How does AI enhance and accelerate ESG Strategies? The first...
Read MoreAdvanced Artificial Intelligence PDF 2025
Table of Contents
ToggleArtificial intelligence has rapidly transformed the technological landscape, becoming a cornerstone of innovation across diverse industries. From generative AI and agentic AI to large language models, these advanced solutions are reshaping how businesses operate and deliver value.
According to Forbes: 64% of businesses believe that AI will help increase their overall productivity.
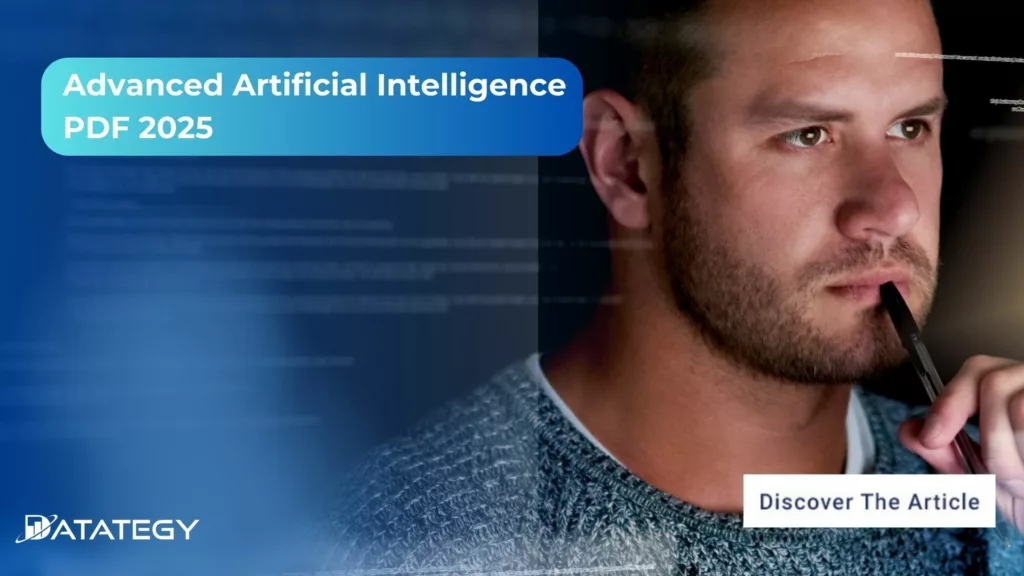
In this article, we’ll explore the latest AI technology trends and dive into sector-specific PDFs that highlight their transformative impact.
Artificial Intelligence Evolution Over Time
1980: AI in the 1980s was mostly concentrated on rule-based, expert, and symbolic systems. These methods used structured data and preset algorithms to mimic human decision-making in particular fields. Notwithstanding its promise, the development of AI during this time was beset by serious obstacles, such as a lack of large-scale datasets and a shortage of processing capacity, which hampered its wider use and performance.
1990-2000: The rise of machine learning (ML) techniques in the 1990s and early 2000s signaled a change in the direction of AI. The ability of AI systems to learn from data increased with the development of statistical techniques and algorithms such as decision trees and support vector machines.
Advances in robotics, computer vision, and natural language processing during this time period increased AI’s potential. Innovations like IBM’s Deep Blue’s 1997 victory against a global chess champion demonstrated AI’s capacity to solve challenging issues.
2010: With the introduction of deep learning in the 2010s, fuelled by developments in neural networks and the availability of large datasets, artificial intelligence entered a new age. Recurrent neural networks (RNNs) improved language comprehension, whereas convolutional neural networks (CNNs) transformed picture recognition.
Through applications like recommendation engines, virtual assistants, and driverless cars, artificial intelligence started to encroach on daily life. With the help of cloud computing and the exponential expansion of data, businesses use AI to optimize operations and develop new business models. Platforms like TensorFlow and PyTorch made AI development more accessible and encouraged creativity in a variety of sectors.
2022 and now: By 2022, artificial intelligence (AI) had developed into an advanced and revolutionary technology with uses in almost every industry. The strength of generative AI was demonstrated by large language models like GPT and multimodal systems, which let computers to create text, visuals, and even music that resembled that of a person.
The autonomy, decision-making, and adaptability of agentic AI systems started to increase. To make sure AI’s development is in line with social norms, ethical issues such as prejudice, privacy, and regulatory compliance become crucial to the conversation. This evolution from symbolic AI to the intelligent and adaptable systems of today demonstrates AI’s remarkable potential to transform whole industries and enhance people’s lives everywhere.
What are the Key Trends in AI for 2025
Here are 8 key trends in AI for 2025:
Agentic AI
The term “agentic AI” describes artificial intelligence systems that operate with some autonomy, allowing them to see, make decisions, and take action in dynamic settings without continual human supervision. The AI is able to handle difficult jobs and make judgments in real-time because to its sophisticated algorithms, contextual awareness, and flexibility. In robots, autonomous cars, and smart systems, where prompt and accurate decision-making is essential, agentic AI has a particularly significant influence.
The capacity of agentic AI to integrate many data streams and adjust to novel circumstances without pre-programmed instructions is its primary novelty. In contrast to conventional AI, which is predicated on rigid rules or specific goals, agentic systems are made to pick up new skills and adjust their tactics in response to their surroundings.
Reinforcement learning and sophisticated neural network topologies frequently drive this evolution, allowing the AI to optimize its behaviors in response to input and results. Agentic AI, for example, uses real-time sensor data in autonomous cars to adapt to erratic situations and safely and effectively handle traffic.
Generative AI
Generative AI employs advanced machine learning models to create content, such as text, photos, audio, and video. In order to generate outputs that replicate human originality and authenticity, this technology makes use of neural networks, namely generative adversarial networks (GANs) and transformer-based architectures. Generative AI can create very realistic and contextually relevant content by identifying patterns in large datasets.
Generative AI has a wide range of innovative applications. It enhances human creativity and facilitates quick prototyping in the creative sectors by producing music, art, and even screenplays. Generative AI streamlines processes and improves consumer engagement in company by powering chatbots, tailored marketing campaigns, and content creation. Generative AI helps the healthcare industry by producing synthetic medical data for training and research, and it is also used in engineering and design to quickly iterate prototypes.
Edge AI
Instead of depending on centralized cloud computing, edge AI refers to the direct implementation of artificial intelligence models and algorithms on devices at the network’s edge, such as smartphones, Internet of Things (IoT) devices, and driverless cars. Because decentralization eliminates the latency and bandwidth constraints that come with sending massive volumes of data to distant computers, AI can function in real-time, processing data locally. Edge AI guarantees quicker decision-making, improved privacy, and less need on constant internet access by executing AI directly on devices.
The capacity of edge AI to process and analyze data locally, resulting in immediate insights and enabling real-time actions, is one of its main advantages. This is especially important for applications like industrial robots, driverless cars, and medical monitoring systems that require low-latency answers. For instance, without waiting for data to be sent to a remote server, an autonomous car driven by edge AI may assess sensor data instantly to identify impediments and make navigational decisions.
Similarly, by evaluating user behavior locally and making instantaneous settings adjustments, edge AI in smart home devices may offer personalized experiences.
Retrieval-Augmented Generation (RAG)
Retrieval-Augmented Generation (RAG) is a new method that integrates an external knowledge retrieval system with the capabilities of generative models. RAG allows the model to search a database or the internet in real-time for pertinent information, rather than depending just on the training data that was supplied. The model is especially useful for tasks like question-answering, conversational AI, and content production since this external retrieval phase improves the model’s capacity to provide replies that are more knowledgeable, correct, and contextually relevant.
A retriever and a generator are the two primary parts of the RAG design. While the generator utilizes the information collected to create a logical and contextually appropriate answer, the retriever looks through a knowledge base for pertinent documents or information. The drawbacks of standard generative models, which are limited to the knowledge they were trained on and may find it difficult to address queries demanding current or domain-specific information, can be solved by models using this hybrid method.
For instance, in a medical application, a RAG model may find the most recent research papers to address a certain medical question, guaranteeing that the solution is up-to-date and correct.
Small Language Models (Small LLMs)
Compact versions of large language models (LLMs), known as small language models (SLMs), are designed to offer comparable features but consume less memory, computing power, and inference energy. Despite the fact that big LLMs like GPT-3 have attracted a lot of interest because to their remarkable language-generating capabilities, their resource requirements make them less appropriate for implementation on edge devices or in settings with constrained computational resources. By balancing efficiency and performance through model architecture optimization, small LLMs provide a more approachable option.
Small LLMs are seen to be more environmentally friendly than big versions due to their lower energy consumption and lower data center requirements, in addition to their usefulness. Their lessened environmental effect is especially significant as the AI community works to lower the carbon footprint associated with big model deployment and training.
By enabling more egalitarian access to AI capabilities, tiny LLMs also let people and organizations take use of state-of-the-art NLP technology without having to invest in the extensive infrastructure needed for large-scale models.
Visual Modes Language (VML)
Multimodal neural networks, which integrate transformer-based designs for natural language processing (NLP) with convolutional neural networks (CNNs) for image processing, are commonly used to construct VML models. Through this fusion, the system is able to comprehend the connections between verbal concepts and visual aspects in addition to identifying objects and situations inside photographs. VML,
for instance, may be applied to visual question answering, where the system responds to queries about an image by integrating data from textual and visual inputs, or picture captioning, where the model produces logical and contextually appropriate descriptions of images.
A key advancement in AI is the combination of language and vision models, which enables more complex and subtle interactions with the surroundings. VML is being utilized more and more in domains like accessibility, where AI can provide visually impaired individuals a more thorough awareness of their environment, and e-commerce, where AI systems may suggest items based on both visual and textual characteristics.
How to implement AI Solutions within your Organizations
Select the Best AI Tool
First of all , you have to select the finest AI tools and technologies to suit your particular requirements. Depending on your objectives, you may need to leverage technologies like computer vision, machine learning, or predictive analytics. For instance, computer vision is particularly useful for visual inspections and defect identification, while machine learning models may be used to forecast problems or optimize operations.
Predictive analytics tools are helpful in anticipating maintenance needs. You must choose technologies that work with your existing systems and infrastructure to guarantee smooth integration and scalability for future needs.
Data Collection and Configuration of the Infrastructure
Gathering relevant data is crucial for training your AI models, as data is the foundation of any AI application. This includes sensor data, production data from the past, and any other inputs pertaining to manufacturing. Since AI models rely on high-quality data to function properly, accurate, clear, and organised data are crucial.
Set up the needed infrastructure. Make sure it connects with your current ERP or MES systems. This will allow for data collection and storage. Installing IoT sensors on manufacturing lines may be needed for real-time monitoring. These sensors collect data on factors like vibration, temperature, and pressure. This helps with analysis and improves efficiency.
Choose the Right AI Model
Once the data has been collected and analyzed, the next step is to train AI models tailored to your quality control processes. This means putting the data into machine learning techniques or deep learning models for computer vision applications.
For example, machine learning models can learn to find defects using past data. However, computer vision systems need many labeled photos to spot product issues. Customization is important to make sure the AI models fit your production environment and product details. Testing, validation, and iteration are key to making sure the models work well in real-life situations.
Pilot Testing and Validation
Before using AI systems in your entire production line, run a pilot test. This test will help you check the system’s performance in a controlled setting. Focus the pilot on a small part of your manufacturing process. This will help you validate the AI models and see how they affect quality control.
During this phase, monitor the AI system’s ability to spot errors, optimize workflows, and predict maintenance needs. Consult with operators and quality control teams to identify any issues or possible areas for improvement. Make the necessary adjustments to the AI models or system integration before scaling the solution.
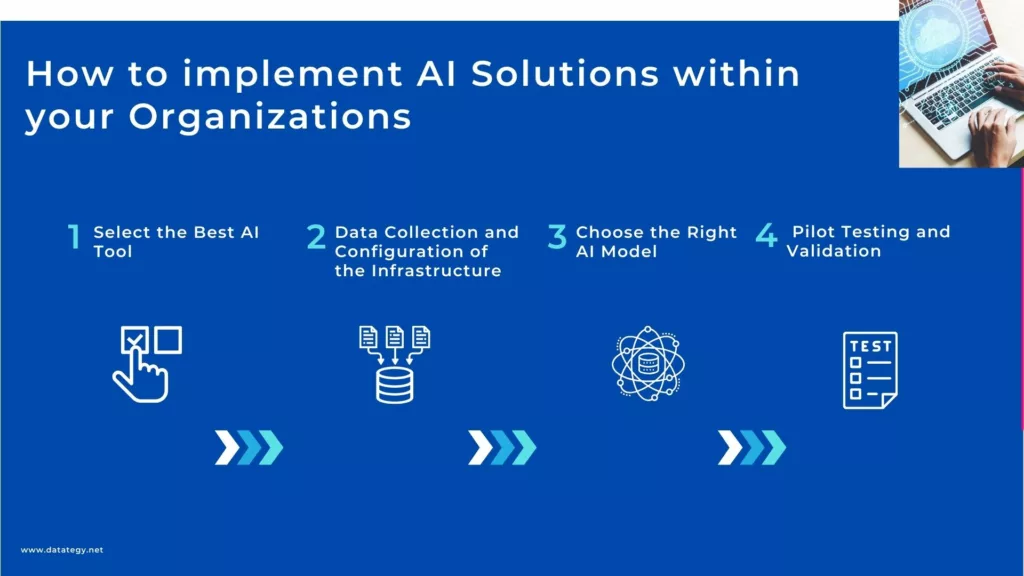
What are the Main challenges of AI adoption in 2025?
Algorithmic Explainability and Transparency
The increasing complexity of AI systems, especially with the emergence of deep learning and neural networks, will make it increasingly difficult to maintain interpretability and transparency. By 2025, businesses will be under more pressure than ever to make AI models easier to understand, particularly in industries where AI judgments have a big impact, including healthcare, banking, and the legal system.
One of the main obstacles to the acceptance of AI models is their “black box” character, which makes it difficult to understand how they make decisions. To fulfil legal standards and foster confidence, it will be crucial to develop interpretable AI approaches, such as explainable AI (XAI), which will enable AI systems to be audited and their results validated.
AI Systems' Scalability
In 2025, scaling AI solutions across organizational settings will still be a technological challenge. Due to infrastructure constraints, high processing needs, and the requirement for real-time processing, many organizations find it difficult to implement AI models on a broad scale. Powerful hardware and specialized infrastructure, such GPUs and distributed computing systems, are needed for large AI models, like deep neural networks.
Organizations must create scalable AI architectures that are tailored for cloud and edge settings if they want to see broad adoption. Furthermore, complex orchestration and deployment techniques will be needed to integrate AI into current IT infrastructures and guarantee that AI solutions can function in a variety of settings, from cloud-based services to on-premises data centers.
Privacy and Security in AI Implementation
There are major security and privacy concerns with AI systems, especially those that handle sensitive data. By 2025, businesses will have to deal with the escalating worries about data security in AI-powered settings.
Data breaches and adversarial assaults must be prevented for models trained on sensitive or private information, such as medical records or financial transactions. Privacy issues will be reduced by using strategies like federated learning, in which data processing takes place locally on devices instead of being sent to central servers.
These models must, meanwhile, be resilient enough to manage a variety of datasets and stay safe from malevolent actors. To guarantee safe and legal adoption, it will be crucial to guarantee the security of AI systems at every stage of their development, from model training to deployment and monitoring.
Real-world Examples of Artificial Intelligence on PDF
How to choose the best AI solution for your data project?
In this white paper, we provide a comprehensive overview of the diverse AI solutions currently available on the market, offering in-depth insights into their functionalities, applications, and potential benefits.
We aim to help organizations understand the wide range of AI technologies and how they can be leveraged to enhance business operations. From machine learning and natural language processing to computer vision and automation tools, we cover various AI models and platforms that cater to different industry needs.
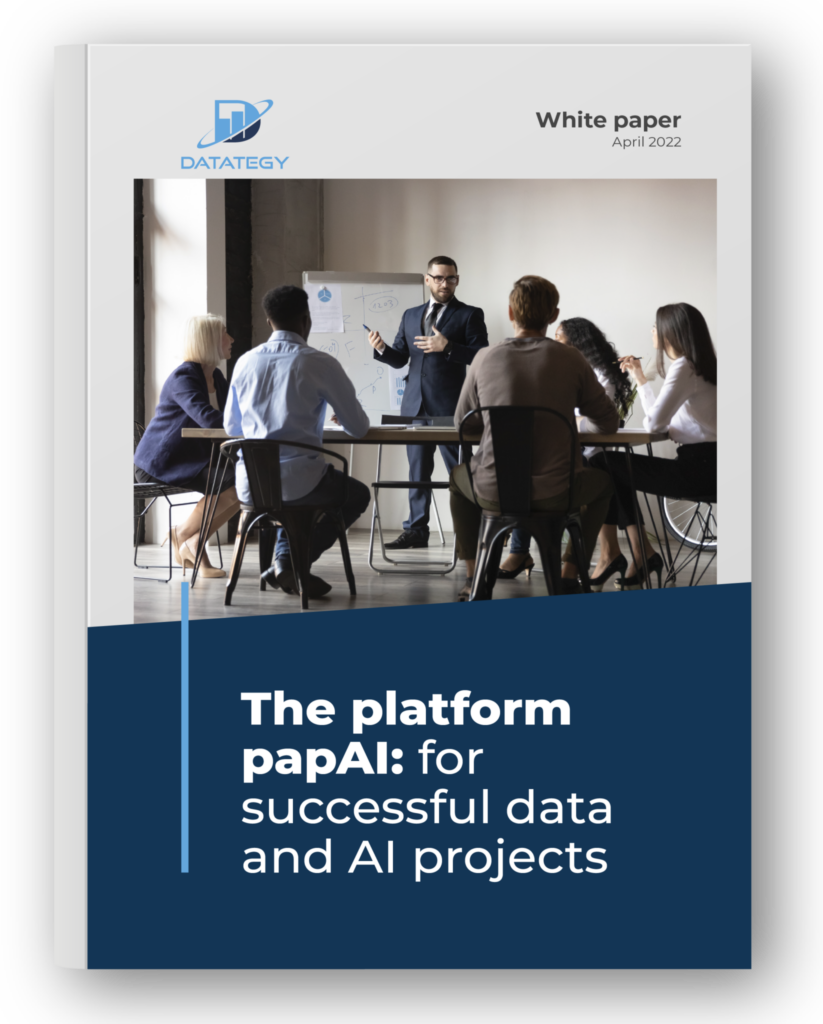
Banking industry : Lead your Business teams to embrace AI
The banking services industry has long been at the forefront of AI implementation, leveraging advanced technologies to streamline operations, enhance customer experiences, and mitigate risks. However, as AI becomes increasingly central to decision-making processes in areas such as fraud detection, credit scoring, and risk management, the need for transparency and trust has never been greater.
This is where Explainable Artificial Intelligence (XAI) comes into play, offering insights into how AI systems reach their conclusions, ensuring decisions are not only accurate but also understandable to both regulators and end-users.
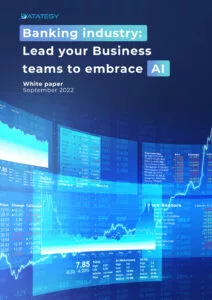
How AI impacts clinical decision Making
Healthcare is a strategic field for artificial intelligence (AI) due to its capacity to address some of the industry’s most pressing challenges while driving innovation.
The sector generates vast and complex datasets, including patient records, diagnostic images, genomic information, and real-time monitoring data from wearable devices. AI’s ability to process, analyze, and interpret these multi-dimensional datasets with speed and precision positions it as a transformative tool for healthcare providers.
Unlike traditional approaches, AI can uncover subtle patterns and correlations within the data that might not be immediately apparent to human experts, aiding in more accurate diagnoses and personalized treatment plans.
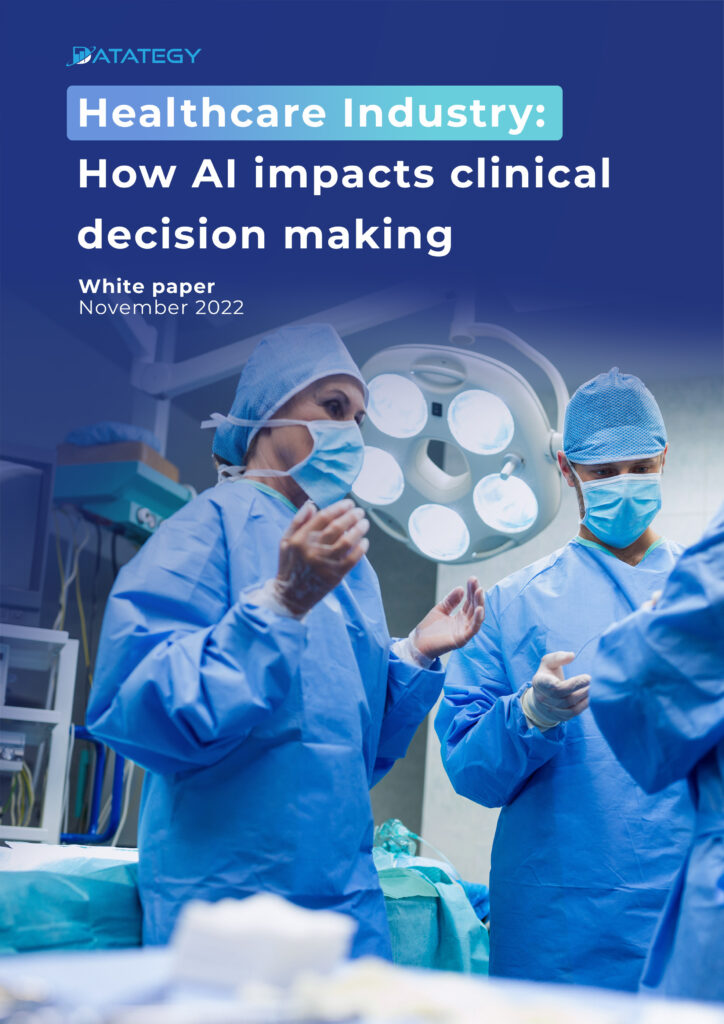
Leading the data revolution: CDO role in today's organizations
The need for Chief Data Officers (CDOs) is rapidly expanding as data becomes increasingly central to the modern corporate environment. Organizations are recognizing that leveraging data effectively is no longer a competitive advantage but a fundamental requirement for success.
The CDO plays a pivotal role as the architect of data-driven strategies, ensuring that data assets are not only accessible but also actionable. This critical position drives digital innovation and transformation by aligning data initiatives with business goals, fostering a culture of data literacy, and promoting informed decision-making across all levels of the organization.
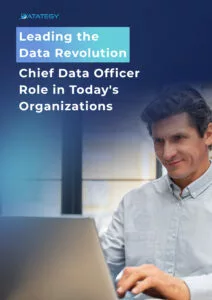
AI for Retail: A Comprehensive Guide to Choosing the Best Solution for Your Business Needs
Choosing the right AI solution for your retail business requires careful evaluation of key factors. Ensure seamless integration with existing systems to avoid disruptions and prioritize data privacy and security to protect sensitive customer information.
The solution should align with your business objectives, whether improving customer experiences, optimizing inventory, or enhancing demand forecasting. Scalability is equally critical, enabling the AI to adapt as your business grows. By considering these aspects, you can select a solution that drives efficiency and innovation. Download our free whitepaper to explore actionable strategies for leveraging AI to thrive in today’s competitive retail landscape.
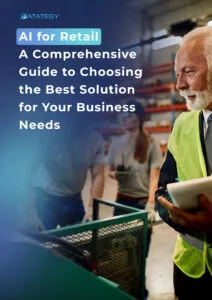
Empowering the Energy Industry through AI Revolution: The Critical Role of CDOs
Chief Data Officers (CDOs) in the energy industry face significant challenges in adopting AI technologies, including driving a cultural shift within organizations to embrace digital transformation. Successfully integrating AI requires overcoming resistance to change, fostering data-driven decision-making, and ensuring teams understand the value of AI solutions.
Despite these hurdles, CDOs play a critical role in exploring and implementing AI to boost operational efficiency, lower costs, and achieve sustainability objectives. To learn how CDOs can navigate these challenges and leverage AI for transformative results, download our free whitepaper for actionable insights and strategies tailored to the energy sector.
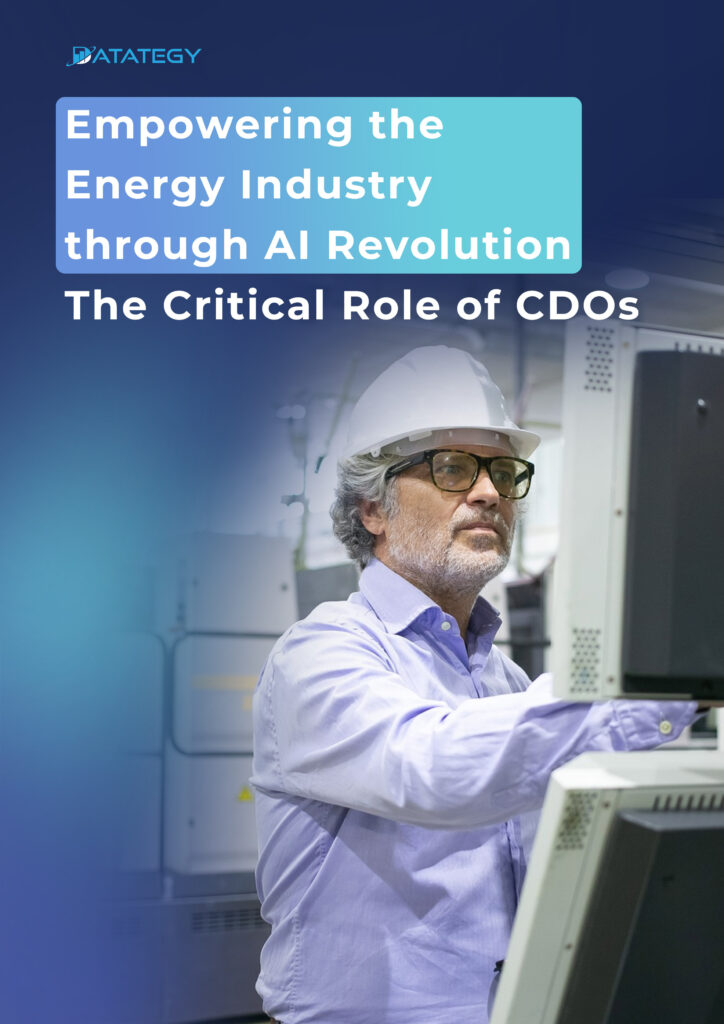
Demystifying AI: A Comprehensive Guide to Key Concepts and Terminology
This guide introduces essential AI terminology that every beginner should know. Whether you’re a student exploring the future of technology, a business owner aiming to integrate AI into your operations, or simply curious about this transformative field, this guide lays the groundwork for understanding key concepts.
By familiarizing yourself with these terms, you’ll gain a clearer perspective on AI’s capabilities, applications, and potential impact. To deepen your knowledge and confidently navigate the world of AI, download our free whitepaper for a comprehensive glossary and actionable insights designed to empower beginners in this rapidly evolving domain.
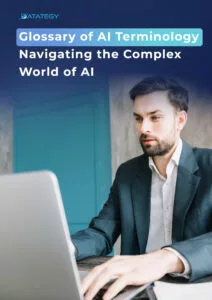
From Data to Action: How AI is Transforming the Energy Industry
The integration of AI and machine learning (ML) technologies is set to transform the energy industry by revolutionizing how energy is produced, distributed, and consumed. Advanced algorithms and data analytics enable enhanced efficiency, improved safety, and greater sustainability in energy systems. From optimizing grid performance to predicting maintenance needs and supporting the transition to renewable energy, AI and ML are driving innovation across the sector.
To explore how these technologies are reshaping the energy landscape and unlocking new opportunities, download our free whitepaper. Gain valuable insights and strategies to harness AI and ML for a smarter, more sustainable future in energy.
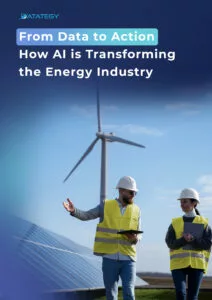
AI and Machine Learning for Sales: The Key to Unlocking Growth Potential
The development of artificial intelligence (AI) has profoundly transformed the sales industry, driving remarkable evolution over time. AI has revolutionized how companies approach sales strategies and processes, enabling smarter decision-making, enhanced customer engagement, and optimized operations. By leveraging AI, businesses can gain actionable insights, streamline workflows, and personalize customer experiences at scale.
In this whitepaper, we delve into the concept of AI in sales, trace its historical evolution, and explore its many advantages for businesses. Download our free whitepaper to discover how AI is reshaping sales and learn actionable strategies to unlock its full potential for your organization.
.
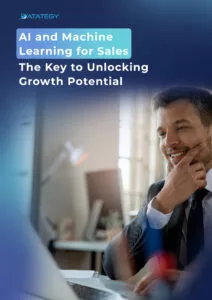
A to Z of Generative AI: An in-Depth Glossary
Generative AI is a groundbreaking form of artificial intelligence that enables machines to autonomously create text, images, music, and more. By harnessing the power of algorithms and deep learning, it is revolutionizing industries through automation, enhanced personalization, and fostering innovation. As businesses increasingly adopt Generative AI, understanding its terminology and applications is crucial.
This guide provides a solid foundation in Generative AI terminology, helping you navigate this exciting field with confidence. To dive deeper into how Generative AI is transforming industries and to explore actionable insights, download our free whitepaper today and stay ahead in this rapidly evolving space.
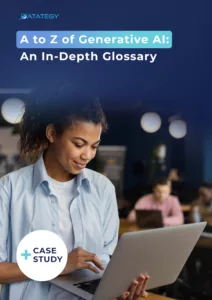
Create your Own AI Models using papAI solution
If you’re looking to build a custom AI solution tailored to your specific use case, turn to papAI, the all-in-one AI platform designed to help you seamlessly develop, deploy, and optimize your AI applications. With papAI, you can harness advanced tools and capabilities to address your unique business needs, ensuring a smarter and more efficient solution.
Schedule a demo now to see how our papAI solution can improve your AI deployement.
Interested in discovering papAI?
Our AI expert team is at your disposal for any questions
How Is AI Transforming Digital Twin in the Industry?
How Is AI Transforming Digital Twin in the Industry? Artificial...
Read More“DATATEGY EARLY CAREERS PROGRAM” With Julien Courteville
“DATATEGY EARLY CAREERS PROGRAM” With Julien Courteville Hello, my name...
Read MoreDatategy + Nedd Technologies: First Center of AI Excellence in USA
Datategy + Nedd Technologies: First Center of AI Excellence in...
Read More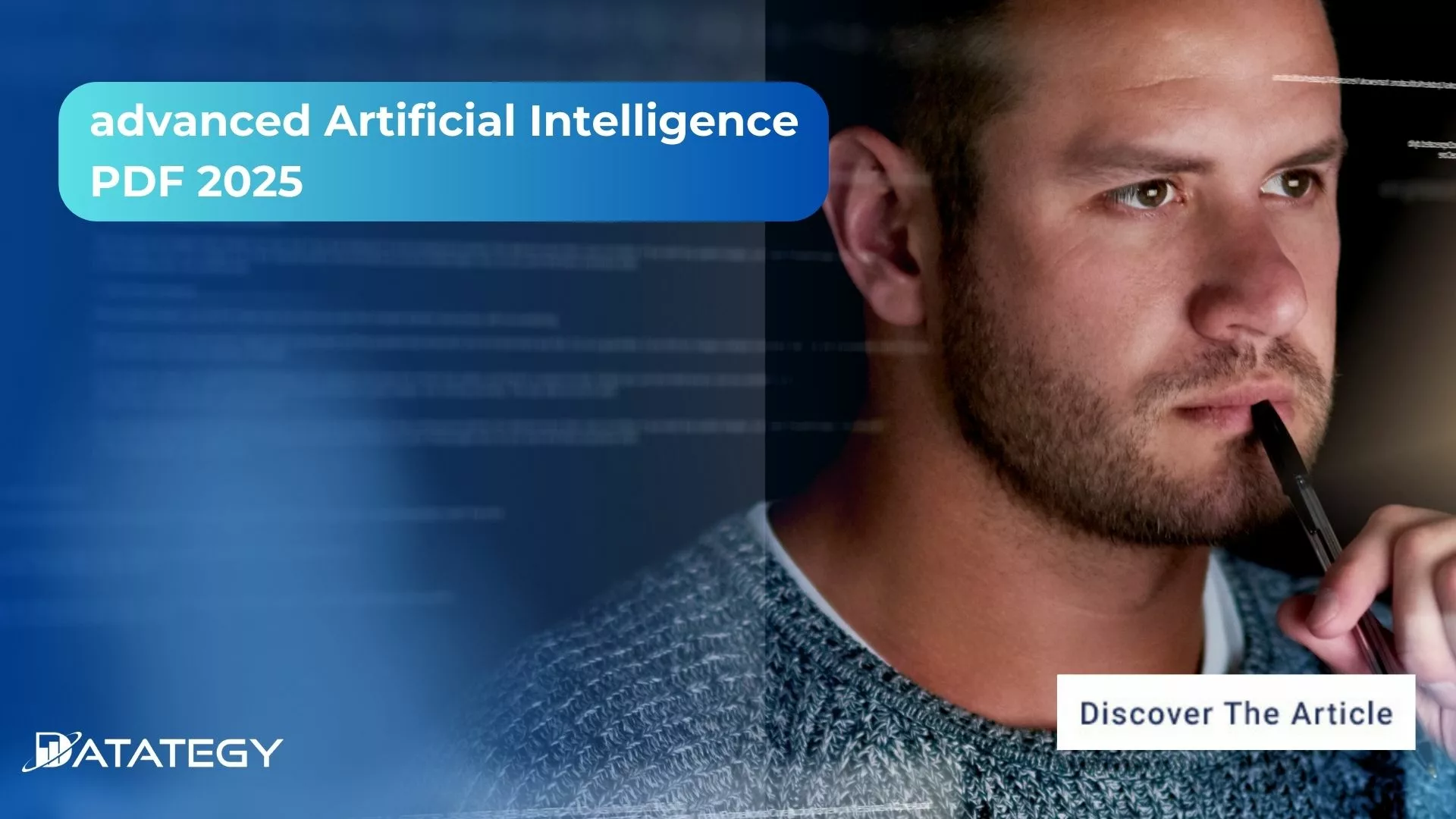
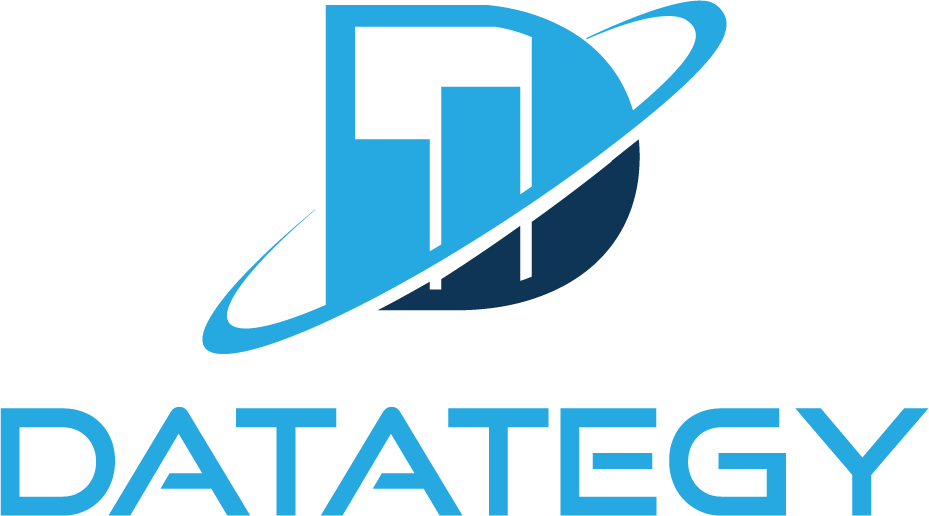