Optimize RAG Systems Like a Pro with Fine-Tuning As artificial...
Read MoreBoosting Profits with AI-Based Dynamic Pricing
Table of Contents
ToggleIn this constantly evolving business landscape, it is crucial to sell the appropriate product at the correct price to boost growth and enhance revenue. For this reason, artificial intelligence solutions can play a critical role in this domain.
Recent studies show that AI-powered dynamic pricing can lead to revenue increases between 5% and 20%.
Find out how papAI can improve the deployment of AI projects in Sales Teams.
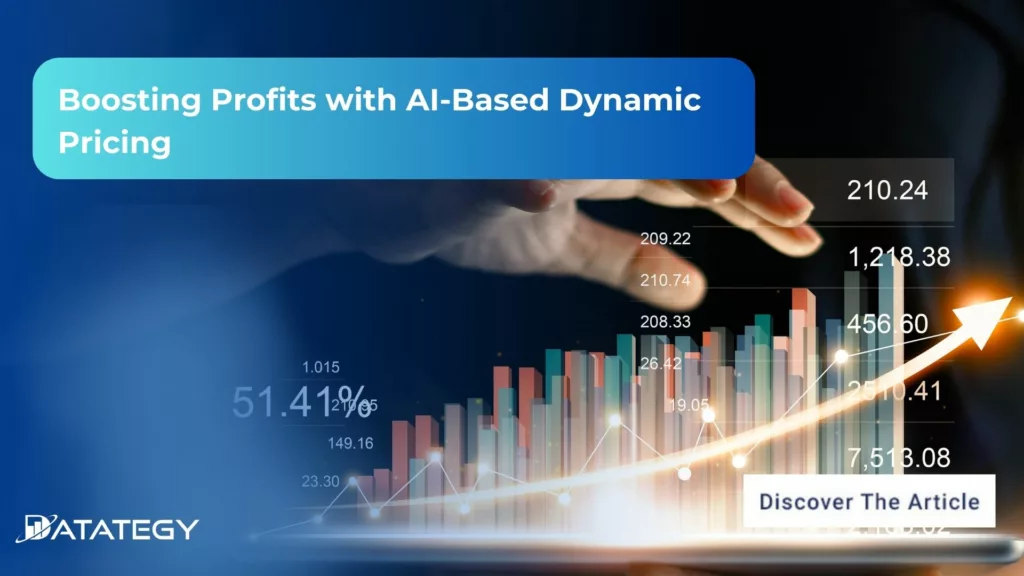
This piece will explore the advantages of employing AI-driven dynamic pricing to boost earnings and revenue, along with its practical implementation in real-life scenarios.
What does Dynamic Pricing Mean?
The airline industry introduced dynamic pricing in the 1980s. Setting rates according to demand, booking trends, and competition set American Airlines apart. This strategy demonstrated the potential of dynamic pricing to increase profits and efficiency by increasing prices during peak times and providing discounts during slower periods, helping airlines maximize revenue.
With the development of the internet in the 1990s, dynamic pricing was extended to e-commerce. Data analytics was used by retailers such as Amazon to make real-time price adjustments based on competitor pricing, inventory levels, and consumer behavior.
Due to the availability of more advanced and accurate pricing models through online data processing, this era transitioned from niche airline strategies to widespread business use.
AI and machine learning have revolutionized dynamic pricing in the twenty-first century, increasing its efficacy. Artificial intelligence (AI) systems generate highly accurate and responsive strategies by analyzing large data sets and making real-time pricing decisions with little assistance from humans.
Advantages of Dynamic Pricing Based on AI
1. Quick Adjustment to the Market
The capacity to quickly adjust to changes in the market is among the most alluring advantages of AI-driven dynamic pricing. AI can change prices instantly, in contrast to conventional pricing methods that could take days or weeks to adjust. Because of their ability to adapt quickly, businesses can take advantage of unexpected changes in consumer demand or quickly respond to price adjustments made by rivals, helping them to remain competitive and relevant in quickly evolving markets.
2. Enhanced Efficiency in Operations
By using AI to automate the pricing process, less manual intervention is required, which frees up important time and resources. Instead of devoting hours to pricing establishment and modification, staff members can concentrate on strategic duties. This efficiency makes the whole operation more responsive and nimble by reducing expenses and expediting the pricing process.
3. More Effective Inventory Control
Dynamic pricing facilitates the matching of inventory levels to real market demand. Businesses can avoid overstocking slow-moving items and understocking high-demand items by modifying prices according to product performance. This equilibrium lowers carrying costs and stockout risks, resulting in a more effective supply chain and satisfied customers who can always find what they need.
Real-world Applications of Using AI-Based Dynamic Pricing
1. E-commerce
AI-driven dynamic pricing is revolutionizing e-commerce by increasing competitiveness and optimizing sales. E-commerce platforms work in a fast-paced market where shifting consumer demand and competitor activity can cause prices to change quickly. Because AI algorithms are so good at processing large amounts of data quickly, businesses can dynamically change their prices to stay competitive and take advantage of market opportunities.
To forecast changes in demand, AI examines consumer browsing habits, purchase trends, and engagement metrics. AI has the ability to automatically modify prices, for instance, to maximize conversion rates and stimulate demand during promotional events or peak shopping seasons. On the other hand, during slower times, prices can be changed to draw in budget-conscious clients without compromising profitability.
2. Hospitality
To determine the best room rates, AI takes into account variables like past booking trends, seasonal trends, nearby events, and competitor pricing. In order to profit from increased demand, AI may suggest higher rates during periods of high demand, such as holidays or significant events. On the other hand, rates can be changed during off-peak hours to maximize occupancy rates and draw in tourists on a tight budget.
3. Transportation
AI-based dynamic pricing is essential to the transportation industry, including ridesharing, airlines, and freight logistics, as it optimises pricing strategies to balance supply and demand.
AI-driven dynamic pricing is used by rideshare companies to adapt to changing demand throughout the day. To dynamically modify fares, algorithms examine real-time data on rider requests, traffic patterns, and driver availability. Prices may rise, for instance, during rush hours or in busy regions to encourage more drivers to enter the market and guarantee quicker pick-ups for clients prepared to pay a higher rate.
How to Implement Dynamic Pricing Strategy in your Organization?
Examine Current Pricing Structure: To start, closely examine your current pricing structure. Determine which domains can benefit most from AI-driven dynamic pricing in terms of increased revenue streams, better inventory control, and better market responsiveness.
Data Integration and Aggregation: Combine information from a variety of sources, such as consumer profiles, competitor pricing patterns, historical sales data, and market dynamics. Combine these datasets to create a cohesive data architecture that facilitates in-the-moment analysis and judgment.
Choose AI Platforms and Tools: select AI platforms and tools that complement your technological infrastructure and business needs. Take into account factors like scalability, interoperability with current IT systems, and the capacity to modify models and algorithms to achieve particular cost goals.
Drive Implementation and Testing: To validate the AI-based dynamic pricing system, start the pilot implementation phase. To assess accuracy, responsiveness, and alignment with business objectives, test its performance with a subset of products or markets. Before deploying on a large scale, thoroughly test the algorithms to improve them and reduce risks.
Training and Adoption: Educate pertinent parties on how to use pricing recommendations wisely and interpret insights produced by AI. To guarantee informed decision-making and optimize the advantages of AI-driven pricing strategies, cultivate a data-driven culture within the company.
AI and Machine Learning for Sales:
The Key to Unlocking Growth Potential
The development of artificial intelligence (AI) has significantly changed the sales industry, which has witnessed a remarkable evolution throughout time. The way companies approach sales processes and strategies has been completely transformed by AI. In this white paper, we will examine the concept of AI in sales, its historical evolution, and the advantages it provides for companies.
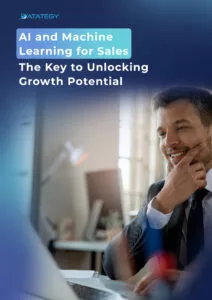
Use Case: Leveraging papAI Solution for Dynamic Pricing in the Airline Industry
Context
Airlines use optimized pricing strategies to try and maximize revenue and market share in the highly competitive field of air travel. This study’s main objective is to create a reliable model with papAI that can dynamically determine each flight’s ideal base price, optimizing revenue for the airline.
The user-friendly AI platform from papAI effectively unifies various data sources, cleans and processes data, and applies cutting-edge predictive models. papAI provides data-driven pricing recommendations by analyzing flight data and pertinent factors such as seasonal trends, demand patterns, and competitive pricing levels. Airlines are equipped with state-of-the-art resources through papAI to negotiate the challenges of dynamic pricing successfully.
1- Data Exploration
To build an effective dynamic pricing model, we need to prepare our initial dataset of 452,088 rows, which includes booking dates, journey details, and pricing information. Using papAI integrated tools, we will clean and organize this data by filtering and restructuring the dataset. This preparation ensures accuracy and reliability in our model, enabling us to maximize airline revenue efficiently.
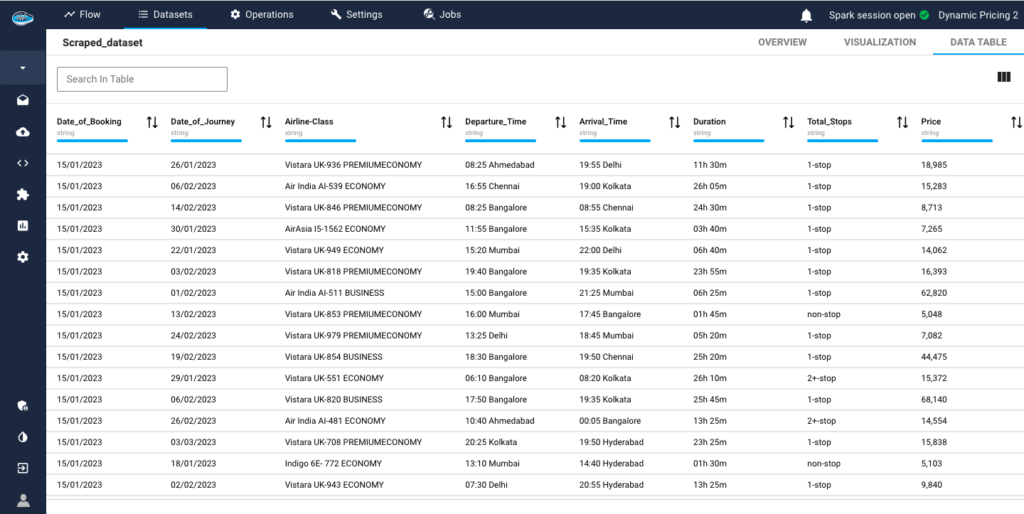
After easily importing the dataset into papAI, we initiated the process by enhancing the “Total Stops” and date columns to provide more meaningful insights for the model. Utilizing papAI’s cleaning step, we converted the values as follows: “non-stop” was assigned 0, “1-stop” was designated as 1, and “2+-stops” were represented by 2. For the date columns, we parsed “date_of_booking” and “date of journey” into proper date formats. These transformations enable the model to effectively interpret and leverage the data for precise predictions.
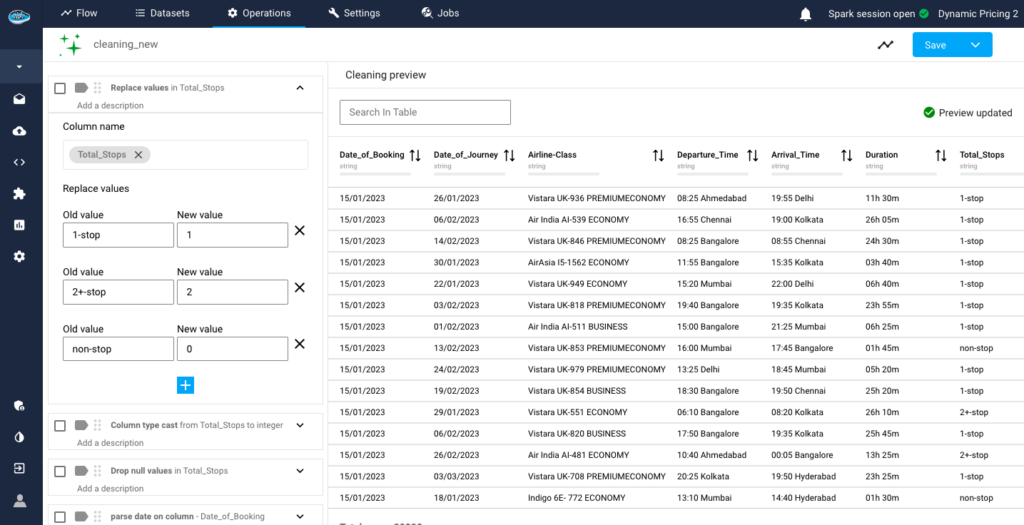
We proceeded by splitting the ‘Departure_Time’ and ‘Arrival_time’ columns, and removing commas from the ‘Price’ column to facilitate conversion into integers, considering it represents values in thousands. To achieve this, we employed papAI’s Spark SQL recipe, streamlining our data cleaning process.
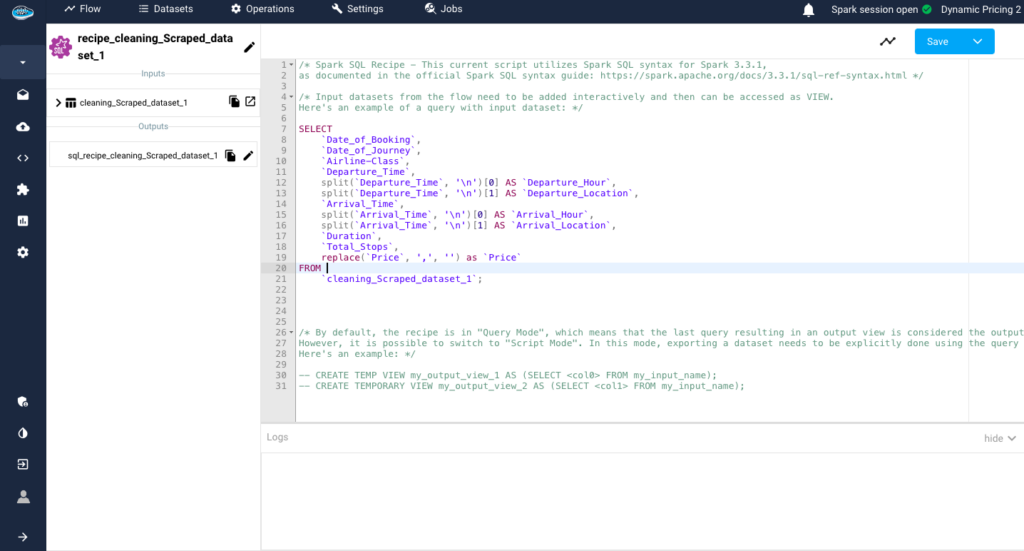
After that, we restructured the ‘Airline-Class’ column, which encapsulates details such as the airline and booking class. This involved splitting it into two distinct columns: ‘Airline-Name’ and ‘Class’. Additionally, we converted the duration to a double format for enhanced interpretation by the model. Leveraging papAI’s Python recipe module, we efficiently applied these transformations using popular libraries like Pandas.
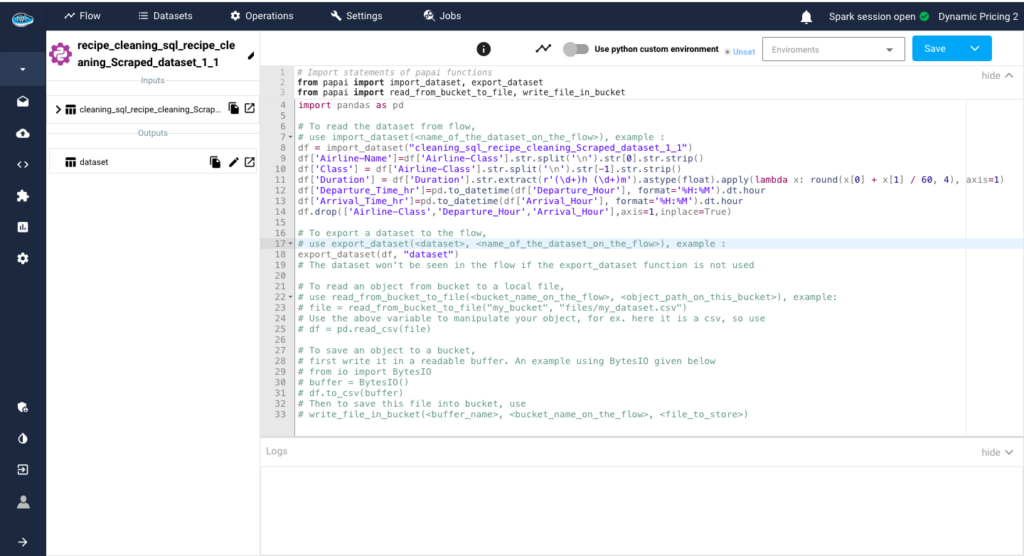
Now, harnessing papAI‘s remarkable time series cleaning feature, we will derive key features from our dataset that influence flight prices. These include:
- Whether the flight occurs on a holiday.
- Whether it falls on a weekend.
- The day of the week.
- The total number of days waited from the time of booking.
By considering factors such as holidays, weekends, and booking timelines, we aim to uncover patterns and trends that significantly impact airfare pricing. Understanding these nuances allows for more accurate predictions.
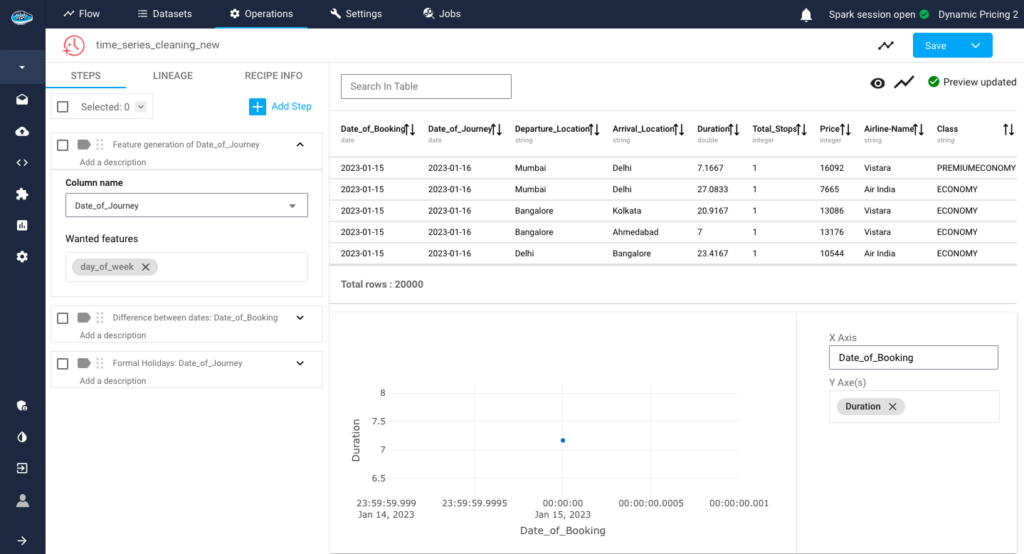
2- Exploratory Data Analysis: Visualisation with papAI
Data visualization is essential in dynamic pricing, offering visual insights into demand patterns, customer behavior, and the competitive landscape among airlines. This visual representation enhances our understanding of the data, facilitating more informed pricing decisions and strategic planning.
Thanks to papAI’s advanced data visualization module, users can explore a diverse array of graphical representations, including 2D, 3D, and cartographic visuals. This capability empowers in-depth analysis and comprehensive assessments of datasets.
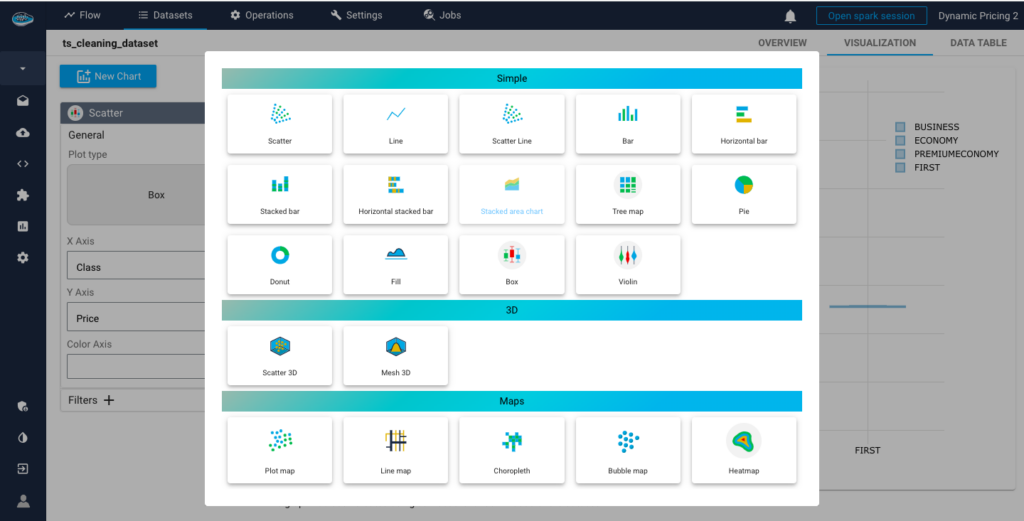
To further analyze the relationship between price and booking class, we are creating a box plot to visualize the distribution of prices across different booking classes. This will help us identify variations and trends within each class. Utilizing papAI, this visualization can be easily generated, allowing us to quickly gain insights into the pricing structure and make data-driven decisions.
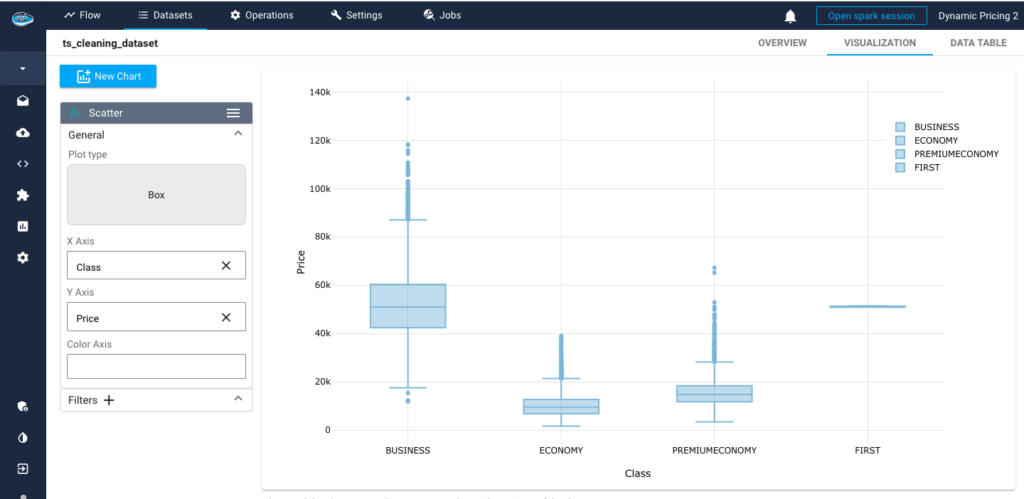
3- Machine Learning Process
3-a) Segmentation
It’s important to segment data and perhaps build different models for different segments and be able to tailor pricing strategies to the unique characteristics of each segment and optimize revenue. First-class and business-class customers often exhibit a high willingness to pay for tickets, as they prioritize luxury, comfort, and personalized services. Customers in the economy are more likely to be price sensitive and prioritize affordability and value when choosing flights.
I therefore proceeded to build a model for economy class and narrowed it down to a specific airline. For this segmentation, we will use papAI’s filter operation to efficiently refine our dataset.
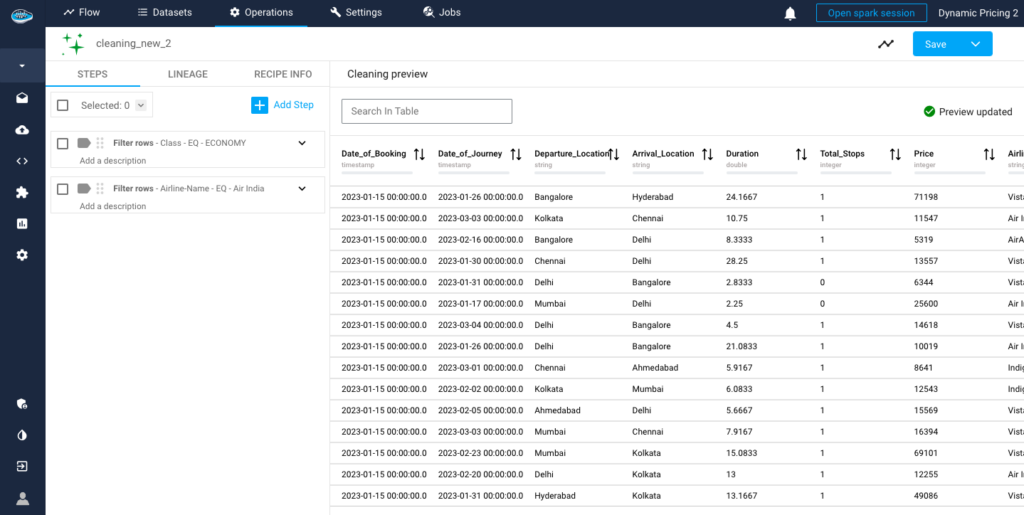
Following this operation, we obtained a dataset containing 449,427 rows. We then proceeded to split this dataset into two parts: one for training the model and another for testing its predictions.
3-b) Model Training
Next, we will build a regression model to predict flight prices. Using papAI’s ML Lab, we can train multiple models utilizing a variety of algorithms, including Linear Regression, Random Forest, and Decision Tree, among others.
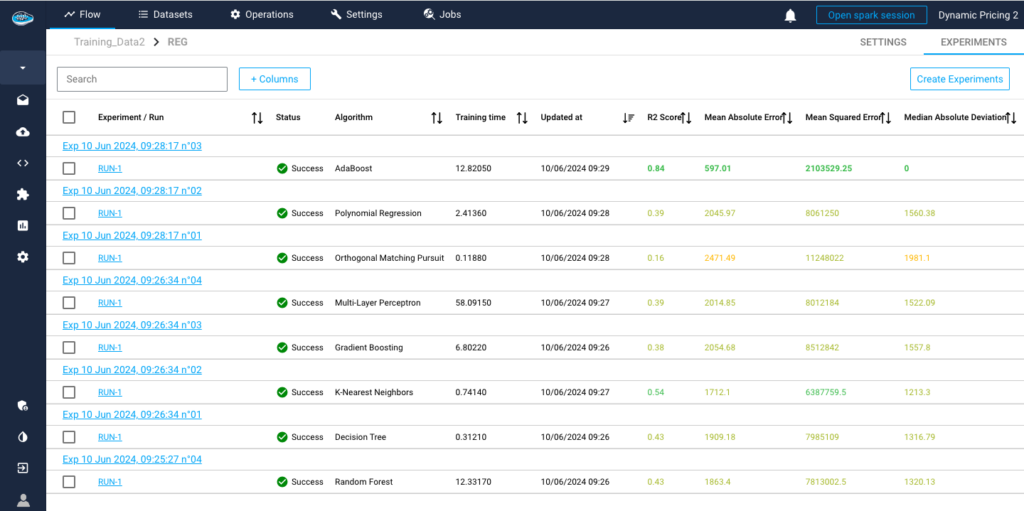
In the provided visualization, algorithms are ranked based on various metrics and color-coded according to their performance, aiding us in selecting the optimal model. Among these, adaBoost emerged as the top performer, achieving an impressive R2 score of 0.84.
3-C) Evaluating the Model
After completing the training, a dashboard displaying various metrics for each model is shown, enabling a thorough understanding and evaluation of their performance. This aids in selecting the most suitable model for the specific use case.
For the Ada Boost model, we observe an excellent evaluation score and a Predicted values vs true values plot that demonstrates the model’s prediction accuracy against actual values. Nonetheless, it is essential to understand the model’s inner workings before making a final selection.
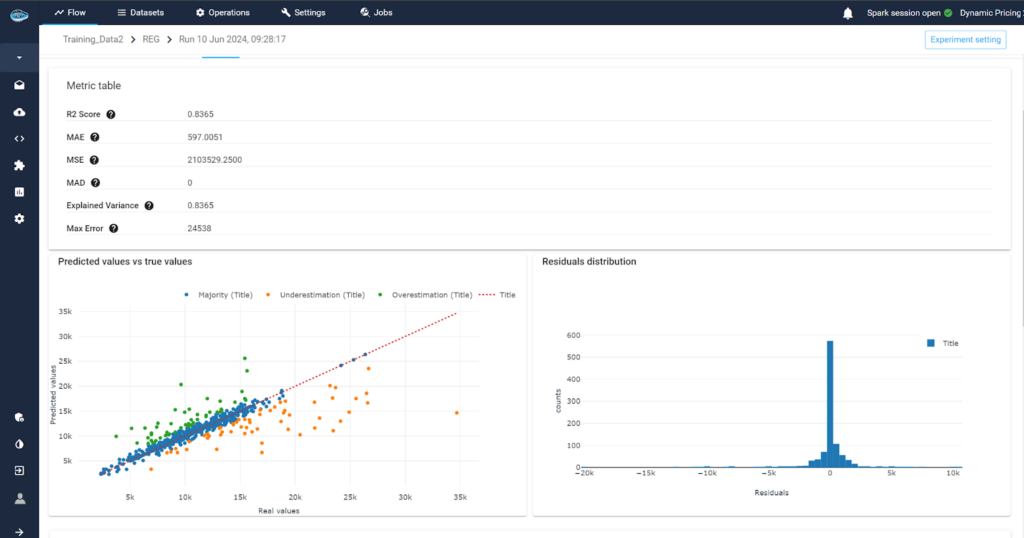
After completing the training, a dashboard displaying various metrics for each model is shown, enabling a thorough understanding and evaluation of their performance.
This aids in selecting the most suitable model for the specific use case. For the Ada Boost model, we observe an excellent evaluation score and a Predicted values vs true values plot that demonstrates the model’s prediction accuracy against actual values. Nonetheless, it is essential to understand the model’s inner workings before making a final selection.
3-d) Interpret the Model
To gain a deeper understanding of the model, papAI includes an explainability module. This module not only evaluates the model but also explains how predictions are made and how various features influence the model’s decisions. As we can see, the model effectively captures all features that influence the price, enabling it to update the price dynamically.
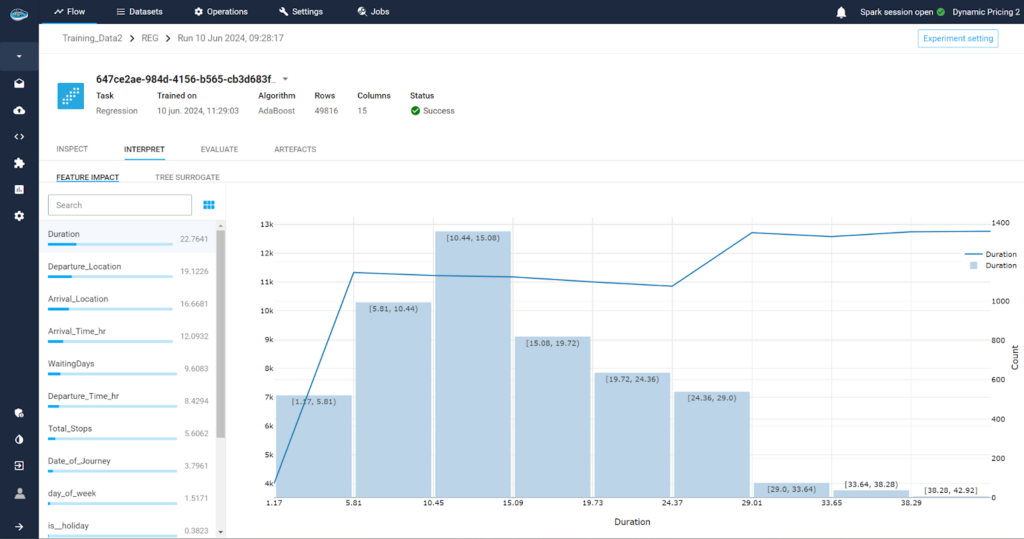
4- AI Model's Prediction
After looking through the explainability module and choosing the right model, you can promote it to apply prediction on other datasets with the same schema used for the training. A new dataset is created to compare the predicted value and the real value.
Additionally, you can examine each individual prediction in detail using our local interpretability module to gain deeper insights into the decision-making process.
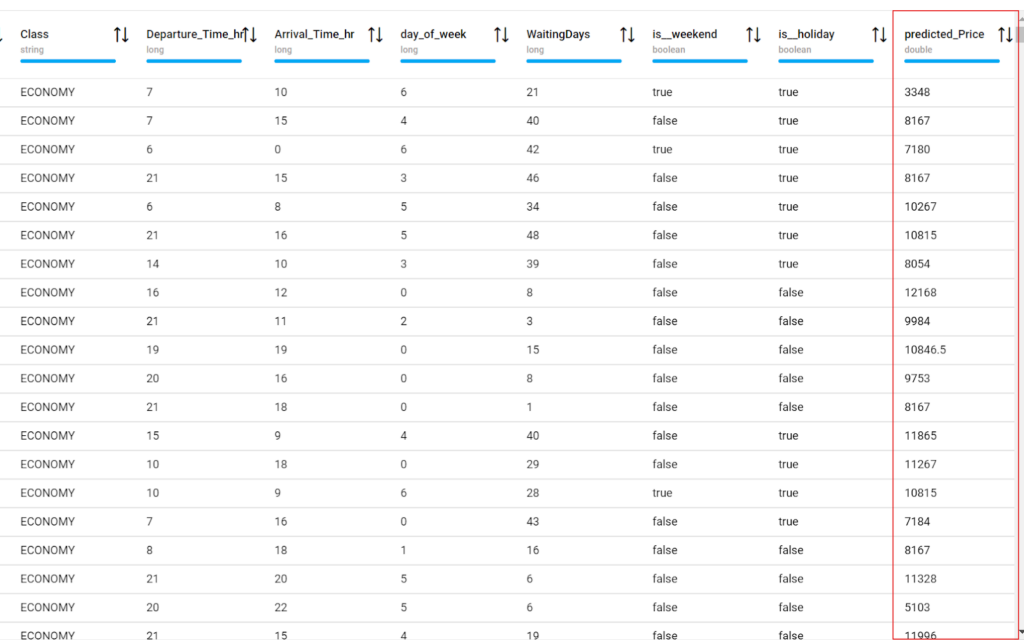
Create your Own AI-based Dynamic Pricing Tool using papAI
Businesses can use papAI’s user-friendly platform to leverage artificial intelligence without requiring a high level of technical expertise.
Businesses can use papAI to create custom algorithms that analyze complex datasets in real-time, including competitor pricing, market trends, and customer behavior. This personalization makes it possible to implement dynamic pricing changes that maximize profits, boost productivity, and raise client satisfaction.
Schedule your demo today. Our team of Data Scientists is poised to help you craft a bespoke AI-based solution perfectly tailored to your organization’s unique needs.
Interested in discovering papAI?
Our AI expert team is at your disposal for any questions
Supervised and Unsupervised Learning: A Comparison Guide
Supervised and Unsupervised Learning: A Comparison Guide Artificial intelligence (AI)...
Read More“DATATEGY EARLY CAREERS PROGRAM” With Zineddine Chikhaoui
“DATATEGY EARLY CAREERS PROGRAM” With Zineddine CHikhaoui Hello, my name...
Read MoreAI Governance Pathway: Challenges and Solutions
AI Governance Pathway: Challenges and Solutions Recent advances in artificial...
Read More