How Law Firms Use RAG to Boost Legal Research RAG...
Read MoreEmpowering Grids:
AI-Driven Microgrid Management Solutions
Table of Contents
ToggleConventional microgrid management approaches are encountering unprecedented challenges in a time when environmental issues are escalating and energy needs are increasing. The need for innovative solutions is more critical than ever as we stand at the cusp of the energy transformation.
Artificial intelligence solutions have become a driving force in addressing this use case. AI-powered microgrids can reduce energy consumption by up to 20% through optimized energy generation, distribution, and demand response. (Source: McKinsey).
Find out how papAI can improve the deployment of AI projects in the Renewable Energy Industry.
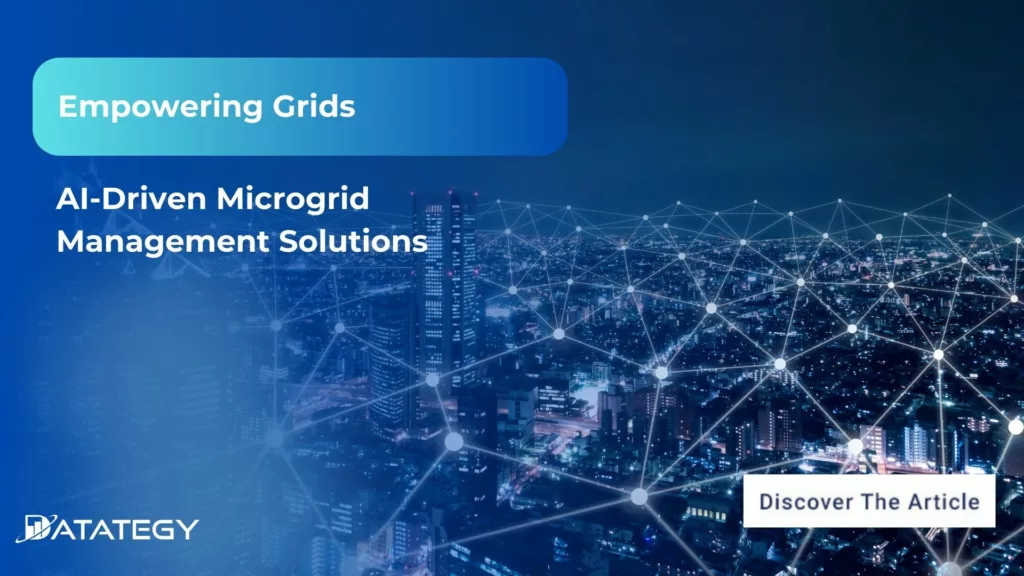
This article explores how intelligent technology can solve energy distribution, resilience, and sustainability issues. It also looks at why AI is being integrated into microgrid management.
What does Microgrid Management Solutions Mean?
Solutions for Microgrid Management are all-inclusive technology plans and setups intended to effectively manage and maximise microgrid operations. Small-scale energy systems, known as microgrids, may function both separately and in tandem with the larger power grid.
Integrated renewable energy sources, energy storage, and sophisticated control systems must all work together to effectively manage these small-scale power networks.
Using technology like artificial intelligence and smart grid capabilities, Microgrid Management Solutions seeks to improve sustainability, resilience, and dependability. Through the usage of these solutions, users may ensure a reliable and effective power supply by tracking, managing, and optimizing energy production and consumption inside the microgrid.
How do Microgrids Enhance Energy Independence and Sustainability?
Let’s look at the areas in which microgrids are used:
Integration in Renewable Energy
The capacity of microgrids to handle the unpredictability of renewable energy sources lies at the core of this change.
For example, the intermittent nature of solar and wind power depends on the weather and the time of day. Microgrids effectively handle this unpredictability because of their capacity to regulate energy supplies locally.
Microgrids can anticipate, modify, and optimise the integration of renewable energy in real-time, guaranteeing a steady and dependable power supply, thanks to sophisticated monitoring and control systems.
Support for Electric Vehicles (EVs)
The need for electric car charging on the current electrical grid is one of the main obstacles to the widespread adoption of electric automobiles.
In order to meet the demands of certain towns, companies, or even individual homes, microgrids provide localized charging infrastructure.
This strategy lessens the burden on the larger electrical system, averting any overload problems that would result from concentrated EV charging requests.
Support for Utility Provider
Thanks to the incorporation of microgrids into the energy ecosystem, utility companies now have the opportunity to diversify their portfolio with a range of energy sources, including renewable ones.
Microgrids are excellent at combining wind, solar, and other renewable energy sources with ease, giving the total energy mix flexibility and adaptability.
This diversity contributes to energy generation’s increased sustainability, which is in line with the overarching objectives of lowering carbon emissions and encouraging eco-friendly behavior.
Microgrid Management Solutions: Old vs. New
Aspect | Old Solutions | New Solutions |
---|---|---|
Control | Basic control systems with limited automation. | Advanced control systems leveraging AI for real-time optimization. |
Integration | Limited integration of renewable sources and energy storage. | Seamless integration of diverse energy sources and storage technologies. |
Reliability | Relatively lower reliability during grid disruptions. | Enhanced reliability through smart grid capabilities and predictive maintenance. |
Sustainability | Minimal focus on sustainability and environmental impact. | Prioritizes sustainability with increased use of renewable energy and reduced carbon footprint. |
Monitoring | Basic monitoring capabilities with limited data insights. | Advanced monitoring and analytics for in-depth data-driven decision-making. |
Empowering the Energy Industry through AI Revolution: The Critical Role of CDOs
Chief Data Officers (CDOs) in the energy industry face several challenges when it comes to adopting AI technologies. There is a cultural shift that must occur within energy companies to embrace AI technologies. CDOs must continue to explore and implement AI technologies to improve efficiency, reduce costs, and meet sustainability goals.
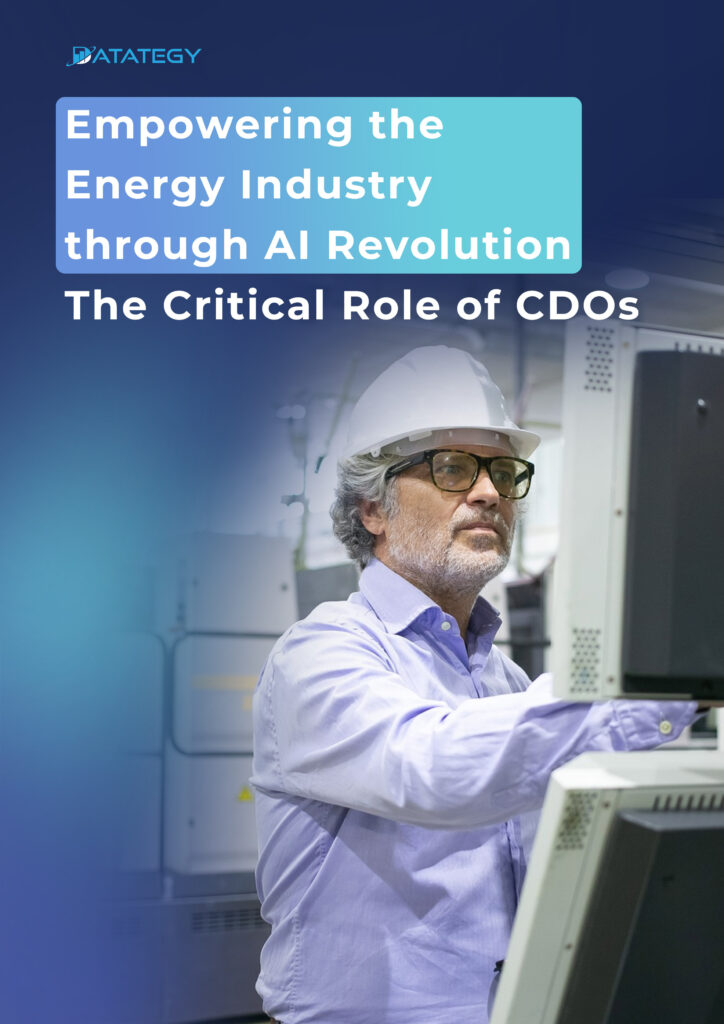
What are the Real-world Use Cases of Utilizing AI in Microgrid Management?
1- Predictive Maintenance:
The scalability of a microgrid is a crucial aspect to consider, especially as energy needs evolve and technology advances. AI plays a vital role in assessing the scalability of a microgrid as it can predict future energy needs and scrutinize current data.
On the other hand, this reactive strategy frequently results in downtime, higher maintenance expenses, and a higher chance of unplanned failures. By proactively monitoring, assessing, and forecasting the health of microgrid components, AI-powered predictive maintenance represents a paradigm leap.
Frost & Sullivan’s study forecasts that AI-driven Predictive Maintenance (PdM) could result in a 50-70% decrease in unexpected outages for distributed energy resources (DERs) within microgrids.
The core principle of Predictive Maintenance revolves around the continuous collection of real-time information from numerous sensors and integrated devices within the microgrid structure. These sensors, capable of gauging crucial parameters such as temperature, voltage, current, and vibration, provide a comprehensive insight into the state of elements like transformers, inverters, and generators.
The collected data powers advanced artificial intelligence (AI) algorithms, allowing the system to recognize trends, spot abnormalities, and forecast possible problems remarkably accurately.
2- Dynamic Load Balancing:
An adaptive approach to load balancing is required due to the dynamic nature of energy consumption, which is impacted by several factors like weather, time of day, and user behavior.
Because AI algorithms are always learning and adjusting to new patterns, they excel at managing these complexity. Because of its flexibility, the microgrid can best match its energy supplies to the current demand, reducing energy waste and increasing overall efficiency.
During moments of high demand, conventional load-balancing systems could find it difficult to avoid overloads, which could result in system malfunctions and disturbances. This problem is addressed by AI-driven Dynamic Load Balancing, which proactively redistributes energy supplies to avoid overloads by forecasting periods of high demand.
This predictive ability makes a substantial contribution to the microgrid’s stability and dependability, guaranteeing a steady supply of electricity even during times of peak demand.
3- Energy Forecasting:
AI-powered energy forecasting is the technique of projecting future energy use from a thorough examination of historical data and current environmental conditions.
A study by the National Renewable Energy Laboratory (NREL) demonstrated a 10% improvement in energy utilization for microgrids using AI-based forecasting compared to traditional methods
Sophisticated AI algorithms examine past consumption trends, meteorological conditions, and even outside events that might affect energy demand. The intricacy is in these algorithms’ capacity to identify subtle relationships among this heterogeneous dataset, allowing precise forecasts of future energy requirements.
Weather patterns are crucial for energy forecasting, particularly for microgrids that rely on renewable energy sources. Artificial intelligence (AI) systems employ meteorological data analysis to predict fluctuations in renewable energy output, including solar and wind energy. For example, if a day with little sunlight is forecast, the system can make the best use of the available resources by predicting a reduction in solar energy output and modifying the energy distribution accordingly.
The considerable improvement in accuracy is one notable statistic highlighting the impact of AI-driven Energy Forecasting. Leading energy organizations have undertaken research that show microgrids using AI for energy forecasting may achieve up to a 25% increase in accuracy on average when compared to traditional forecasting techniques.
4- Grid Resilience Enhancement:
A microgrid’s scalability is an important factor to take into account, particularly as energy demands change and technology develops. AI is essential for evaluating a microgrid’s scaling potential since it can forecast future energy requirements and analyse existing data.
This makes it possible for decision-makers to put in place a flexible infrastructure that can easily adjust to variations in demand, guaranteeing that the microgrid will continue to function well even when energy needs alter.
Moreover, the adaptability aspect of Grid Planning and Expansion is crucial in anticipating and accommodating emerging technologies and energy trends. AI algorithms continuously learn from ongoing operations and evolving energy landscapes, allowing the microgrid to adjust its planning strategies in real-time.
This adaptability ensures that the microgrid remains at the forefront of technological advancements and can readily integrate innovations such as new energy storage technologies or improved renewable energy sources.
5- Optimized Battery Storage:
Optimised battery storage offers advantages that go beyond improved operational effectiveness right away. Increasing battery longevity lowers the need for replacements more frequently, which lessens the environmental effect of battery manufacture and disposal.
Furthermore, effective energy storage utilisation supports overall grid stability by guaranteeing a steady supply of electricity even in the face of variations in demand or the availability of renewable energy sources.
AI algorithms examine past data to find use trends and environmental factors that affect battery efficiency before starting the optimization process. After that, these algorithms forecast future energy use and the availability of renewable energy, enabling the microgrid to proactively modify the cycles of charging and discharging to meet demands.
By ensuring that batteries run within ideal conditions and prevent stress on the cells, this predictive function extends the life of batteries.
6- Cybersecurity Measures:
In the context of microgrid management, the proactive aspect of AI-driven cybersecurity measures is very important. Microgrids frequently rely on networked sensors, devices, and control systems, which increases the attack surface available to cybercriminals.
AI systems keep a close eye on user activity, system interactions, and network traffic in order to spot any abnormalities that may point to a cyber attack. Rapid reactions are made possible by this real-time analysis, which also helps to avoid unwanted access and any disturbances to the microgrid’s operations.
A report by Darktrace claims its AI-powered cybersecurity platform helped a major energy company achieve a 60% reduction in security incidents within a year.
AI helps in the creation of frameworks for adaptive security. Artificial intelligence (AI) algorithms adapt cybersecurity measures in response to emerging patterns and strategies in cyber attacks.
This flexibility offers a future-proof approach for protecting vital infrastructure by ensuring that the microgrid’s defence mechanisms stay strong and efficient in the face of new cybersecurity threats.
Create your Custom AI tool to Improve your Energy Management Process with papAI
The use of advanced technology is crucial in enhancing energy management practices. Companies can tailor solutions to meet their specific energy management needs using papAI, our custom AI tool. This innovative platform allows customers to create personalized AI applications that boost the effectiveness and efficiency of energy-related operations.
Businesses can easily include artificial intelligence in their energy management strategy with the help of papAI, which provides a flexible and scalable framework for predictive maintenance, load forecasting, and dynamic load-balancing operations.
To find out how our bespoke AI solution may be adapted to meet the particular energy issues faced by your organization,
Schedule a demo now. Explore the potential for improved productivity, lower operating expenses, and sustainable energy methods. With papAI, you can easily access the future of energy management.
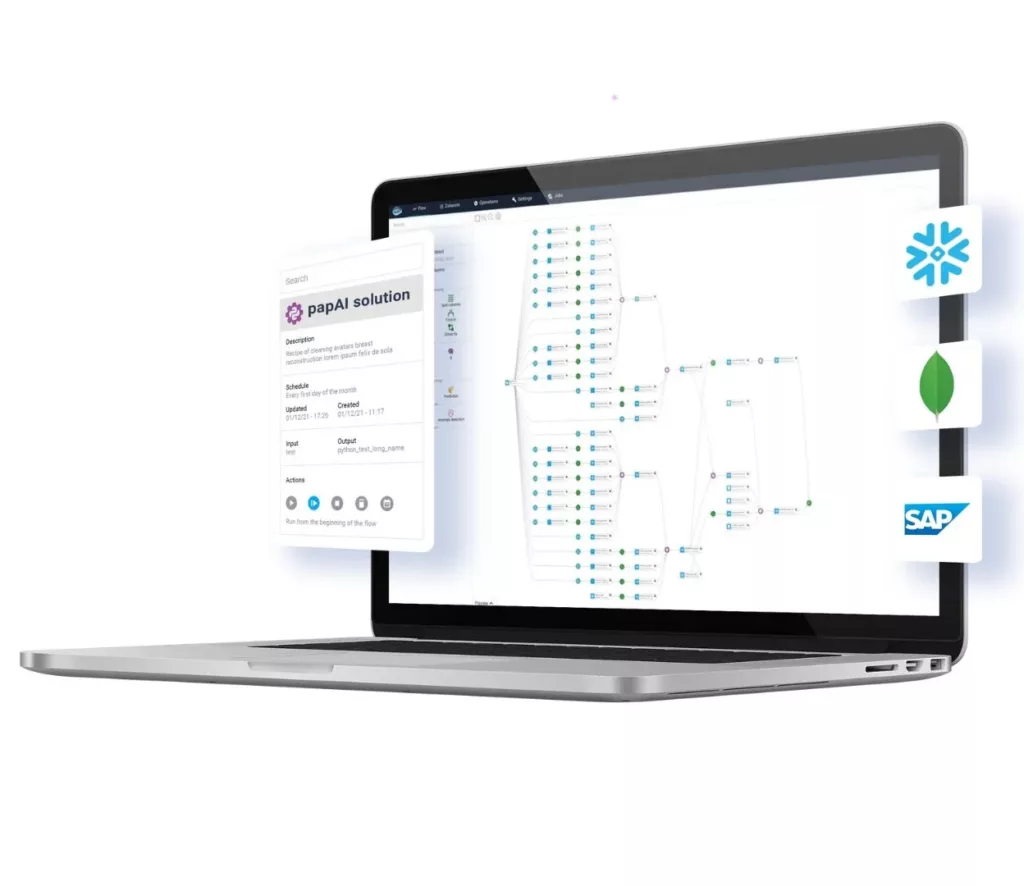
Interested in discovering papAI?
Our AI expert team is at your disposal for any questions
How RAG Systems Improve Public Sector Management
How RAG Systems Improve Public Sector Management The most important...
Read MoreScaling RAG Systems in Financial Organizations
Scaling RAG Systems in Financial Organizations Artificial intelligence has emerged...
Read MoreHow AgenticAI is Transforming Sales and Marketing Strategies
How AgenticAI is Transforming Sales and Marketing Strategies Agentic AI...
Read MoreSolutions for Microgrid Management are all-inclusive technology plans and setups intended to effectively manage and maximise microgrid operations. Small-scale energy systems, known as microgrids, may function both separately and in tandem with the larger power grid.
Microgrids enhance energy independence and sustainability by seamlessly integrating renewable energy sources, providing robust support for electric vehicles (EVs), and offering essential backing for utility providers, fostering a more resilient and eco-friendly energy ecosystem.
1- Predictive Maintenance.
2- Dynamic Load Balancing.
3- Energy Forecasting.
4- Grid Resilience Enhancement.
5- Optimized Battery Storage.
6- Cybersecurity Measures.