How does AI enhance and accelerate ESG Strategies? The first...
Read MoreTime Series Forecasting in Wind & Solar Grid Management
Table of Contents
ToggleNowadays, wind and solar energy are the most reliable and safe of the primary renewable energy sources. Consistent energy production is significantly hampered by their inherent variability. Wind and sunshine are inherently unpredictable, and weather patterns are subject to sudden changes. Here’s where artificial intelligence (AI) comes in, providing a revolutionary way to maximize and steady the production of renewable energy.
AI in renewable energy is projected to grow to $4.6 billion by 2032, with a yearly growth rate of 23.2% from 2023 to 2032.
Find out how papAI can improve the deployment of AI projects in the Renewable Energy Industry.
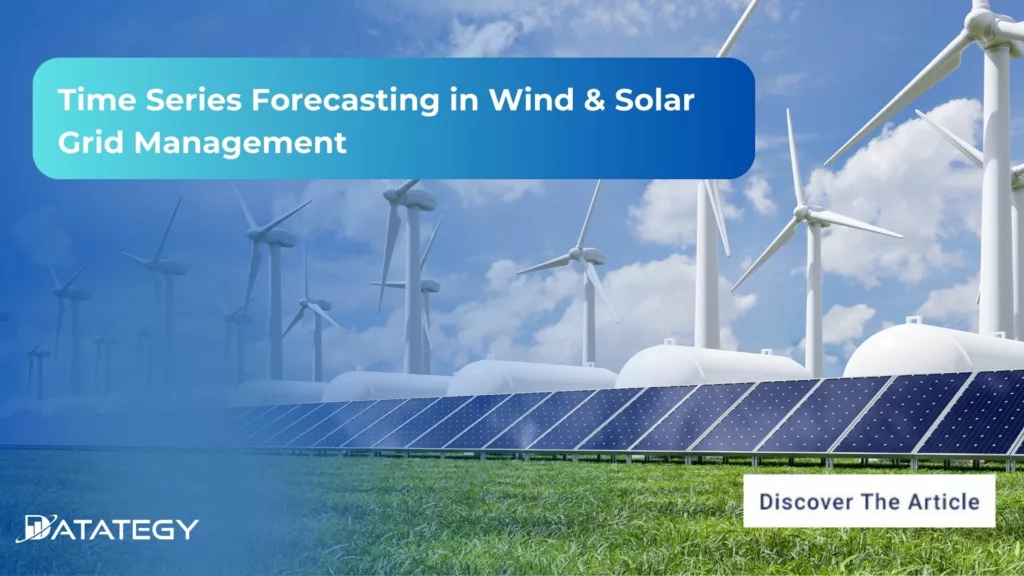
In this article, we define the role of time series in the field of renewable energy and their importance for improving productivity.
What is Time Series Forecasting?
Time series forecasting is a statistical technique that makes predictions about the future from previously observed data points that are usually gathered over time at regular intervals.
Time series forecasting is particularly concerned with predicting what will happen next, in contrast to other types of data analysis that might concentrate on comprehending relationships or trends within a dataset. This makes it especially useful in situations like stock market trends, weather patterns, or energy consumption prediction, where anticipating future behavior is essential.
Time series forecasting is fundamentally the analysis of patterns in the data, including trends, seasonal fluctuations, and cyclical movements. Models that are able to predict future values with a certain degree of accuracy are then developed using these patterns.
Techniques can be as basic as moving averages and exponential smoothing, or as sophisticated as machine learning algorithms and ARIMA (AutoRegressive Integrated Moving Average). The intricacy of the data and the particular needs of the forecast frequently influence the method choice.
What are The Benefits of using Time Series Forecasting in Renewable Grid Management?
Accurate Energy Demand and Supply Prediction:
Predicting energy supply and demand is essential for effective grid management, and time series forecasting is a potent tool for this task. Utility companies can develop models that precisely predict future energy needs by examining past patterns of energy consumption, including daily and seasonal variations. With the help of these forecasts, grid managers can plan for times when demand will be highest, like during heat waves or cold snaps.
Operators can control the output of power plants to ensure that there is always enough energy to meet demand without overproducing, which can be expensive and wasteful, by anticipating these peaks in output.
Proactive Maintenance and Risk Management:
Time series forecasting helps in risk management for unforeseen occurrences like storms and abrupt increases in energy consumption. By anticipating these occurrences and their possible effects on the grid, operators can take proactive steps like bolstering susceptible infrastructure or increasing backup power sources.
By taking a proactive stance, the probability of outages is decreased, and the financial and operational consequences of such incidents are also lessened. Grid operators can sustain a higher degree of service reliability and customer satisfaction by anticipating possible problems before they arise.
Integration of Renewable Energy Sources:
The inherent variability of renewable energy sources, such as solar and wind power, makes their integration into the grid a complex challenge. Renewable energy sources are unpredictable in terms of output because they rely on natural conditions, in contrast to traditional power sources. This problem is met by time series forecasting, which estimates future energy production from these sources by examining weather trends, past generation data, and other pertinent variables.
Grid operators, who have to make sure that the energy supply doesn’t change even when renewable output varies, need to be aware of this foresight.
What are the Pillars of Successful Time Series Forecasting Model?
Data Preparation and Quality: Well-prepared, high-quality data is the cornerstone of any successful time series forecasting model. In order to do this, pertinent historical data reflecting the patterns and trends of the variable being forecasted must be gathered. Steps in data preparation include dealing with missing values, fixing irregularities, and guaranteeing consistency. When data is prepared correctly, the model can learn from past trends and seasonal effects, producing forecasts that are more accurate.
Feature engineering: Developing and choosing pertinent features that capture underlying patterns is a common step in improving your time series model. This could entail figuring out and taking into account cyclic patterns, seasonal trends, and outside variables that affect the time series. Moreover, feature engineering entails converting unprocessed data into more illuminating forms, like lag variables or moving averages. These characteristics aid the model’s comprehension and forecasting of future values.
Constant Monitoring and Improvement: Time series forecasting is a continuous process rather than a one-time event. To guarantee the accuracy of the model over time, it is imperative to conduct ongoing performance monitoring. This entails periodically adding fresh data to the model, assessing its efficacy, and making the required corrections. You can preserve the model’s efficacy and relevance by making adjustments based on the most recent data and input, guaranteeing that your projections continue to be precise and useful.
From Data to Action: How AI is Transforming the Energy Industry
The integration of AI and ML technologies in the energy industry has the potential to revolutionize the way we produce, distribute, and consume energy. By leveraging advanced algorithms and data analytics, AI and ML can improve the efficiency, safety, and sustainability of energy systems. Find out more in this white paper.
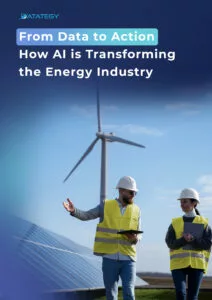
Use Case: Leveraging Time Series Forecasting for Wind & Solar Grid Power Production
Context
Renewable energy sources, like solar and wind power, have become increasingly important in the world energy scene in recent years. In order to maintain sustainability and efficiency in the energy market, the Commission de Régulation de l’Énergie (CRE) is essential in monitoring and controlling the French energy sector.
A comprehensive dataset comprising hourly records of wind and solar energy production (measured in megawatts, MW) for the French grid since 2020 has been gathered to support these efforts. This dataset is primarily intended to make it easier to calculate the reference price that is needed to determine additional compensation for the solar and wind energy industries. Through the analysis of this data, interested parties can anticipate future production and obtain insights into the patterns of renewable energy production,
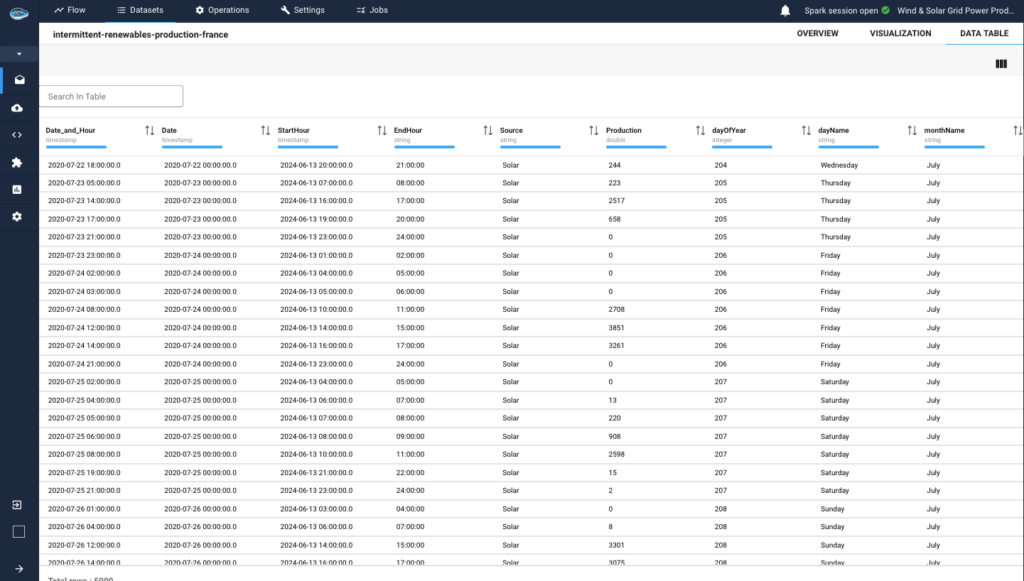
1- Data Exploration
papAI can compute automated statistics calculation, providing users with a detailed understanding of dataset characteristics. This feature computes various statistics for each variable. For numerical variables, it calculates the mean value, standard deviation, minimum value, maximum value, and other metrics.
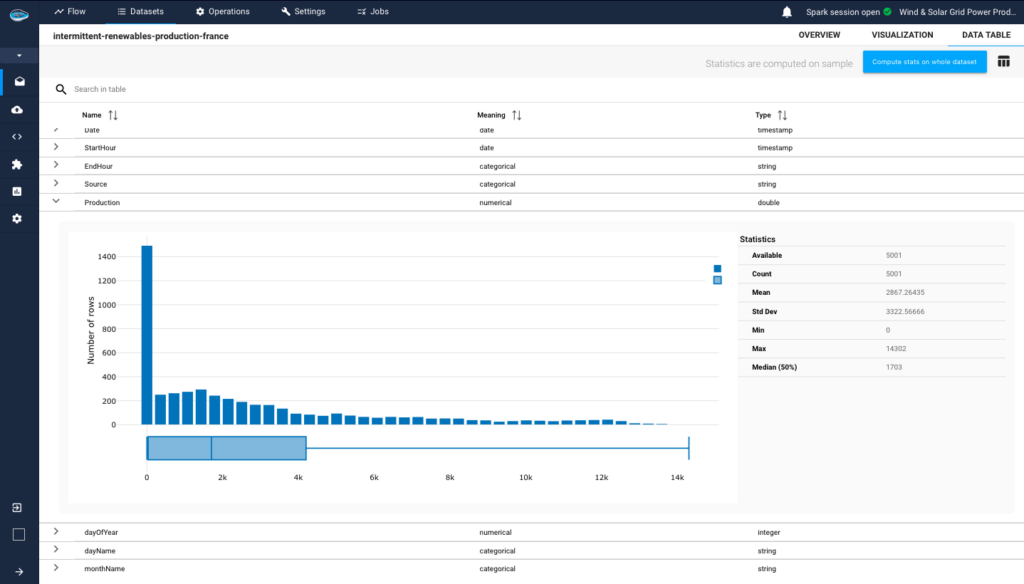
For categorical variables, it identifies distinct categories and their frequencies, offering insights into the distribution of categorical data.
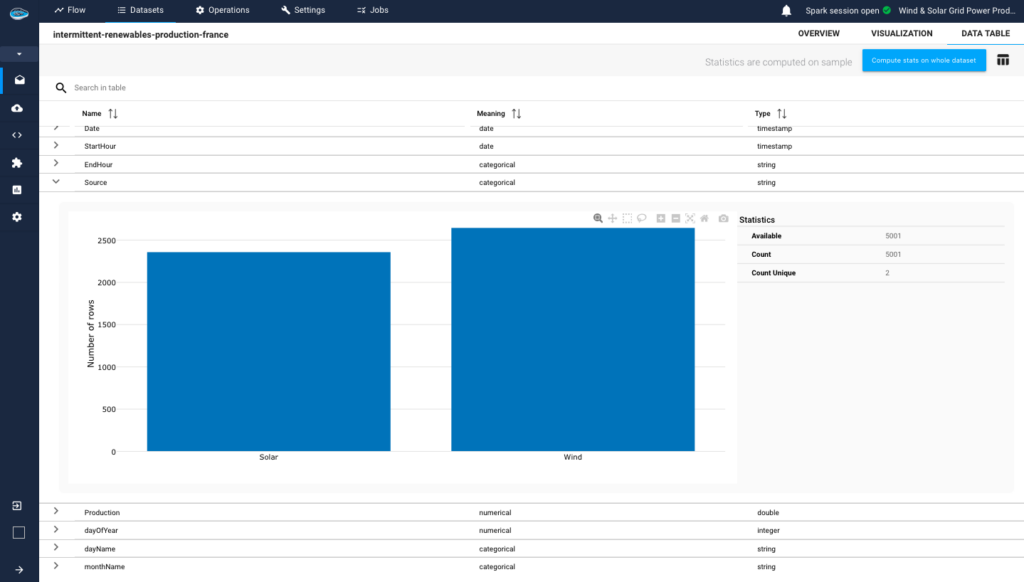
papAI facilitates comprehensive data visualization, making it easier to derive meaningful insights from complex datasets. Whether it’s understanding seasonal trends, comparing different energy sources, or analyzing temporal patterns, papAI provides the tools necessary for effective data analysis and decision-making.
Let’s analyze some visualizations to understand how papAI helps in data visualization and interpretation.
- Box Plot Analysis: Using papAI, we can compare the production of solar and wind energy. The box plot shows that wind energy production is generally higher and more variable than solar energy. This suggests that while wind energy has a greater potential for high output, it also experiences more fluctuations, which can impact energy planning and stability.
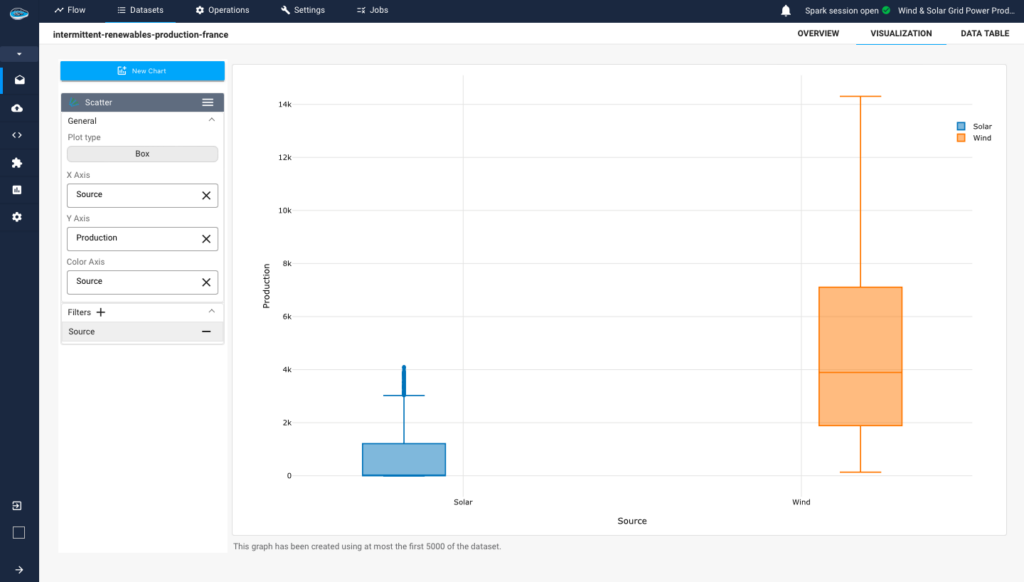
Pie Chart Analysis: Thanks to papAI, we can observe the monthly distribution of intermittent renewable energy production in France. The pie chart reveals that February has the highest production, followed by January, April, and May. This seasonal pattern indicates that specific months are more favorable for renewable energy generation, likely due to weather conditions.
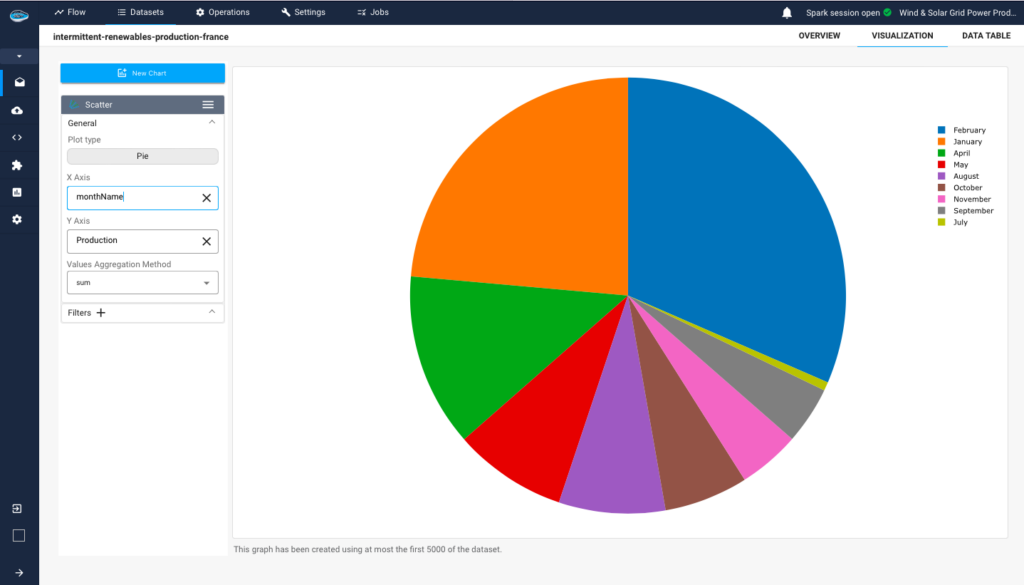
Line Chart Analysis: With papAI’s line chart, we can track the average renewable energy production over time. The chart shows significant peaks in early 2021 and noticeable drops around mid-2020. These variations could be attributed to seasonal changes, policy impacts, or other external factors influencing energy production.
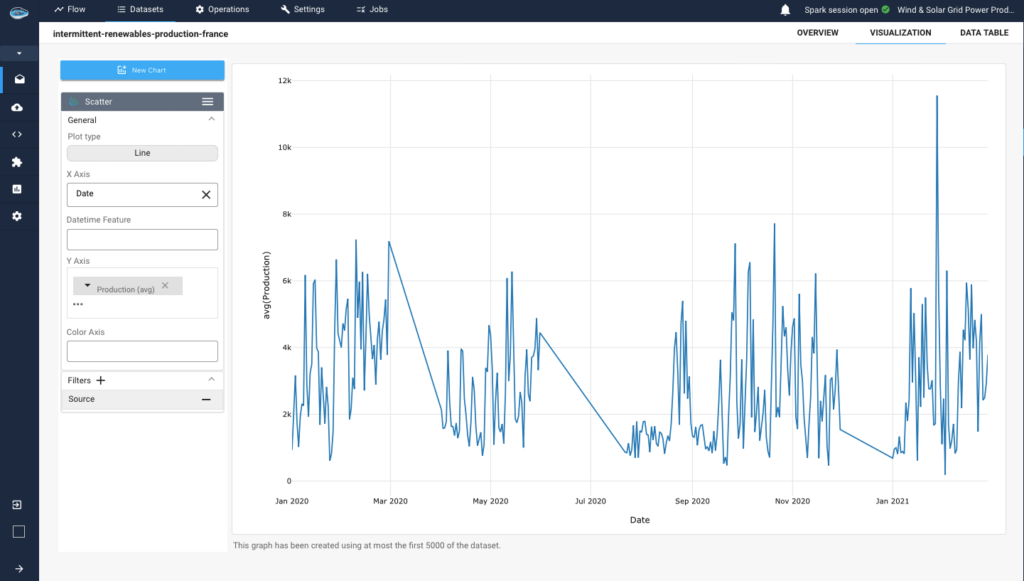
2- Preprocessing Data :
One of the important steps is preprocessing, where we clean our dataset to make it ready before building our model and starting the predictions. With the help of papAI, we can efficiently perform this preprocessing. For instance, we have a datetime column from which we need to extract specific time-related information. Using papAI, we can extract the hour from the datetime column, enabling us to analyze data based on specific times of the day. This extraction helps in capturing temporal patterns that could be crucial for our analysis and predictions
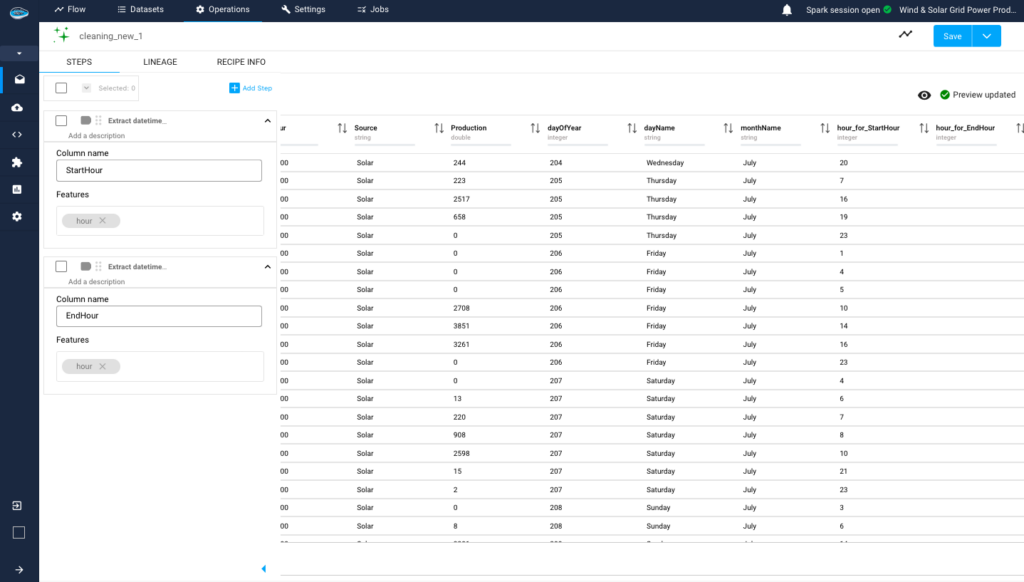
Another essential preprocessing task is the generation of new columns to enrich our dataset. With papAI, we can create additional columns such as day_of_year, month, and hour from a datetime column. By adding these columns, we can analyze seasonal trends, monthly variations, and hourly fluctuations in our data. This approach enhances our ability to identify patterns and relationships that might not be apparent with the original dataset
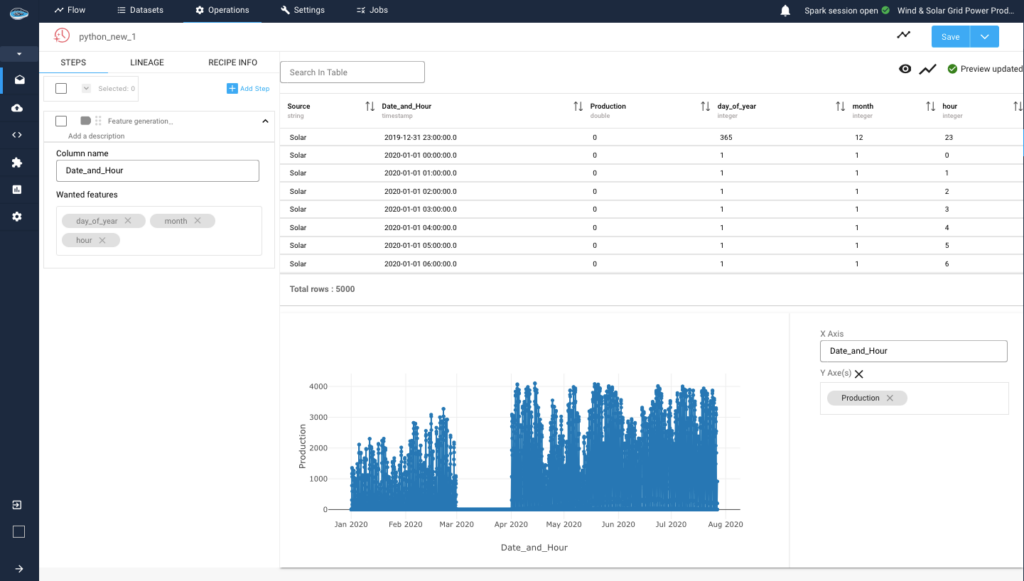
Handling missing data is a critical part of preprocessing. With the assistance of papAI, we can replace null values in our dataset with a constant value, such as 0. This method ensures that our dataset remains complete and consistent, without the introduction of random values that could distort our analysis and predictions. By using a constant like 0, we maintain the integrity of our dataset while effectively managing missing data points.
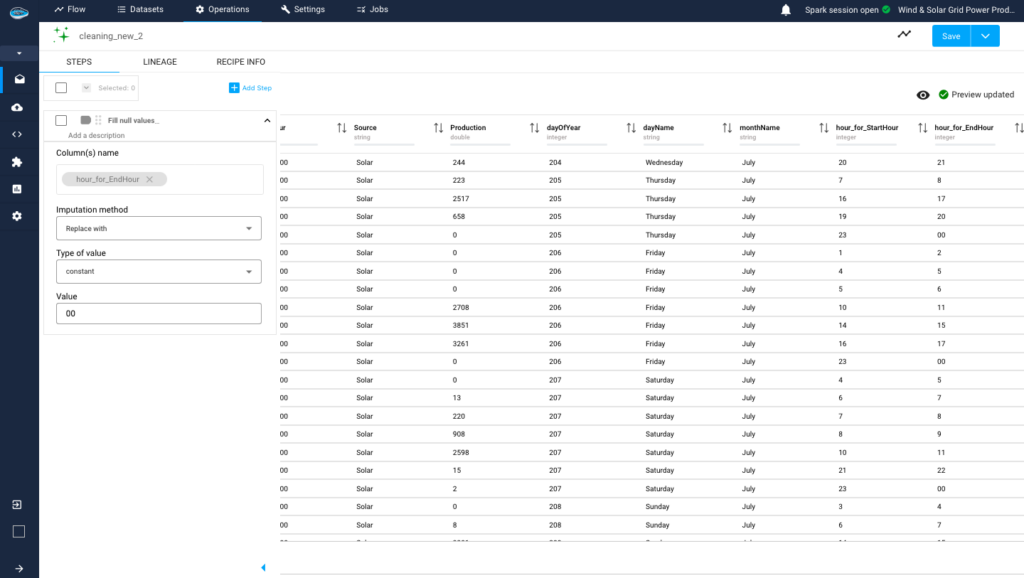
3- Building Model :
papAi offers all the necessary tools to proceed without needing to code the models. We simply need to select the ML task we want. In our case, since we want to predict the production over a period of time, the suitable approach would be to use TS Forecasting.
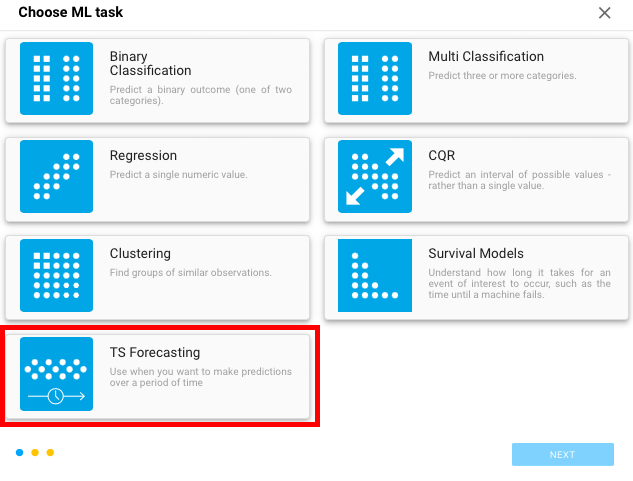
When launching a timeseries forecasting model, it is crucial to ensure the integrity of the timeseries data. With papAI, this process is streamlined and efficient. During the initial stages, papAI performs a comprehensive sanity check on the timeseries data. Here It identified that our timeseries data was not resampled and detected potential issues due to multiple timestamps being duplicated. To address this, papAI recommended resampling the data with a frequency of 1 hour. This recommendation helps to standardize the timeseries data, ensuring that each timestamp is unique and the data is evenly spaced. By following papAI’s suggestions, we can enhance the accuracy and reliability of our forecasting model.
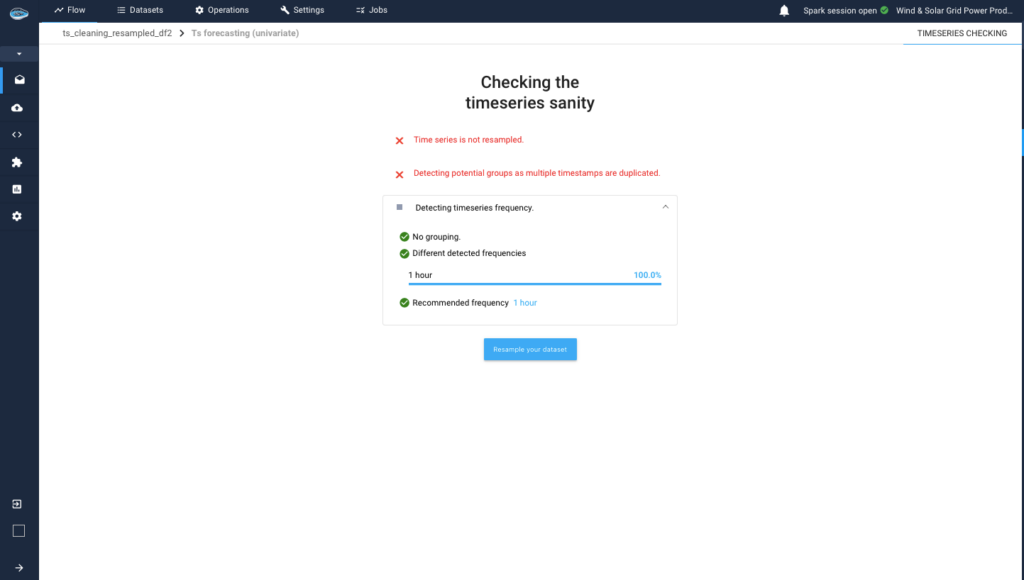
Using papAI, we resampled our timeseries data to have a consistent frequency of 1 hour. This process involved aggregating and filling data points to ensure uniform intervals. By resampling, we standardized the timeseries, eliminating duplicates and ensuring that each timestamp is unique. This step is essential for improving the accuracy and reliability of our forecasting model, providing a solid foundation for predictive analysis.
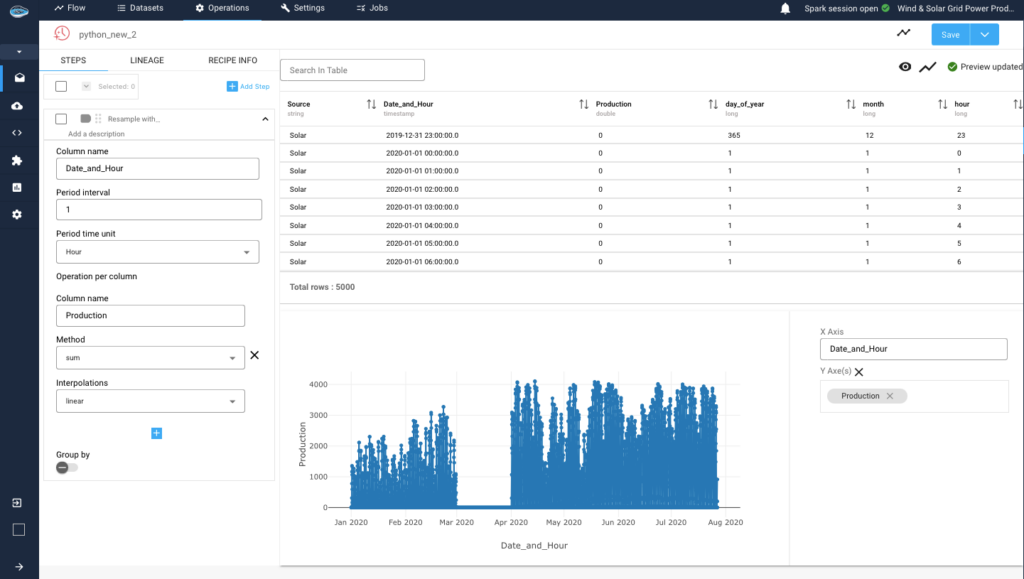
Next, we choose the algorithm or algorithms to use. papAi offers the most commonly used ones.
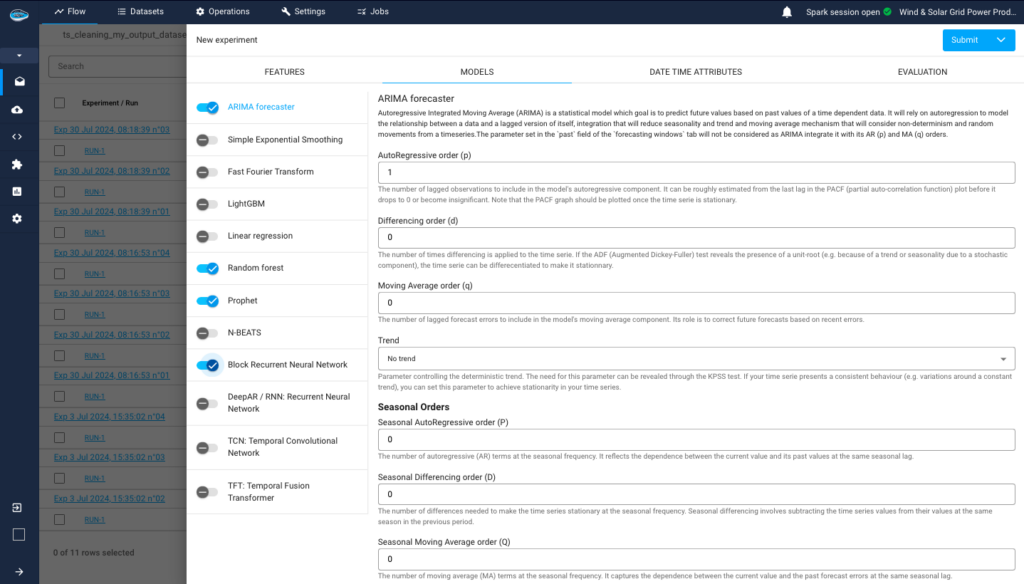
4- Model Evaluation and Interpretation :
After preprocessing the data and ensuring its integrity, We launched the forecasting model using Fast Fourier Transform (FFT) with the help of papAI. The model’s performance was evaluated using various metrics, yielding the following results:
- R² Score: 0.83
- Mean Absolute Error (MAE): 1.48
- Mean Squared Error (MSE): 8.32
- Mean Absolute Percentage Error (MAPE): -1%
- Symmetric Mean Absolute Percentage Error (sMAPE): 27.99%
These metrics provide a comprehensive understanding of the model’s accuracy and precision. An R² score of 0.83 indicates that the model explains 83% of the variance in the data, demonstrating a strong fit. The MAE of 1.48 and MSE of 8.32 show the average error and the variability of the error, respectively. The MAPE and sMAPE values further illustrate the model’s forecasting accuracy in percentage terms. With these insights, we can confidently move forward with the model for practical applications.
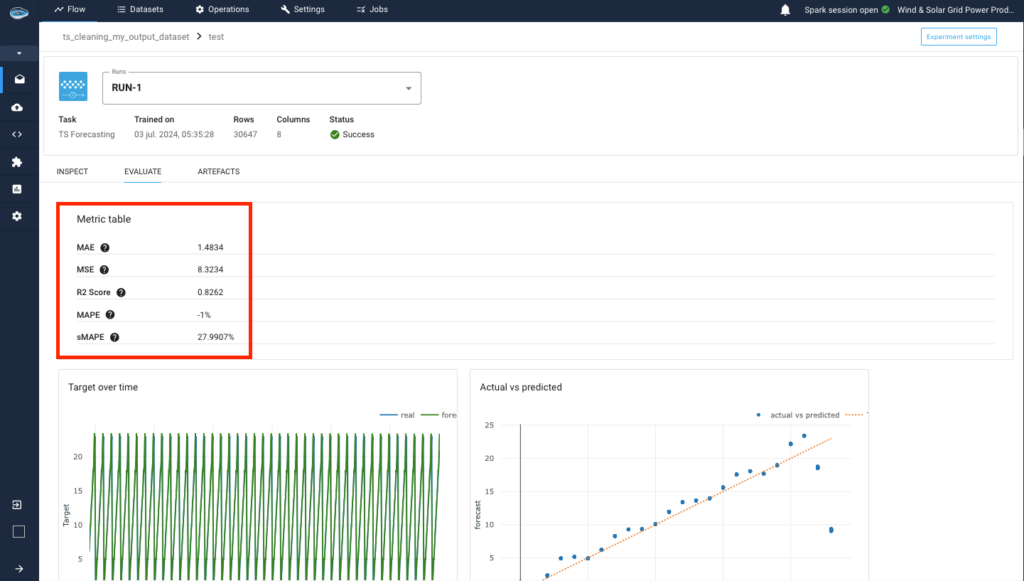
After evaluating the model’s performance, We visualized the results using a graph that compares the real values and the forecasted values, with a line representing the regression fit. This visualization provides a clear and intuitive understanding of how well the model predicts the actual data.
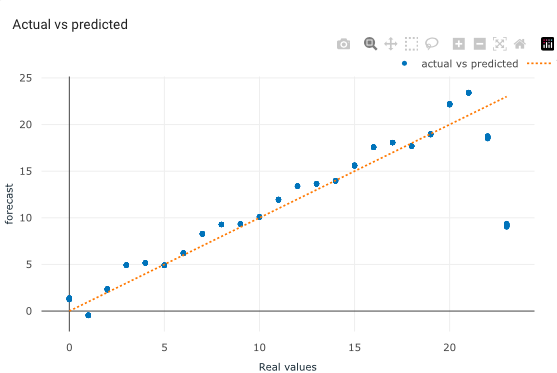
By using papAI, not only were we able to preprocess the data and build a robust forecasting model, but we also effectively visualized the model’s performance. This step is crucial in validating the accuracy of our predictions and communicating the results in a comprehensible manner.
Creating Your Own Time Series Forecasting Model using papAI
In summary, developing a time series forecasting model with papAI empowers you to leverage cutting-edge technology to make precise predictions and informed decisions. papAI is an advanced platform that simplifies the complexities of time series analysis, offering intuitive tools and robust features to enhance your forecasting capabilities.
Ready to see how papAI can transform your forecasting approach? Book a demo today to explore how our platform can meet your specific needs and drive your success.
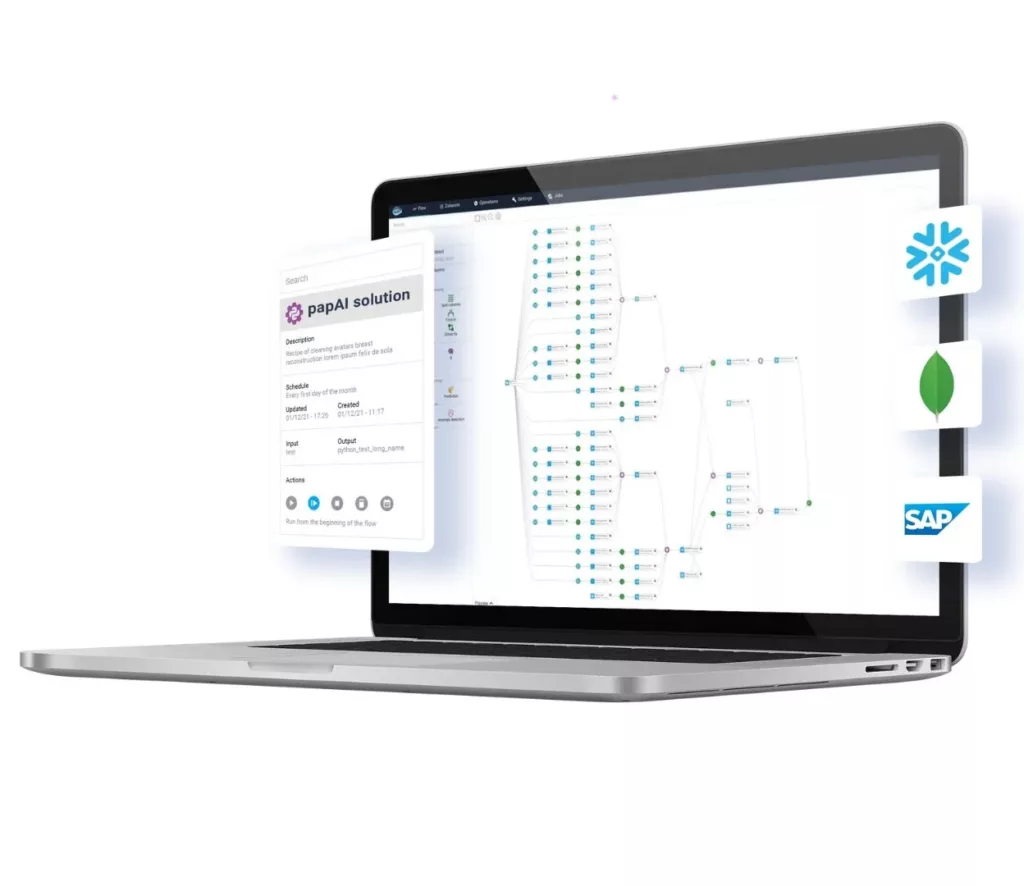
Interested in discovering papAI?
Our AI expert team is at your disposal for any questions
How Is AI Transforming Digital Twin in the Industry?
How Is AI Transforming Digital Twin in the Industry? Artificial...
Read More“DATATEGY EARLY CAREERS PROGRAM” With Julien Courteville
“DATATEGY EARLY CAREERS PROGRAM” With Julien Courteville Hello, my name...
Read MoreDatategy + Nedd Technologies: First Center of AI Excellence in USA
Datategy + Nedd Technologies: First Center of AI Excellence in...
Read More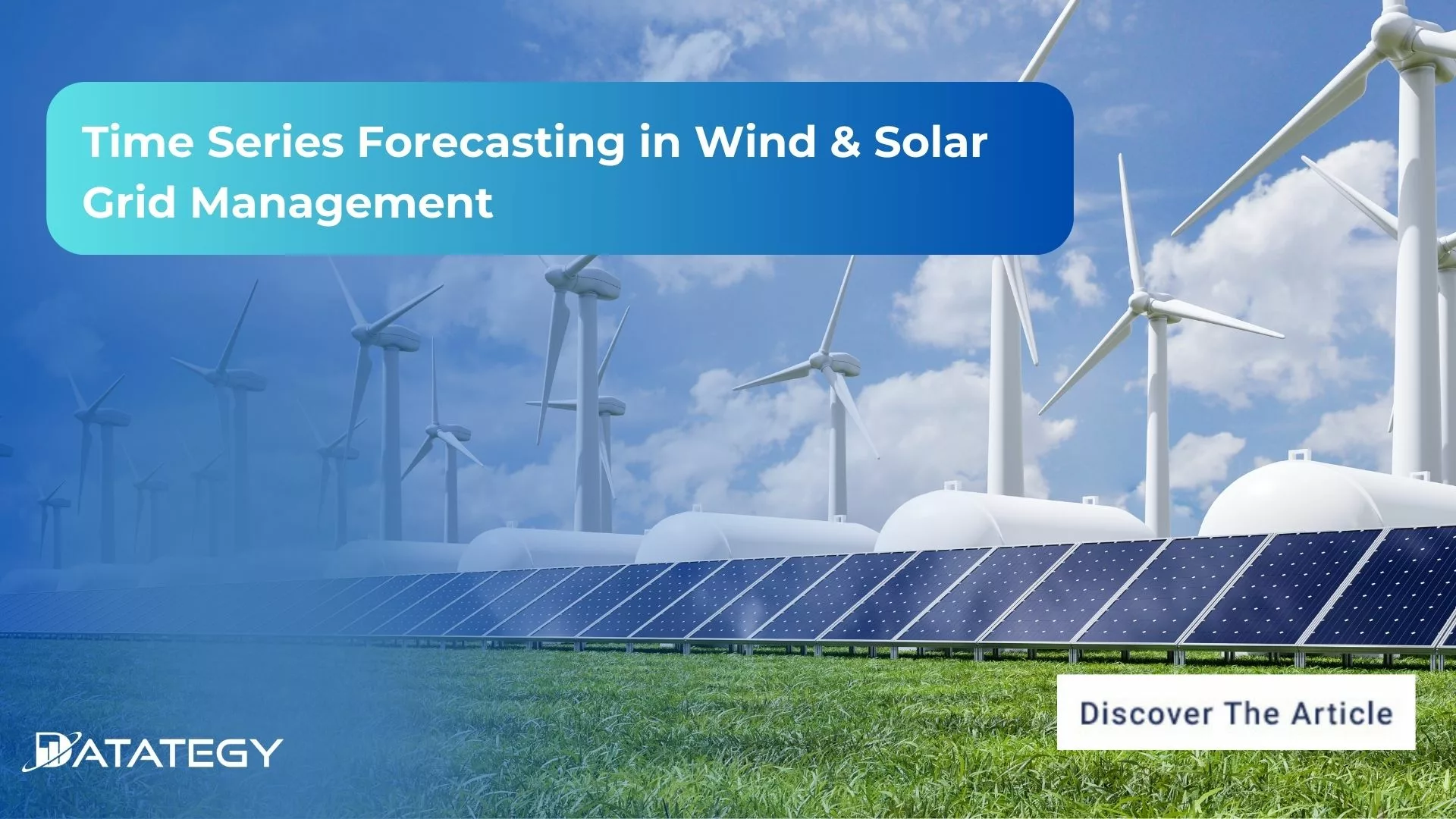
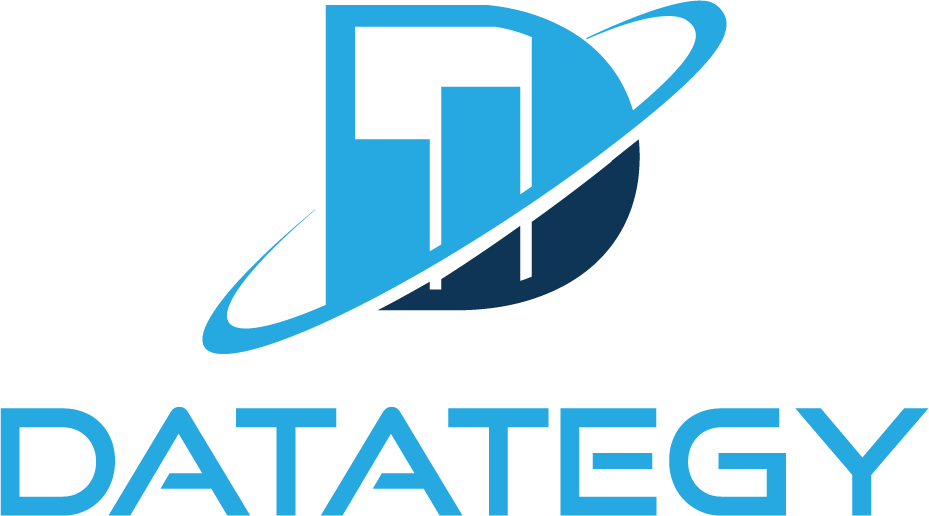