How Law Firms Use RAG to Boost Legal Research RAG...
Read MoreScaling RAG Systems in Financial Organizations
Table of Contents
ToggleArtificial intelligence has emerged as the key tool that every industry needs to improve its operations and customer service. Within this transformative landscape, Retrieval-Augmented Generation (RAG) has rapidly emerged as a pivotal technique, offering a powerful pathway to achieve these crucial improvements with greater speed and reliability.
Retrieval-Augmented Generation (RAG) is a powerful technique that improves the answers generated by artificial intelligence by drawing relevant context from an external knowledge base, usually stored in a vector database.
This dynamic approach allows AI systems to provide more accurate, contextually rich, and up-to-date responses by grounding their generation in verifiable information.
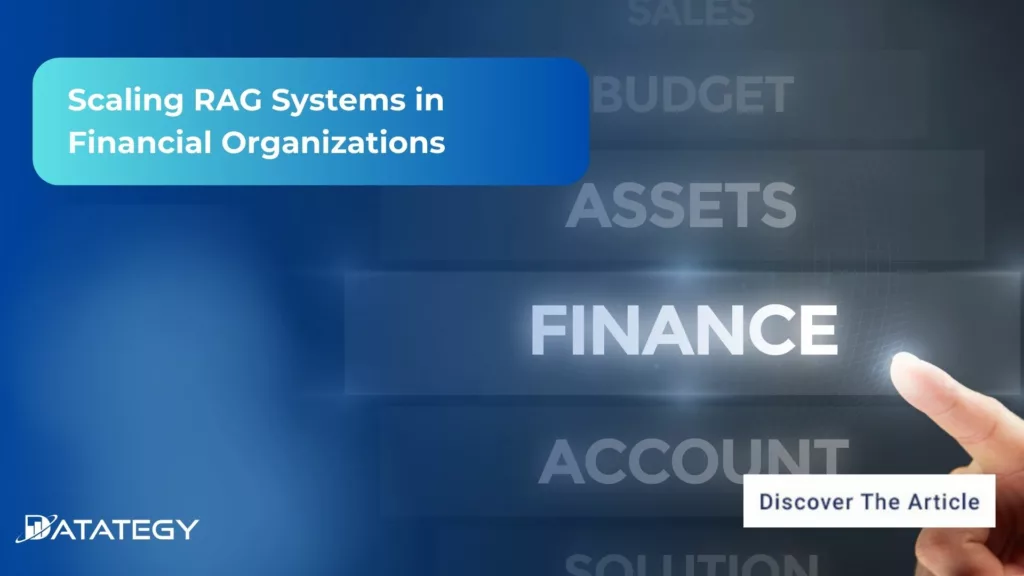
In this article, we will define what RAG means and its implications for financial organizations. Specifically, we will explore how this innovative technology can revolutionize various aspects of their operations.
What does RAG (Retrieval-Augmented Generation) mean?
Retrieval-Augmented Generation (RAG) is a cutting-edge AI technique that incorporates an external retrieval mechanism to improve conventional generative models. Before producing an answer, RAG dynamically extracts pertinent data from a database, documents, or other structured sources, unlike typical AI models that just use their trained knowledge.
This procedure guarantees that the output is more current and contextually precise, which makes it especially helpful for sectors where factual accuracy and real-time information are essential. In financial services, for example, this means AI can retrieve the latest market trends, compliance updates, or risk assessments before making predictions or providing advice.
What Are a RAG System's Fundamental Elements?
Embedding Models
Embedding models, which transform raw text into dense vector representations that let computers to understand and manipulate word and phrase meaning, are a crucial component of RAG systems. Quick generation and retrieval of relevant information is made possible by these embeddings, which capture the semantic relationships between words. It is essential to select an embedding model that is suitable for the specific task at hand.
For instance, models like BERT or GPT are commonly used because of their ability to provide contextual embeddings of the highest calibre. Retrieval accuracy and answer relevance are improved by optimizing these models on task-specific data since they capture the nuances of the field.
Retrievers Systems
In a RAG system, the retriever is the first step of the RAG pipeline and is responsible for locating and obtaining relevant information from a vast amount of data. Sparse retrieval and dense retrieval are the two main methods that retrievers often use. Sparse retrieval, like BM25, is based on keyword matching and is ideal for large document searches where speed is a major consideration.
On the other hand, dense retrieval enhances its capacity to capture query context and complexity by matching learned embeddings with semantic meaning. The ability of the retriever to select relevant, high-quality data is critical to the overall performance of the system, since it directly impacts the quality of the response generated by the subsequent generation.
Generators
The generator in a RAG system is responsible for converting the relevant information that the retriever has acquired into text that is grammatically correct, appropriate for the context, and readable by humans. A powerful generator is necessary when dealing with complex queries or large amounts of data in order to ensure that the RAG system produces results that appear authentic.
Generators frequently use large language models, such as GPT or T5, to generate text based on the collected data. Certain output types, such as creating debates, summarising papers, or answering questions, can be better handled by modifying these models.
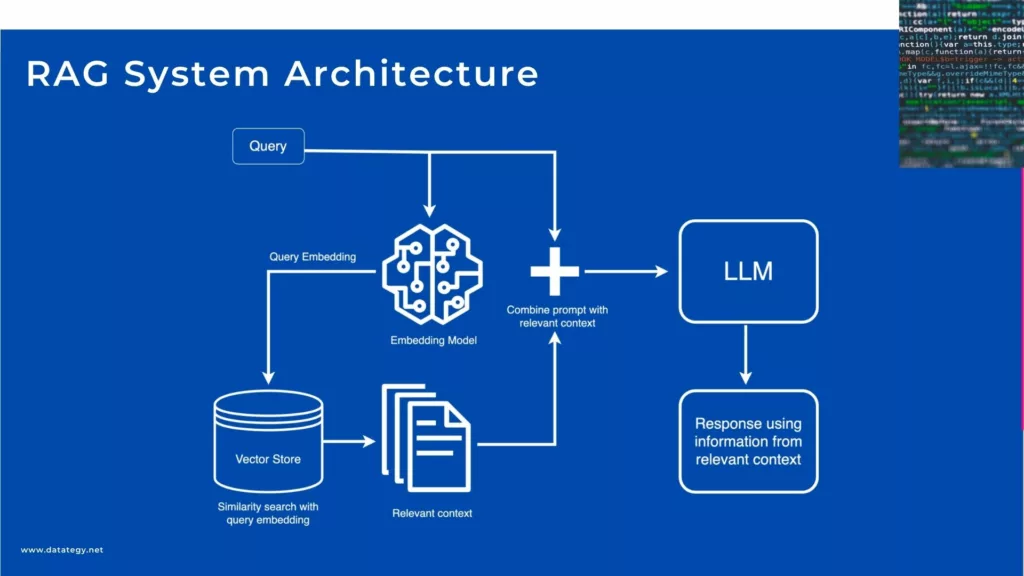
RAG System Architecture
What are the Benefits of Implementing RAG in Financial Institutions?
Standardization of the Level of Information
In financial services, inconsistent or fragmented data can result in errors, inefficiencies, and compliance issues. By guaranteeing that all AI-generated insights are based on the same trustworthy and current data sources, RAG helps standardise the amount of information throughout an organisation.
RAG guarantees consistency in the information being used, minimising inconsistencies and misunderstandings, whether it be in risk assessments, financial reports, or consumer insights.
For example, disparate teams at global financial institutions frequently use disparate data sources, which causes reporting and decision-making to be out of sync. By retrieving the same high-quality data across departments, RAG serves as a unifying framework that guarantees that customer support teams, compliance officers, and financial analysts all work with standardised, validated data.
Improved Decision-Making Through Real-Time Data Access
Since financial services choices frequently entail substantial risks and regulatory considerations, they must be made swiftly and accurately. Decision-making is improved by retrieval-augmented generation (RAG), which gives users instant access to the most current and pertinent data.
Before producing answers, RAG gathers new data from outside sources, including financial reports, stock market updates, and regulatory filings, in contrast to conventional AI models that only use previously learned information. This guarantees that financial experts, ranging from risk managers to investment analysts, base their choices on the most recent information rather than stale or out-of-date data.
By integrating real-time retrieval, RAG enables financial institutions to react faster to market fluctuations, fraud alerts, or regulatory change
Enhanced Accuracy and Efficiency in Financial Analyses
Accurate, consistent, and fast data are essential for financial analysis. Though they frequently have trouble keeping up with new data sources or adjusting to diverse financial settings, traditional AI models can offer broad insights.
By dynamically accessing pertinent financial data prior to producing reports, predictions, or risk assessments, RAG gets around this restriction. By eliminating the need for human data collection and validation, this increases efficiency in addition to improving analysis accuracy.
RAG can also be used by compliance teams to minimise compliance risks by ensuring that risk models are in line with the most recent regulatory frameworks. RAG greatly reduces the burden by automating the retrieval and synthesis of financial data, freeing up professionals to concentrate on strategic decision-making instead of laborious data collecting.
Personalized Customer Experiences and Services
AI is being used by financial institutions more and more to improve client experiences, yet conventional models frequently fall short in offering genuinely tailored recommendations.
By obtaining pertinent, up-to-date information on each individual client, RAG revolutionizes customer interactions by enabling AI to provide recommendations and answers that are customized to meet their unique requirements. This results in a more engaging and customized experience, which raises client loyalty and happiness.
By integrating real-time retrieval with AI-driven personalization, RAG helps financial institutions build stronger, more meaningful relationships with their clients.
What are the Key Applications of RAG in Financial Services?
Credit Risk Assessment
A crucial task in financial services is credit risk assessment, which establishes a borrower’s likelihood of loan repayment. The most recent economic conditions or an applicant’s changing financial status may not always be reflected in traditional risk models, which rely on past data and predetermined criteria.
By obtaining up-to-date financial information, including recent transactions, income patterns, and macroeconomic indicators, RAG improves credit risk assessment prior to producing a risk score or suggestion.
For instance, a RAG-powered system can pull the most recent market conditions, interest rate changes, and even behavioral spending data from numerous sources rather than evaluating a loan application only on the basis of prior credit ratings.
By lowering the chance of default and guaranteeing that credit is given to the appropriate people or companies, helps financial institutions make better-informed lending decisions. Furthermore, it ensures that lenders’ risk models stay current and relevant by assisting them in swiftly adjusting to shifting financial environments.
Enhance Market Research
In finance, market research necessitates ongoing examination of economic indicators, business performance, and industry trends. The enormous volume of new data generated every day frequently makes it difficult for traditional AI models to keep up.
In order to ensure that analysts and investors have access to the most current and pertinent information, RAG solves this problem by retrieving real-time financial records, earnings statements, news, and government policies prior to producing insights.
A RAG-powered system, for example, can be used by an investment business looking at emerging market opportunities to compile and examine recent trade agreements, industry changes, and economic policies.
As a result, market analysis is much better, enabling businesses to make wise investment choices. Additionally, RAG reduces biases that can result from depending just on one dataset by getting data in real-time from numerous sources, producing more thorough and balanced study results.
Fraud Detection and Anti-Money Laundering (AML)
For financial institutions, fraud and money laundering are serious issues that call for advanced detection techniques. Static rule-based systems or machine learning models trained on historical fraudulent activity are the foundation of traditional fraud detection technologies.
Although somewhat successful, these techniques frequently fall short in identifying new fraud trends or changing money laundering strategies.
Before producing risk assessments, RAG dynamically retrieves and analyses transaction information, behavioural trends, and regulatory updates to improve fraud detection and AML operations. For instance, RAG may immediately retrieve information from news sources, internal risk reports, and worldwide financial crime databases if an AI system notices an odd transaction.
This allows RAG to determine whether the transaction is connected to known fraudulent activity. Financial losses are decreased and worldwide regulatory compliance is enhanced when financial institutions use this real-time strategy to detect and stop fraud more quickly.
Investment Advisory and Portfolio Management
To make strategic investment decisions, portfolio managers and investment advisors rely on enormous volumes of data. Conventional AI-driven advisory systems frequently lack real-time insights into market changes, even though they might offer general recommendations based on past performance.
By accessing the most recent economic information, geopolitical events, and stock market trends before producing tailored portfolio recommendations, RAG improves investment advice services.
For example, in order to provide customized investment recommendations, an AI-powered investment assistant can use RAG to examine a client’s risk tolerance, historical investment behavior, and current market conditions.
By offering prompt, thoughtful advice, this not only facilitates better decision-making but also increases client engagement. RAG assists wealth managers and individual investors in optimizing their portfolios in a constantly shifting market environment by fusing generative AI with real-time financial data.
Regulatory Compliance and Reporting
One of the most difficult problems facing the financial services industry is regulatory compliance. Financial institutions must make sure that their operations stay compliant with changing laws and regulations in order to avoid significant penalties and legal repercussions.
Conventional compliance monitoring is ineffective and prone to errors since it frequently entails manual data collecting and drawn-out review procedures.
By obtaining the most recent financial rules, policy modifications, and legal updates prior to producing compliance reports, RAG streamlines regulatory compliance. A bank that uses RAG, for instance, can automatically scan new regulatory rules and compare them to its internal procedures to find any potential risks or gaps.
This lessens the workload for compliance teams while guaranteeing that financial institutions remain current with legal obligations. RAG can also produce thorough audit trails, which helps businesses prove compliance with authorities.
What is the Challenge in implementing RAG in Financial Organizations?
The Importance of Fine-Tuning in RAG
Fine-tuning the generation layer in RAG systems is crucial for financial organizations because it directly impacts the accuracy and relevance of AI-generated responses. While RAG systems retrieve data from various sources, the generation layer determines how that information is synthesized and presented.
Without proper fine-tuning, the AI may generate responses that are overly generic, misinterpret complex financial data, or fail to align with industry-specific terminology. By refining this layer with domain-specific knowledge, financial institutions can ensure that AI-generated insights are precise, contextually appropriate, and actionable for decision-making.
Five Fine-Tuning Strategies for RAG Components
Fine-tuning Embedding Models with Domain Specificity in Mind
Optimizing a RAG system for certain activities or sectors requires fine-tuning embedding models for domain specialization. The purpose of embedding models is to transform textual input into dense vector representations that capture word associations and semantic meanings. Pre-trained models, such as BERT or GPT, are less useful for specialized applications that ask for domain-specific expertise since they are usually trained on huge, generic datasets.
By exposing these models to a smaller, domain-specific corpus, fine-tuning helps close this gap by enabling them to more accurately represent the vocabulary, subtleties, and context of the subject. Optimizing embedding models guarantees that the system can comprehend and interpret domain-specific language, whether in the fields of healthcare, finance, or law. This enhances the precision of information retrieval and the caliber of the generated outputs
Supervised learning is used in the fine-tuning step when a labeled dataset pertinent to the particular domain is used to train the model. These might be legal documents for a task involving the law or medical research articles for a healthcare application. The model’s parameters are changed during fine-tuning in order to better reflect the context and vocabulary semantics of the domain.
Direct Preference Optimization (DPO)
To enhance a model’s performance on a particular job, Direct Preference Optimisation (DPO) is a fine-tuning technique that focusses on learning a preference function. The sharpness of the preference learning aim is adjusted by the preference temperature parameter introduced by DPO, in contrast to conventional fine-tuning methods that only use loss minimization over labeled datasets.
The degree to which the model favors some outputs over others can be adjusted by researchers using this parameter. When it comes to text creation or ranking systems, for example, DPO can adjust the model to better match the intended results by learning to favor particular kinds of replies. This makes it especially helpful for jobs like recommendation systems and conversational AI that call for subjective or nuanced judgments.
By comparing outputs, DPO iteratively enhances the model’s preference for task-aligned outcomes. In recommendation systems, for example, the model may be tuned to give precedence to outcomes that are both contextually relevant and correct. This method guarantees that the behaviour of the model is closely matched with certain goals, improving its applicability and task performance as a whole. Developers may better control the model’s outputs and make more focused and precise optimizations by utilizing DPO.
Low-Rank Adaptation (LoRA)
A fine-tuning technique called Low-Rank Adaptation (LoRA) makes it possible to alter big pre-trained models in an effective and scalable manner. LoRA adds tiny, trainable low-rank matrices to the network in place of changing the whole set of model parameters. Only a portion of the parameters are changed by these matrices, enabling task-specific customization while maintaining the majority of the model’s initial weights.
LoRA is a great option for modifying large models like GPT or BERT to fit specialized domains because of its selective parameter adjustment, which lowers computational overhead and lowers the chance of overfitting. For example, LoRA preserves the broad knowledge of the original model while concentrating primarily on domain-relevant modifications when fine-tuning a language model to specialize in legal or scientific material.
LoRA allows fine-tuning even in contexts with limited resources without compromising accuracy or performance since it is lightweight, resource-efficient, and effective.
Improved Vector Search in RAG Systems
A key component of the retrieval system in a RAG (Retrieval-Augmented Generation) architecture is vector search, also known as semantic search. This part is in charge of finding and collecting pertinent facts from big external knowledge bases, giving the language model the context it needs to produce outputs that are precise, logical, and contextually correct. Vector embeddings, which are high-dimensional numerical representations of words, phrases, or documents that capture their semantic meaning rather than simply their surface-level keywords, are used by the retrieval system to do this.
Using an embedding model, such BERT or sentence transformers, the system first converts a query into a vector representation in vector search. The context, subtleties, and intended meaning of the question are all captured in this vector. A database of pre-computed vectors from texts, articles, or knowledge bases is then compared with this query vector by the retrieval system.
The algorithm ranks and returns the most pertinent texts or passages that closely match the semantic meaning of the query by utilizing similarity metrics like dot product or cosine similarity. This makes it possible for the retrieval system to take into consideration the deeper meanings and relationships between phrases in addition to keyword matching.
Enhancing Generators for Contextual Relevance
Fine-tuning a generator on task-specific data is essential to improving it for contextual relevance. It is necessary to expose the model, which is frequently built on sizable pre-trained architectures like GPT or T5, to a range of example scenarios pertinent to the field in which it will be employed. For example, a dataset of previous client questions and answers might be used to improve the generator in a customer support program.
This enables the model to learn how to respond to various client problems, decipher the subtleties of inquiries, and produce useful and contextually relevant answers. The model gains a better understanding of domain-specific scenarios, specialized vocabulary, and the subtleties of tone or formality required in the outputs through fine-tuning.
Adding features that enable the generator to concentrate on the most pertinent data that the retrieval system has collected is another crucial tactic for improving it. The generator should be taught to recognize and highlight the most important elements of the retrieved information, rather than merely producing text based on a general context.
In order to keep the answer on topic and prevent it from veering into irrelevant or peripheral material, this may include making use of attention mechanisms, which enable the generator to “attend” to particular portions of the input more forcefully. Furthermore, the generator’s replies may be guided to adhere to predetermined patterns by using structured prompts or templates, which enhances coherence and relevancy.
Why is LAFT (Layer Augmentation Fine-Tuning) the most effective approach?
Layer Augmentation Fine-Tuning (LAFT) is the most effective approach because it enhances Large Language Models (LLMs) without altering their core architecture. By adding trainable layers to both the encoder and decoder while keeping the base model’s parameters frozen, LAFT allows for targeted fine-tuning on domain-specific datasets.
This ensures that the model adapts to specialized financial, legal, or technical contexts without losing its general reasoning capabilities. Unlike traditional fine-tuning, which modifies all model parameters and risks overfitting or catastrophic forgetting, LAFT preserves the foundational knowledge of the model while enabling domain adaptation in a controlled manner.
Another key advantage of LAFT is its efficiency in terms of computational resources and training stability. Since only the additional layers are trained, the process requires significantly less data and computing power compared to full fine-tuning.
This makes LAFT particularly valuable for financial organizations, where real-time adaptation to regulatory changes and market conditions is crucial. Moreover, freezing the base model ensures that updates are more stable, preventing unintended drifts in model behavior that could lead to errors in critical financial applications.
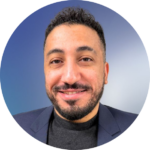
“With LAFT, we bridge the gap between adaptability and stability, enhancing domain-specific performance without compromising the foundational intelligence of large language models.”
Mohamed Elhawary
R&D Data Scientist
A to Z of Generative AI: An in-Depth Glossary
This guide will cover the essential terminology that every beginner needs to know. Whether you are a student, a business owner, or simply someone who is interested in AI, this guide will provide you with a solid foundation in AI terminology to help you better understand this exciting field.
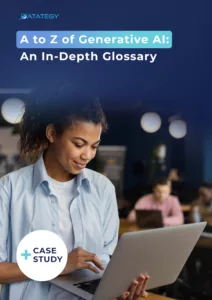
How papAI Helps you to Build Rag Systems ?
papAI is an all-in-one artificial intelligence solution made to optimize and simplify processes in various sectors. It offers strong data analysis, predictive insights, and process automation capabilities.
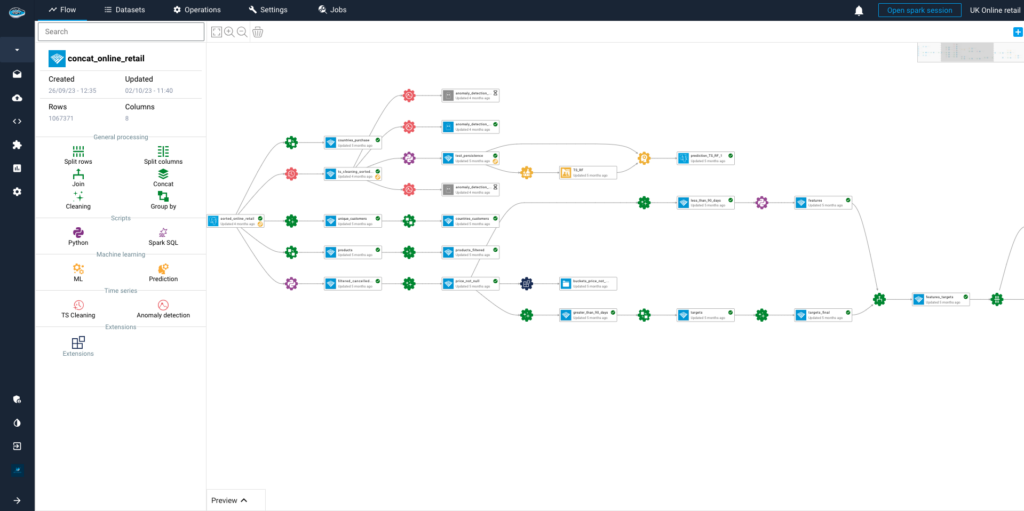
Here’s an in-depth look at the key features and advantages of this innovative solution:
Optimizing Document Retrieval
papAI-RAG combines papAI’s sophisticated capabilities for preprocessing with an improved asynchronous vector database search, enabling a powerful dual-approach system for seamless retrieval. papAI’s modular workflow would handle chunking and indexing while maintaining clear data lineage with its hyper-layered approach that enables multi-granularity search across different data levels.
The key benefits include significantly faster retrieval times (64.5% latency improvement), better data quality through papAI’s preprocessing, and more comprehensive search results through the combined retrieval methods.
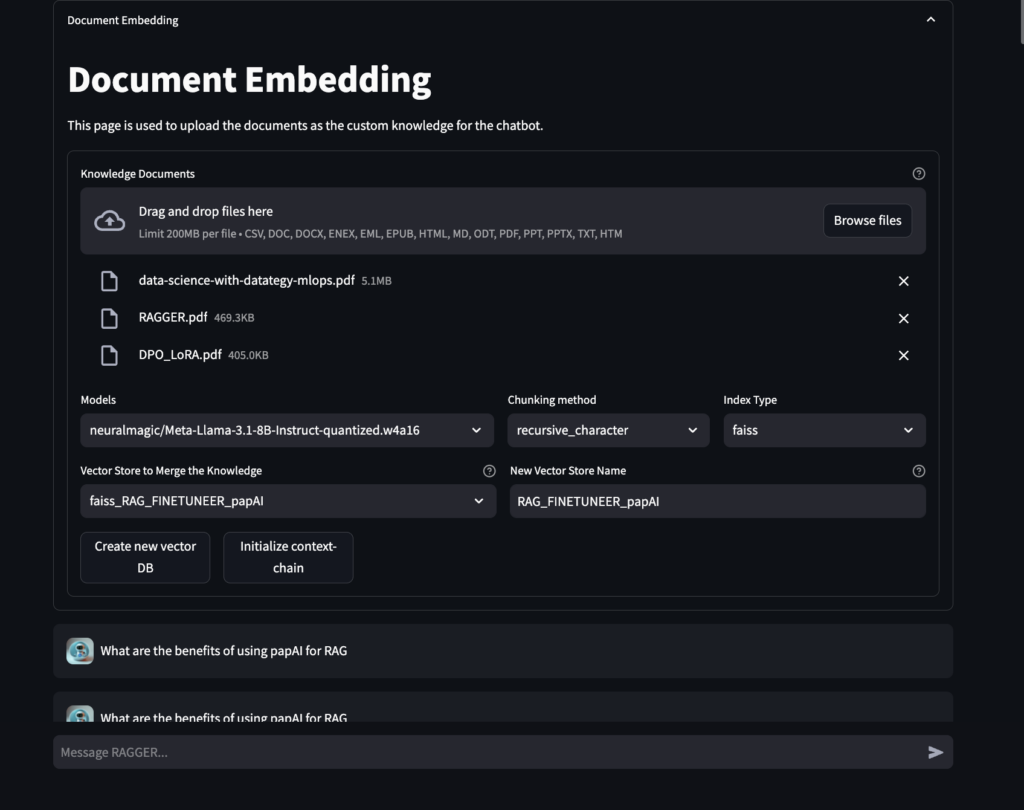
Response Generation
the combines papAI-RAG leverages papAI’s data validation and quality control features alongside advanced weighted reciprocal rank fusion and reranking methods for context selection and response generation. Benefits include reduced hallucination rates, improved response relevance, better explainability through visualizations, and a 66.6% reduction in energy consumption compared to traditional RAG approaches.
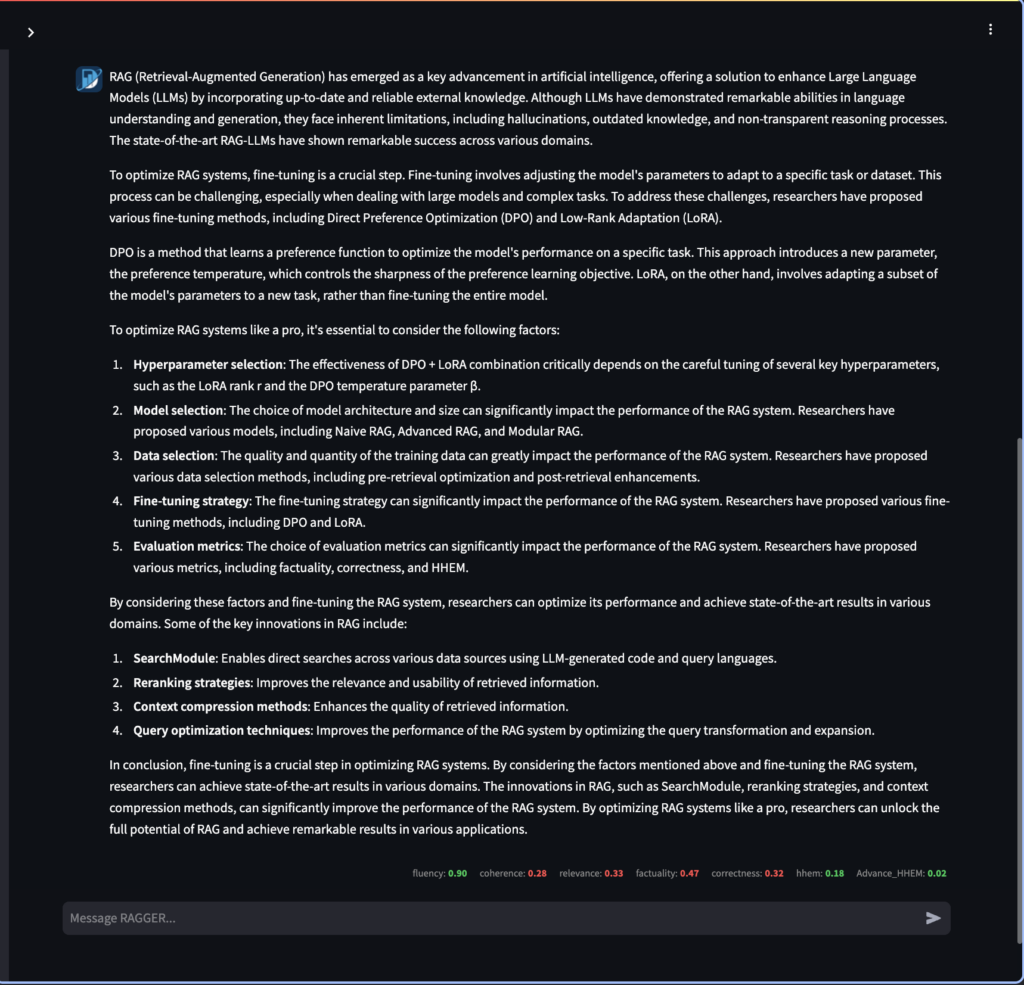
Create your Own Rag to Leverage your Data using papAI solution
To sum up, developing your own Retrieval-Augmented Generation (RAG) system can greatly improve your financial organization’s accuracy, efficiency, and decision-making skills.
You can make sure that your systems are always current and able to provide useful insights by combining real-time data retrieval with powerful generation models. Personalized client services, fraud detection, or credit risk assessment are just a few of the ways that RAG systems may help you remain ahead of the market and boost productivity.
To show how a customized RAG system may be implemented in your company, we encourage you to schedule a demo if you’re prepared to move on with the next phase of financial operations transformation. Let’s discuss how we might help you maximize the value of your data and promote more intelligent, well-informed choices.
RAG is a natural language processing (NLP) technique that combines a generator, responsible for creating contextually appropriate responses, with a retriever that selects the most relevant information from external data sources.
Fine-tuning optimizes RAG systems by enhancing retrieval accuracy, domain-specific precision, and output coherence. For instance, fine-tuning retrievers ensures that the system selects highly relevant information from external sources, while fine-tuning generators improve the clarity and relevance of the responses. This process helps adapt RAG systems to specific tasks and industries like healthcare, legal, or finance.
- Embedding Models: Transform raw text into dense vector representations for semantic understanding.
- Retrievers: Extract relevant information using sparse (e.g., keyword matching) or dense (e.g., semantic embeddings) retrieval techniques.
- Generators: Convert retrieved data into grammatically coherent, contextually relevant text that is easy to understand.
LAFT enhances Large Language Models (LLMs) without altering their core architecture. It adds trainable layers to the model, enabling domain-specific adaptation while preserving the model’s general reasoning capabilities. This makes LAFT highly efficient for financial organizations, allowing them to quickly adapt to market or regulatory changes without overfitting, ensuring stability and minimizing computational costs.
Interested in discovering papAI?
Our AI expert team is at your disposal for any questions
How RAG Systems Improve Public Sector Management
How RAG Systems Improve Public Sector Management The most important...
Read MoreScaling RAG Systems in Financial Organizations
Scaling RAG Systems in Financial Organizations Artificial intelligence has emerged...
Read MoreHow AgenticAI is Transforming Sales and Marketing Strategies
How AgenticAI is Transforming Sales and Marketing Strategies Agentic AI...
Read More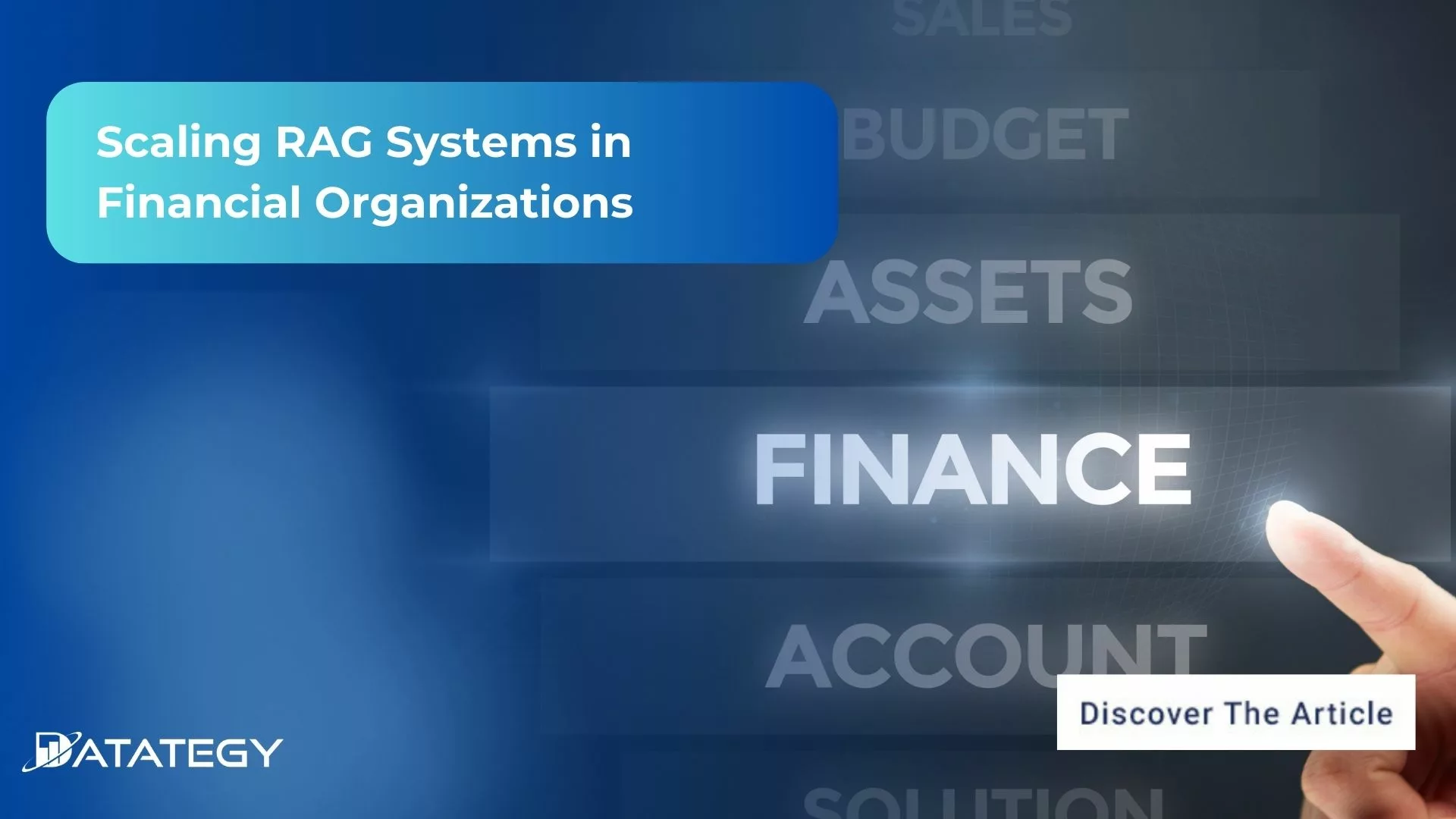
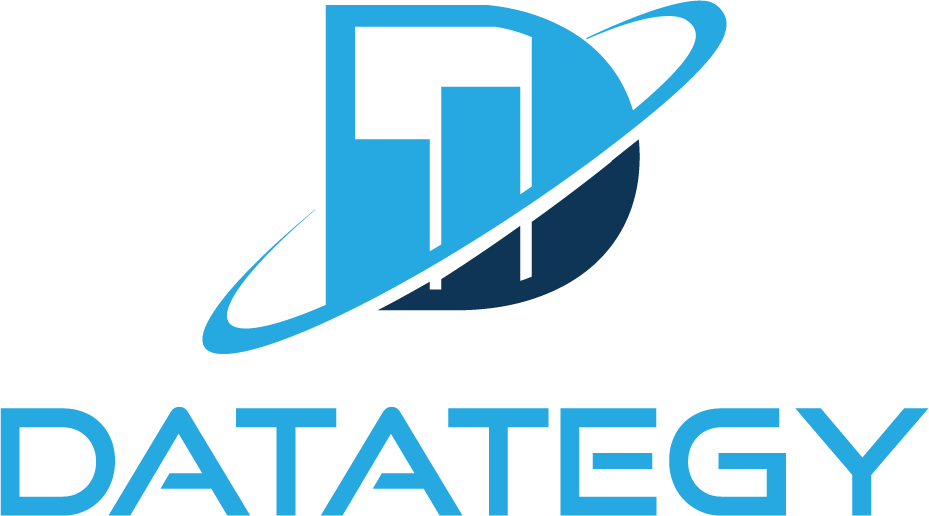