Scaling RAG Systems in Financial Organizations Artificial intelligence has emerged...
Read MoreHow AI drives Operational Excellence in Manufacturing Industry
Table of Contents
ToggleOperational excellence is a key concern for all stakeholders in the manufacturing industry. Operational excellence aims to make an organization’s operations more efficient while minimizing waste and costs, and continuously improving.
Artificial intelligence (AI) has quickly become an important technology. It helps improve many parts of business and daily life. As companies look to make their operations better, AI has become essential. It streamlines workflows, analyzes complex data, and offers valuable insights. These insights help in making better decisions.
According to Accenture. Automating repetitive tasks with AI can increase manufacturing productivity by 15-20%.
Find out how papAI can improve the deployment of AI projects in the Manufacturing Industry.
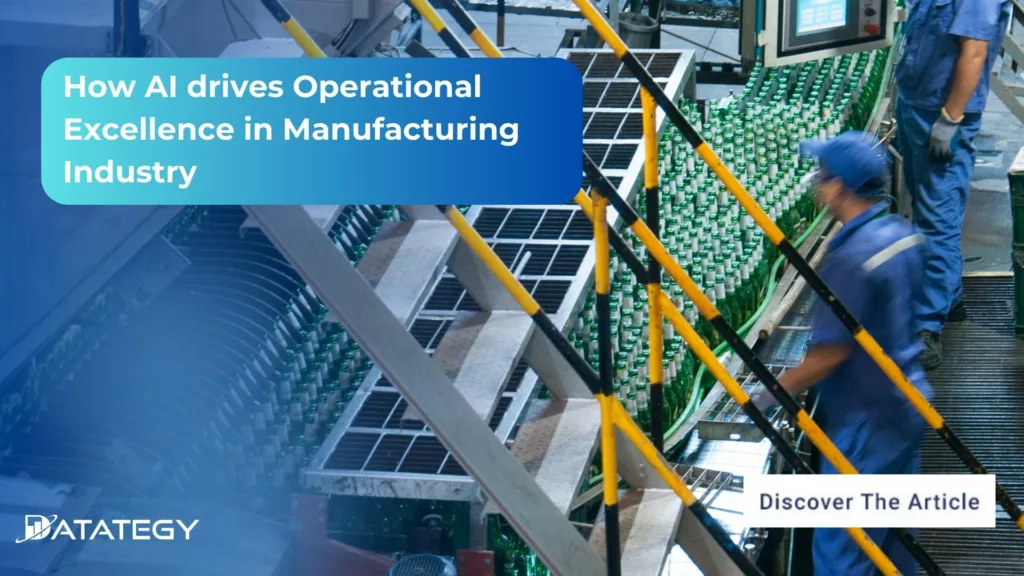
In this article, we will explore how artificial intelligence (AI) drives Operational Excellence.
What does Operational Excellence in Manufacturing Mean?
In manufacturing, operational excellence refers to managing a company as effectively and efficiently as feasible. It involves figuring out how to use resources more effectively, streamline procedures, and provide clients with high-quality goods. This strategy aims to increase value, solve issues more quickly, and remain adaptable when circumstances change, not only reduce expenses. When a business prioritizes operational excellence, all employees collaborate to achieve the same objective: completing tasks correctly and continuously improving.
Why Artificial Intelligence has Emerged Now?
Data Proliferation: The evolution of artificial intelligence has been fueled by our unprecedented data production. Every second, a vast amount of data is generated from various sources, ranging from online transactions to industrial sensors. This data enables AI systems to learn, adapt, and improve by identifying patterns and insights that were once challenging to uncover.
Algorithmic Advancements: Recent breakthroughs in machine learning and deep learning algorithms have significantly amplified AI’s capabilities. These algorithms’ capacity to ingest and understand data in a manner akin to human intelligence has propelled applications such as image recognition, natural language processing, and predictive analytics to new levels of performance.
Advancements in Computing Power: The birth of AI is intrinsically tied to the development of faster and more powerful computer technologies. The infrastructure provided by high-performance CPUs, GPUs, and cloud computing platforms makes it feasible and accessible to handle complex AI algorithms and vast datasets, thus enabling AI applications.
Escalation in Investment and Innovation: The expansion of AI is fueled by the escalating interest of governments, corporations, and investors. Companies are pouring substantial resources into R&D, triggering a chain reaction where improved technology leads to more practical applications, which subsequently propels a surge in AI adoption across various sectors.
What are the Key Challenges in Traditional Manufacturing Processes?
Control of Quality and Management of Defects:
The significant issue with conventional production is maintaining constant product quality. Manual inspections are frequently used for quality control, although they can be laborious and prone to human mistakes. It gets harder to keep an eye on things when manufacturing numbers rise, which might cause flaws to slip through the gaps. In addition to causing monetary losses, defective items may damage a business’s reputation.
Manufacturers must put in place more automated and data-driven quality control procedures in order to overcome this obstacle. Real-time production monitoring, anomaly detection, and remedial action before flaws affect the finished product are all possible with these technologies.
Waste and Inefficiency:
Inefficiency, which is frequently brought on by antiquated equipment, manual workflows, and disjointed systems, is one of the biggest problems with traditional manufacturing processes. Longer manufacturing times, higher expenses, and excessive material and energy waste are the results of these inefficiencies.
Traditional setups cause overproduction or underutilization since machines follow established timetables rather than current demand. Furthermore, in manual operations, human mistakes can exacerbate inefficiencies, resulting in delays, rework, and variable product quality. A comprehensive evaluation of processes and the use of more flexible, data-driven techniques are necessary to address inefficiencies and maximize resource utilization while streamlining production.
Restricted Scalability and Flexibility:
Conventional production methods frequently find it difficult to adjust to shifting consumer tastes and market needs. Smaller manufacturing batches or customization cannot be accommodated by rigid production processes built for large output. A manufacturer’s capacity to innovate or swiftly change course in response to fresh possibilities or difficulties is hampered by this rigidity.
It can be costly and take a lot of time to increase production to meet changing demand, especially with old systems. Flexible and scalable processes are crucial for businesses to remain competitive. They help companies produce a wide range of products without losing quality or profits.
What are the Key Benefits of Artificial Intelligence (AI ) in Operational Excellence?
Improved Quality Assurance:
In manufacturing, upholding high standards of quality is essential, and artificial intelligence has revolutionized this field. Conventional quality control techniques frequently depend on human inspection, which is subject to mistakes and irregularities. AI, on the other hand, inspects items in real-time with unmatched precision using sophisticated computer vision and machine learning algorithms.
These systems quickly find flaws, irregularities, or issues with products. This ensures that only high-quality items reach customers. AI also looks at past data to find trends that could cause problems. This helps producers fix issues before they happen. As a result, waste is reduced, and product quality improves. This builds customer loyalty and trust.
Enhanced Productivity and Efficiency:
In the industrial industry, artificial intelligence is transforming productivity and efficiency. Artificial intelligence frees up human workers to concentrate on more strategic and creative duties by automating time-consuming and repetitive jobs. For instance, AI-powered robotic process automation (RPA) may swiftly and accurately manage material handling or assembly lines, greatly cutting cycle times.
By anticipating possible equipment problems before they happen, AI-powered predictive maintenance solutions also reduce unscheduled downtime and maintain uninterrupted operations. Additionally, by examining variables like machine availability, material supply, and demand estimates, AI algorithms optimize production plans. Manufacturing processes are made faster, more dependable, and more economical with this degree of automation and optimization.
Cutting Expenses:
Through waste reduction, resource optimization, and increased operational efficiency, artificial intelligence (AI) helps manufacturers save money. AI-powered energy management systems, for example, track and regulate energy use throughout manufacturing plants, reducing wasteful use and utility costs.
By anticipating demand patterns, AI also improves inventory management by ensuring that producers maintain the proper level of stock without going over or under. AI reduces expensive downtime, rework, and defects by automating procedures and lowering mistakes; this eventually boosts profitability while upholding high production standards.
How to implement AI Solutions for Process Optimization within your Organizations
Select the Best AI Tools
First of all , you have to select the finest AI tools and technologies to suit your particular requirements. Depending on your objectives, you may need to leverage technologies like computer vision, machine learning, or predictive analytics. For instance, computer vision is particularly useful for visual inspections and defect identification, while machine learning models may be used to forecast problems or optimize operations.
Predictive analytics tools are helpful in anticipating maintenance needs. You must choose technologies that work with your existing systems and infrastructure to guarantee smooth integration and scalability for future needs.
Data Collection and Configuration of the Infrastructure
Gathering relevant data is crucial for training your AI models, as data is the foundation of any AI application. This includes sensor data, production data from the past, and any other inputs pertaining to manufacturing. Since AI models rely on high-quality data to function properly, accurate, clear, and organised data are crucial.
Set up the necessary infrastructure. Ensure it connects with your current ERP or MES systems. This will help with data collection and storage. You may need to install IoT sensors on manufacturing lines for real-time monitoring. These sensors gather data on vibration, temperature, and pressure. This information aids in analysis and boosts efficiency.
Choose the Right AI Model
Once the data has been collected and analyzed, the next step is to train AI models tailored to your quality control processes. This means putting the data into machine learning techniques or deep learning models for computer vision applications.
For example, machine learning models can learn to find defects using past data. However, computer vision systems need many labeled photos to spot product issues. Customization is important to make sure the AI models fit your production environment and product details. Testing, validation, and iteration are key to making sure the models work well in real-life situations.
Pilot Testing and Validation
Before using AI systems in your entire production line, run a pilot test. This test will help you check the system’s performance in a controlled setting. Focus the pilot on a small part of your manufacturing process. This will help you validate the AI models and see how they affect quality control.
During this phase, monitor the AI system’s ability to spot errors, optimize workflows, and predict maintenance needs. Consult with operators and quality control teams to identify any issues or possible areas for improvement. Make the necessary adjustments to the AI models or system integration before scaling the solution.
What are the Main Technologies Behind the use of AI in Operational Excellence?
Predictive Analytics: Time Series Forecasting
AI quality control predictive analytics uses anomaly detection models and time-series forecasting to anticipate possible production process failures. To examine past production data and forecast future trends, time-series forecasting methods like LSTM (Long Short-Term Memory) networks and ARIMA (AutoRegressive Integrated Moving Average) are used.
These models help find changes in typical patterns based on temperature, humidity, or equipment wear. This allows the system to predict when a quality problem might happen. AI algorithms can also spot unusual patterns in production data. They use methods like clustering (e.g., k-means) or advanced techniques like autoencoders. Predictive analytics learns from new data. It not only finds potential quality issues but also helps manufacturers improve maintenance schedules.
Machine Learning Models: Supervised, Unsupervised, and Reinforcement Learning
In AI-driven quality control, machine learning models use different methods for various tasks. Supervised learning needs labeled datasets. This method is often used to identify defective and non-defective products based on size or color. Common algorithms for this task include Random Forests, decision trees, and Support Vector Machines (SVM).
On the other hand, when labeled data is scarce, unsupervised learning is used for finding anomalies and clustering. This method groups similar product features together. It also helps find outliers that may indicate flaws. Algorithms such as K-means and DBSCAN are commonly used. K-means is a clustering method, while DBSCAN stands for Density-Based Spatial Clustering of Applications with Noise. However, unsupervised learning is not often used in quality control.
Computer Vision: Advanced Image Processing and Feature Extraction
Computer vision is key to AI-driven quality control. It uses advanced image-processing methods to check products. The system takes clear pictures of products on the production line with high-definition cameras. Then, it uses feature extraction algorithms to analyze these images. This helps find important parts like edges, textures, and shapes.
These features are studied using deep learning models, especially Convolutional Neural Networks (CNNs). These models mimic how people see and understand images. The system can spot problems like cracks, scratches, or alignment issues. It learns from large sets of labeled images. The system can also verify labels or serial numbers using optical character recognition (OCR).
Robotic Process Automation (RPA)
The technique known as robotic process automation (RPA) uses software bots to automate repetitive, rule-based processes that are normally completed by people. RPA focuses on automating digital activities including data input, order processing, and report production, in contrast to conventional industrial robots that do physical tasks. RPA bots are faster and more accurate than human users when interacting with databases, systems, and applications.
RPA is crucial for enhancing operational and administrative processes in the manufacturing sector. For instance, RPA can streamline inventory management by generating purchase orders when supplies dwindle and updating inventory levels in real-time. Moreover, it can handle supplier interactions, process billing documents, and generate compliance reports. RPA reduces human mistakes, boosts efficiency, and facilitates the automation of these monotonous tasks.
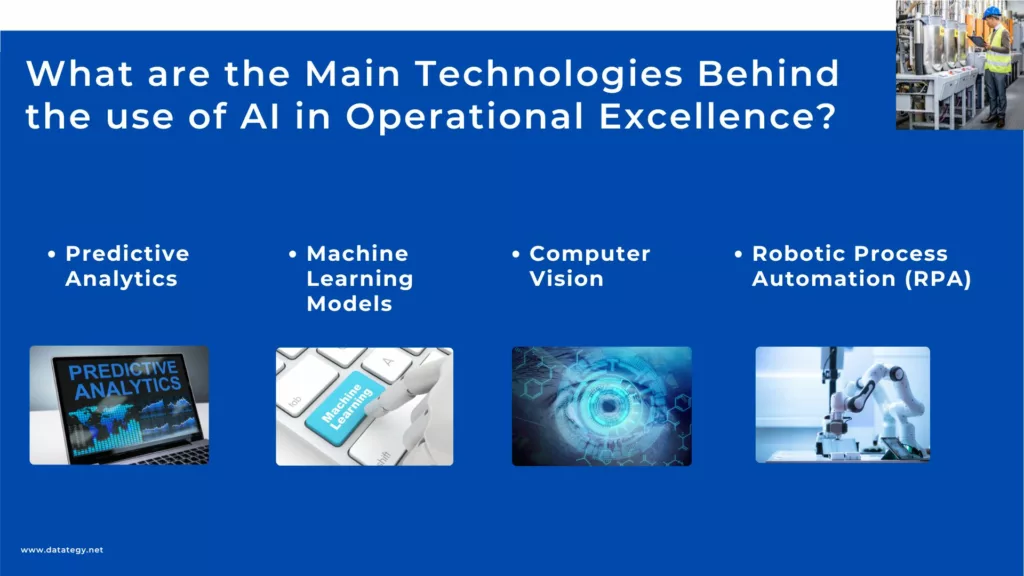
Main Technologies Behind AI in Operational Excellence
Real-World Examples of Companies Achieving Operational Excellence using AI
General Electric (GE) has leveraged AI to redefine how maintenance is handled in its manufacturing plants
To increase productivity and cut expenses, GE has used predictive maintenance in place of more conventional reactive or planned maintenance.
The system gathers real-time data on performance indicators including temperature, vibration, pressure, and energy usage using sensors that are integrated into machines. AI-powered algorithms that can identify minute trends or irregularities that can point to possible equipment breakdown are used to analyse this data. AI can handle enormous volumes of data fast and precisely, unlike human operators, and can spot problems before they cause malfunctions.
Beyond efficiency and cost savings, GE’s use of AI in conjunction with IoT technologies demonstrates the company’s dedication to innovation. It is part of a larger trend in the industrial sector towards digital transformation, where data and cutting-edge technology lead to more intelligent choices. Through resource optimization and equipment longevity, this method not only increases dependability but also promotes sustainability.Source
Optimizing Supply Chain Efficiency with AI: Siemens' Smart Approach
Siemens greatly increases production and delivery efficiency by optimizing its supply chain with artificial intelligence. Siemens is able to analyze enormous volumes of data from a variety of sources, including production schedules, historical sales data, and market trends, by utilizing AI algorithms. The organization can more accurately forecast future demand thanks to this data analysis, which enables them to modify production and inventory levels appropriately.
Inventory management is another area in which the AI system is essential. Siemens can prevent both overstocking and stockouts by improving its demand forecasts and ensuring that it has the appropriate quantity of goods on hand. This avoids production delays brought on by shortages and lowers the expenses related to surplus inventory.Source
Enhancing Quality Control with AI: Caterpillar's Approach to Manufacturing Excellence
In order to improve quality control in its production processes and guarantee that its goods satisfy high standards, Caterpillar employs artificial intelligence. Artificial intelligence (AI) technologies are incorporated into the production process to check products in real-time and identify any flaws or irregularities that could occur during manufacture. A more complete and accurate evaluation of every product is ensured by these technologies’ ability to spot even the slightest flaws that human inspectors could overlook.
Over time, the AI systems’ accuracy increases as they continually learn and adjust to new data. This enables Caterpillar to adjust to shifting production demands and maintain a constant level of quality across its product line. As a consequence, the business can keep up high standards of quality while cutting expenses related to waste and faults.Source
Before using Artificial Intelligence, what obstacles must we overcome?
Businesses must overcome a number of significant challenges prior to using artificial intelligence in order to guarantee its effective deployment. The amount and quality of accessible data is a significant barrier. Large datasets are essential for AI learning and accurate prediction, but many organizations struggle with data that is inconsistent, fragmented, or scattered across several systems. To get around this, companies need to make investments in data integration, cleansing, and appropriate data governance to guarantee that they can supply AI systems with accurate, high-quality data.
How to choose the best AI solution for your data project?
In this white paper, we provide an overview of AI solutions on the market. We give you concrete guidelines to choose the solution that reinforces the collaboration between your teams.
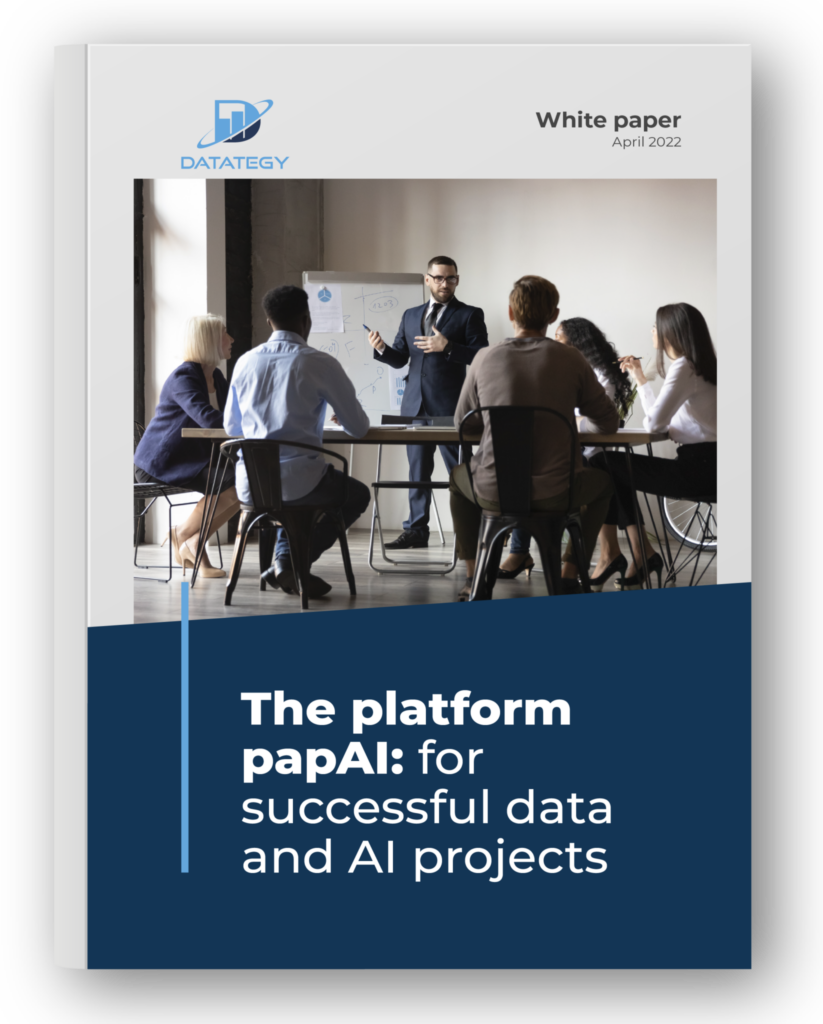
How papAI Helps Build Effective AI-Powered Process Optimization?
papAI is a comprehensive artificial intelligence solution made to optimize and simplify processes in a variety of sectors. It offers strong data analysis, predictive insights, and process automation capabilities by fusing state-of-the-art machine learning and artificial intelligence technology.
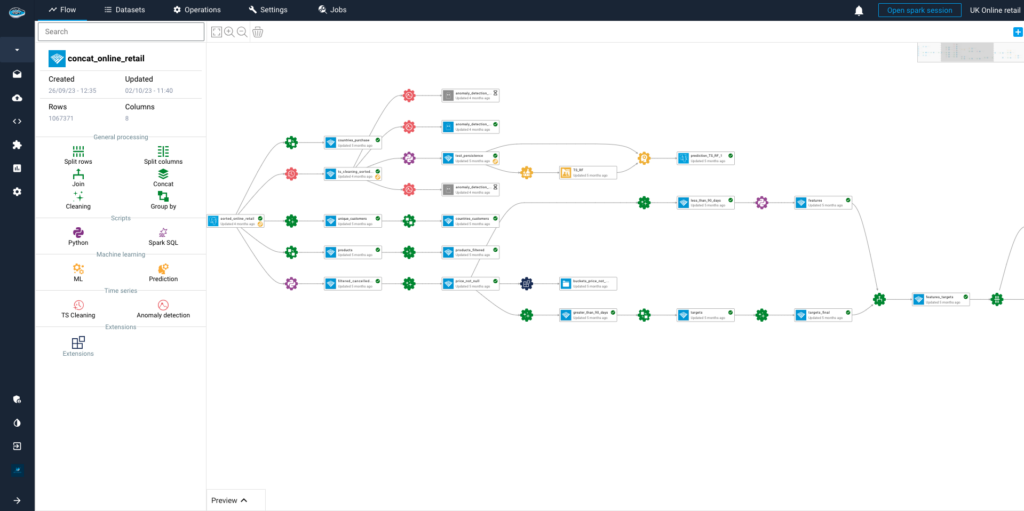
Here’s an in-depth look at the key features and advantages of this innovative solution:
Data Integration and Streamlining
First, papAI connects with many data sources. These include sensor data, manufacturing logs, and past quality control documents. It brings together large amounts of data from different systems, like ERP and MES, onto one platform. For accurate analysis and decision-making, papAI needs real-time access to all important production data. This access is provided through this interface.
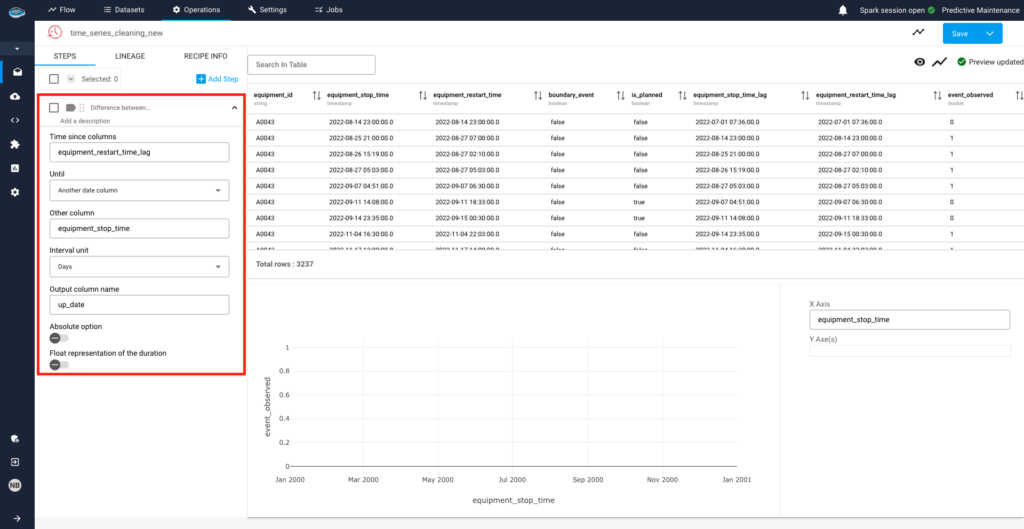
Advanced Predictive Analytics and Real-time Insights
Using machine learning algorithms to analyse production factors like temperature, pressure, and vibration, papAI predicts potential issues in the manufacturing process. Businesses may take proactive steps, such as scheduling maintenance or altering production settings, to prevent quality issues by using these predictive information.
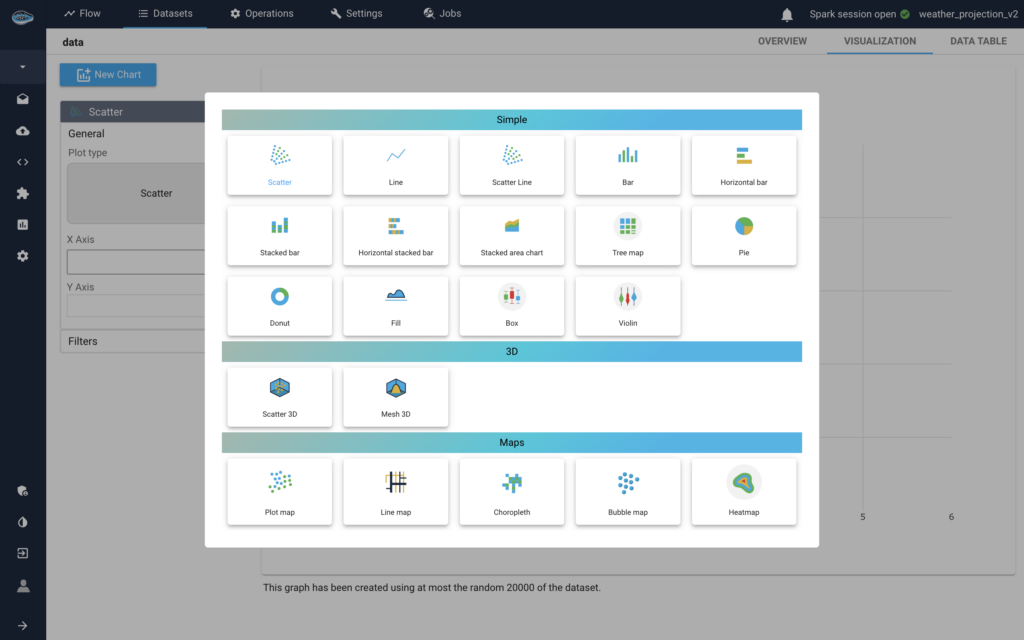
Smooth Integration in Different Production Lines
papAI is made to easily scale across a variety of industrial environments and production lines. PapAI can adjust to a variety of production needs and setups, regardless of whether your business has a single manufacturing line or many lines spread over different locations. By adapting automatically to modifications in operational procedures, product types, or production volume,
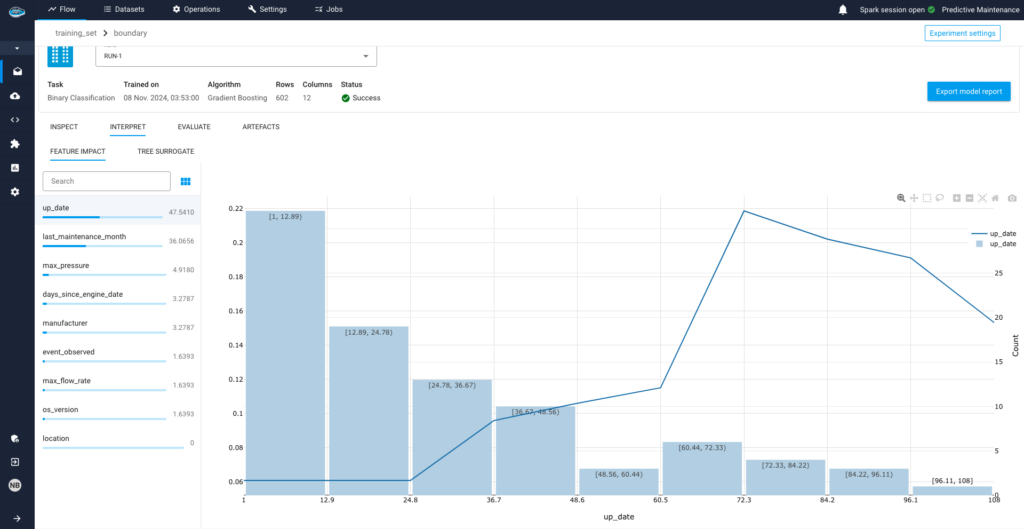
Create your Own AI Solution for Operational Excellence using papAI solution
With the correct tools and tactics, achieving operational excellence in manufacturing is no longer a pipe dream but rather a feasible reality. Through customised insights, predictive analytics, and process optimization, the papAI solution enables companies to fully utilise artificial intelligence.
From increasing efficiency and decreasing waste to boosting quality assurance, papAI offers a complete platform to handle the particular difficulties of your business.
Book a demo today and discover how our solution can help you drive efficiency, reduce costs, and achieve operational excellence in your industry.
Operational excellence in manufacturing refers to managing operations as effectively and efficiently as possible. It focuses on optimizing resources, streamlining processes, and delivering high-quality products to customers. Beyond cost reduction, it emphasizes solving problems quickly, staying adaptable, and creating value by involving all employees in continuous improvement.
- Data Abundance: With the rapid increase in data from sources like industrial sensors and online transactions, AI can identify patterns and generate insights.
- Algorithm Innovations: Advances in machine learning and deep learning have enhanced AI’s ability to process data with near-human intelligence.
- Computing Power: Faster and more powerful processors, GPUs, and cloud platforms enable AI to handle complex algorithms and large datasets.
- Increased Investment: Growing interest and funding from governments and businesses drive innovation, making AI more accessible and impactful.
- Quality Control: Manual inspections can be inconsistent and error-prone, especially as production scales.
- Inefficiency: Outdated equipment and workflows result in waste, higher costs, and longer production times.
- Lack of Flexibility: Rigid systems struggle to adapt to changing consumer demands, limiting innovation and scalability.
- Improving Quality Assurance: AI systems use computer vision and machine learning to detect defects in real-time with high precision, ensuring consistent quality.
- Enhancing Productivity: Automation and predictive maintenance reduce downtime and streamline workflows.
- Cutting Costs: AI optimizes resources, reduces waste, and prevents overproduction, improving profitability without compromising standards.
Interested in discovering papAI?
Our AI expert team is at your disposal for any questions
How AgenticAI is Transforming Sales and Marketing Strategies
How AgenticAI is Transforming Sales and Marketing Strategies Agentic AI...
Read More“DATATEGY EARLY CAREERS PROGRAM” With Abdelmoumen ATMANI
“DATATEGY EARLY CAREERS PROGRAM” With Abdelmoumen ATMANI Hello, my name...
Read MoreIntroducing the Error Analysis Tree: A Smarter Way to Improve AI Models
Introducing the Error Analysis Tree: A Smarter Way to Improve...
Read More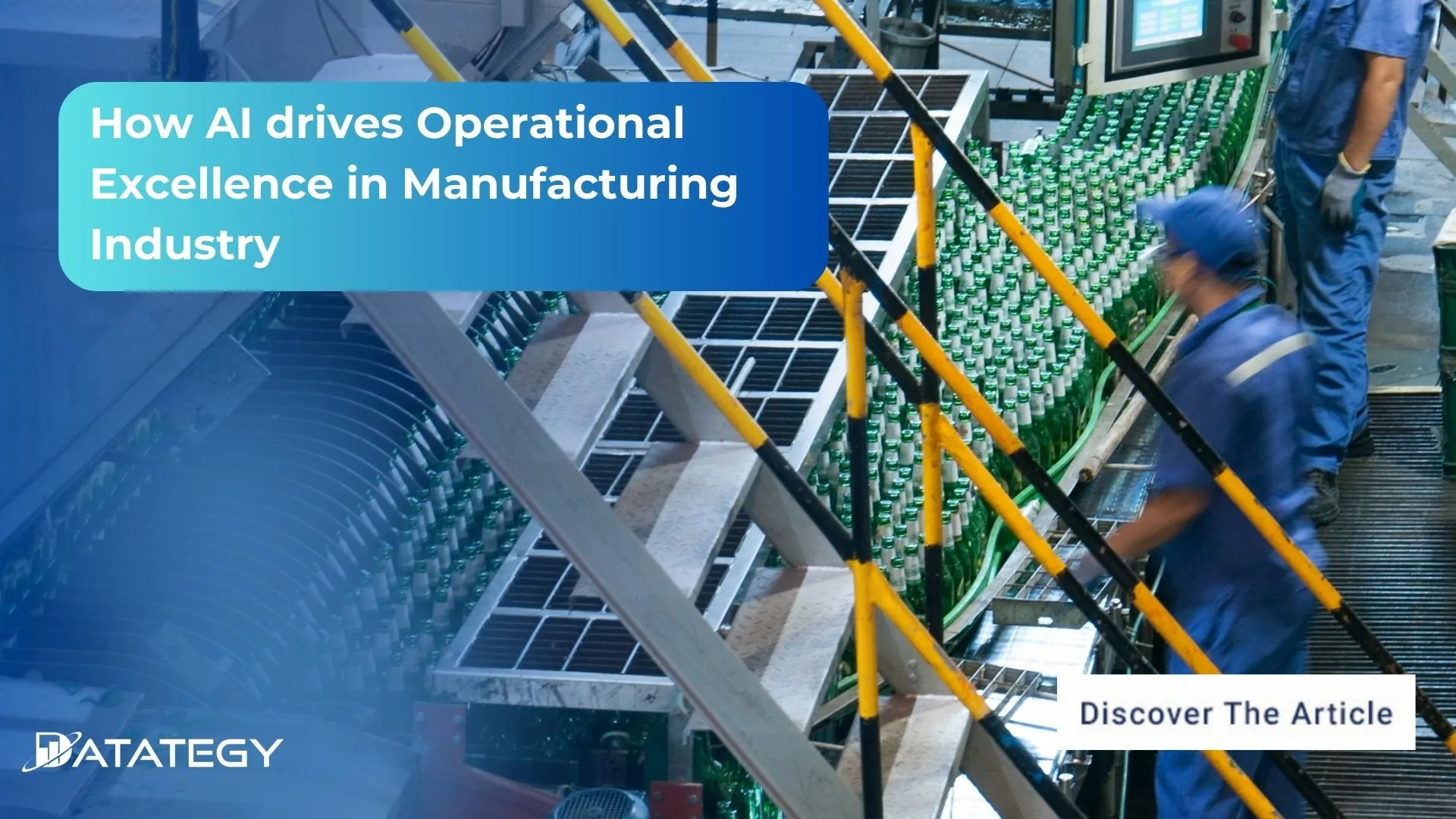
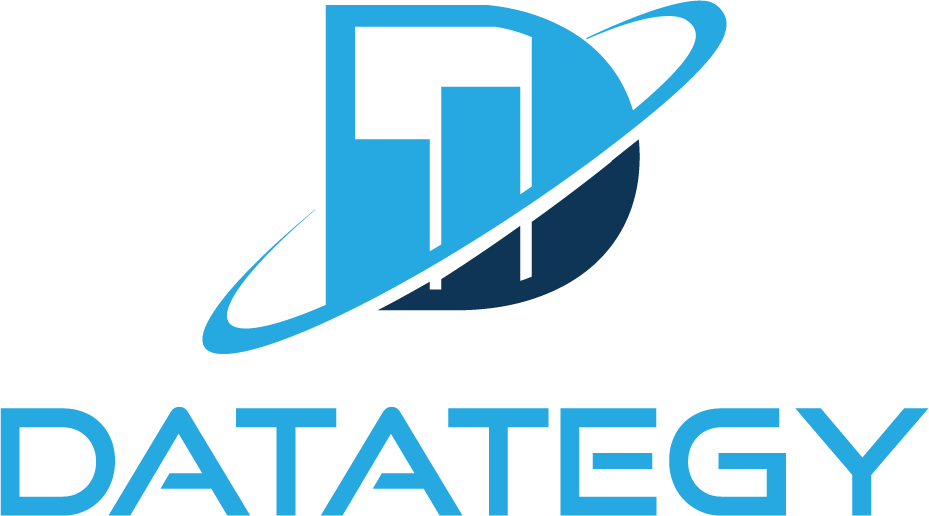