How Is AI Transforming Digital Twin in the Industry? Artificial...
Read MoreHow Is AI Transforming Digital Twin in the Industry?
Table of Contents
ToggleArtificial intelligence (AI) has become a driving force behind technological disruption across industries, and the digital twin ecosystem is no exception. As businesses seek smarter, data-driven solutions to optimize operations, AI is redefining how digital twins function, making them more intelligent, predictive, and autonomous.
AI is turning digital twins into powerful tools for innovation, whether it is by allowing self-learning models, boosting real-time simulations, or strengthening decision-making through sophisticated analytics. AI-powered digital twins are opening up new efficiencies, lowering operational risks, and laying the foundation for the future of smart industries by bridging the gap between the digital and physical worlds.
Find out how papAI can improve the deployment of AI projects in the Manufacturing Industry.
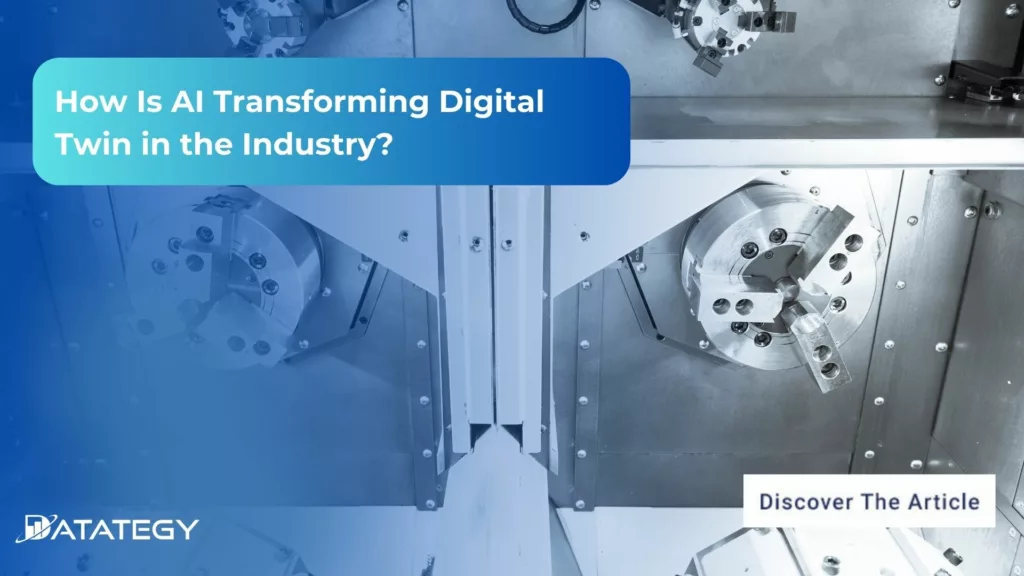
In this article, we will examine how digital twin technology is driven by artificial intelligence (AI).
How did Digital Twin technology come about?
The origins of digital twin technology can be found in the early years of space travel. NASA employed a type of digital twin technology in the 1960s to model and keep an eye on spacecraft while they were on missions.
The Apollo 13 mission is the most well-known example, in which engineers on Earth built a virtual replica of the damaged spacecraft to test fixes in real time. This method demonstrated the effectiveness of building a digital model of a physical system by enabling them to diagnose issues and safely return the astronauts home.
As processing power and data-gathering capabilities increased throughout the decades, the idea changed. Dr. Michael Grieves first proposed the concept of digital twins in the industrial sector at the beginning of the new millennium.
He suggested that digital models be used to replicate a product’s whole lifespan, from design and manufacturing to upkeep. By switching from expensive physical testing to data-driven simulations, companies were able to foresee failures, optimise performance, and cut costs.
What is Digital Twin Technology?
From Wikipedia, A digital twin is a system or process that serves as a digital counterpart of it for purposes such as simulation, integration, testing, monitoring, and maintenance.
At Datategy, we consider that with digital twin technology, a physical object is represented virtually and updated in real time. Businesses can more precisely monitor, analyse, and optimise processes because of the dynamic digital model it develops that mimics its real-world counterpart. Digital twins, which combine sensors, Internet of Things devices, and data analytics, offer profound insights into performance, forecast possible faults, and enhance decision-making in a variety of businesses.
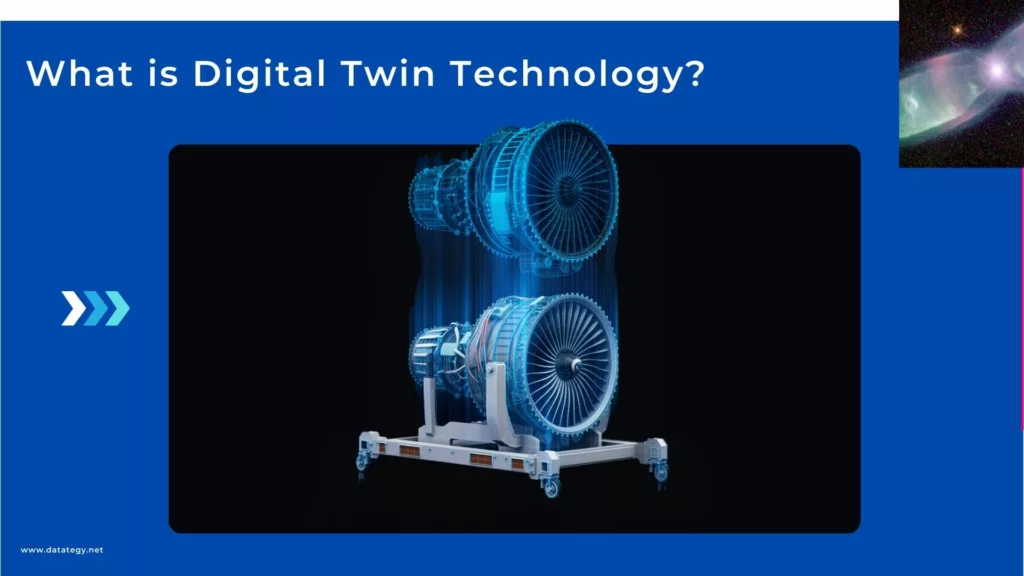
Digital Twin Example
What are the Key Components of Digital Twin Technology?
3D Digital Model (Virtual Representation)
CAD software or more sophisticated technologies like 3D scanning or photogrammetry are usually used to build this model. It replicates the asset digitally, emulating both its functional and physical attributes. The digital twin model is more than just static representations; it changes over time by modifying its structure and behaviour based on real-time inputs.
Users may see the asset’s performance, lifespan advancement, and present status thanks to this. The 3D model can incorporate simulated behaviours, such as mechanical stress or thermal dynamics, along with visual components, like geometry and material qualities, to guarantee a very realistic virtual representation of the real-world system.
Data Connectivity
Sensors, the digital twin, and physical assets are all connected by data connection. By enabling connection between different parts, middleware plays a crucial role in guaranteeing seamless data transfer from the physical asset to the digital model. This involves controlling the transmission, processing, and storage of data. Data is frequently sent between the sensors, cloud or edge computing infrastructure, and the digital twin via communication protocols like MQTT or HTTP REST APIs.
Because of this connectedness, the digital twin can stay in sync with the real asset and always get the most recent information. The seamless operation of the complete digital twin ecosystem is made possible by middleware, which makes sure that data is prepared appropriately and sent to the right systems.
Analytics and Machine Learning Models
Analytics systems and machine learning algorithms are used to process the sensor data. These models assist in data interpretation and produce insights that can influence business choices. When it comes to anticipating asset failure, optimising performance, and recommending preventative maintenance, machine learning (ML) models are very helpful since they utilise existing data to anticipate future events.
By identifying patterns in the data, these algorithms are also utilized to find abnormalities or inefficiencies inside the system. Predictive analytics, for instance, may let operators know when an asset is likely to fail so they can take precautions. By combining AI and ML, the digital twin is made into an active instrument for increasing operational effectiveness and lowering risks rather than only a passive model.
Simulation and Computational Models
A fundamental part of digital twin technology, simulations enable testing and predictive modelling of different situations. Physical behaviors inside the digital twin are frequently modeled using simulation techniques, including multi-physics simulations, Finite Element Analysis (FEA), and Computational Fluid Dynamics (CFD).
These tools model the effects of variables such as vibration, temperature, airflow, and stress on the physical asset’s performance. Users may then evaluate possible modifications or enhancements before putting them into practice in the real world by using the digital twin to test various variables in a secure virtual setting. This predictive power minimises expensive errors, speeds up innovation, and lowers the dangers related to physical testing.
User Interfaces and Visualization Tools
In order to communicate with the digital twin, user interfaces and visualisation tools are necessary. Operators, engineers, and decision-makers may visualise and track the asset’s performance in real time via dashboards, augmented reality (AR), and virtual reality (VR) interfaces. By converting complicated data into graphical representations like charts, maps, or 3D models, these technologies offer an easy-to-understand method.
In certain situations, users can engage with the digital twin in immersive settings by overlaying information directly over the real counterpart with AR or “walking through” an item with VR. This improves the user’s capacity to identify problems, evaluate performance indicators, and make defensible choices based on current data.
What's role can AI play in the digital twin field?
Analytics systems and machine learning algorithms are used to process the sensor data. These models aid in data interpretation and produce insights that can influence business choices. When it comes to anticipating asset failure, optimising performance, and recommending preventative maintenance, machine learning (ML) models are very helpful since they utilise existing data to anticipate future events.
The most important way that AI can help digital twins is to simulate a wide range of scenarios involving many factors, including changes in customer demand, manufacturing rates, supply chain interruptions, and raw material prices. Manufacturers are able to make better judgments by using these models to forecast how various tactics would succeed in certain scenarios.
For instance, under changing climatic circumstances or market needs, a business might evaluate the effects of different production methods on throughput, quality, and costs. With the use of these insights, the company could modify its plans without having to risk making mistakes in the real world.
AI can also enhance:
Production Line Optimization
By evaluating real-time data from digital twins, artificial intelligence (AI) plays a crucial part in manufacturing production line optimisation. Numerous factors can affect the effectiveness of manufacturing processes, which can be quite complicated. Operators can swiftly resolve problems by using AI to automatically identify production bottlenecks, imbalances, or inefficiencies.
The flow of resources, components, and materials may be optimised using machine learning algorithms, guaranteeing that waste is kept to a minimum and production deadlines are fulfilled. AI can forecast and modify the manufacturing process to prevent delays, minimise downtime, and guarantee on-time product delivery by continually learning from past data and real-time sensor input. Manufacturers may accomplish high production objectives with this dynamic, data-driven strategy without sacrificing quality or operating expenses.
Quality Control
It is crucial to maintain product quality during production, and AI-powered digital twins improve the effectiveness and efficiency of quality monitoring. AI is capable of real-time manufacturing process monitoring, utilizing sensor data to identify quality standard deviations. AI is able to recognise patterns linked to flaws in raw materials, equipment failures, or manufacturing procedures with the use of machine learning algorithms.
It can monitor changes in temperature, pressure, or speed, for instance, which may point to a possible flaw. Once identified, AI can prompt operators to take preventative action or initiate quick remedial action. In addition to minimising scrap rates and waste, this degree of automation significantly lowers the likelihood that damaged items will reach the consumer. Higher customer happiness, reduced expenses, and better product quality are the outcomes.
Manufacturing Process Simulation
Before implementing any tangible changes to their operations, manufacturers may evaluate and improve their production processes using AI-driven simulations that use digital twins. Numerous manufacturing scenarios, such as modifications to material kinds, process parameters, or novel production techniques, may be modelled using these simulations.
Manufacturers may save time and money by using AI to model the results of various strategies without having to risk doing real experiments. Based on real-time input from the digital twin, AI may also optimise these simulations to identify the most effective manufacturing techniques. Applying the knowledge gained from these simulations to the actual manufacturing process may increase productivity, lower expenses, and accelerate the time it takes for new goods to reach the market.
What are the Emerging Trends and Innovations in digital twin Technology?
Blockchain for Data Security
Blockchain’s primary advantages are its decentralised, transparent, and unchangeable characteristics, which make it the perfect tool for guaranteeing the security and dependability of data produced by digital twins.
Every modification made to the digital model is documented as a transaction in a distributed ledger when digital twins are connected to blockchain. All parties involved have access to this ledger, making it possible to monitor and validate any changes made to the data. A digital twin might, for instance, depict a product as it progresses through several production phases in supply chain management. The blockchain would record each modification to the product’s state or condition, including quality assurance inspections and transportation.
AR/VR for Immersive Visualization
Virtual reality allows users to see and interact with a digital twin as if they were really there, completely immersing themselves in a 3D recreation of the model. In fields like architecture and construction, where teams may virtually tour a structure before it is constructed to spot design faults or inefficiencies, this is extremely helpful. For instance, engineers may traverse the whole manufacturing line and test various configurations in the automobile sector by using virtual reality (VR) to mimic the construction of a car. By clearly visualising mechanical systems, this degree of engagement not only improves training but also facilitates maintenance duties.
In contrast, augmented reality allows users to view both the virtual and physical worlds at the same time by superimposing digital data on top of the actual environment. When doing repairs or maintenance, professionals can utilise AR glasses to see a digital duplicate of a machine or system in a manufacturing setting. To improve the technician’s capacity to do repairs quickly, the AR display might highlight possible problems that require attention or offer real-time data, such performance metrics. Human mistake is reduced and downtime is decreased as a result.
Cloud Computing and Edge Computing
By combining digital twin technology with cloud and edge computing, data processing and management have been revolutionised, allowing for the full potential of digital models to be used for real-time decision-making. While these computing paradigms have different functions, they work together to improve the responsiveness, scalability, and performance of digital twins in a variety of sectors.
The infrastructure required to store, process, and analyse the massive volumes of data produced by digital twins is made available via cloud computing. The cloud is perfect for companies that need cooperation between several teams or stakeholders since it makes it simple to exchange and access digital twin models across multiple locations. Digital twin data may be remotely accessible and centrally stored via cloud-based technologies, guaranteeing that everyone is working with the most recent data.
What are the Benefits of Adopting AI in Digital Twin
Real-Time Monitoring & Insights
Digital twins driven by AI transform monitoring by continually evaluating data from sensors, Internet of Things devices, and other data sources. This real-time analysis gives immediate input on efficiency, performance, and any problems.
For instance, sensors built inside machinery gather information on pressure, vibration, and temperature in manufacturing. Real-time artificial intelligence analyses this data, spotting irregularities that might point to a malfunction before it becomes a major issue. Companies may avoid expensive downtime by taking a proactive strategy, which enables them to fix problems before they arise.
Optimized Performance & Resource Utilization
The capacity to test many scenarios prior to applying changes in the actual world is a significant benefit. An AI-enhanced digital twin, for example, may model several supply chain paths in logistics to determine the most economical and efficient course. By modifying operating parameters, artificial intelligence (AI) may optimise machine performance in industrial settings, lowering energy consumption while preserving maximum production.
Sustainability is another important area. AI-driven digital twins optimise energy use, material use, and overall efficiency to reduce resource waste. To cut down on wasteful energy use, a smart building system, for instance, may examine occupancy trends and modify lighting, heating, and cooling.
Enhanced Decision-Making
AI-powered digital twins turn unstructured data into insightful, useful information that businesses can use to make better, quicker choices.
Conventional decision-making procedures frequently depend on past patterns and static information, both of which can soon become out of date. AI, on the other hand, examines real-time data streams, finding trends, forecasting results, and making suggestions instantly. This is especially helpful in sectors where prompt, data-driven choices may have a big influence on results, including manufacturing, healthcare, and finance.
Demystifying AI: A Comprehensive Guide to Key Concepts and Terminology
This guide will cover the essential terminology that every beginner needs to know. Whether you are a student, a business owner, or simply someone who is interested in AI, this guide will provide you with a solid foundation in AI terminology to help you better understand this exciting field.
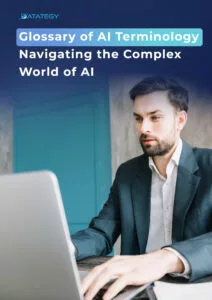
How papAI Helps Build Effective Digital Twin Approach?
papAI is a complete artificial intelligence system designed to streamline and optimize operations across several industries. By integrating seamlessly with digital twin platforms, papAI acts as the intelligence layer that processes vast amounts of real-time and historical data, providing actionable insights, predictive maintenance, and scenario simulations.
This AI-driven approach ensures that digital twin models are not just passive representations of physical systems, but dynamic, responsive tools that drive efficiency, optimize operations, and enable smarter decision-making across industries.
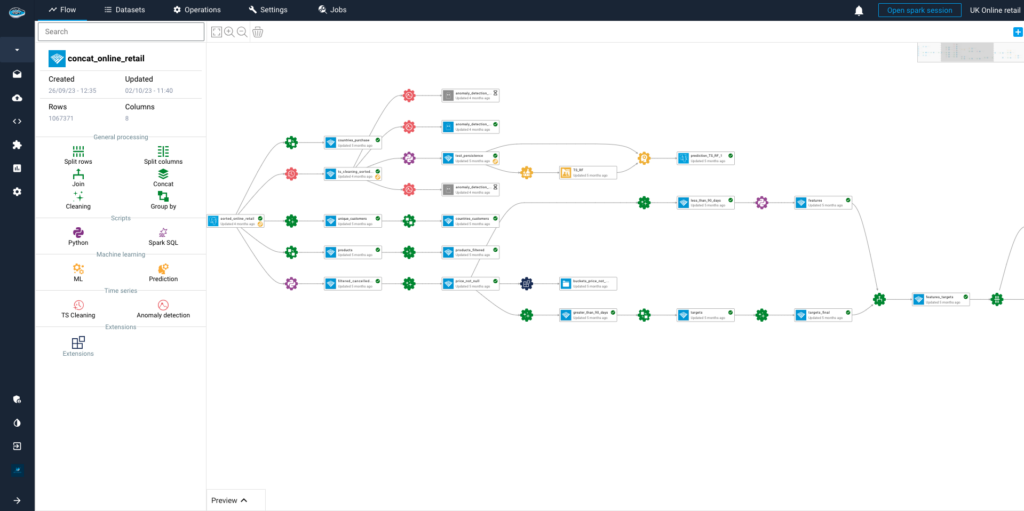
Here’s an in-depth look at the key features and advantages of this innovative solution:
Scalable Data Analysis
Large volumes of data from digital twins can be handled using papAI, which can process the data at scale to find patterns and trends that human analysts could not manually find.
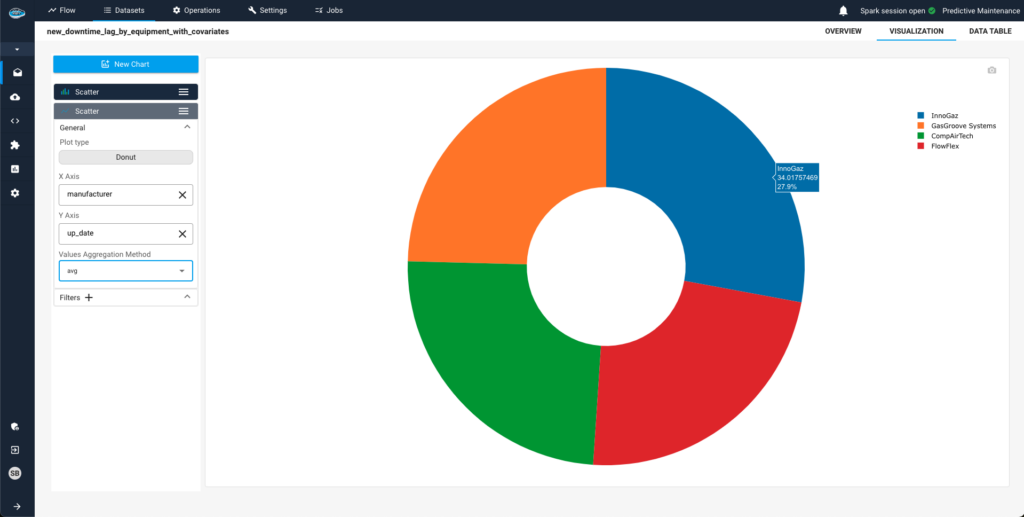
Advanced Predictive Analytics
In order to improve decision-making and save downtime, papAI can evaluate both past and current data from digital twin systems to forecast future trends, failures, and maintenance requirements.
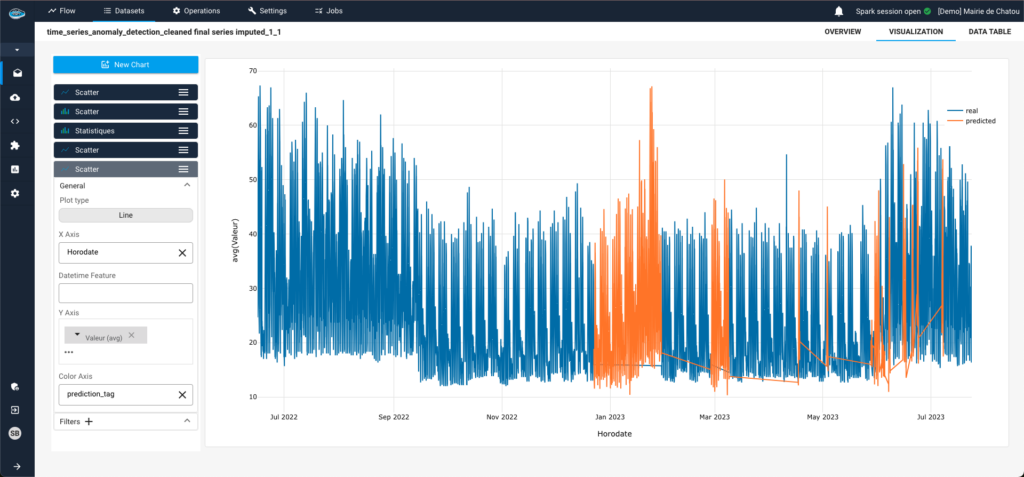
Scenario Simulation
By simulating various operational situations using digital twin data, papAI enables companies to test various approaches and comprehend their effects before to implementing them in the real world.
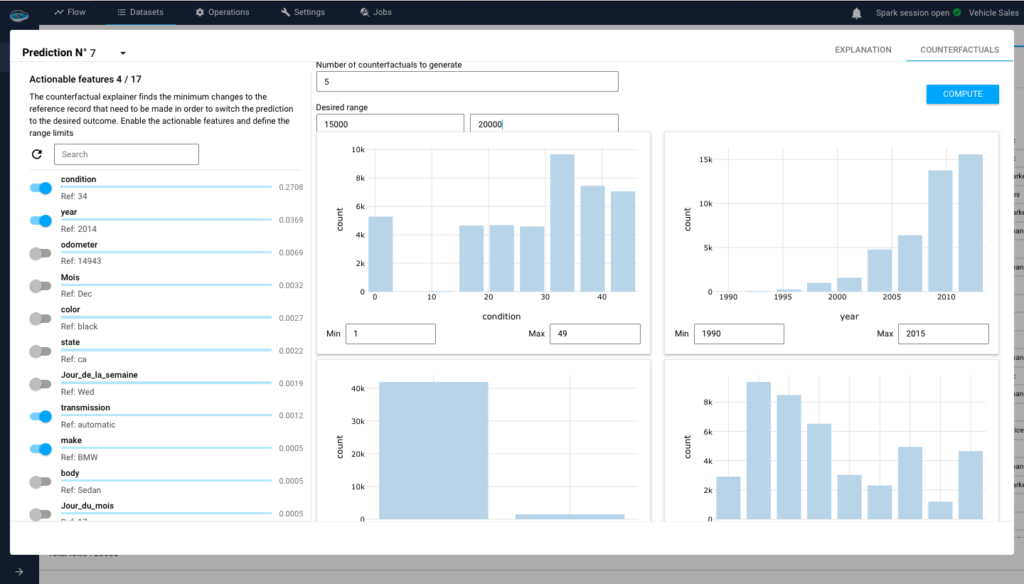
Anomaly Detection
In order to assist stop failures, mistakes, and inefficiencies before they become serious issues, papAI uses machine learning to identify performance anomalies in systems represented by digital twins.
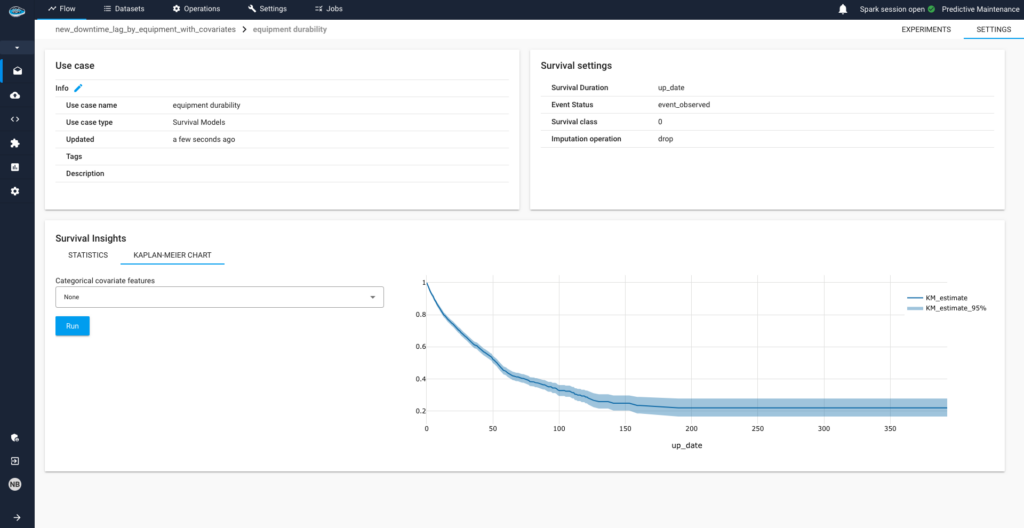
Enhance Your Digital Twin with Predictive and Analytical AI from papAI
Advanced predictive and analytical AI layers must be included in order to fully use the potential of digital twins. By offering profound insights via machine learning, real-time analytics, and predictive modelling, papAI improves digital twin technology.
Book a demo today and discover how our solution can help you drive efficiency, reduce costs, and achieve operational excellence in your industry.
With digital twin technology, a physical object, system, or process is represented virtually and updated in real time using technologies like 3D.
AI and IoT work together by allowing IoT-connected devices to gather real-time data. AI processes this data, identifying trends, detecting anomalies, and providing insights like predictive maintenance, which helps prevent equipment failures and optimizes production.
Industries such as manufacturing, healthcare, smart cities, energy, and transportation can leverage AI-powered digital twins to enhance productivity, improve maintenance strategies, and optimize resource management.
Challenges include ensuring high-quality data for AI models, managing real-time data processing, addressing cybersecurity risks, and integrating AI solutions with existing digital twin infrastructures. Overcoming these challenges is key to maximizing the benefits of AI-driven digital twins.
Interested in discovering papAI?
Watch our platform in action now
“DATATEGY EARLY CAREERS PROGRAM” With Julien Courteville
“DATATEGY EARLY CAREERS PROGRAM” With Julien Courteville Hello, my name...
Read MoreDatategy + Nedd Technologies: First Center of AI Excellence in USA
Datategy + Nedd Technologies: First Center of AI Excellence in...
Read MoreHow AI Drives the Evolution of Industry 4.0
How AI Drives the Evolution of Industry 4.0 Today, Industry...
Read More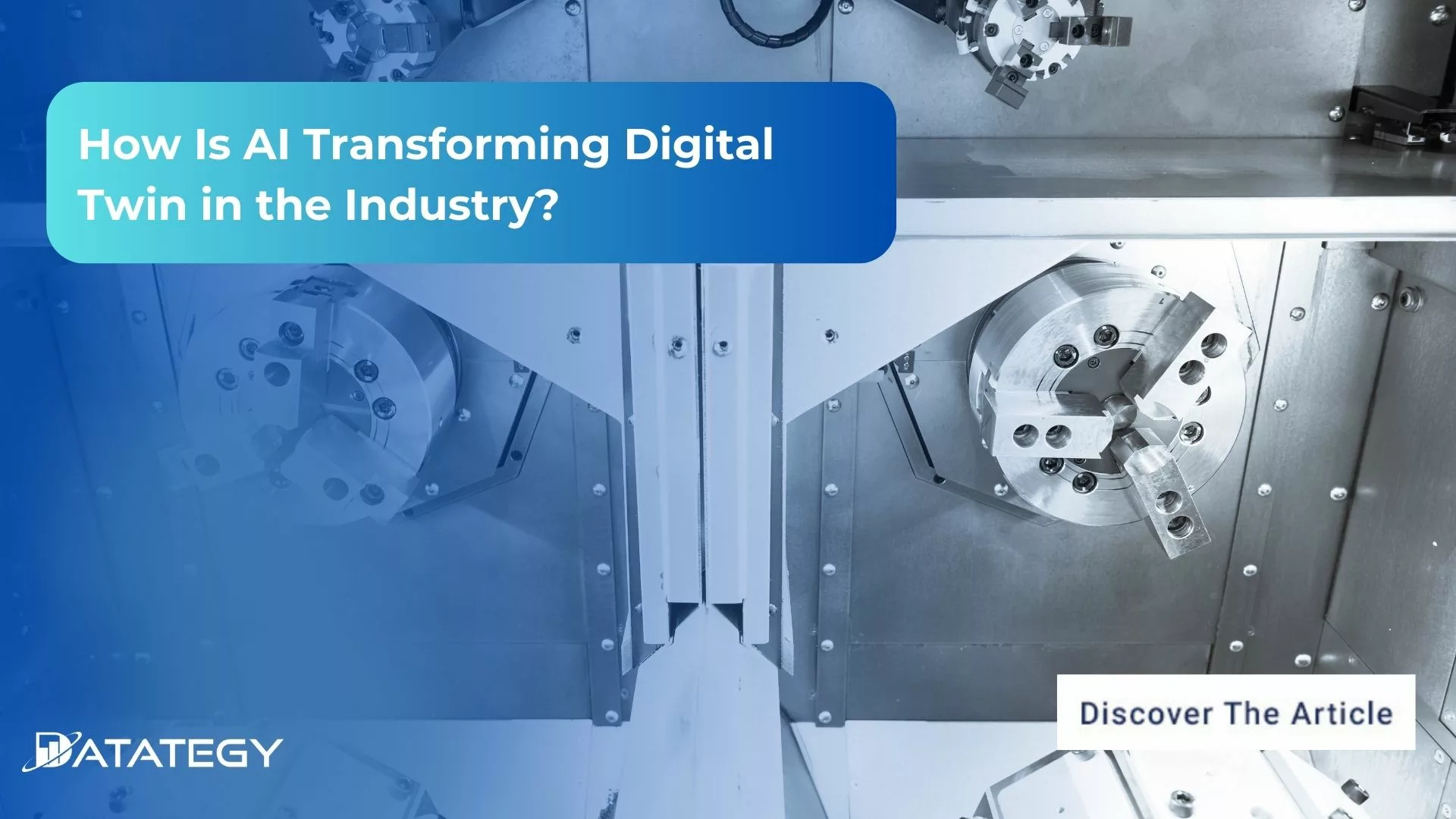
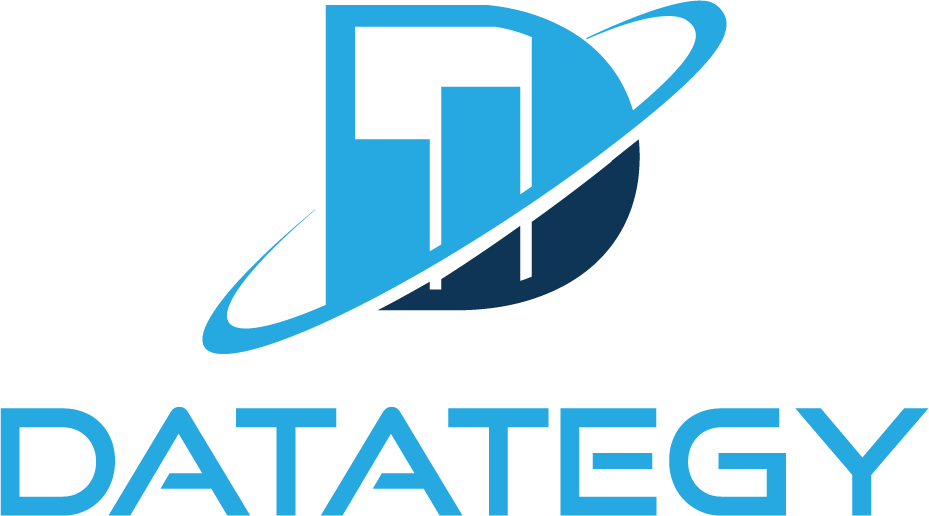