How Law Firms Use RAG to Boost Legal Research RAG...
Read MoreAI Governance Pathway: Challenges and Solutions
Table of Contents
ToggleRecent advances in artificial intelligence techniques have raised new questions about governance. Due to the large amount of sensitive data, the question of access to this data arises. However, in industries like healthcare, banking, and others where privacy and compliance are unavoidable, increasing dependence on data poses serious questions about how information is accessed, utilized, and protected.
Several nations had implemented important AI governance programs. For instance, the European Union’s AI Act is scheduled to go into force in 2025, the United States issued an Executive Order on AI in October 2023, and China debuted its Algorithm Registry in December 2022.
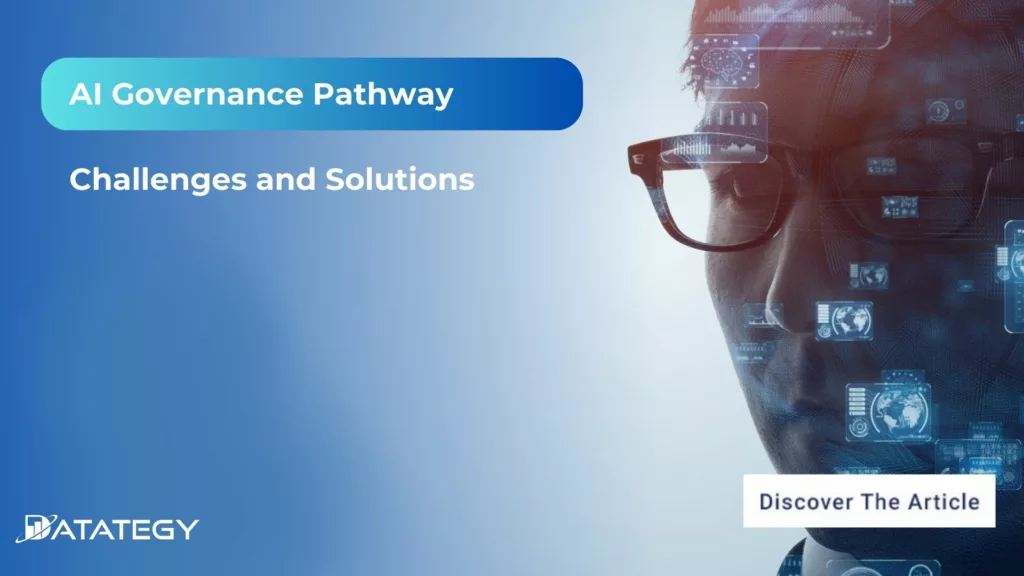
In this article, we’ll explore some of the most pressing data governance challenges facing AI systems today
What does AI Governance Mean?
The structures, regulations, and procedures known as AI governance are intended to guarantee that AI systems are created, implemented, and utilized in an ethical manner. It involves formulating rules that give ethical decision-making in AI, accountability, transparency, and fairness a priority. Given how much AI depends on data, governance also entails controlling the collection, archiving, and training of this data. AI may inadvertently perpetuate prejudices, break privacy regulations, or make opaque or unaccountable judgments in the absence of adequate control.
AI governance is establishing the “ground rules” for AI to guarantee that it minimizes dangers and maximizes benefits for everybody. Technical steps like creating explainable AI models and organizational procedures like assigning who is in charge of keeping an eye on AI systems are both part of it. Governments and businesses already understand the possibilities and perils of AI, and AI governance is crucial to ensuring that AI solutions are not only efficient but also morally and socially responsible.
Why AI Needs Robust Governance frameworks?
Ensures Privacy: AI systems frequently handle private information, including financial data, medical records, and personal preferences. Serious privacy violations might result from the abuse or exposure of this data in the absence of a robust governance mechanism. Sensitive data is safeguarded by a strong AI governance framework that complies with privacy regulations such as GDPR and implements rigorous data security measures.
Additionally, it guarantees that users’ consent is acquired for data gathering and that data is anonymized or encrypted as required. People are more confident in AI technology as a result of this protection of individual rights and prevention of any exploitation of personal data.
Conserve Transparency: Establishing confidence in AI systems requires transparency. People are less inclined to trust AI when they don’t understand how it makes judgements, particularly in crucial fields like healthcare, banking, or law enforcement. Strong AI governance frameworks guarantee that AI decision-making is transparent and comprehensible.
This might entail explaining to users why particular choices were taken or improving the interpretability of AI algorithms. Businesses that employ transparent AI procedures assist consumers in comprehending the logic behind AI results, which may result in better decision-making and increased technology adoption.
Guarantee Fairness: Although AI systems are educated on enormous volumes of data, they may make unjust choices if the data contains biases. For instance, an AI system that evaluates job applicants and is educated on skewed historical data may unjustly give preference to some groups over others. Strong governance encourages equity in the way AI systems function and guarantees that the data used to train AI is impartial and representative.
This entails ensuring that AI choices do not unjustly penalize particular people or groups. Organizations can prevent these biases and ensure AI works for everyone by creating norms for ethical data usage, testing for fairness, and continuously reviewing the results of AI models.
What are the Key AI Regulatory Developments Around the World?
Artificial Intelligence Act of the European Union:
One of the first extensive legislative frameworks intended to control the research and application of AI inside the European Union is the EU Artificial Intelligence Act, which was proposed on April 21, 2021. According to the degree of danger caused by the AI applications, the Act assigns different levels of regulatory requirements to different AI systems.
Stricter rules will be applied to high-risk AI systems, such those in criminal justice or healthcare, to guarantee security, equity, and openness. In order to guarantee that AI is created in accordance with European principles and human rights, the AI Act also tackles concerns of data privacy, bias, and accountability.
H.R. 3831, the AI Disclosure Act of 2023, US:
The purpose of the June 5, 2023, proposed AI Disclosure Act of 2023 is to make sure that businesses reveal how they employ AI in their decision-making. The goal of this rule is to help people and customers understand how and when AI affects decisions that impact them.
According to the statute, businesses must disclose all relevant information regarding the AI systems they employ, including the data and algorithms that underlie their choices. In addition to supporting the ethical use of AI technology, the law aims to safeguard people from any damage caused by AI-driven judgments by fostering openness.
China’s Temporary Administrative Steps to Manage Generative AI Services:
Enacted on July 13, 2023, China’s Interim Administrative Measures for the Management of Generative AI Services govern the usage of generative AI technology, including deep learning models and content creation. With a focus on content management, data protection, and halting the spread of harmful or unlawful information, this policy aims to guarantee that generative AI systems function safely and morally.
According to the regulations, AI developers must make sure their models don’t generate inaccurate, biased, or dangerous data. China’s attempts to create a robust regulatory framework for new AI technologies while preserving control over digital material and safeguarding its citizens are reflected in the rule.
Region | Country | Regulation | Status |
---|---|---|---|
Americas | US | Algorithmic Accountability Act 2023 (H.R. 5628) | Proposed (Sept. 21, 2023) |
Americas | US | AI Disclosure Act of 2023 (H.R. 3831) | Proposed (June 5, 2023) |
Americas | US | Digital Services Oversight and Safety Act of 2022 (H.R. 6796) | Proposed (Feb. 18, 2022) |
Americas | Canada | Artificial Intelligence Data Act (AIDA) | Proposed (June 16, 2022) |
Europe | EU | EU Artificial Intelligence Act | Proposed (April 21, 2021) |
Asia | China | Interim Administrative Measures for the Management of Generative AI Services | Enacted (July 13, 2023) |
What are the Best Practices for Effective AI Governance?
Clearly Defined Roles and Responsibilities: Clearly defining roles within the organization and defining who is in charge of what are essential for successful data governance. This involves determining the important people or groups in charge of data security, privacy, compliance, and quality. Everyone knows their obligations and who to contact in the event of a problem when roles are clearly defined.
Additionally, it helps to prevent misunderstandings and guarantees that no one is overlooked when it comes to data management. For example, legal teams may supervise adherence to data protection laws, while data stewards might be in charge of guaranteeing data quality. Roles that are clearly defined foster responsibility, which is necessary to avoid errors or omissions that could result in problems like data breaches or legal infractions.
Integrating Ethical Standards into AI Development: In order to guarantee that AI systems are utilized sensibly and do no damage, it is imperative that ethical standards be included in AI development. Establishing ethical standards to control AI’s research, design, and use is crucial as it continues to advance and affect many facets of society.
This involves developing AI systems that are impartial, open, and devoid of prejudices. To prevent biases that can result in prejudice, developers should, for instance, make sure that the data used to train AI models is representative and varied. Making AI systems visible so that users can comprehend the decision-making process is another aspect of ethical AI development. When anything goes wrong, accountability is made possible by this transparency, which also fosters confidence.
Designing a Centralised Data Governance Framework: To manage data uniformly throughout an organization, a centralized data governance framework is necessary. This framework guarantees that all data adheres to the same set of rules and norms, regardless of its source or intended purpose. Monitoring data security, compliance, and quality is made simpler with a centralized approach.
Organizations may establish a clear, uniform policy for the collection, processing, and sharing of data by unifying all data management procedures under a single framework. Because all teams adhere to the same standards and procedures, decision-making may also be done more effectively. Because everyone follows the same governance structure, a centralized framework also makes it possible for departments to collaborate more effectively.
How to implement AI Solutions for Process Optimization within your Organizations
Select the Best AI Tools
First of all , you have to select the finest AI tools and technologies to suit your particular requirements. Depending on your objectives, you may need to leverage technologies like computer vision, machine learning, or predictive analytics. For instance, computer vision is particularly useful for visual inspections and defect identification, while machine learning models may be used to forecast problems or optimize operations.
Predictive analytics tools are helpful in anticipating maintenance needs. You must choose technologies that work with your existing systems and infrastructure to guarantee smooth integration and scalability for future needs.
Data Collection and Configuration of the Infrastructure
Gathering relevant data is crucial for training your AI models, as data is the foundation of any AI application. This includes sensor data, production data from the past, and any other inputs pertaining to manufacturing. Since AI models rely on high-quality data to function properly, accurate, clear, and organised data are crucial.
Set up the necessary infrastructure. Ensure it connects with your current ERP or MES systems. This will help with data collection and storage. You may need to install IoT sensors on manufacturing lines for real-time monitoring. These sensors gather data on vibration, temperature, and pressure. This information aids in analysis and boosts efficiency.
Choose the Right AI Model
Once the data has been collected and analyzed, the next step is to train AI models tailored to your quality control processes. This means putting the data into machine learning techniques or deep learning models for computer vision applications.
For example, machine learning models can learn to find defects using past data. However, computer vision systems need many labeled photos to spot product issues. Customization is important to make sure the AI models fit your production environment and product details. Testing, validation, and iteration are key to making sure the models work well in real-life situations.
Pilot Testing and Validation
Before using AI systems in your entire production line, run a pilot test. This test will help you check the system’s performance in a controlled setting. Focus the pilot on a small part of your manufacturing process. This will help you validate the AI models and see how they affect quality control.
During this phase, monitor the AI system’s ability to spot errors, optimize workflows, and predict maintenance needs. Consult with operators and quality control teams to identify any issues or possible areas for improvement. Make the necessary adjustments to the AI models or system integration before scaling the solution.
How to choose the best AI solution for your data project?
In this white paper, we provide an overview of AI solutions on the market. We give you concrete guidelines to choose the solution that reinforces the collaboration between your teams.
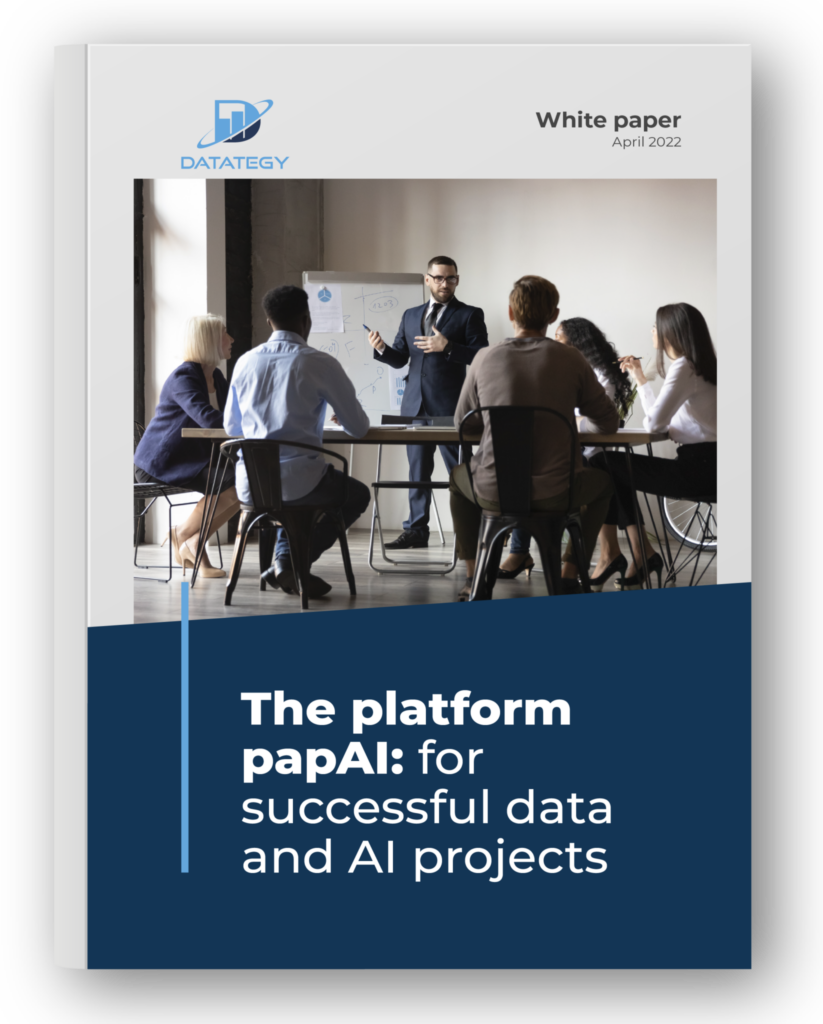
How to Build a Governed foundation using papAI?
papAI is an all-in-one AI platform designed to simplify and accelerate the development, deployment, and management of artificial intelligence solutions. Built with versatility and scalability in mind, papAI provides businesses with the tools they need to leverage AI across various use cases, from automation and data analysis to decision-making and predictive insights.
Its no-code and low-code interface and powerful capabilities make it accessible to both AI experts and business users, ensuring that organizations of all sizes can harness the power of AI to drive innovation and efficiency.
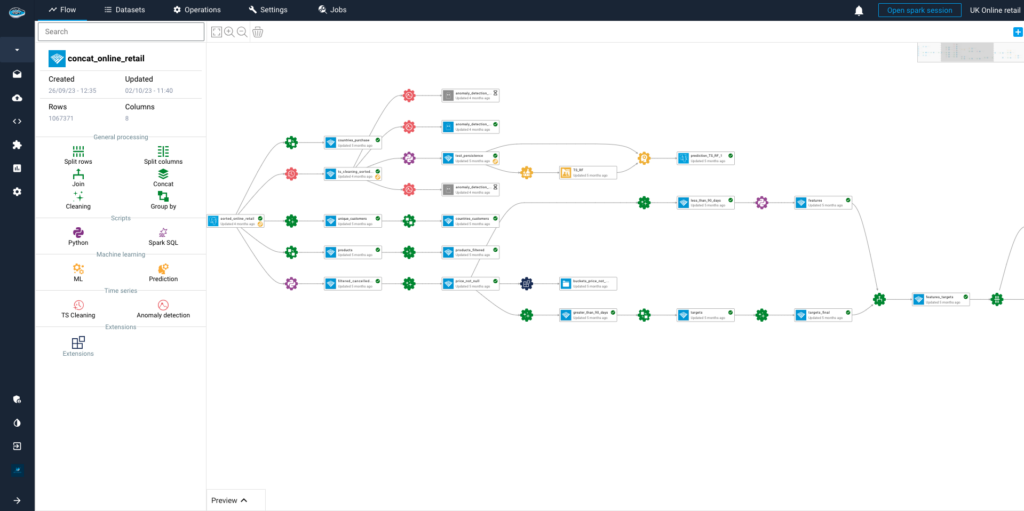
Define Clear Data Governance Policies
The first step in creating a regulated foundation with papAI is to create explicit data governance principles that complement the goals of your company and legal requirements. This entails establishing guidelines for the gathering, use, sharing, and storage of data. PapAI can easily integrate into your governance structure by specifying who is in charge of data management, how it should be kept up to date, and the privacy and security mechanisms in place. This will guarantee that all data inputs and outputs are reliable and compliant.
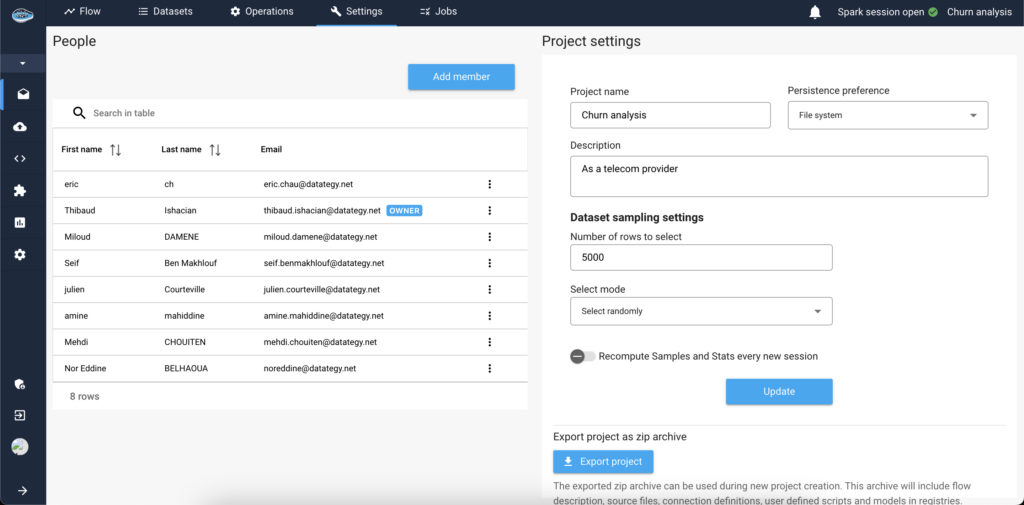
Automate Data Lineage Tracking
To guarantee accountability and transparency in your AI models, automate data lineage tracking using papAI. This entails recording how data moves from its source via a number of changes before being used in decision-making. You can spot any flaws, such poor data quality or compliance concerns, and fix them before they affect AI results by visualising this process. The automated features of papAI support the upkeep of a dependable and effective data governance architecture.
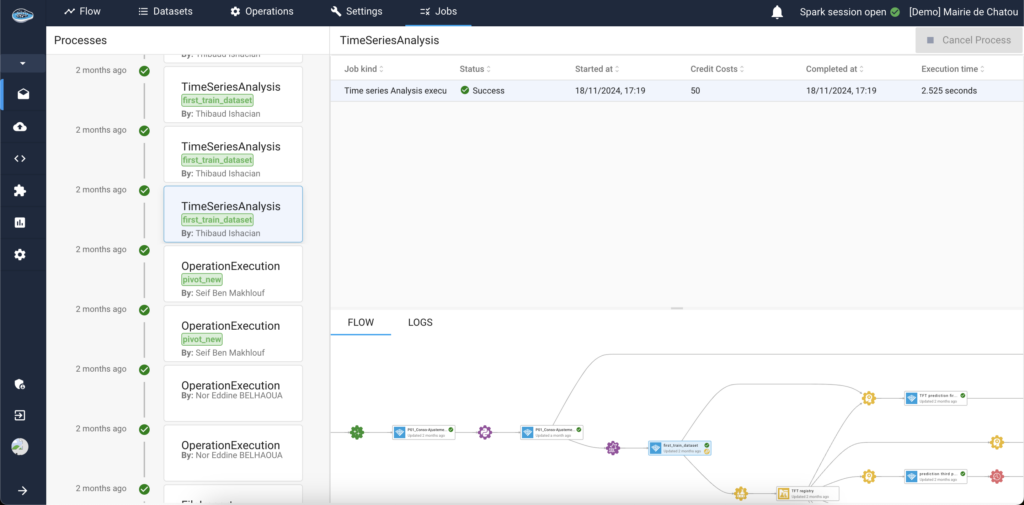
Integrate Data Quality Management
Use the integrated data quality management tools in papAI to keep an eye on and guarantee that the data is correct, consistent, and comprehensive. To find and fix mistakes like duplication, missing numbers, or inconsistencies, you may build up real-time monitoring using papAI. In addition to lowering the possibility of bias and subpar decision-making, papAI helps guarantee that the AI models developed on this data generate dependable, trustworthy outcomes by upholding strict data quality standards.
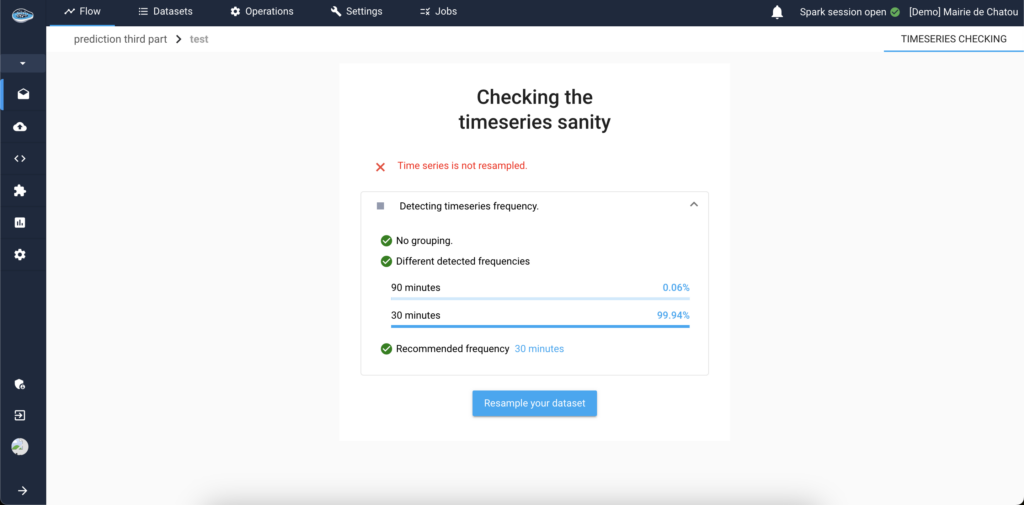
Create your Own AI Governed Platform using papAI solution
You can easily and confidently construct, monitor, and manage your AI systems thanks to papAI, which streamlines the complicated process of AI creation. You can make sure that your AI models are moral, open, and in line with industry standards by including governance at each stage of the process.
Don’t allow the difficulties of AI governance to stop you from empowering your company with a reliable platform that will serve your needs both today and in the future.
Book a demo today and discover how our solution can help you create your own AI-governed platform, tailored to your specific needs and goals.
AI Governance refers to the policies, regulations, and procedures that ensure artificial intelligence systems are developed, deployed, and operated ethically and responsibly. This involves setting rules that prioritize transparency, fairness, accountability, and ethical decision-making. AI governance is vital for managing data, ensuring privacy, and protecting against potential biases and security risks in AI models.
AI Governance is essential to maintain trust in AI systems. It ensures the privacy of sensitive data, such as medical or financial records, and safeguards individuals’ rights by adhering to strict data privacy laws like GDPR. A robust AI governance framework also promotes transparency, allowing users to understand how AI makes decisions, and ensures fairness by addressing biases that could lead to unjust outcomes.
- Algorithm Innovations: Advances in machine learning and deep learning have enhanced AI’s ability to process data with near-human intelligence.
- Computing Power: Faster and more powerful processors, GPUs, and cloud platforms enable AI to handle complex algorithms and large datasets.
- Increased Investment: Growing interest and funding from governments and businesses drive innovation, making AI more accessible and impactful.
Best practices for AI governance include defining clear roles and responsibilities to ensure accountability, integrating ethical standards in AI development to prevent biases, and creating a centralized data governance framework to standardize data management across the organization. These practices foster transparency, fairness, and security, helping organizations deploy AI systems responsibly and effectively.
To implement AI solutions for process optimization, organizations must first select the right AI tools based on their needs, such as predictive analytics or machine learning. Then, collect high-quality data, configure infrastructure to support data flow, and train AI models tailored to the specific processes. Finally, pilot test the AI system on a small scale, validate its performance, and iterate before full deployment. This ensures the system meets operational needs and improves efficiency.
Interested in discovering papAI?
Our AI expert team is at your disposal for any questions
How RAG Systems Improve Public Sector Management
How RAG Systems Improve Public Sector Management The most important...
Read MoreScaling RAG Systems in Financial Organizations
Scaling RAG Systems in Financial Organizations Artificial intelligence has emerged...
Read MoreHow AgenticAI is Transforming Sales and Marketing Strategies
How AgenticAI is Transforming Sales and Marketing Strategies Agentic AI...
Read More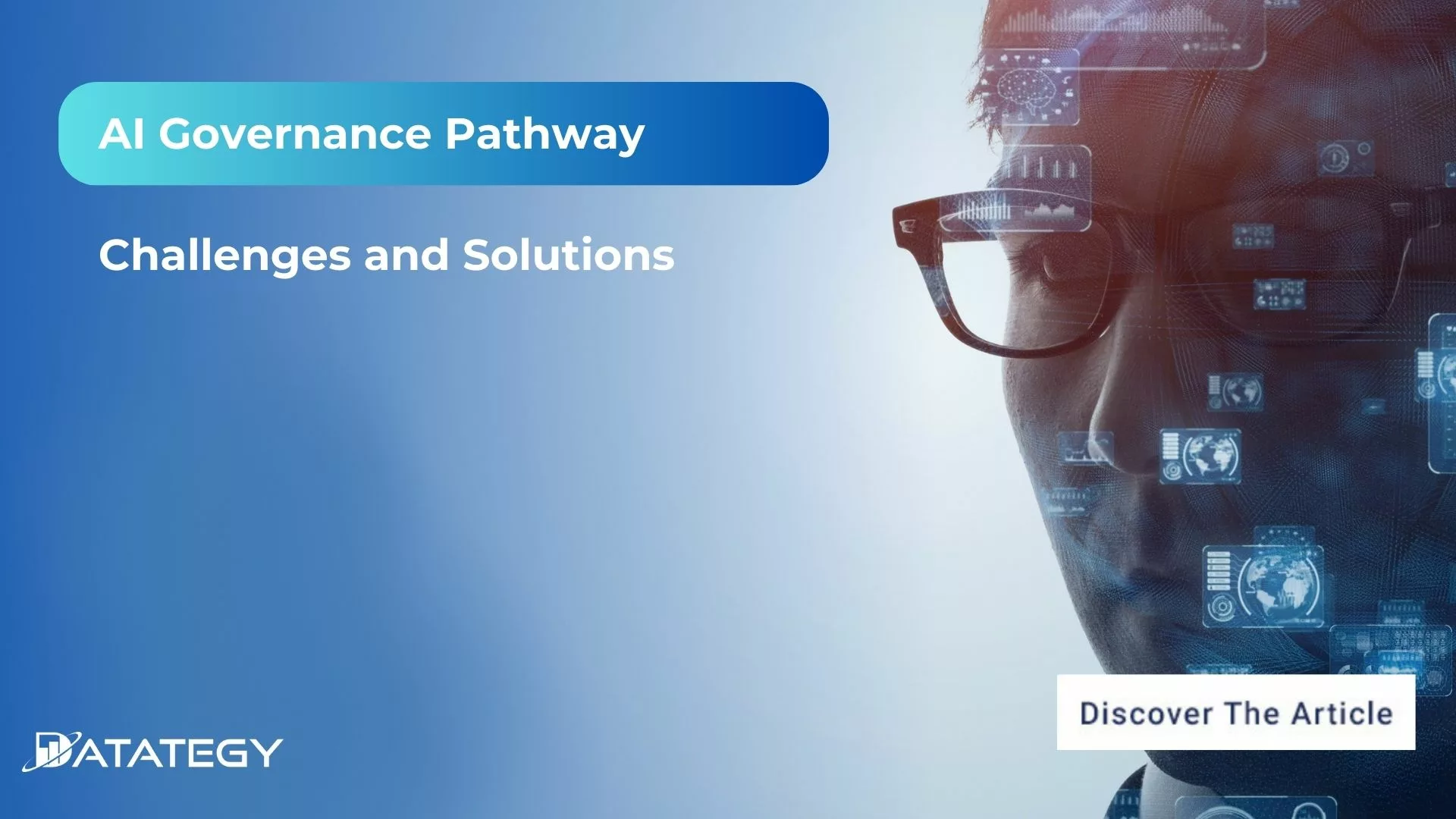
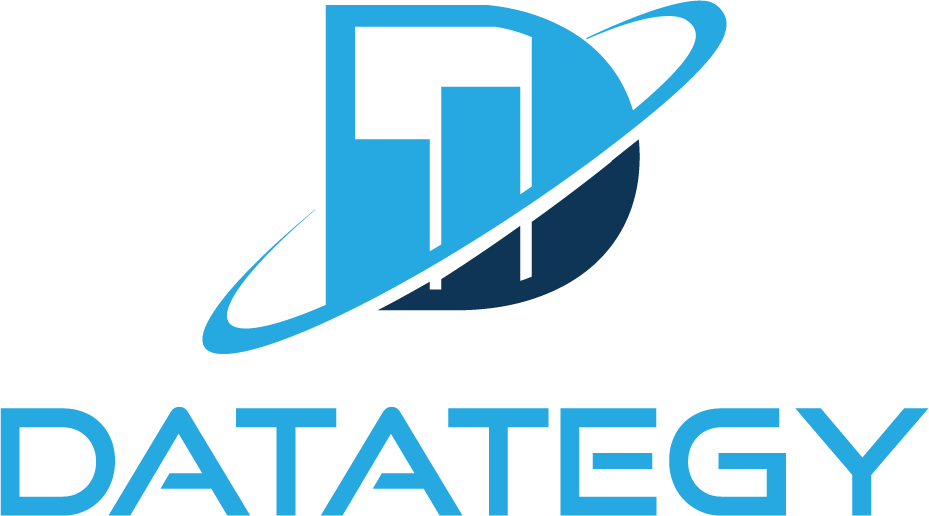