Supervised and Unsupervised Learning: A Comparison Guide Artificial intelligence (AI)...
Read MoreUnderstanding Multimodal AI Complexity
Table of Contents
ToggleBusinesses commonly use the term “multimodal” to promote their AI services in today’s tech environment. However, due to its frequent use, people frequently misunderstand what it actually means. its interpretation and application in various contexts often lack coherence and consistency. This article aims to demystify multimodal AI by examining its intricacies, difficulties, and potential to revolutionize a number of industries.
By 2037, North America is anticipated to account for about 35.9% of the multimodal AI market’s revenue, a reflection of the region’s sophisticated technical infrastructure and early adoption of AI breakthroughs. (Source: Research Nester).
Find out how papAI can improve the deployment of AI projects in Sales Teams.
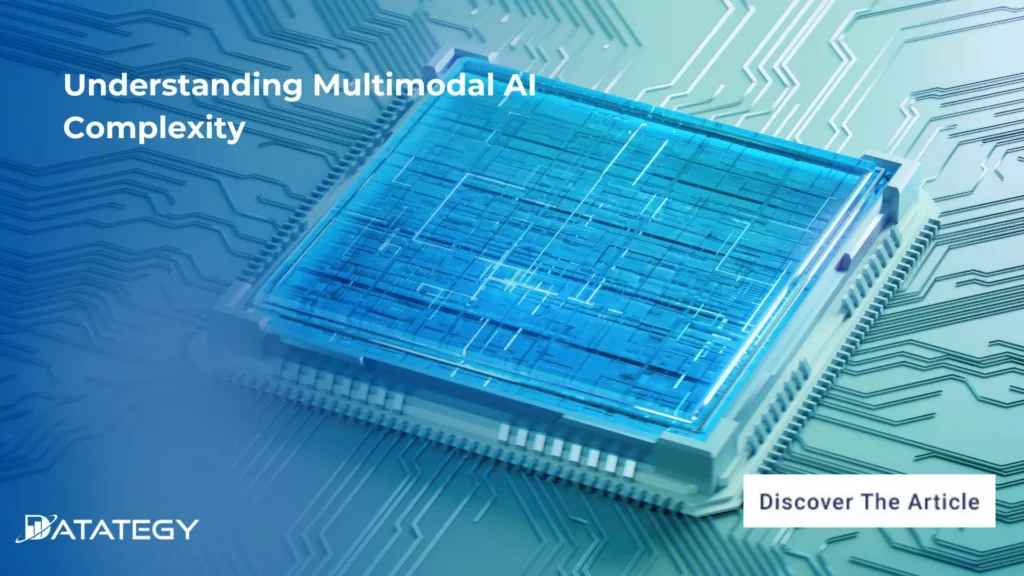
In order to improve comprehension and decision-making, multimodal artificial intelligence refers to systems that process and integrate various types of data, including text, images, audio, and more. Despite the term’s increasing popularity, let’s see the details below!
What does Multimoldal AI Mean?
Fundamentally, multimodal AI aims to emulate human cognitive capacities, as humans inherently integrate data from diverse sensory inputs to comprehend our surroundings and arrive at conclusions.
In order to execute more intricate and nuanced tasks, artificial intelligence systems that are multimodal are able to process and integrate various types of data, including text, images, audio, and video. Multimodal artificial intelligence (AI) draws on the advantages of multiple data sources, as opposed to traditional unimodal AI, which concentrates on a single type of data,
in order to improve comprehension and make better decisions. For instance, to provide a thorough diagnosis, a multimodal AI system in the medical field might concurrently evaluate patient data, medical images, and doctor’s notes..
What Characterises Multimodal AI from other types of AI?
The ability of multimodal AI can interpret and comprehend several data types such as text, graphics, audio, and even video sets it apart from other kinds of AI. To develop a more thorough grasp of the context, multimodal AI integrates data from several sources, in contrast to standard AI systems that only use one kind of input. It may, for instance, combine a picture with a description to fully understand the content, which improves the accuracy and intelligence of its answers or actions.
The capacity of multimodal AI to replicate human perception is another important characteristic. Multimodal AI seeks to mimic how humans naturally employ a variety of senses, including sight, hearing, and touch, to comprehend the environment. In addition to increasing accuracy, this method creates new application opportunities, such as healthcare solutions that integrate medical pictures with patient histories or virtual assistants that can interpret both spoken words and facial emotions.
Multimodal AI vs Unimodal AI
Aspect | Multimodal AI | Unimodal AI |
---|---|---|
Data Input | Processes multiple data types (e.g., text, images, audio) | Processes a single data type (e.g., text only or images only) |
Complexity | More complex due to integration of diverse modalities | Simpler as it focuses on one type of data |
Applications | Used in tasks like visual question answering and autonomous systems | Limited to tasks specific to one modality, like text translation |
Accuracy | Higher accuracy due to richer contextual understanding | Accuracy depends solely on a single data source |
Human-Like Perception | Mimics human ability to combine multiple senses | Limited to processing one "sense" at a time |
Development Challenges | Requires advanced models and more computational resources | Easier to develop with fewer computational demands |
What are the Types of Modalities in AI?
- Text: One of the most widely used modalities in AI is text, which includes both structured data and written language. Text-based AI models translate, summarise, translate, and create human language. They can also be used for sentiment analysis and other tasks. In order to comprehend the syntax, semantics, and context of the text, these models rely on natural language processing (NLP) techniques, which allow machines to effectively interpret and respond to human language.
- Visual: Another important modality in AI is visual data, which mainly consists of pictures and videos. AI models can now interpret and process visual data thanks to computer vision techniques, which also enable object recognition, scene comprehension, and even the ability to infer emotions from facial expressions. Visual AI has applications in everything from autonomous cars and medical imaging diagnostics to augmented reality and facial recognition. Convolutional neural networks (CNNs) are frequently used by these models to examine patterns and characteristics in images.
- Audio: The processing of sound, including speech and background noise, is a component of audio modality. Systems for audio analysis and speech recognition translate spoken words into text and recognise sounds, allowing for the use of voice assistants, transcription services, and music recommendation engines, among other applications. This modality relies heavily on technologies like natural language understanding (NLU) and automatic speech recognition (ASR), which enable AI to comprehend and produce human speech.
Challenges and Limitations of Multimodal AI
Multimodal AI models’ high processing requirements are one of their main drawbacks. These systems are resource-intensive because processing multiple types of data at once calls for a significant amount of RAM and processing power.
Training multimodal AI models entails managing sizable datasets, executing intricate algorithms, and making sure the model exhibits strong cross-domain generalization. This presents difficulties when implementing these models in real-time applications where resources might be scarce, in addition to raising the cost and duration of development.
In multimodal AI, data accuracy and reliability are also crucial problems. To guarantee that the AI system operates as intended, the data from each modality must be precise, dependable, and of the highest caliber.
One modality’s inconsistent or noisy data can have a detrimental effect on the model’s overall performance. Furthermore, it can be challenging to collect enough data using all available modalities, particularly in fields where some data types are expensive or hard to come by. Because it might not be able to learn from incomplete or unbalanced datasets, this could cause biases in the model.
The creation and application of multimodal AI are significantly influenced by ethical and privacy issues. There are more risks associated with data privacy and security when different data types are integrated, including sensitive information like medical records or private communications.
Ensuring responsible use and data safeguarding are of utmost importance. In order to prevent misuse and guarantee that AI systems respect users’ privacy, developers must put strict data protection measures in place and follow ethical guidelines.
Demystifying AI: A Comprehensive Guide to Key Concepts and Terminology
This guide will cover the essential terminology that every beginner needs to know. Whether you are a student, a business owner, or simply someone who is interested in AI, this guide will provide you with a solid foundation in AI terminology to help you better understand this exciting field.
What are the actual Multimodal AI tools in 2025?
OpenAI GPT: For tasks like picture description and context understanding, OpenAI’s GPT-4 model can handle both text and images thanks to its multimodal features.
Google DeepMind’s Gemini: A multimodal AI system that advances computer vision and language processing applications by handling text, pictures, and other data kinds.
Meta’s LLaMA (Large Language Model Meta AI): Multimodal capabilities in recent iterations of LLaMA allow textual and visual data to be integrated for more dynamic interactions.
Microsoft Azure AI: Provides multimodal capabilities with services like Text Analytics and Computer Vision, allowing companies to merge various data sources for insightful analysis.
Hugging Face Transformers: Offers open-source multimodal models like CLIP and Flamingo that are intended for applications like visual Q&A and picture captioning that combine textual and visual information.
Real-world Multimodal AI Applications
1- Self-Driving Cars:
Description: Multimodal AI is used by autonomous cars to interpret data from a variety of sensors, such as GPS, LIDAR, radar, and cameras. Accurate real-time navigation, obstacle detection, and decision-making are made possible by this integration.
For instance, Tesla’s Autopilot system uses sensor data, maps, and visual data from cameras to enable semi-autonomous driving and improve road safety.
2- Customization at Retail:
Multimodal artificial intelligence (AI) in retail can improve the shopping experience by examining past purchases, online browsing patterns, and customer feedback. Targeted marketing campaigns and customised product recommendations are made possible by this thorough analysis.
As an example, Amazon employs multimodal AI to make product recommendations based on a blend of browsing history, past purchase information, and user-generated content like ratings and reviews.
3- Content Moderation Description:
By examining text, photos, and videos, multimodal AI is used by social media sites and online communities to monitor and control content. This makes it easier to recognise and filter offensive or damaging content.
Example: Facebook employs multimodal AI, which combines text analysis and image recognition to find and address community standards violations, to identify and remove harmful content.
4- Interactive Education Platforms:
Description: By combining text, audio, video, and interactive elements, educational platforms use multimodal AI to create adaptive learning environments. This makes educational content more suited to each student’s needs and learning style.
For instance, Duolingo combines interactive practice sessions, speech recognition, and text-based exercises with multimodal AI to improve language learning.
5- Medical Surveillance:
Multimodal AI is used by wearable health devices to track heart rate, activity levels, and sleep patterns, among other health metrics. AI can identify possible problems and offer insights into general health by combining this data.
Fitbit devices, for instance, integrate information from heart rate monitors, accelerometers, and sleep trackers to provide thorough health insights and tailored recommendations.
6- Financial Fraud Detection Description:
To identify and stop fraudulent activity, multimodal AI in finance examines communication data, transaction data, and user behaviour patterns. The efficacy and accuracy of fraud detection systems are improved by this method.
For instance, Mastercard analyses transaction patterns, user behaviour, and contextual information from communication channels to identify fraudulent transactions using multimodal AI.
How papAI Platform Will Support You in This Area?
At the forefront of multimodal AI technology, papAI platform provides reliable solutions that are suited to different industries’ varied needs.
Customizable Models: papAI provides AI models that are adaptable to the demands of particular industries. papAI can create solutions that meet your specific goals and challenges, regardless of your industry—healthcare, retail, finance, or another. We also make sure that the AI complies with your operational needs.
Insights and Advanced Analytics: Using papAI, you can take advantage of its advanced analytics features to glean insightful information from your multimodal data. Our AI tools are made to manage difficult data fusion tasks and give you thorough, useful insights that improve strategic planning and decision-making.
Scalable AI: papAI’s solutions are designed to grow with your needs, making them scalable. Our AI systems are designed to grow and adapt in response to the volume and complexity of your data, so you can keep taking advantage of the most recent developments in multimodal AI technology without sacrificing reliability.
Easy to Use Interfaces: papAI places a strong emphasis on easy to use interfaces and seamless system integration. With our solutions, multimodal AI can be effectively implemented throughout your company and adopted with ease thanks to their intuitive and simple design.
Our solutions enable you to harness the full potential of multimodal AI, driving innovation and achieving better outcomes in your field. Book your demo now to watch our platform in action.
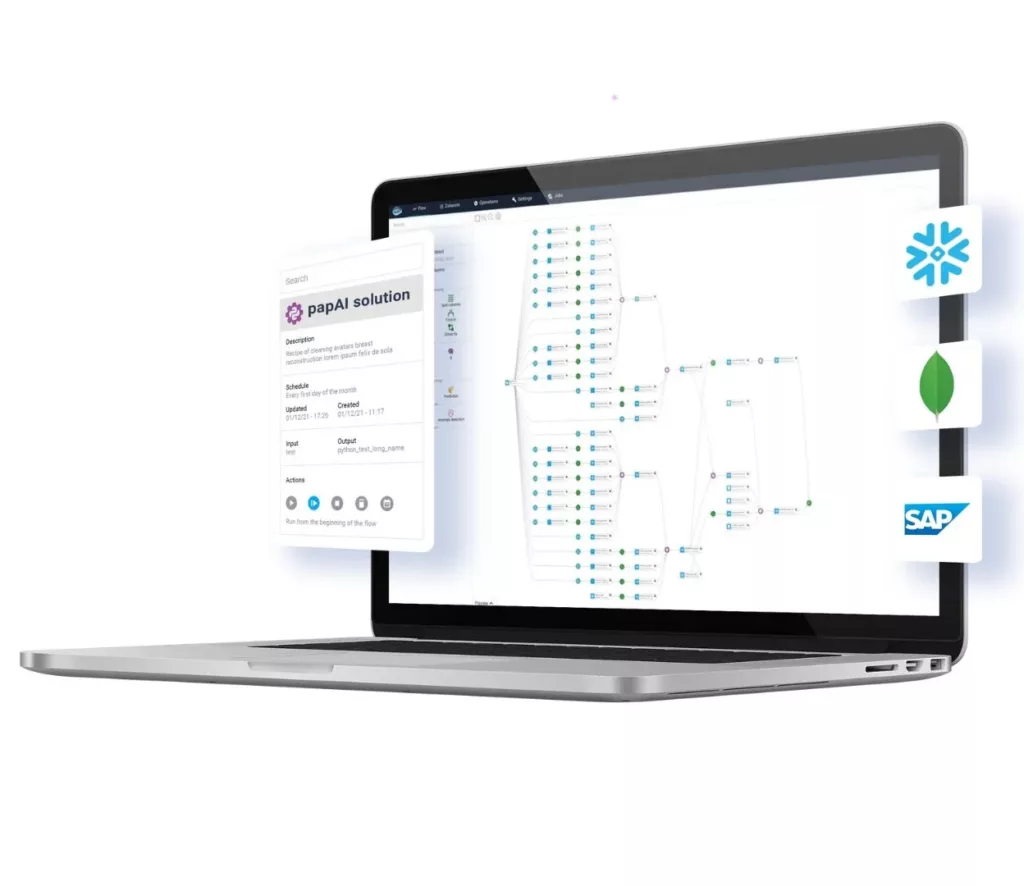
Interested in discovering papAI?
Our AI expert team is at your disposal for any questions
“DATATEGY EARLY CAREERS PROGRAM” With Zineddine Chikhaoui
“DATATEGY EARLY CAREERS PROGRAM” With Zineddine CHikhaoui Hello, my name...
Read MoreAI Governance Pathway: Challenges and Solutions
AI Governance Pathway: Challenges and Solutions Recent advances in artificial...
Read MoreHow AI drives Operational Excellence in Manufacturing Industry
How AI drives Operational Excellence in Manufacturing Industry Operational excellence...
Read More