“DATATEGY EARLY CAREERS PROGRAM” With Julien Courteville Hello, my name...
Read MoreHow Generative AI is Transforming Manufacturing?
Table of Contents
ToggleGenerative AI has emerged as a game-changer in a wide range of industries, and manufacturing is no exception. Thanks to its ability to generate images, text, songs and videos, generative AI covers a wide range of needs for stakeholders in the manufacturing industry. The future of manufacturing is generative AI. Imagine intelligent factories capable of adapting in real-time to market changes. That’s what generative AI promises.
Gartner predicts that by 2026, more than 80% of enterprises will have used Generative AI APIs or deployed Generative AI-enabled applications in production environments.
Find out how papAI can improve the deployment of AI projects in the Manufacturing Industry.
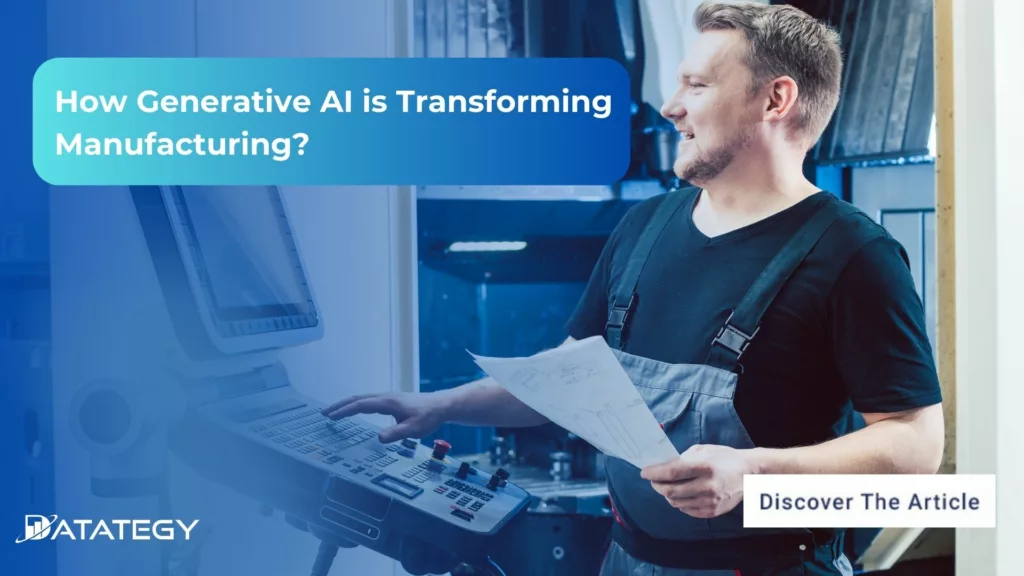
in this article we will explore real-world use cases of using generative ai in the manufacturing industry and its benefits.
What is Generative AI?
In the field of artificial intelligence, generative AI uses data to generate new content. In contrast to traditional AI, which concentrates on finding patterns and forecasting outcomes, generative AI generates unique outputs like text, images, music, and even 3D models. Large datasets are used for training, and sophisticated algorithms, such as neural networks are used to comprehend the underlying structures. It can produce new content that mimics the characteristics, tone, or style of the data it was exposed to after it has been trained. Examples include programs like DALL-E for creating images and GPT for creating text.
Why Manufacturing Needs Generative AI?
A key component of the global economy, manufacturing is under increasing pressure to change as a result of shifting consumer demands, advances in technology, and growing sustainability concerns. Even though they are dependable, traditional methods frequently fall short of the demands for increased customisation, quicker production cycles, and resource efficiency. Manufacturers must embrace creative solutions in an increasingly competitive environment to remain relevant and satisfy consumer demands. This necessitates a move away from inflexible, linear procedures and towards more flexible, data-driven strategies that can quickly adjust to shifting supply chain and demand conditions.
The Key Technology Behind Generative ai
Networks of Neural Systems
The foundation of generative AI is neural networks, which are made to mimic how the human brain interprets data. They are made up of interconnected layers of nodes, or artificial neurons. The network is able to learn intricate patterns and relationships because each node processes input data and forwards the results to the following layer. A subset of machine learning called “deep learning” makes use of multi-layered deep neural networks to process complex data and generate precise predictions. These networks perform especially well on tasks that combine text, audio, and images.
Generative Adversarial Networks (GANs)
One particular kind of neural network architecture that is essential for producing high-quality content is GANs. They are made up of two rival networks: a discriminator and a generator. New data samples are generated by the generator, and their authenticity is assessed by the discriminator. The generator improves its outputs based on the discriminator’s feedback, and the two networks cooperate. High-quality, realistic images, audio, or text can be produced through this iterative process.
Transformer Models
Particularly in natural language processing, transformer models have transformed generative artificial intelligence. Transformers handle data in parallel, which makes them much more efficient for large datasets than traditional models, which process data sequentially. Due to their exceptional context awareness, these models are able to produce text that is both logical and appropriate for the given context. Many cutting-edge AI tools, such as language models like GPT, are built on top of them.
How to implement Generative AI within your Organizations?
Choose the Right AI Tools
Choosing the best AI tools and technologies to meet your unique needs comes next after determining which areas need improvement. You might need to use technologies like computer vision, machine learning, or predictive analytics, depending on your goals. For example, machine learning models can be used to predict failures or optimize workflows, while computer vision is especially helpful for visual inspections and defect detection. Tools for predictive analytics are useful for predicting maintenance requirements. In order to ensure seamless integration and scalability for future requirements, you must select tools that are compatible with your current infrastructure and systems.
Data Gathering and Infrastructure Configuration
Since data is the cornerstone of any AI implementation, collecting pertinent data is essential for training your AI models. This comprises sensor data, historical production data, and any additional manufacturing-related inputs. Accurate, clean, and structured data are essential because AI models depend on high-quality data to work well. Install the infrastructure required to facilitate data gathering and archiving, making sure that it integrates with your current ERP or MES systems. In order to enable real-time monitoring and analysis, it might also be necessary to install IoT sensors on production lines to collect data in real-time on variables like temperature, pressure, or vibration.
Customization and Model Training
Training AI models that are specific to your quality control procedures comes next after the data has been gathered and processed. For computer vision applications, this entails feeding the data into deep learning models or machine learning algorithms. For instance, computer vision systems will need sizable datasets of labeled images to detect product flaws, whereas machine learning models can be trained to identify defects based on historical data. In order to make sure that the AI models are tailored to your manufacturing environment and the particulars of your products, customization is essential. To make sure the models function well in practical settings, testing, validation, and iteration are crucial.
Pilot Testing and Validation
Perform a pilot test to assess the system’s performance in a controlled setting prior to implementing AI systems throughout your whole production line. To validate the AI models and evaluate their effect on quality control, the pilot should concentrate on a small section of your production process. Keep an eye on the AI system’s capacity to identify flaws, streamline processes, and forecast maintenance requirements during this stage. To find any problems or potential areas for improvement, get input from operators and quality control teams. Prior to scaling the solution, make the required modifications to the AI models or system integration.
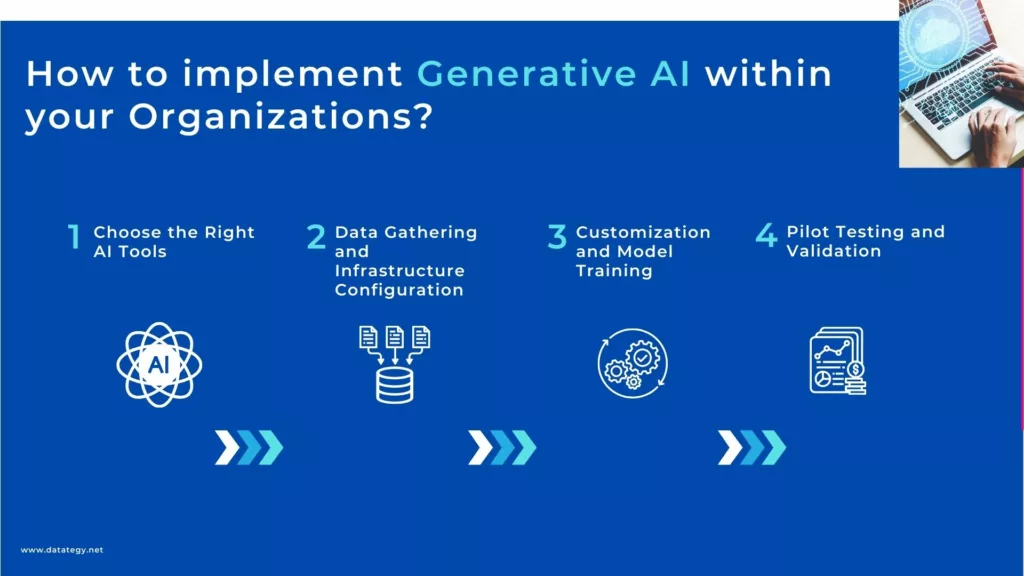
Process of Gen AI Implementation
Real-World Applications of Generative AI in Manufacturing Industry
Generative AI in Predictive Maintenance
By giving maintenance workers prompt, data-driven advice on how to handle equipment problems, generative AI is improving predictive maintenance. Manually diagnosing issues is a common part of traditional maintenance, which can be laborious and cause delays. Maintenance personnel can repair equipment more quickly and effectively because to generative AI’s real-time insights and practical solutions. The AI creates customized recommendations on how to repair certain pieces of equipment by analyzing sensor readings, historical data, and patterns from prior maintenance operations. This decreases the possibility of errors and needless downtime by speeding up reaction times and increasing the accuracy of the corrections.
Additionally, generative AI assists maintenance staff by providing detailed instructions on how to adhere to established repair procedures. This involves suggesting particular equipment, components, and repair techniques in order to guarantee a consistent and efficient maintenance approach. By producing these instructions, artificial intelligence (AI) lessens reliance on memory or paper-based processes, providing a more dependable method that lowers human error. The ability to adhere to exacting repair procedures guarantees a faster turnaround and longer asset lifespan in vital businesses where downtime can have major financial consequences.
Generative AI in Quality Control and Assurance
By improving the precision and effectiveness of defect detection, generative AI is transforming quality assurance and control. Manual inspections and simple automated systems are frequently used in traditional quality checks, which can overlook minute defects or irregularities. However, the ability to generate synthetic data offers another layer of improvement for quality control teams: it enables the creation of virtual models, or “digital twins,” of pieces or form factors. These models simulate real-world conditions and failures, allowing maintenance teams to practice repairs virtually before performing them in the field. This minimizes errors and enhances team preparedness.
Generative AI may improve its forecasts and suggestions with continuing learning and real-time feedback, resulting in ongoing maintenance strategy optimization.. Manufacturers may drastically cut down on waste, rework, and customer complaints by incorporating AI into quality control, which will increase customer satisfaction with the final product. Research indicates that AI-powered quality control can lower defect rates by as much as 90%, increasing output and financial gain.
Generative AI in Supply Chain
For supply chain managers, generative AI provides significant advantages, especially in data-driven decision-making and demand forecasting. The AI will examine past data, market trends, seasonal patterns, and external factors like supply disruptions or economic shifts in order to provide supply chain managers with forecasts on certain items, locations, or time periods. Supply chain managers can make better judgments on production plans, procurement tactics, and inventory levels by utilizing this capacity. Generative AI continuously improves its predictions in real-time, enabling managers to make dynamic strategy adjustments rather than depending on static, one-time forecasts. In addition to improving agility and preventing stockouts or overstocking, the flexibility to obtain personalized projections at any time maximizes operational effectiveness and guarantees that output closely matches demand.
Apart from forecasting and optimization, generative AI enhances supplier-manufacturer collaboration. AI can provide useful information on supplier performance, recommending different suppliers or goods that can be more affordable. Additionally, it can pinpoint possible supply chain hazards like interruptions or bottlenecks and offer solutions to lessen them. Businesses may optimize their supply chains and build more robust, transparent, and responsive networks by incorporating generative AI into their operations. AI’s influence on supply chains will only increase as it develops, boosting productivity and competitiveness across sectors.
Generative AI for Manufacturing Workers
Manufacturing workers’ roles are changing as a result of generative AI, which improves their decision-making, productivity, and safety. Workers have always carried out their responsibilities through hard labor, frequent inspections, and intensive training. AI integration gives employees access to cutting-edge tools that increase productivity, decrease human error, and help them anticipate problems. Real-time production data analysis by AI-driven systems can identify possible issues or inefficiencies before they arise, empowering employees to take preventative measures. This guarantees a greater degree of accuracy and precision in their daily responsibilities in addition to streamlining their work procedures.
Based on real-time data, generative AI may help employees by providing customized recommendations and optimal solutions. For example, by modeling various situations and results, AI can produce the optimal assembly or manufacturing methods, assisting people in making well-informed choices. This lessens the need for continual supervision and decision fatigue. By creating immersive, AI-generated learning environments that let employees practice on virtual equipment or production lines, AI also helps with training. This type of online instruction guarantees that employees are equipped to manage both common and unforeseen circumstances.
What Challenges need to be Overcome before Implementing Generative AI
The Integration: The technological difficulty of integrating generative AI into current systems is another significant obstacle. The size and complexity of AI technologies are too much for many organizations’ legacy systems to manage. AI integration into these systems necessitates extensive training, customization, and upgrades—all of which can be expensive and time-consuming. Furthermore, to maintain their efficacy and alignment with corporate goals, AI systems frequently require ongoing monitoring and adjustment. In order to effectively support AI models, organisations must also construct the required infrastructure, which includes cloud solutions, processing capacity, and storage capacities. Indeed, according to a Deloitte survey, 58% of organisations stated that integration complexity was a major obstacle to the adoption of AI.
Ensuring Data Quality for Reliability: Before generative AI is widely used, there are a number of important issues that need to be resolved when using it in sectors like manufacturing, supply chains, or healthcare. The availability and quality of data is one of the main challenges. For generative AI models to work well, enormous volumes of high-quality data are required. However, a lot of businesses deal with problems like inconsistent, unstructured, or inadequate data, which might impair the performance of AI models. For AI to produce significant and trustworthy outcomes, data accessibility, consistency, and correctness must be guaranteed. Before using generative AI solutions, organisations must make an investment in strong data management systems to clean, standardise, and integrate their data.
Leading the data revolution: CDO role in today's organizations
The need for CDOs is expanding as a result of the growing significance of data in today’s corporate environment. Any organization’s success depends on the CDO, who is the primary force behind digital innovation and change.
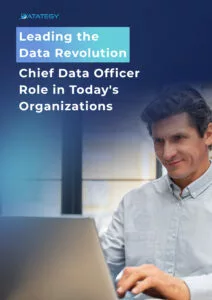
How papAI Helps Build Effective Generative AI Solutions?
With the help of papAI‘s simple deployment method, companies can swiftly incorporate generative AI into their current infrastructure. Suitable for enterprises of all sizes, the platform facilitates cloud-based solutions that grow effectively. With the increasing demand for AI-powered solutions, papAI guarantees that companies can build their AI capabilities without worrying about system performance or capacity constraints.
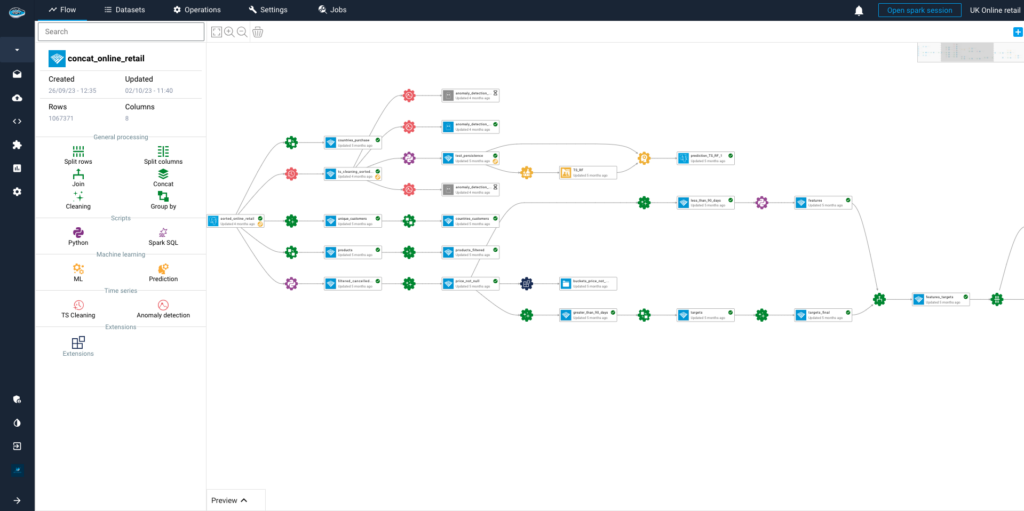
Generative AI vs. Context-Aware Generative AI:
The idea behind “Context-Aware Generative AI” is that AI could create content while simultaneously comprehending and adjusting to its surroundings. This AI paradigm fills the gap between processing unprocessed data and understanding it at a level that is comparable to that of a human. Imagine an AI system that does more than just produce text, images, or responses; it also takes into account the fundamental components of context, such as who, what, where, when, and why.
For example, the most recent version of papAI allows the user to select the text length that will be automatically generated for product descriptions. This criterion allows the user to write a description that is suitable for his internal brochures, social media accounts, and online store while also taking into consideration the latter’s constraints.
How papAI helps Leveraging Large Language Models for Precise Output ?
The ability of Large Language Models (LLMs) has sparked a significant change in artificial intelligence about how robots understand and generate human-like writing. These models have demonstrated proficiency in reproducing linguistic patterns and generating comprehensible text after being pre-trained on massive datasets. However, the true power of LLMs emerges when they are improved for context awareness, transforming their output from mere coherence to contextual relevance.
Applying these pre-trained models to specific industries, companies, or even particular applications might enhance LLMs. Pre-training provides them with a general command of language, but fine-tuning sharpened their understanding to match the nuances of real-world situations. This method enables a model to generate content that appeals to specific audiences and goals in addition to its broad language proficiency.
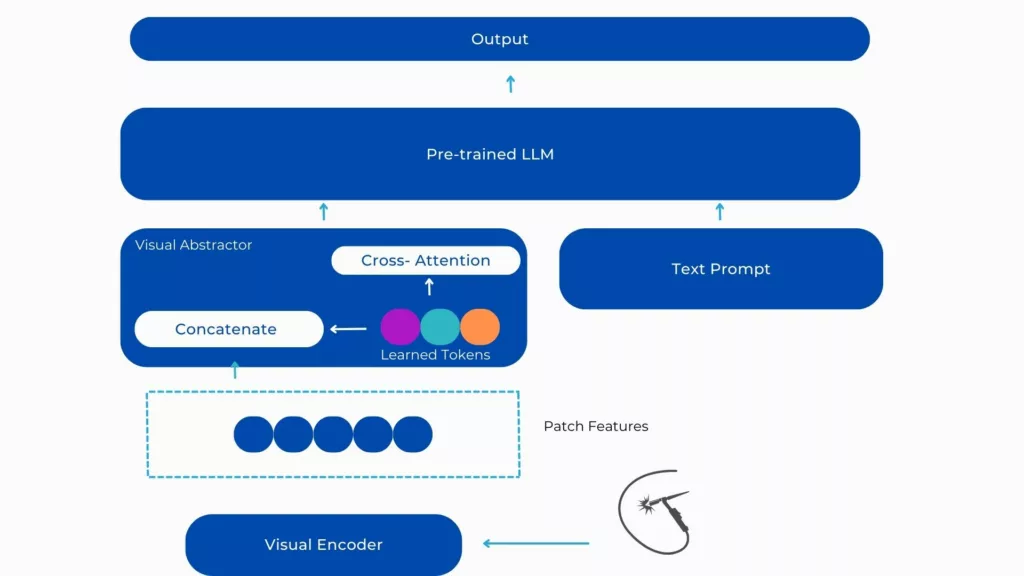
Watch papAI Demonstration & Create your Own Generative AI Application
To see how papAI can transform your operations with powerful, context-aware predictive insights, book a demo today and discover how our AI platform can optimize your processes, improve decision-making, and boost your bottom line.
Generative AI creates new content like text or images, while traditional AI focuses on pattern recognition and predicting outcomes based on existing data.
Generative AI offers flexible, data-driven solutions for faster production, enhanced customization, and improved resource efficiency, helping manufacturers adapt to market shifts and increasing competitiveness.
Generative AI analyzes real-time data, offering maintenance teams actionable, data-driven recommendations, optimizing repairs, reducing errors, and extending equipment lifespan by adhering to standardized processes.
Challenges include integrating AI into legacy systems and ensuring high-quality, consistent data for training models. Both require significant investments in infrastructure and data management systems.
Interested in discovering papAI?
Our AI expert team is at your disposal for any questions
Datategy + Nedd Technologies: First Center of AI Excellence in USA
Datategy + Nedd Technologies: First Center of AI Excellence in...
Read MoreHow AI Drives the Evolution of Industry 4.0
How AI Drives the Evolution of Industry 4.0 Today, Industry...
Read MoreOptimize RAG Systems Like a Pro with Fine-Tuning
Optimize RAG Systems Like a Pro with Fine-Tuning As artificial...
Read More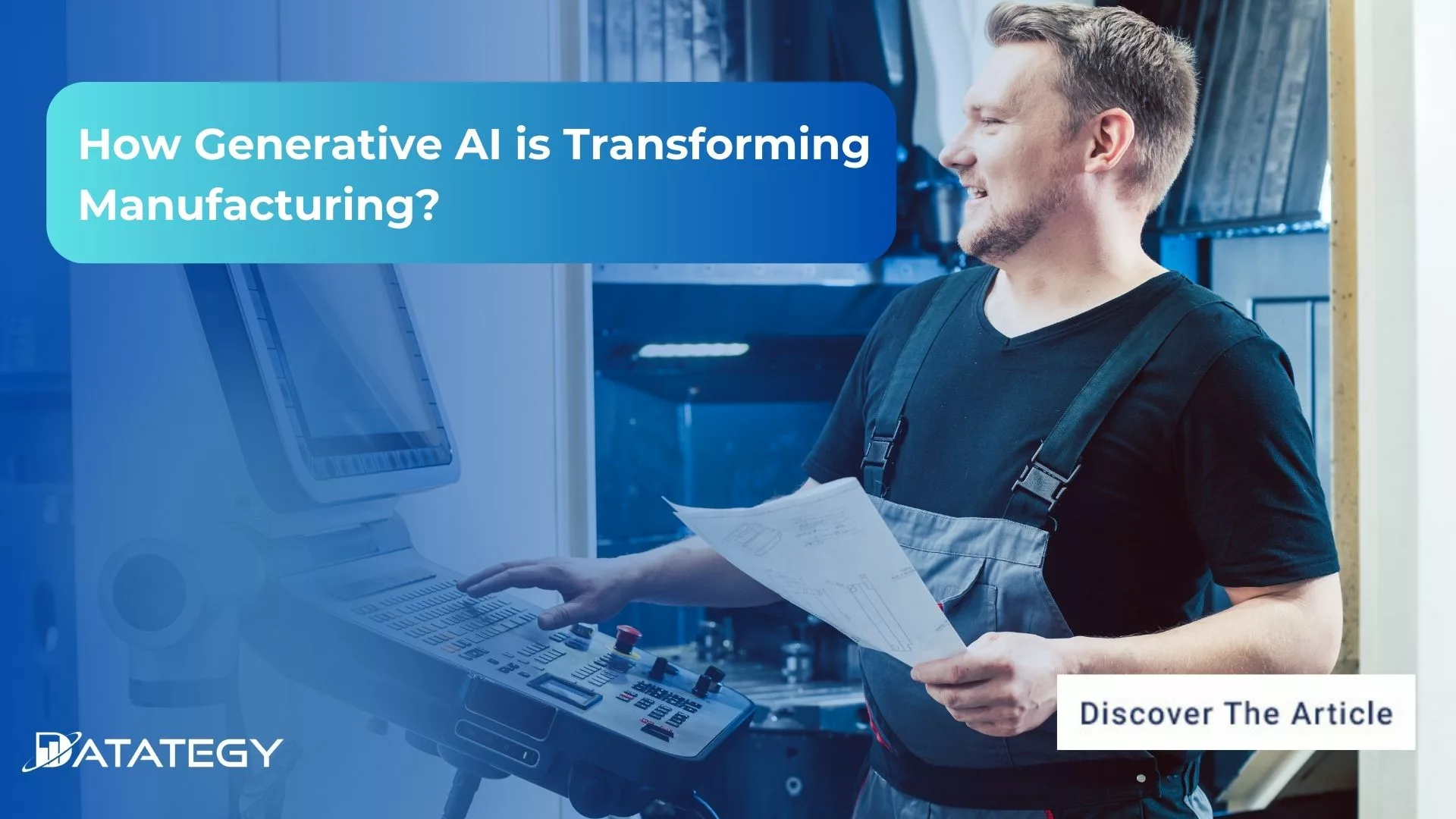
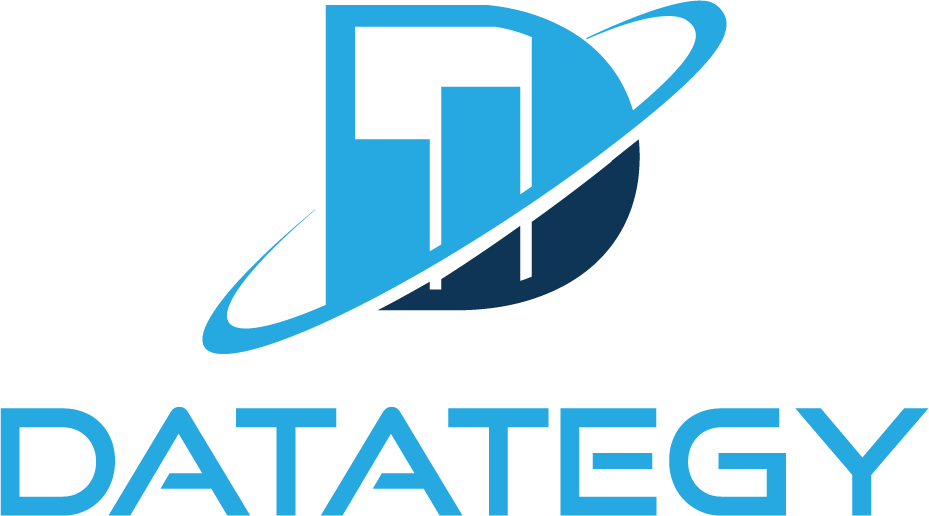