The Next AI Frontier: Jean-Marc Briquet Talks to Datategy’s Future...
Read MoreHow AI Revolutionizes Failure Prevention and Machine Lifetime Forecasting
Table of Contents
ToggleMachine failures have been a constant problem for industries for decades, frequently leading to expensive repairs, lost productivity, and costly downtime. Conventional maintenance methods were primarily reactive, repairing machines after they broke, or preventive, which, although helpful, frequently resulted in labor and part replacements that weren’t needed. Once the gold standard, these techniques lacked accuracy, leaving companies to deal with inefficiencies and unplanned malfunctions.
Today, the survival analysis approach has been made possible by developments in data analytics and predictive modeling. Predicting machine reliability and failure patterns is a ground-breaking use for this potent statistical tool, which was first created for medical research. Through the examination of past data, survival analysis offers practical insights into the likelihood of a machine failure, enabling industries to minimize downtime, optimize operational efficiency, and implement timely maintenance.
According to Gartner research, implementing AI-driven predictive maintenance can result in a 30% decrease in unplanned downtime
Find out how papAI can improve the deployment of AI projects in the Manufacturing Industry.
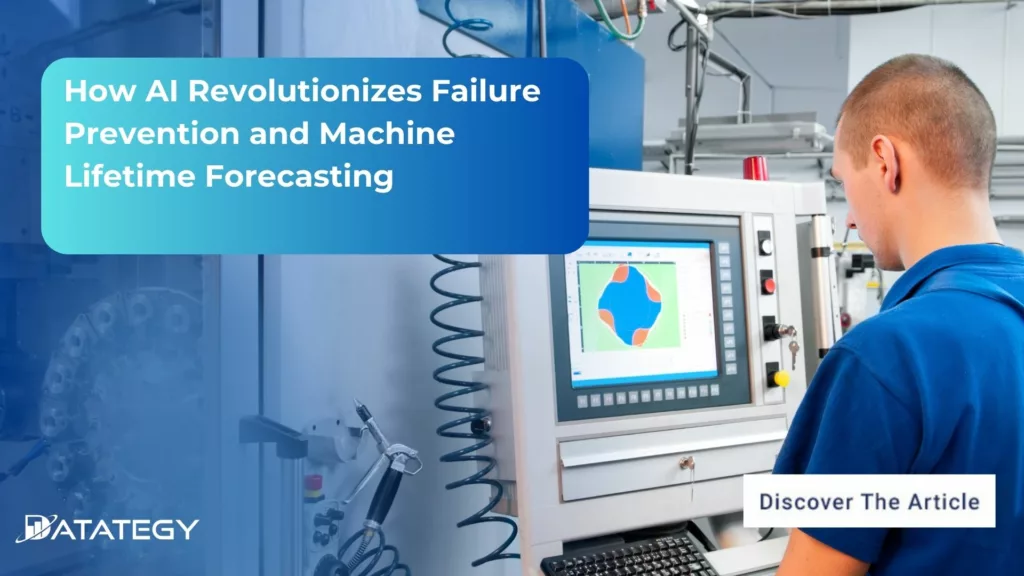
This article explores the benefits of leveraging artificial intelligence and survival analysis for failure prevention and extending machine lifetime forecasting.
Types of AI Models for Predictive Maintenance
-
Time-Series Models:
Time-series models, such as ARIMA (AutoRegressive Integrated Moving Average) or LSTM (Long Short-Term Memory networks), process sequential data like temperature, vibration, or pressure readings from sensors. These models excel in identifying trends and forecasting when critical thresholds will be breached, signaling potential failures. -
Classification Models:
Classification algorithms, such as decision trees or random forests, categorize machinery states into “normal,” “at risk,” or “critical.” By learning from labeled data, these models help maintenance teams prioritize which machines need immediate attention, reducing the risk of unexpected breakdowns. -
Regression Models:
Regression models, including linear regression or neural networks, estimate the exact time-to-failure based on input variables. These models are particularly useful for continuous data, providing precise predictions that enable well-timed maintenance actions. - Survival Analysis Models:
In predictive maintenance, survival models analyze historical data to estimate the remaining useful life of machinery. These models calculate hazard rates (failure probabilities at specific times) and survival functions (the likelihood of continued operation over time), enabling proactive decision-making to minimize downtime and optimize equipment usage.
What does Survival Analysis Mean?
Created in the domains of biology and medicine, survival analysis is a statistical technique used to examine the amount of time until a specific event takes place, such as the survival period of patients following treatment. Its fundamental goal is to comprehend and forecast the probability of an event occurring over time by estimating its potential occurrence time using historical data.
“Hazard rates,” which measure the likelihood that an event will occur at a specific time, and “survival functions,” which characterize the likelihood that an event won’t occur up to a specific time, are important ideas in survival analysis. Even in cases where certain outcomes are unclear or insufficient—a situation known as censoring—this methodology offers an organized method for analyzing time-to-event data.
What are the Key Principles of Survival Analysis?
Define the Event of Interest
Clearly defining the “event” under study is the first step towards applying survival analysis successfully. This is usually a failure in machinery, like a part failing, a system failing, or a piece of equipment stopping working. Accurately defining the event guarantees reliable and significant data gathering. For instance, think about whether a “failure” only refers to complete breakdowns or also includes partial malfunctions.
The integrity of the analysis depends on this clarity. Furthermore, by defining the event, the study’s objectives—like anticipating failures or enhancing maintenance plans—are in line with practical insights.
Analyze Time-to-Event Data
The main goal of survival analysis is to determine how long it takes for an event to occur. This could entail tracking the amount of time that passes between the beginning of operation and the first failure in machinery.
Time-to-event data provides information about how long a piece of equipment is dependable in typical circumstances. By going beyond averages, this method offers complex perspectives on durability and dependability. To guarantee high-quality insights from the analysis, precise time tracking and appropriate documentation of operational milestones are crucial.
Measure the Hazard Function
Assuming the system has not yet failed, the hazard function concentrates on the likelihood of failure at a particular point in time. This dynamic metric indicates when machines are at high risk of malfunctioning. A spike in hazards at 1,000 operating hours, for instance, can be a sign that a component needs to be replaced. Failures can be avoided proactively by keeping an eye on hazard rates.
What are the Benefits of Survival Analysis for Machine Maintenance
Increasing the Lifespan of Machines
Survival analysis assists in anticipating when machines are most likely to malfunction by examining the time-to-failure data and spotting trends that result in malfunctions. This realization makes it possible to take prompt action, like changing or adjusting components before they hit crucial failure points. Increasing a machine’s lifespan postpones the need for expensive replacements and saves money on repairs.
Additionally, it can guarantee that a business gets the most out of its equipment, which lowers the total amount of capital expenditure. Maintenance teams can make well-informed decisions to prolong a machine’s life by using the data-driven survival analysis approach, which gives them a precise understanding of the machine’s condition.
Saving Money on Maintenance
Survival analysis optimizes the timing of interventions, which lowers maintenance costs. Even when the machine is still operating properly, traditional maintenance techniques like time-based preventive maintenance frequently lead to needless part replacements or maintenance work. Businesses use survival analysis to transition from a scheduled or reactive approach to a predictive model in which maintenance is only carried out when required.
It lowers the frequency of expensive emergency repairs and prevents resource waste on unnecessary interventions by spotting early warning signs and anticipating failures. This focused strategy minimizes labor expenses and downtime by ensuring that parts are replaced at the most economical moment.
Reducing Downtime
survival analysis helps businesses plan maintenance tasks for planned breaks or off-peak hours, reducing downtime. Instead of waiting for an unplanned breakdown to interrupt operations, maintenance teams can replace or repair parts in advance when they know when a machine is likely to fail.
This proactive maintenance strategy guarantees seamless, continuous operations, boosts productivity, and reduces unscheduled downtime. In the end, survival analysis-driven predictive maintenance increases operational effectiveness and lessens the effect of interruptions on overall company performance.
Improved Compliance and Safety
Workplace safety is also influenced by real-time monitoring. Through constant monitoring of machinery and environmental factors, producers are able to identify risks before they endanger employees. Furthermore, data analysis lowers the danger of legal or regulatory problems by ensuring that activities meet necessary standards and maintaining compliance with safety rules.
Increasing Safety Requirements
Environmental variables including temperature, pressure, and air quality are tracked by real-time monitoring systems, which aid in spotting any risks before they have an adverse effect on worker safety. Manufacturers may satisfy compliance standards and promote employee well-being by promptly implementing remedial steps to guarantee a safe working environment with real-time warnings.
What are the Common Challenges in Applying Survival Analysis in Real-World Applications?
Ensuring Data Quality and Accuracy
Assuring complete and high-quality data is one of the biggest obstacles to using survival analysis for actual machine maintenance. Historical performance information, including machine usage, failure incidents, and maintenance logs, is crucial to survival analysis. Incomplete, erroneous, or inconsistent data can greatly distort the analysis’s findings and produce incorrect predictions. In real-world applications, equipment may run in a variety of settings with varying conditions, and not all maintenance procedures or failures may be accurately recorded.
Integration with Existing Systems
Integrating survival analysis with current machine monitoring and maintenance systems presents another difficulty. Predictive maintenance platforms, asset management software, and enterprise resource planning (ERP) systems are already used by many businesses. The intricate statistical models needed for survival analysis may be too much for these systems to handle. It can be challenging and resource-intensive to integrate survival analysis models into these current workflows, necessitating a high level of technical expertise
Resistance to Adoption and Change
Organizational resistance may result from survival analysis’s departure from conventional maintenance procedures. Many maintenance teams are used to reactive repairs or time-based, scheduled maintenance. A cultural and operational change is necessary to implement survival analysis, and stakeholders must accept and trust data-driven decision-making. This entails overcoming reluctance to adopt new technologies and altering ingrained maintenance practices for a lot of businesses.
Datategy Magazine: AI Industrialization & Future Challenges
Datategy Magazine serves as your premier source for in-depth data insights, offering comprehensive coverage of the latest trends, strategies, and developments in the data industry. With a focus on AI industrialization and future challenges, we provide valuable analysis and expert commentary to help you stay ahead in this rapidly evolving landscape.
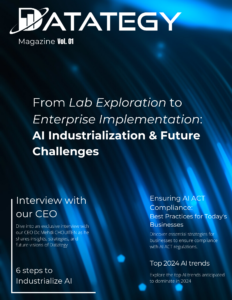
Use Case: How papAI Revolutionizes Failure Prevention and Machine Lifetime Forecasting with Survival Analysis
1- Data Import and Integration
Raw Data Collection
Every predictive maintenance journey begins with data. Sensor data from machines, often stored in databases, forms the backbone of the analysis.
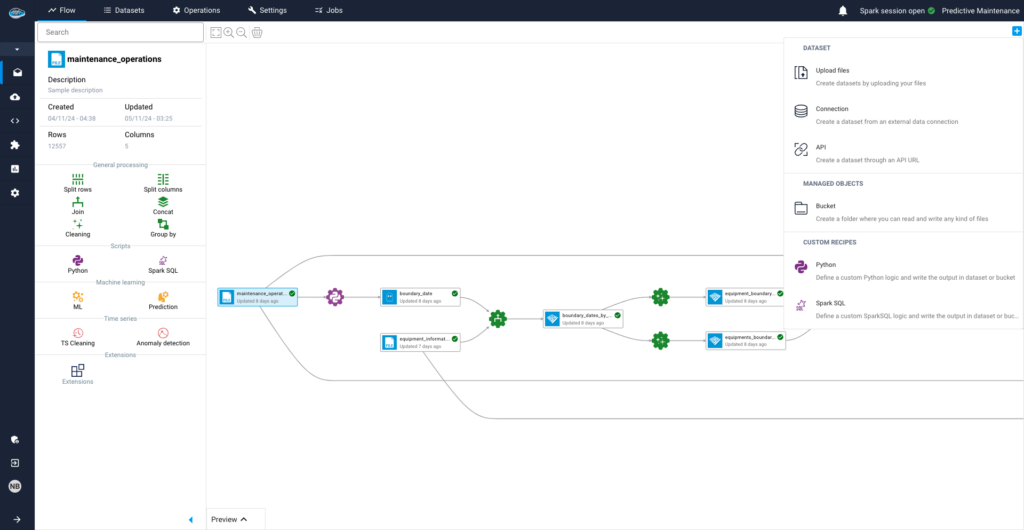
Seamless Connectivity with papAI
papAI’s integrated connectors simplify the data import process. In just a few clicks, raw data is imported, automatically processed, and ready for analysis, eliminating time-consuming manual configurations.
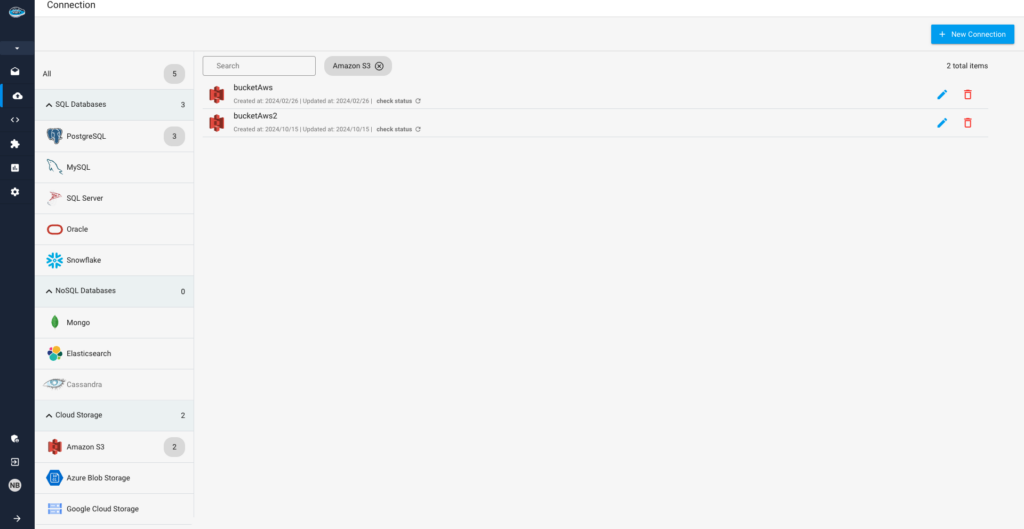
2- Data Cleansing: The Foundation of Accurate Models
Raw data is rarely perfect. It often contains missing values, errors, or anomalies that can degrade model performance. Proper data cleansing ensures models are accurate and unbiased.
Data Cleansing: The Foundation of Accurate Models
- Join Operations: Combine datasets from various sources seamlessly, regardless of their original structure.
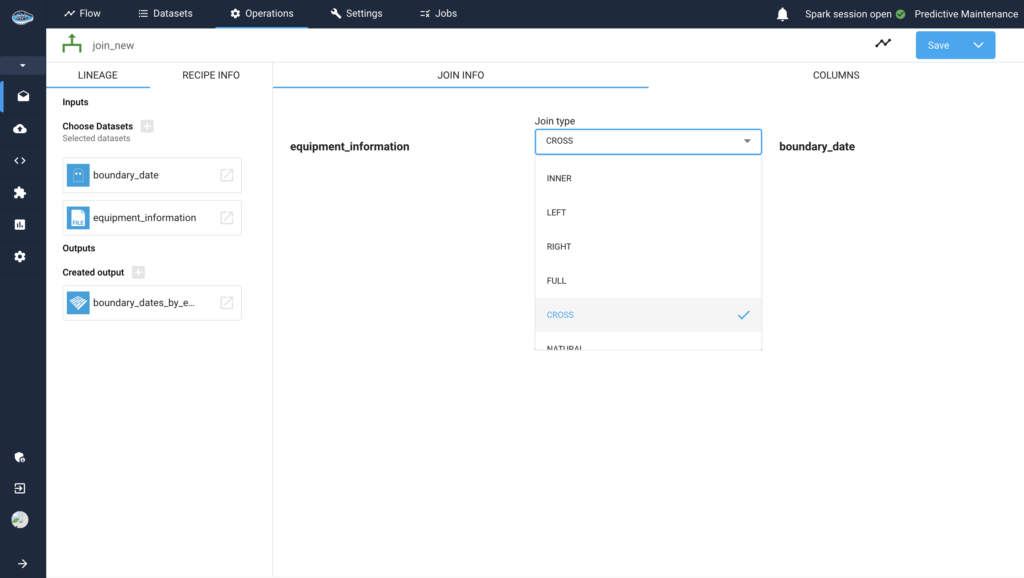
- Scripting Capabilities: Use Python or SparkSQL for custom data transformations. For instance, a SparkSQL recipe in papAI generates a comprehensive list of maintenance events, helping isolate critical data.
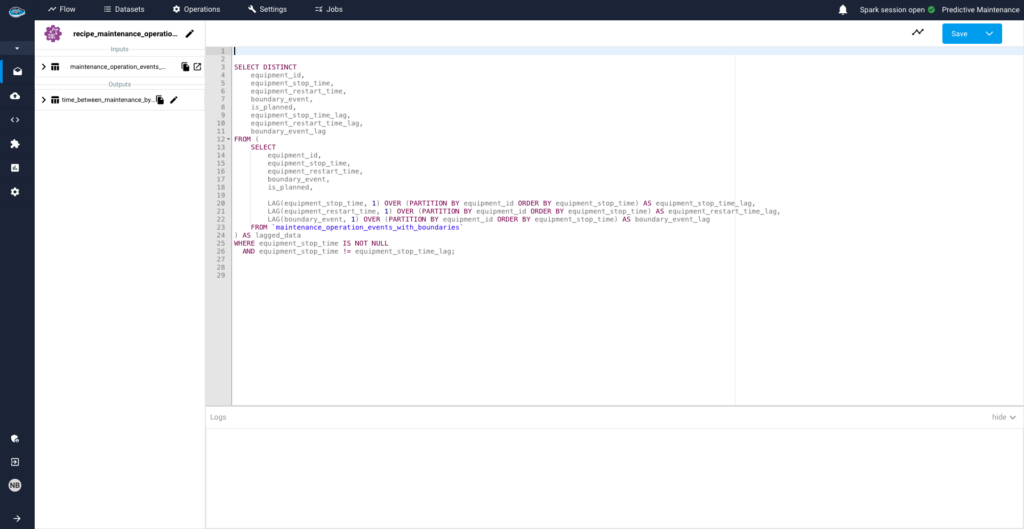
- Targeted Cleaning: With papAI’s Formula Edition, unexpected downtimes can be isolated by excluding planned maintenance. This defines precise targets for survival analysis.
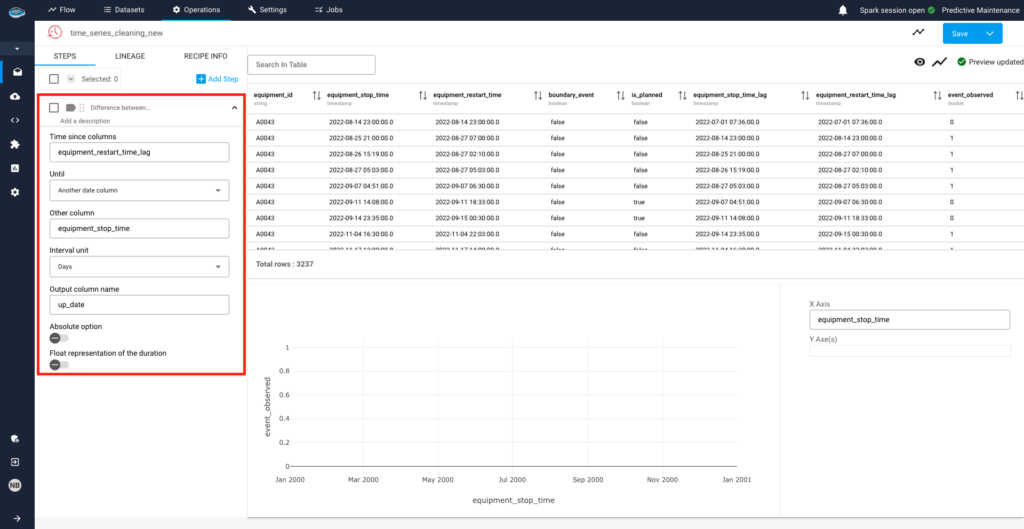
- Time Series Cleaning: By including the “up_date” variable, which tracks machine operation since the last maintenance, papAI enhances survival model predictions of remaining operational time.
3- Extending the Experience: Interactive Applications
- From Scripts to User-Friendly Applications
papAI transforms complex maintenance scripts into interactive dashboards, tailored to specific projects. These dashboards offer easy access to insights for all team members.
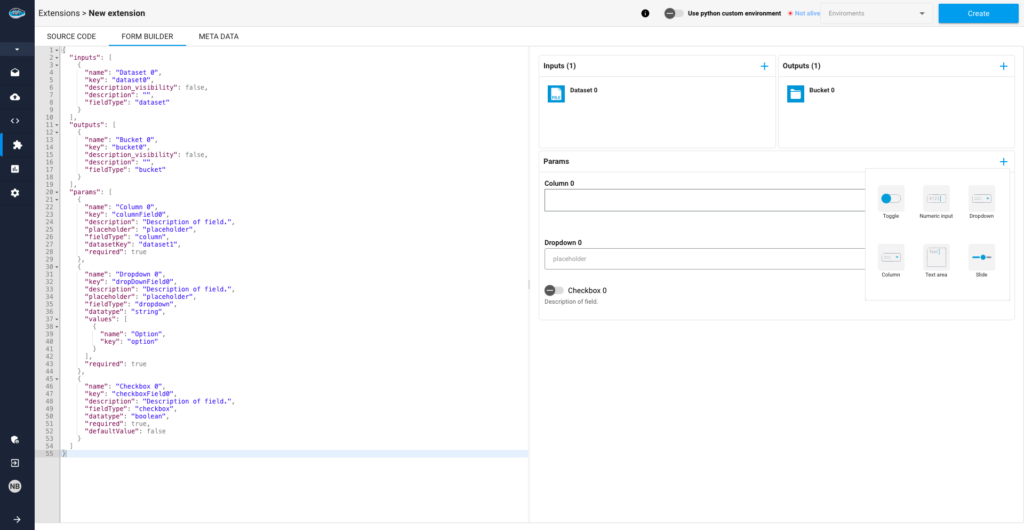
- Automation and Accessibility
Collaborators can share these tools, ensuring automated workflows and streamlined decision-making.
4- Survival Analysis for Machine Life Prediction
- The Survival Model Explained
Survival models estimate the likelihood of a machine’s operation over time. By leveraging historical data, these models predict the remaining life of equipment and assess failure risks.
- Predictive Maintenance in Action
Combining sensor data (e.g., vibration, temperature) with survival models, papAI calculates the probability of machine failure over varying timeframes. This enables maintenance managers to identify at-risk machines and prioritize interventions.
- Kaplan-Meier Chart
Visualize machine survival probabilities with a Kaplan-Meier chart. This intuitive tool highlights critical periods when machines are more prone to failure.
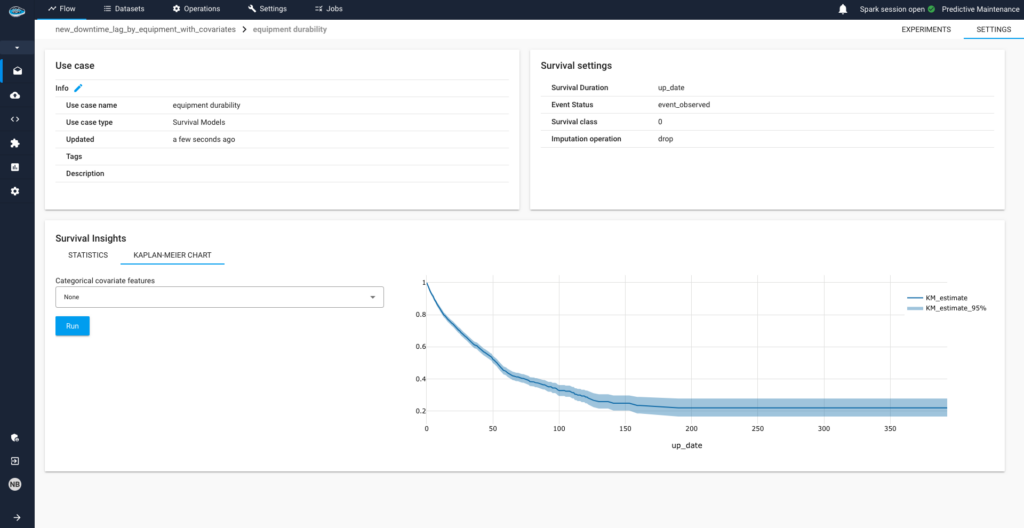
- Characteristic Coefficients
Survival models in papAI include coefficients that reveal the impact of variables like vibration or temperature. As new data is collected, these coefficients are refined, continuously improving model accuracy.
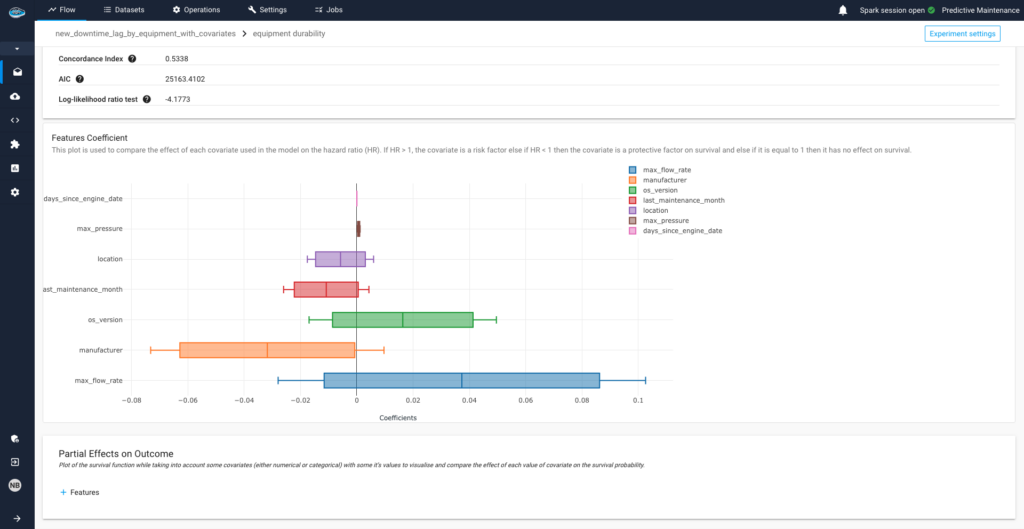
Classification of Threshold Events: Preemptive Warning Signals
- Understanding Threshold Events
Sensor-detected anomalies, or threshold events, indicate machines operating at their safety limits. These events are early warning signs of potential failures.
- Event Classification and Response
papAI uses classification algorithms to categorize these events by criticality. Maintenance teams can then prioritize responses, focusing on high-risk machines while monitoring minor anomalies.
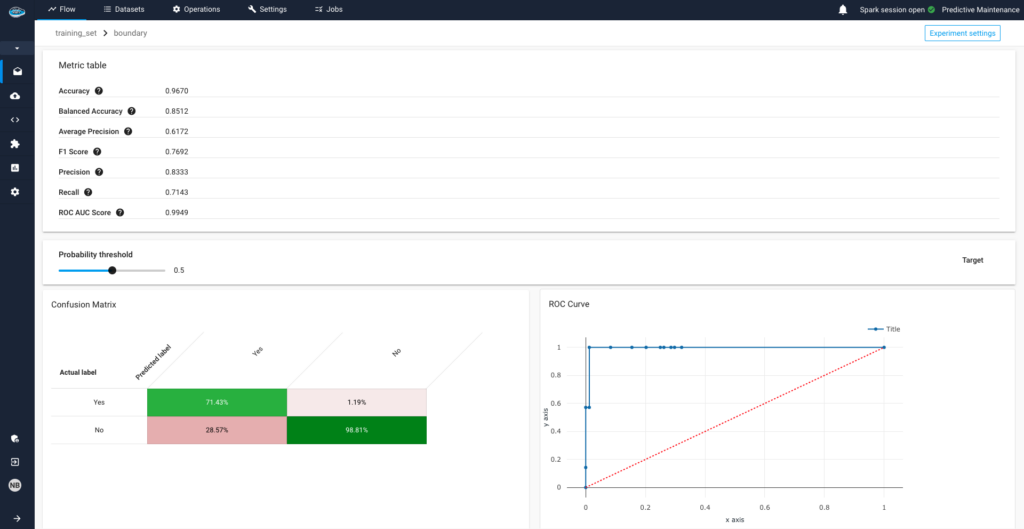
- Explainability and Counterfactuals
papAI’s explainability features (e.g., SHAP values) reveal why an event is critical, increasing team confidence. Counterfactuals simulate alternative scenarios, showing how changes in conditions (like reduced vibration) might lower failure risk. This helps teams take targeted corrective actions.
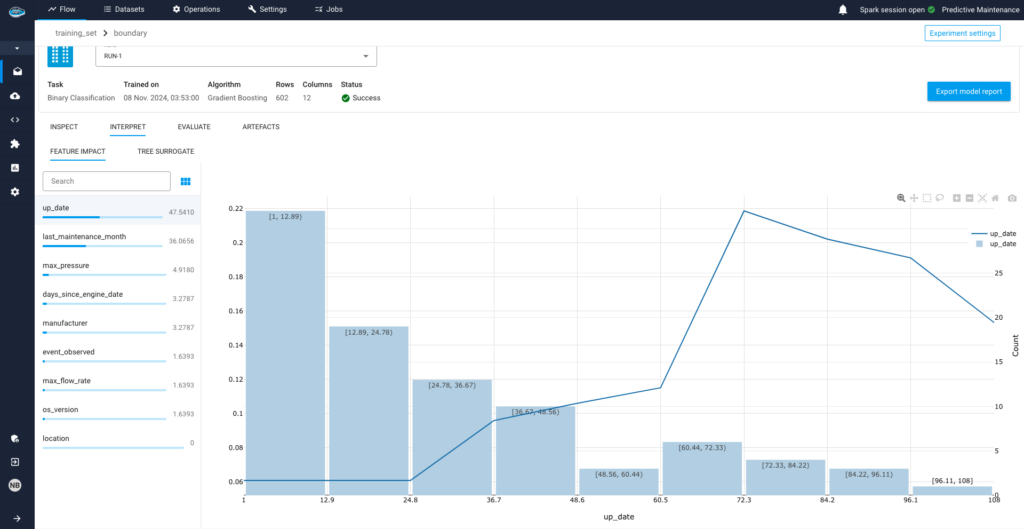
Create Your Own Failure Prevention Model using papAI solution
AI is revolutionising traditional maintenance techniques by using sophisticated algorithms and data processing to forecast when equipment is likely to break. AI helps industries transition from reactive to predictive maintenance strategies by evaluating both historical and real-time machine data. AI is a vital tool for industries looking to increase productivity and dependability because it reduces downtime, streamlines operations, and prolongs the lifespan of machinery.
In order to predict potential machine failures, AI-driven predictive maintenance analyses enormous volumes of sensor and operational data. AI helps industries schedule timely maintenance during planned downtimes and prevent unplanned breakdowns by generating hazard rates and identifying patterns. In addition to cutting expenses, this proactive strategy improves machine performance, operational safety, and compliance, guaranteeing maximum uptime.
- Data Quality: Incomplete, inaccurate, or inconsistent data can undermine AI model predictions, making thorough data collection and cleansing essential.
- System Integration: Organizations often face difficulties incorporating AI models into existing enterprise systems and workflows for seamless operation.
- Adoption Resistance: Transitioning to AI-based solutions requires a cultural shift, stakeholder buy-in, and trust in AI-generated insights.
Interested in discovering papAI?
Our AI expert team is at your disposal for any questions
How AI Transforms Production Lines into Smart Manufacturing Systems
How AI Transforms Production Lines into Smart Manufacturing Systems AI...
Read MoreHow Artificial Intelligence Simplifies Spare Part Searches
How Artificial Intelligence Simplifies Spare Part Searches Artificial intelligence is...
Read MoreLife at Datategy : Miloud Damene
Life at Datategy : Miloud Damene Hello everyone, my name...
Read More