How Law Firms Use RAG to Boost Legal Research RAG...
Read MoreHow Agentic AI is Transforming Logistics and Supply Chain Management?
Table of Contents
ToggleAI is developing beyond automation to create intelligent, self-governing agents that have the potential to completely transform logistics and supply chains. Businesses can now handle difficult operational challenges with previously unheard-of efficiency and flexibility thanks to agentic AI.
Agentic AI offers a new era of intelligent automation, unlocking the potential to optimize supply chains and logistics operations. These advanced AI systems can proactively respond to disruptions, improve forecasting accuracy, and enhance overall supply chain visibility
According to a recent Grand View Research survey, the global AI in the supply chain market is expected to grow at a compound annual growth rate (CAGR) of 38.8% from 2024 to 2030, reaching $41.23 billion.
Find out how papAI can improve the deployment of AI projects in the Retail sector here.
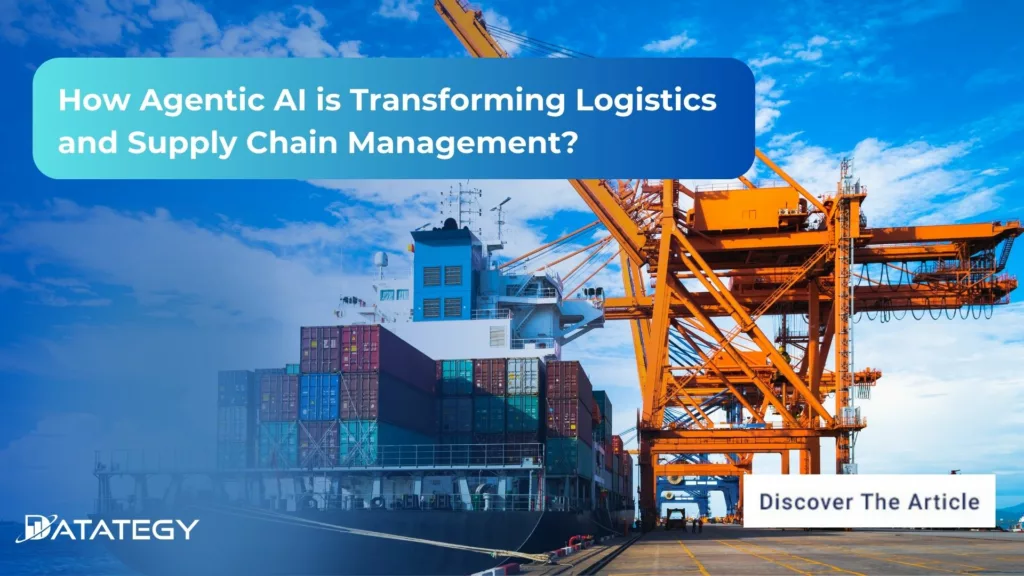
What is Agentic AI?
Agentic AI refers to artificial intelligence systems that can work on their own. They have a level of “agency” that allows them to make decisions and take actions. These actions are based on set goals or situations, and they do not need constant help from humans.
Agentic AI can look at its surroundings, consider different options, and make quick decisions. This is different from traditional AI, which often relies on fixed programming or simple reactions. Agentic AI aims to complete complex tasks in a more flexible and independent way. It uses machine learning, data analysis, and decision-making algorithms to respond to changes effectively.
Because of their independence, agentic AI systems can function effectively in dynamic settings like logistics, where schedules, routes, and resource availability can change suddenly.
What are the different Types of Agentic AI?
We have identified several types of agentic AI, broken down by their unique characteristics and functions:
Basic Reflex Agents:
- Function according to “condition-action” rules.
- Without taking into account past events or potential consequences, respond directly to current environmental inputs.
- Perfect for basic sensor-driven operations and other repetitive, simple tasks that need quick responses.
Reflex Agents Based on Models:
- Create a model of the world to monitor environmental elements that cannot be seen.
- Make better decisions by using this model, which will enable you to deal with changing circumstances more effectively.
- Ideal for applications where tasks are still quite simple but context is crucial.
Goal-Based Agents:
- Strive to accomplish particular objectives, allowing them to make plans that go beyond quick fixes.
- Determine whether various courses of action or pathways bring you closer to the intended result.
- Helpful in situations like route optimization where the agent needs to aim for a specific outcome.
Utility-Based Agents:
- Maximize a utility function that reflects performance or satisfaction in order to make more informed decisions.
- Able to select actions that provide the greatest value or benefit by weighing various outcomes.
- Perfect for complicated decisions, particularly those involving trade-offs, such as resource allocation.
Learning Agents:
- Continuously enhance their performance by taking lessons from previous decisions and results.
- Utilise methods like machine learning to gradually modify and improve your decision-making.
- Advantageous in dynamic settings with shifting conditions, such as logistics demand forecasting.
Multi-Agent Systems (MAS):
- Consists of several agents operating in the same environment, either cooperatively or competitively.
- Can work better as a team than as a solo agent at delegating, negotiating, and problem-solving.
- Frequently utilized in distributed systems, such as supply chain management networks.
Hierarchical Agents:
- Layered structure with higher-level agents supervising and directing lower-level agents.
- Give complex systems structure so that the actions of each agent are in line with the overall objectives.
- Efficient in complex, large-scale settings such as automated warehouse operations.
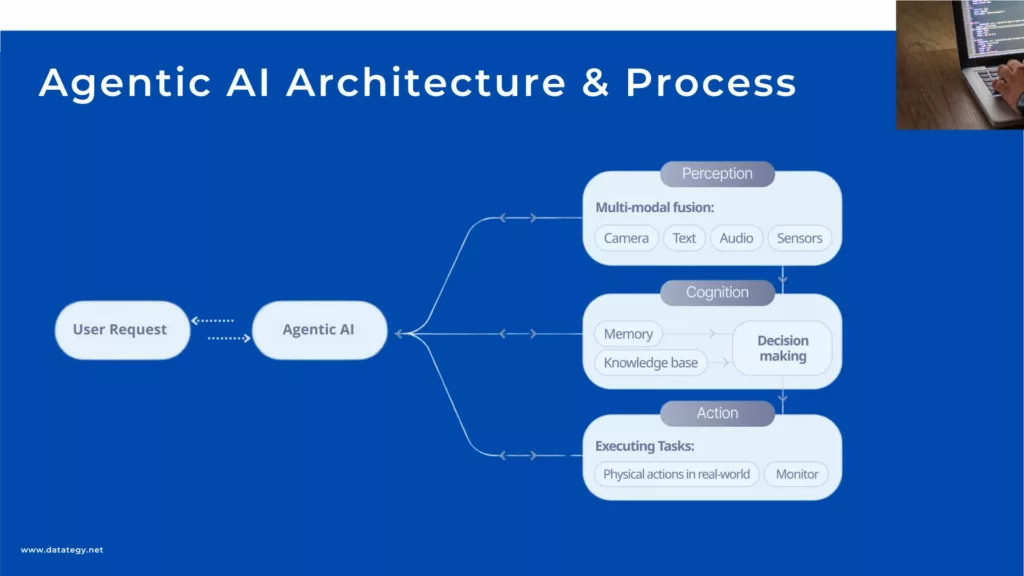
Agentic AI Architecture & Process
What are the Advantages of Agentic AI in Modern Logistics
Increased Effectiveness and Financial Savings
Given the potentially narrow margins in logistics, efficiency and cost-effectiveness are critical. From inventory management to route planning, agentic AI streamlines a variety of procedures by learning and responding to operational demands. In order to reduce needless fuel consumption and labour hours, it can, for instance, dynamically allocate resources based on current demand or predict the most fuel-efficient routes. This results in substantial cost savings, which streamline logistics operations and enable businesses to either reinvest in expanding their services or pass savings on to clients.
Increased Predictive Precision
Agentic AI provides a high degree of accuracy in forecasting future demand and inventory requirements by examining historical data trends and contrasting them with current information. It’s particularly helpful for forecasting abrupt changes and planning for seasonal demand. Because of this predictive accuracy, businesses can better anticipate demand changes without running the risk of stockouts or overstocking. By optimizing stock levels and lowering the need for costly last-minute shipments or storage overflow expenses, it assists logistics companies in maintaining a smooth flow of goods.
Capacity to Adjust to Changing Situations
Logistics is rarely predictable because so many outside factors affect day-to-day operations. Agentic AI is excellent at responding to changes as they occur, whether it’s reallocating resources in the event of a warehouse disruption, rerouting due to road closures, or modifying delivery schedules based on customer preferences. Businesses can maintain operations even in unexpected situations thanks to this flexibility, which greatly lessens the impact of delays and improves overall service reliability.
Decreased Need for Human Intervention
Agentic AI frees up workers for more strategic roles by managing predictable or repetitive tasks on its own, eliminating the need for continual human supervision. This reduces the possibility of human error in routine tasks, such as updating schedules or tracking shipments, while simultaneously increasing productivity. Without sacrificing accuracy, employees can concentrate on strategic planning and complex problem-solving, enabling logistics operations to be more customer-focused and flexible.
Flexibility and Scalability
Because of its great scalability, agentic AI is an effective tool for logistics firms that deal with varying demands or rapid expansion. Without requiring significant reconfigurations, these systems can easily adjust to manage an increasing volume of deliveries, routes, or inventory. Agentic AI can adapt to new operational scales as businesses grow, handling bigger datasets and intricate workflows without compromising efficiency. This adaptability guarantees that logistics operations can expand alongside the company while retaining responsiveness and efficiency.
What are the Top Applications of Agentic AI in Logistics & Supply chain
There are several advantages for using Agentic AI in your organization:
Managing and Optimising Inventory
By evaluating data to forecast demand trends and maximize stock levels, agentic AI significantly contributes to the simplification of inventory management. Conventional inventory systems are based on past data and frequently result in stockouts or overstocking, both of which are expensive for companies. However because agentic AI analyses both historical and real-time data, it can predict demand more precisely.
In order to guarantee that the proper quantity of product is available when and where it is needed, it recognizes patterns, such as seasonal peaks, and modifies stock levels appropriately. Agentic AI helps reduce warehousing costs by minimizing overstock and out-of-stock scenarios. It also ensures that items are always available, which improves customer satisfaction. Additionally, it enables businesses to make more flexible choices and promptly adjust to changes in customer demands.
Predictive Maintenance for Equipment and Fleet
One crucial use of agentic AI in logistics is predictive maintenance, which helps businesses to proactively maintain their equipment and fleet, minimising costly downtime and unplanned malfunctions. Agentic AI uses sensor data and maintenance history to find trends that predict when a machine or car will break down.
The lifespan of priceless equipment is increased and operational disruption is reduced by scheduling maintenance before a breakdown happens. Predictive maintenance keeps machines and vehicles in top operating condition, reducing repair costs and preserving seamless operation in logistics, where on-time delivery is essential. Logistics companies can increase their productivity and dependability by preventing unscheduled downtime.
Enhance Demand Forecasting
Another agentic AI’s primary advantage is demand forecasting, which enables businesses to more precisely plan inventory and order management. Agentic AI can accurately forecast future demand by examining past sales data, market trends, and outside variables like seasonal events or changes in the economy.
Logistics and supply chain managers can plan for demand swings with this predictive capability, whether they’re ramping up for the holidays or responding to unforeseen shifts in the market. Demand forecasting also makes order management easier because businesses can adjust their inventory and resources to meet demand without putting too much strain on their logistics infrastructure. By reducing the possibility of shortages and overstock, this proactive strategy balances inventory levels and enhances customer service.
Build Process Automation for Warehouses
Agentic AI-driven automation is revolutionizing fulfillment procedures in contemporary warehouses. AI-powered robots and systems greatly speed up operations and eliminate the need for manual labor by performing tasks like sorting, picking, and packing. By making decisions on its own based on current demands, such as allocating resources during peak hours or prioritizing high-urgency orders, agentic AI goes beyond simple automation.
Additionally, it can monitor warehouse inventory levels, causing restocking or modifying the distribution of shelf space. Agentic AI helps warehouses run more efficiently by automating repetitive tasks and streamlining workflow, which lowers labor costs, minimizes human error, and speeds up order fulfillment overall. flexibility, companies can concentrate on expansion plans without having to worry about outgrowing their IT infrastructure.
What is the Biggest Challenge in Agentic AI implementation within your Organizations?
One of the biggest challenges companies face is using advanced AI solutions. They struggle to integrate agentic AI into their existing systems. Supply chain management and logistics systems have often been built as rule-based, modular structures. These systems manage separate tasks that are often isolated from each other. Many older systems do not have the flexibility or ability to work with agentic AI.
The real-time data processing and autonomous decision-making capabilities of agentic AI are frequently incompatible with these older systems, making integration a challenging undertaking that may call for significant customization, retrofitting, or, in certain situations, a total redesign. Furthermore, current systems might store data in disparate databases or in incompatible formats, resulting in data silos that reduce AI’s ability to provide a comprehensive picture of the operation.
Datategy Magazine: AI Industrialization & Future Challenges
Datategy Magazine serves as your premier source for in-depth data insights, offering comprehensive coverage of the latest trends, strategies, and developments in the data industry. With a focus on AI industrialization and future challenges, we provide valuable analysis and expert commentary to help you stay ahead in this rapidly evolving landscape.
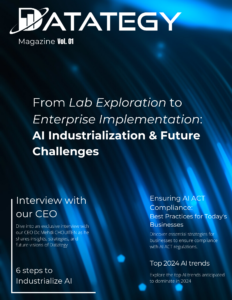
What are the Advantages to use papAI in that case?
Here’s an in-depth look at the key features and advantages of this innovative solution:
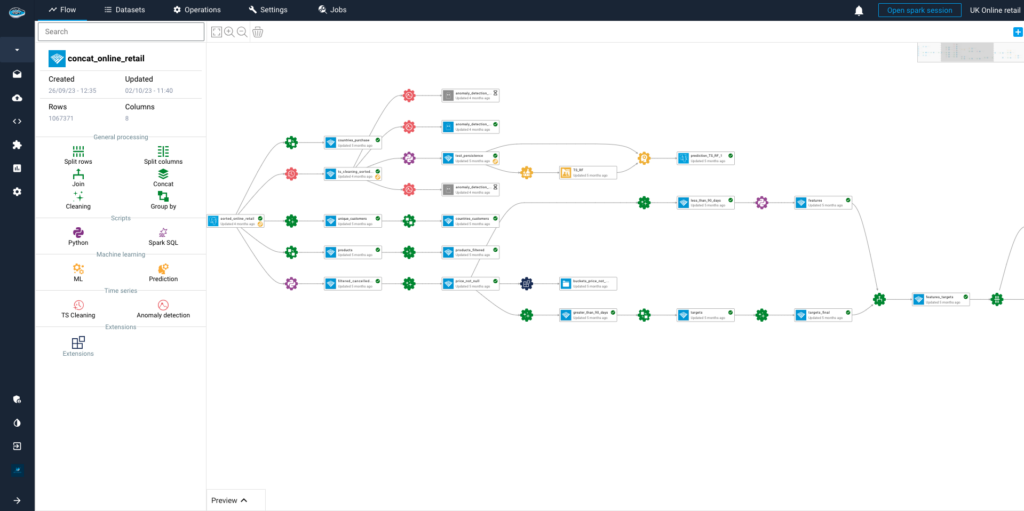
Seamless Integration with IoT Devices
Data collection and monitoring have been transformed by the incorporation of Internet of Things (IoT) devices into supply chain operations. papAI7 is made to easily interface with a variety of Internet of Things devices. This feature gives businesses real-time insights into their operations by enabling the monitoring of shipments, inventory levels, and equipment performance.
In order to prevent spoiling and guarantee adherence to safety regulations, temperature sensors in refrigerated transport, for instance, can transmit data to papAI7, which can notify managers if conditions diverge from acceptable ranges. PapAI7 increases the visibility and transparency of supply chain operations by utilizing the data produced by IoT devices. This allows businesses to react promptly to any interruptions and make well-informed decisions that increase productivity and decrease costs.
Robust API Support for Third-Party Tools
The strong application programming interface (API) support of papAI7 is one of its best qualities; it makes it simple to integrate with current software tools and systems. A wide range of specialized software is used by numerous supply chain organizations for order processing, inventory management, and transportation management.
A comprehensive understanding of supply chain operations is made possible by the seamless communication and data sharing between these systems made possible by papAI7’s API. Because companies can continue to use their current tools while utilizing the sophisticated capabilities of papAI7, this integration reduces disruption during the shift to AI-enhanced processes. In addition to improving interoperability, API support enables customization, allowing businesses to adapt the platform to their unique operational requirements and increase supply chain management effectiveness and efficiency.
Comprehensive Data Analytics and Visualization Tools
Supply chain managers can extract useful insights from complicated datasets with the help of papAI7’s advanced data analytics and visualization tools. The platform provides user-friendly dashboards that visually engage users by showcasing trends, operational metrics, and key performance indicators. This feature enables users to make well-informed decisions based on real-time data, swiftly evaluate the state of their supply chain, and pinpoint areas for improvement.
A logistics manager, for example, is able to identify delays or inefficiencies in particular regions by visualizing shipment performance across various regions. Deep dives into historical data are also made possible by the analytics capabilities, which aid organizations in comprehending past performance and implementing strategic changes for operations in the future.
Deploy your own Agentic AI using papAI solution
A future where your supply chain is proactive rather than reactive, foreseeing obstacles and opportunities before they materialize, is possible if you embrace agentic AI with papAI solution. Gaining a competitive edge, improving customer satisfaction, and promoting sustainable growth are all possible with the ability to analyze real-time data and make decisions on your own. Don’t pass up the chance to advance your company’s operations.
Is your company prepared to investigate the possibilities of agentic AI? Schedule a demo with our specialist right now to find out how papAI solution can create a smart plan tailored to your supply chain requirements. Let us assist you as you move towards a future that is more nimble and efficient.
Interested in discovering papAI?
Our AI expert team is at your disposal for any questions
How RAG Systems Improve Public Sector Management
How RAG Systems Improve Public Sector Management The most important...
Read MoreScaling RAG Systems in Financial Organizations
Scaling RAG Systems in Financial Organizations Artificial intelligence has emerged...
Read MoreHow AgenticAI is Transforming Sales and Marketing Strategies
How AgenticAI is Transforming Sales and Marketing Strategies Agentic AI...
Read More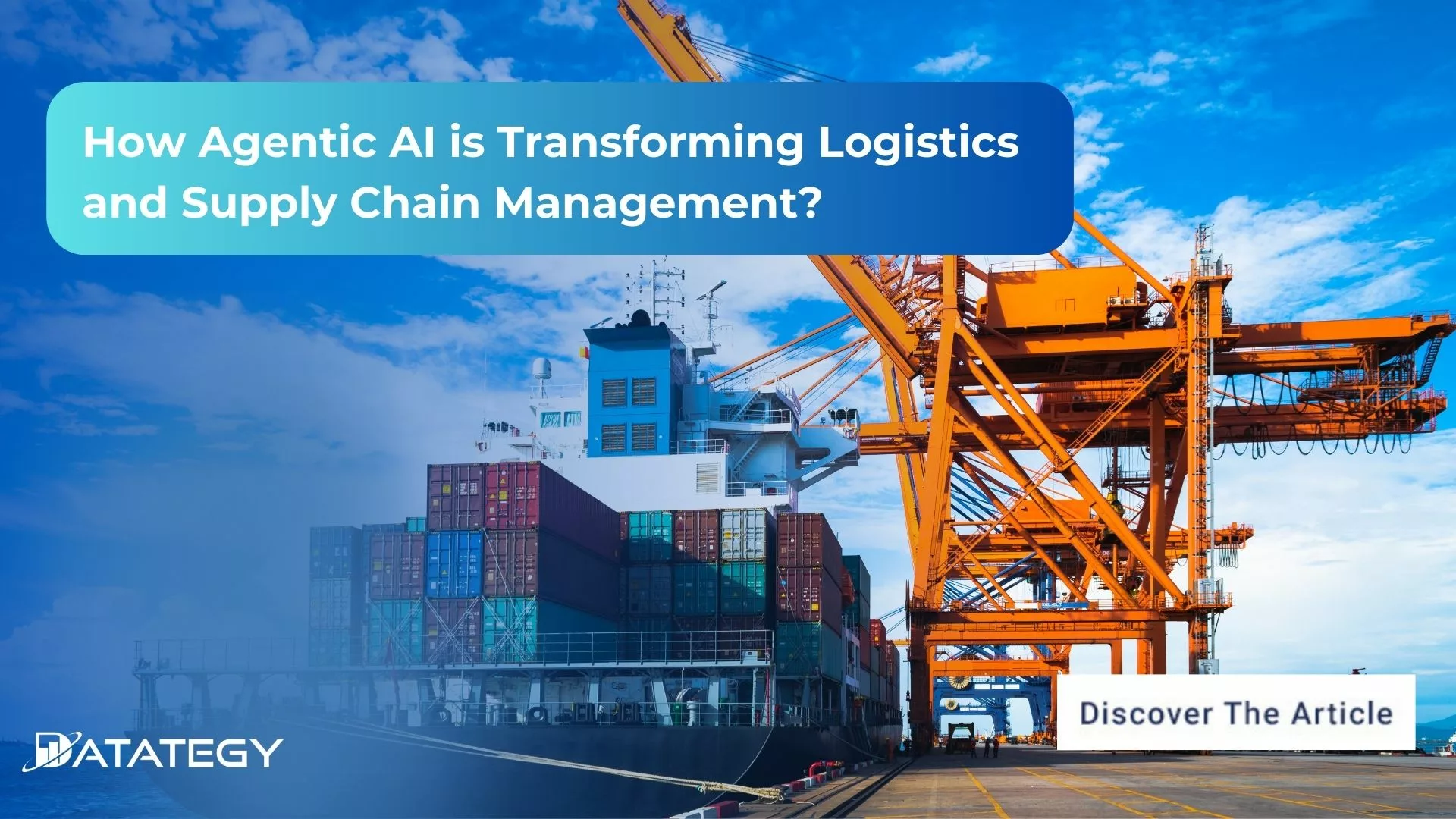
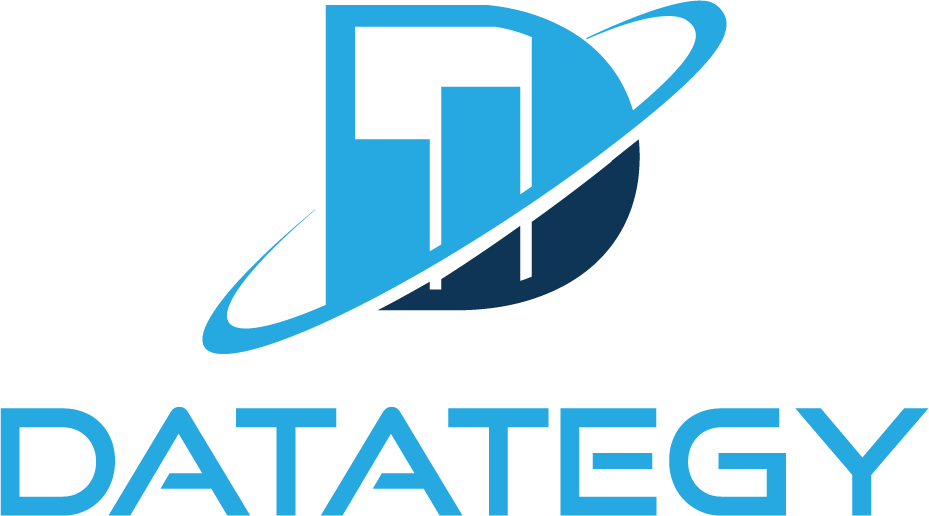