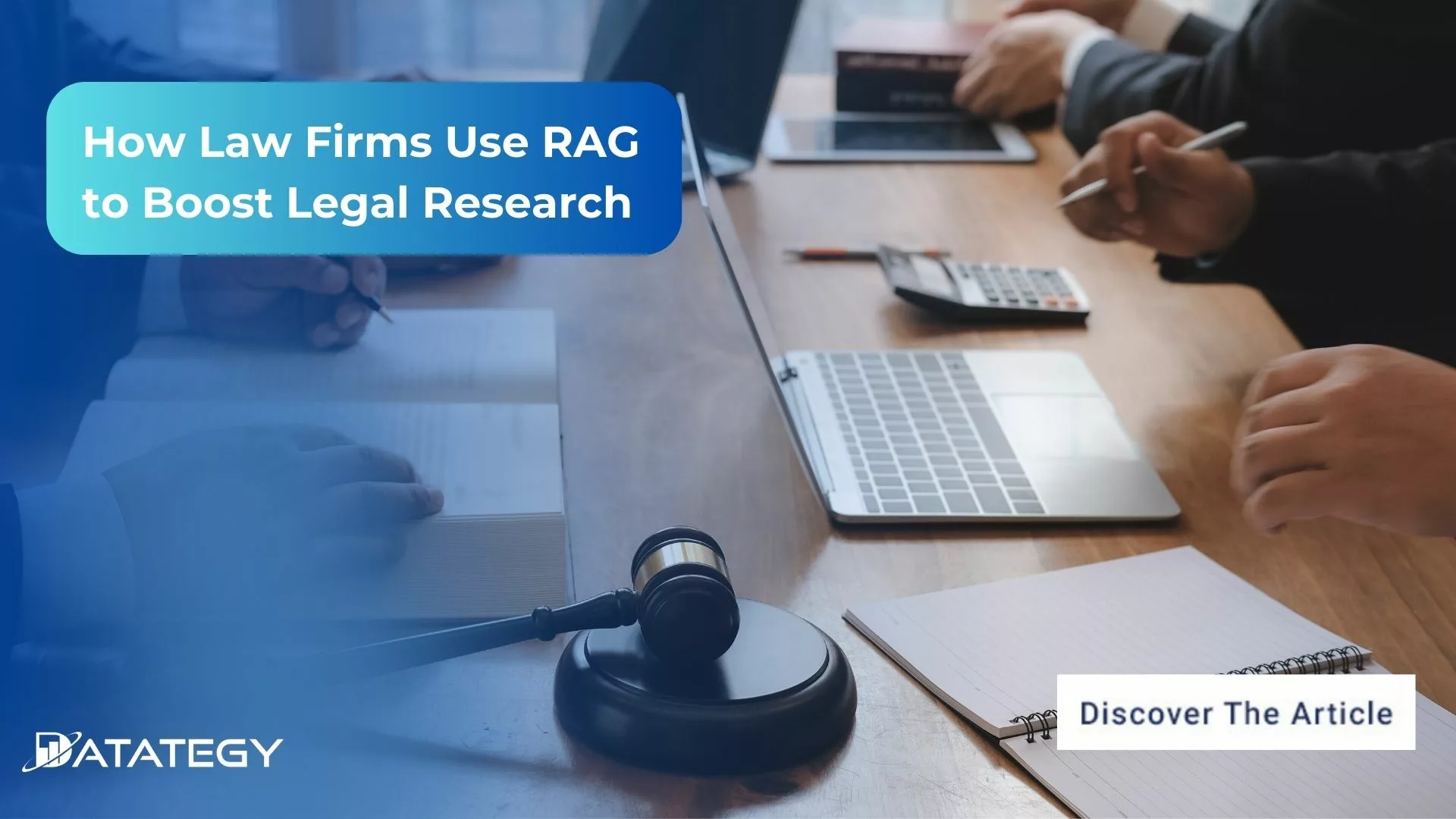
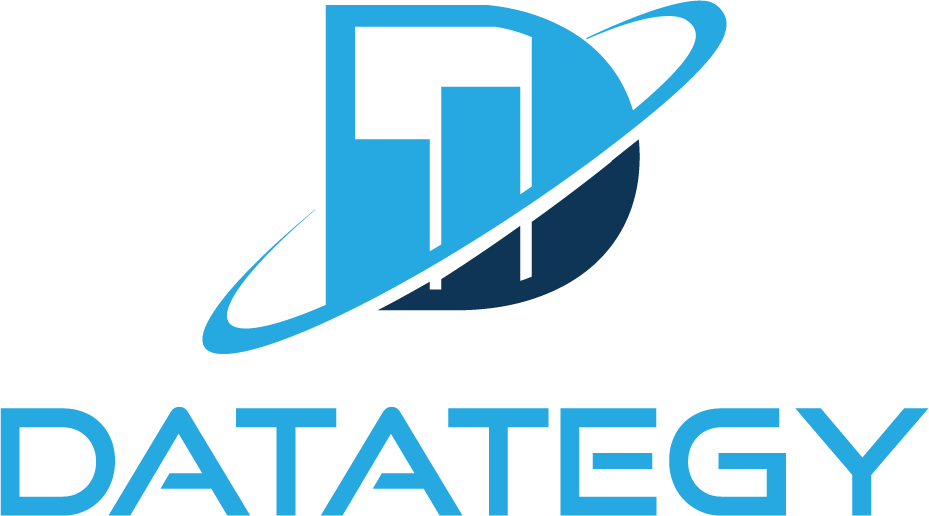