You Don’t Need Big Investments to do AI anymore. Here’s...
Read MorePredict Customer Life-time Value using AI
Table of Contents
ToggleUnderstanding your customers is essential in sales to propel company expansion. Customer lifetime value is a crucial indicator to address this need. Businesses can accurately predict customer lifetime value (CLV) and adjust their strategies to optimize customer engagement and profitability by gathering multiple data points, including subscription dates, total purchase amounts, and historical usage patterns.
20% of customers account for 80% of future revenue. By using AI to understand CLV, businesses can maximize revenue potential by focusing marketing and retention efforts on high-value customers first.
Find out how papAI can improve the deployment of AI projects in Sales Teams.
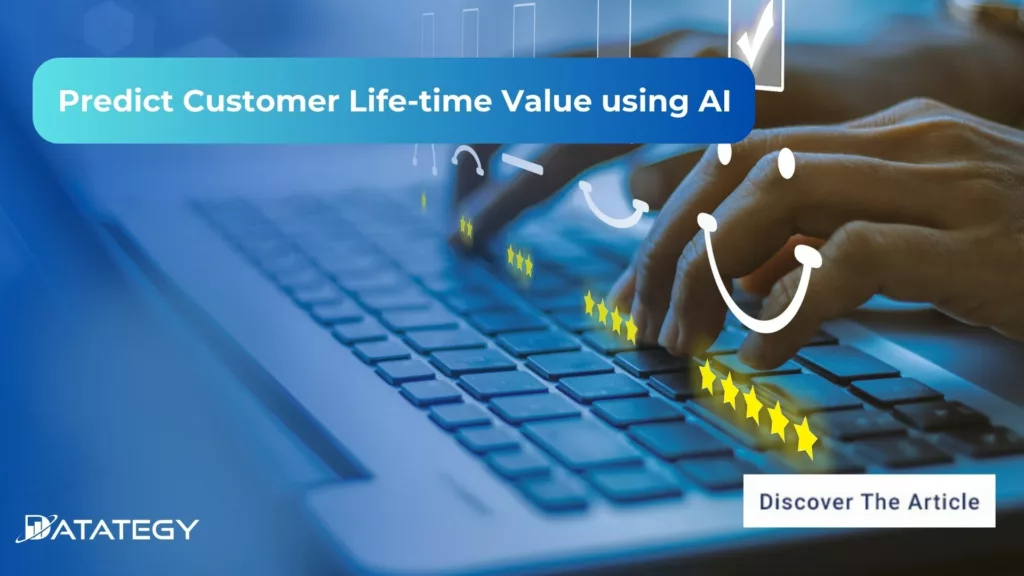
This article will examine the benefits of using AI to increase productivity and profits as well as how it can be applied to this use case.
What does Customer Life-time Value Mean?
Customer Lifetime Value (CLV) is a significant indicator that shows how much money a company can make from a single customer over the course of their relationship. Data collection and analysis from a variety of customer touchpoints are required for CLV prediction.
This involves monitoring past purchases, participation in marketing initiatives, and even exchanges on social media. Accurate CLV prediction is made possible by advanced analytics and artificial intelligence (AI), which enables companies to segment their clientele and offer individualized experiences. These insights can result in more focused marketing campaigns that raise customer satisfaction levels while also increasing rates of loyalty and retention.
Accurate CLV prediction is made possible by advanced analytics and artificial intelligence (AI), which enables companies to segment their clientele and offer individualized experiences.
Businesses in the digital age gather a tonne of information from different customer interactions, such as past purchases, website usage, and social media activity. Even though there is a lot of information here, it can be difficult to process and evaluate by hand.
Artificial intelligence (AI) is useful in this situation. Businesses can efficiently sort through this massive amount of data and derive actionable insights that improve Customer Lifetime Value (CLV) thanks to AI’s sophisticated data processing capabilities.
Benefits of Using AI in Customer Lifetime Value Prediction
1. Increased Prediction Accuracy:
More accurate CLV predictions result from AI’s capacity to combine data from multiple sources and examine intricate patterns. Conventional techniques frequently find it difficult to manage the enormous amount and variety of data produced in today’s digital environment. AI algorithms are very good at processing big datasets and spotting minute relationships in the data because they are driven by machine learning and deep learning techniques.
Businesses can make sure that every piece of customer data is used to its maximum potential by utilising AI. AI algorithms can find important insights that traditional methods might miss, whether it’s transaction history, website interactions, or social media engagement. Businesses can now allocate resources more intelligently and make better decisions regarding marketing tactics, customer retention campaigns, and resource allocation.
2. Enhanced Client Segmentation:
as AI can segment customers based on complex behavioral data, marketing campaigns are more focused and have a higher return on investment. In order to target particular audience segments with marketing messages and offers, customer segmentation is crucial. Traditional segmentation techniques, on the other hand, frequently depend on demographic information or broad categories, which might not fully capture the complexity of consumer behavior.
A multitude of factors are considered in AI-driven segmentation, such as past browsing activity, purchase patterns, and product preferences. Using machine learning models, this data is analyzed to find trends and create customer segments based on shared characteristics. This enables companies to develop highly focused marketing campaigns that speak to the particular interests and preferences of each segment.
3. Improvement in Decision-Making:
Businesses can make better decisions throughout the company with the help of AI’s data-driven insights. Conventional approaches to decision-making frequently depend on personal opinion or scant information, which can produce less-than-ideal results. Contrarily, AI-driven insights are derived from thorough examination of sizable datasets and are capable of revealing hidden patterns and trends that human analysts might miss.
Businesses can make decisions with more assurance and accuracy by utilising AI, which lowers the possibility of expensive mistakes and lost opportunities. When it comes to pricing strategies, product launches, or market expansion, AI-driven insights offer invaluable advice that enables companies to successfully navigate difficult situations and seize new opportunities.
Real-world Applications of Using AI to Predict CLV
Retail Customer Segmentation: Using AI, retailers can divide up their clientele into groups according to potential CLV. In order to maximize long-term profitability, they can identify segments with the highest customer lifetime value (CLV) and customize marketing strategies by analyzing past purchasing behavior, demographics, and engagement patterns.
Customer Lifetime Value Prediction in Telecommunications: Telecommunications companies use AI to forecast their customers’ CLV. Through the examination of usage trends, past customer interactions, and service records, they are able to pinpoint high-value clients and apply focused retention tactics to minimize attrition and optimize client lifetime value.
Cross-selling in the financial services industry: Banks and other financial organizations use AI to forecast customer lifetime values (CLVs) and spot chances to cross-sell extra goods and services. They can target high CLV customers with tailored offers by examining transaction history, financial behavior, and customer demographics. This will increase.
Player Retention in Online Gaming: AI is used by online gaming companies to predict players’ CLV and maximize player retention. They can detect high CLV players and customize gameplay and promotions to boost retention and optimize lifetime value by examining gaming behavior, in-game purchases, and engagement patterns.
Subscription services for digital media: AI is used by digital media platforms to forecast subscribers’ lifetime values and maximize subscription retention. Through the examination of viewing patterns, preferred content, and engagement metrics, they are able to pinpoint high CLV subscribers and customize subscription offers and content suggestions to boost retention and optimize earnings.
How to Implement AI to CLV Prediction?
1. Gathering and preparing data:
Let’s start by collecting all the information required to estimate Customer Lifetime Value (CLV). We’re discussing anything and everything, including past purchases, website clicks, and social media interactions. Getting as much information as you can from a variety of sources, such as CRM systems, e-commerce sites, and even customer reviews, is crucial in this situation. After obtaining all of this data, we must tidy it and put it in a proper order. This entails removing any duplicates, correcting any mistakes, and ensuring that everything is in a format that our AI models can comprehend.
2. Selecting the Appropriate AI Model:
Now that we have flawless data, it’s time to select the appropriate AI model to utilise. Numerous AI algorithms are available, each with unique advantages and disadvantages. Taking into account our unique dataset and business objectives, we must select the model that is most appropriate for predicting CLV. There may be some trial and error involved here, but that’s all the fun! The objective is to identify which machine learning algorithm—a more complex deep learning model or a machine learning algorithm like linear regression—produces the most accurate predictions.
3. Training and Testing the Model:
The next step is to test our AI model after we’ve selected it. This process, known as training the model, will begin with us giving it a portion of our data to work with. It’s similar to teaching a dog new tricks in that it becomes more adept at predicting CLV the more data it is given. However, we must ensure that it is performing its duties effectively; we cannot simply rely on its word. Testing is then relevant. To see how well the model predicts CLV, we will feed it some fresh, unseen data.
AI and Machine Learning for Sales:
The Key to Unlocking Growth Potential
The development of artificial intelligence (AI) has significantly changed the sales industry, which has witnessed a remarkable evolution throughout time. The way companies approach sales processes and strategies has been completely transformed by AI. In this white paper, we will examine the concept of AI in sales, its historical evolution, and the advantages it provides for companies.
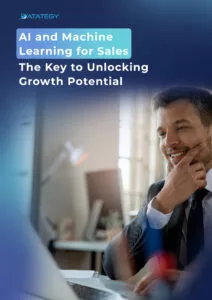
Use Case: Use Case: Using papAI Solution to predict Customer Life-time Value
Context
This dataset provides a comprehensive view of the factors influencing CLV, it contains detailed information about customers and their interactions with an insurance company, such as Customer ID, State, Gender, Income, and Marital Status; insurance-specific data such as Coverage, Education, Employment Status, Policy Type, Policy, Renew Offer Type, Sales Channel, Total Claim Amount, Vehicle Class, and Vehicle Size; and time-related factors like Effective Date, Months Since Last Claim, Months Since Policy Inception, and Number of Open Complaints. The Customer Lifetime Value is the target variable, while Response indicates whether a customer responded to marketing efforts.
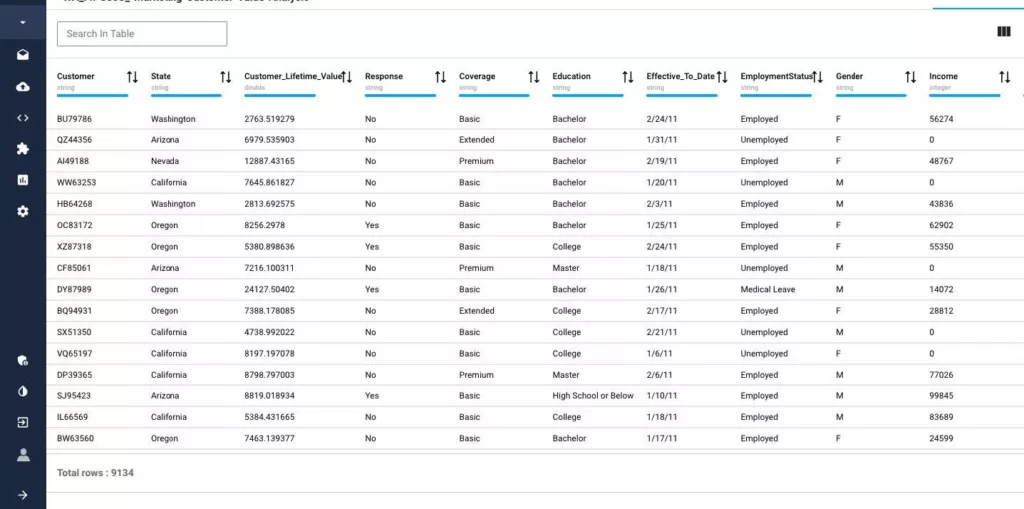
1- Data Exploration
papAI further enhances its functionality with an automated statistics calculation feature, offering users an in-depth understanding of dataset characteristics through detailed statistical analysis.
This feature automatically computes various statistics for each variable within the dataset. Key statistics calculated include the type of each variable, which can be either “categorical” or “numerical”.
The system computes average value, standard deviation (a measure of data dispersion), minimum value, maximum value, and other relevant metrics for numerical variables.
For categorical variables, the feature identifies the distinct categories and their respective frequencies, offering insights into the distribution of categorical data. This robust analysis equips users with a thorough understanding of their data, facilitating more informed decision-making and analysis.
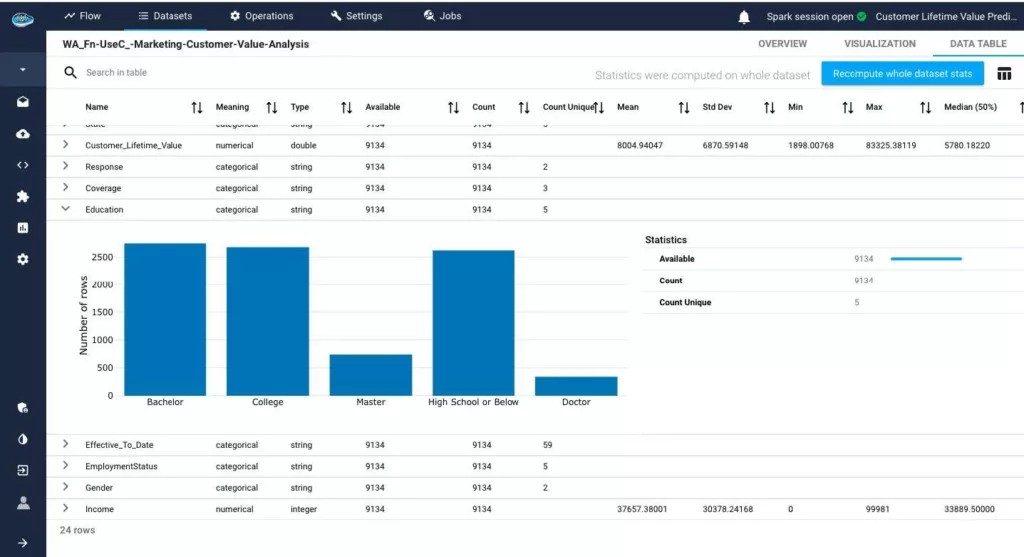
The provided dataset has lots of details, we can cite :
– There are 9134 Observations of 24 Variable
– There is a mix of categorical and continuous DataType.
– There are no null values, so no further action is required to replace
missing or null values.
– Mean of CLV is 8004.94047 and the median is 5780.18220 .
– There are a lot of Customers with low CLV. Very few customers
with high CLV.
papAI also provides an extensive array of visualization tools, including bar plots and box plots. Let’s explore some visualizations related to CLV based on Monthly premium, Coverage, Employment using bar plots , Scatter and box plots.
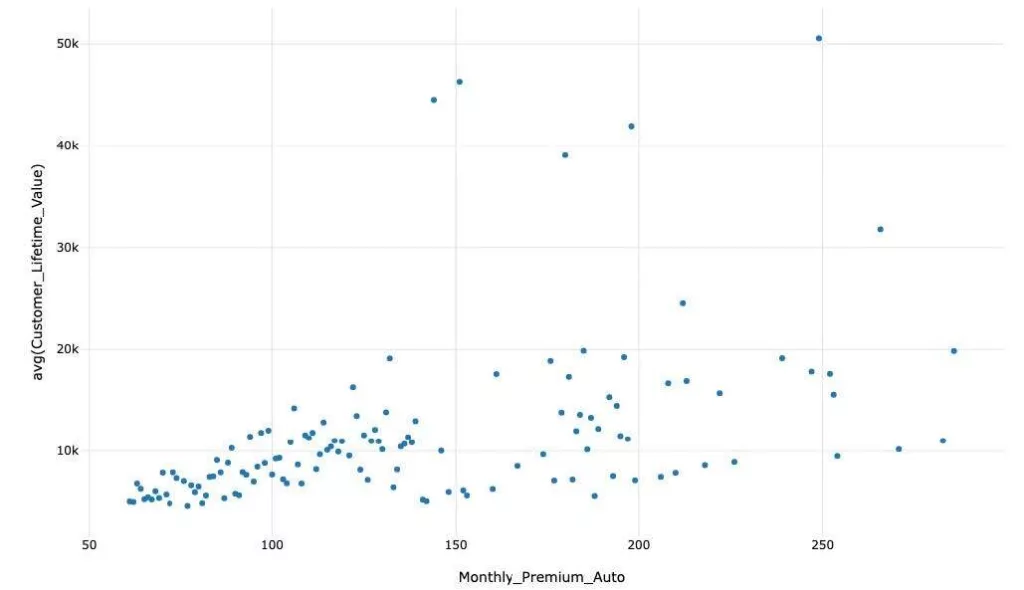
There is a Positive Corelation of 39.62 % of MPA with CLV. From scatter plot, it is clearly visible that on Monthly premium , CLV is also Increasing.
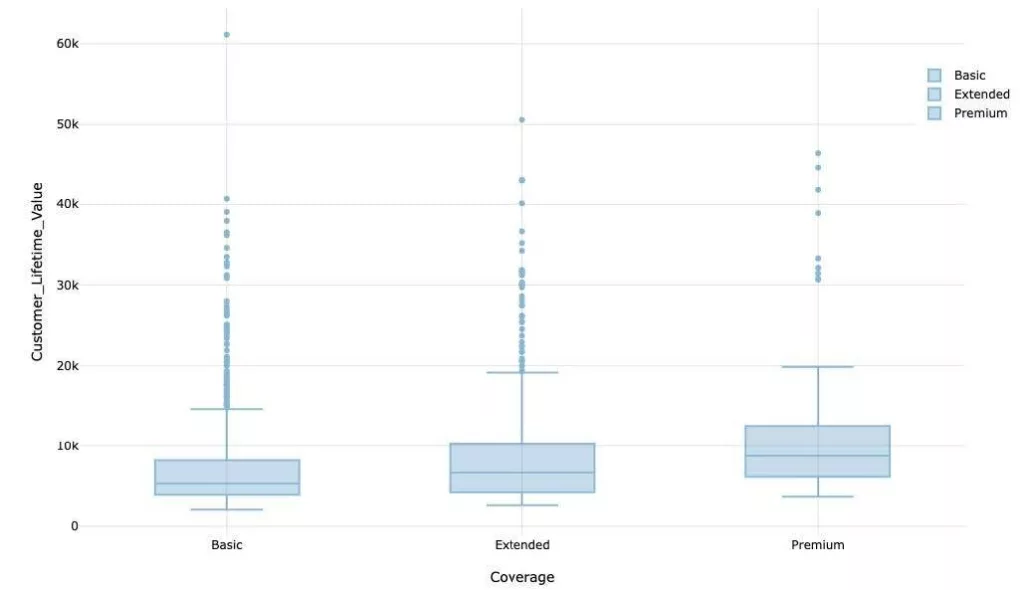
Customers who have taken Basic Insurance for their vehicles are more valuable then Extended or Premium Insurance Policy holders.
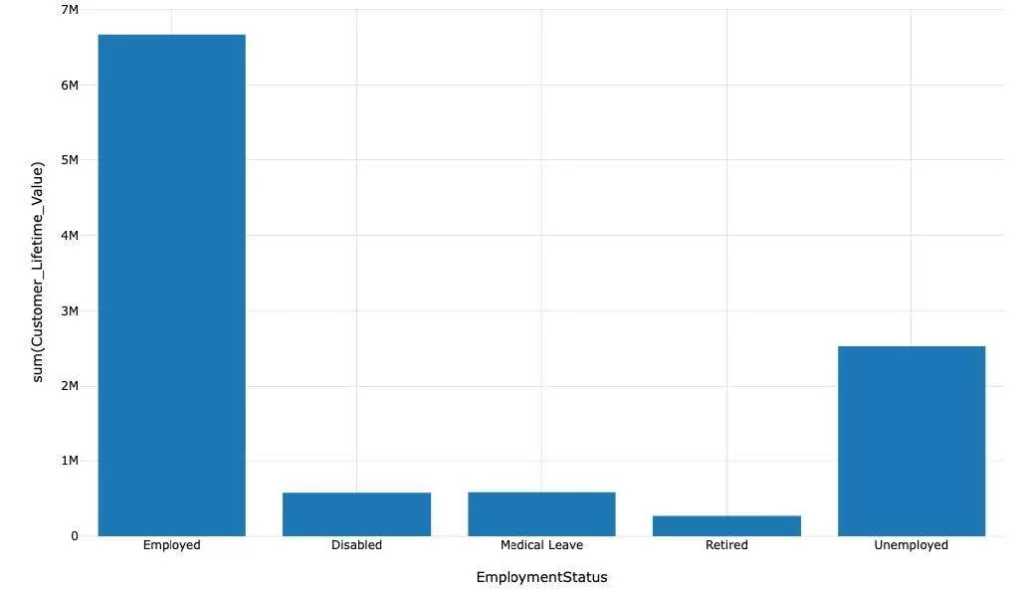
Employed customers are more valuable than others as compared to Retired, Unemployed, or Disabled Customers.
3- Machine Learning
Before training our model, we examined the dataset and discovered that the “Effective to Date” column was not correctly interpreted as a date. To rectify this issue, we used papAI’s Cleaning feature to correctly parse the dates in this column.
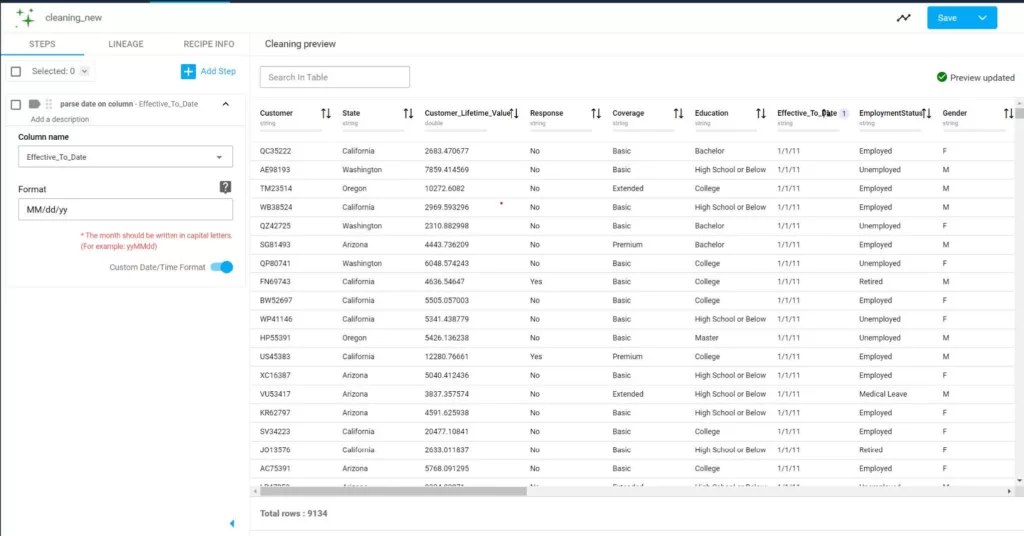
We will employ regression models to predict Customer Lifetime Value (CLV). Regression models are particularly well-suited for this task as they can effectively capture the relationships between the dependent variable (CLV) and a wide array of independent variables.
For this process, we will utilize papAI’s Machine Learning Lab, which offers a wide range of pre-built machine learning algorithms and model selection tools
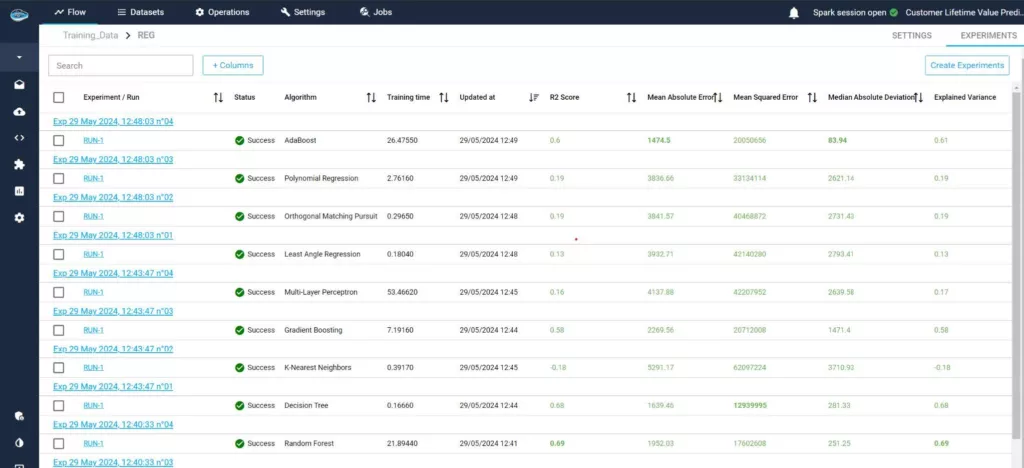
Thanks to the automated model evaluation metrics calculation feature of papAI, we can easily identify and select the best model. In this case, the Random Forest algorithm produced the best results. By clicking on the model, we can access further evaluation and detailed insights
4- Interpretability & Explainability (XAI)
In predicting customer lifetime value, understanding feature importance, counterfactuals, and explanations is essential for interpreting and comprehending the model’s insights. Feature importance involves assessing the impact of various factors like ‘Coverage,’ ‘Employment Status,’ and ‘Total Claim Amount’ on the model’s predictions, helping identify key drivers influencing overall customer value.
In this model, the features “Number_of_Policies” and “Monthly_Premium_Auto” had the most impact on the model.
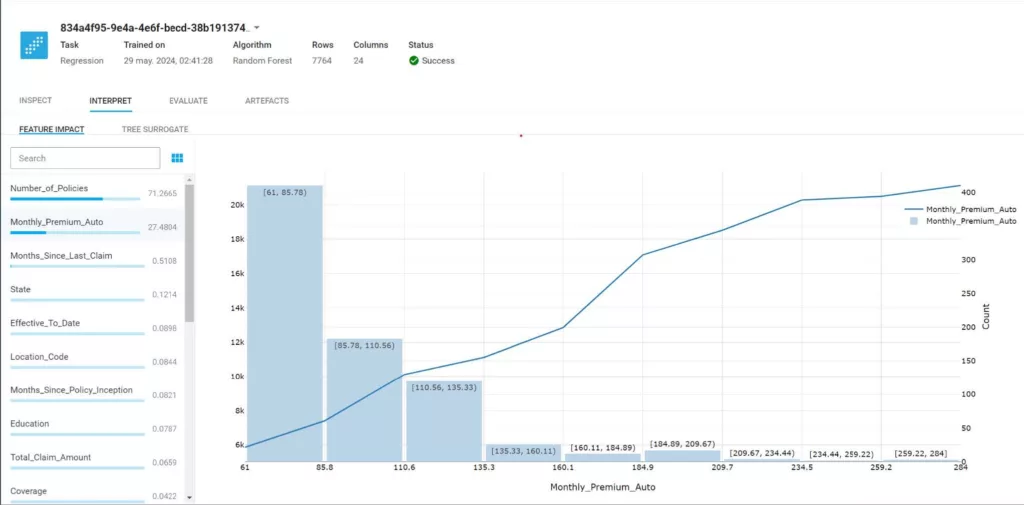
Counterfactuals
papAI’s innovative counterfactual explainer feature is particularly intriguing, as it enables us to understand the impact of each feature on a customer’s spending.
This tool empowers us to determine precisely which values of each feature influence customer spending and suggests adjustments to optimize revenue generation.
This advancement revolutionizes the sales process by equipping sales professionals with actionable insights on the most effective offers to propose to each individual customer.
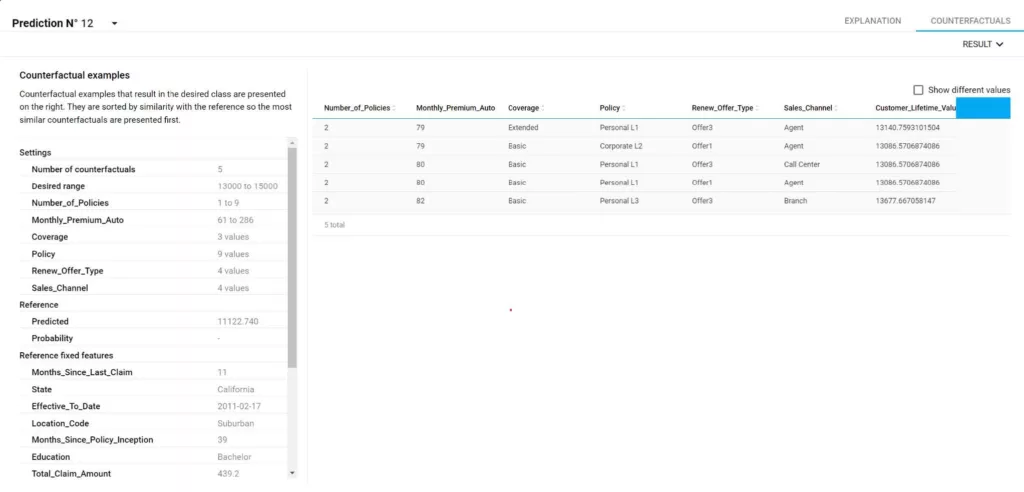
Conclusion
Concluding our study, we’ve derived several business recommendations based on our data analysis:
A) Targeting educated, married, employed customers residing in urban areas with mid-size vehicles could significantly enhance Customer Lifetime Value (CLV).
B) Failure to promptly resolve customer open complaints and failure to reduce claim amounts may lead to a decrease in Customer Lifetime Value (CLV).
C) Agents contributed approximately 38% of the value to the company, whereas call centers contributed only 20%. Hence, prioritizing agents over call centers for selling auto insurance to customers is advisable.
D) Factors positively influencing CLV include Monthly Premium and Number of Policies, while Open Complaints and Claim Amount have the potential to decrease CLV. These factors should be carefully managed to optimize customer value.
Create your AI-based Tool to Enhance Your Customer Life-Time Value Prediction using papAI
As we have seen, papAI is an all-in-one AI platform designed to help data scientists and business users deploy machine learning projects efficiently. From data preparation to model deployment, papAI covers the entire lifecycle of AI projects
Schedule your demo today. Our team of AI specialists is poised to help you craft a bespoke AI-based solution perfectly tailored to your organization’s unique needs.
Interested in discovering papAI?
Our AI expert team is at your disposal for any questions
How to Measure RAG from Accuracy to Relevance?
How to Measure RAG from Accuracy to Relevance? Retrieval-Augmented Generation...
Read MoreHow to Build a RAG System for Real-World Use?
How to Build a RAG System for Real-World Use? Large...
Read MoreAI in Defense: Smarter Aircraft Recognition with papAI 7
AI in Defense: Smarter Aircraft Recognition with papAI 7 The...
Read More