How does AI enhance and accelerate ESG Strategies? The first...
Read MoreThe Future of ESG: AI-Driven Streamlined Analysis
Table of Contents
ToggleToday, environmental and social governance are of considerable importance in all organizations, from retail and finance to government structures. According to the World Economic Forum, 80% of companies believe that AI is essential to achieving their ESG objectives. Thanks to the advent of data, teams can now analyze and generate meaningful information about their company’s ESG performance.
90% of investors consider ESG factors when making investment decisions (PwC).” This figure, highlighted by PwC, paints a compelling picture of the evolving landscape of finance, where environmental, social, and governance (ESG) considerations have moved from the periphery to the very heart of investment strategies
Find out how Datategy can improve the deployment of AI projects in your organizations.
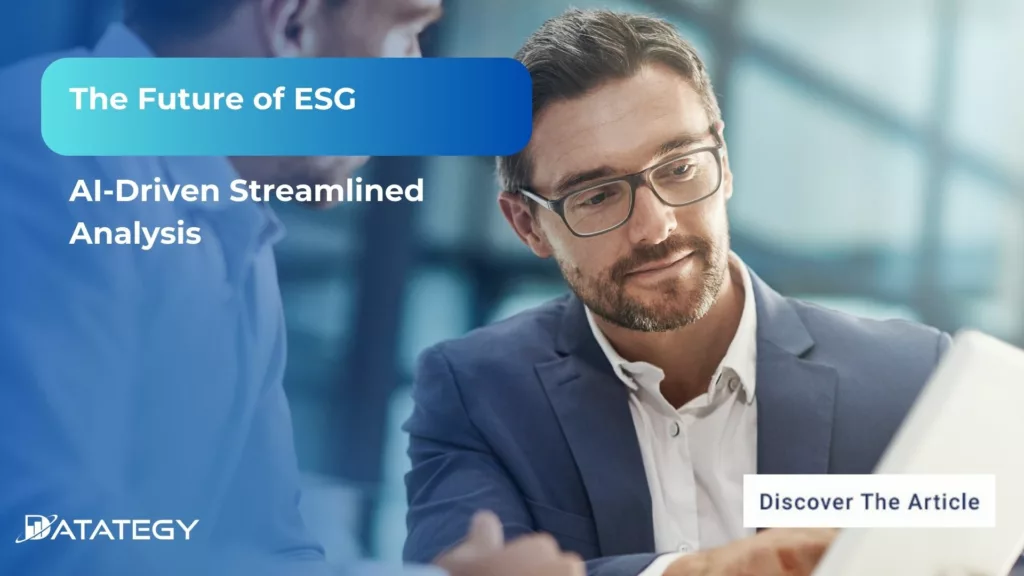
In this article, we’ll define what ESG businesses are and how AI can help sustainability teams streamline data analysis.
What is ESG?
The Environmental, Social, and Governance (ESG) approach is utilized to evaluate how well an organization performs on a range of ethical and sustainable business challenges. It also offers a means of quantifying commercial possibilities and risks in those domains. ESG investing is the practice of certain investors in the capital markets using ESG criteria to assess firms and assist in determining their investment plans.
An ESG program’s purpose is to guarantee accountability and the implementation of systems and procedures to manage a company’s impact, such as its carbon footprint and how it treats employees, suppliers, and other stakeholders, even though sustainability, ethics, and corporate governance are typically thought of as non-financial performance indicators. ESG programs support larger business sustainability initiatives, which put organizations in a position for long-term success through ethical corporate governance and sound business practices.
Environment, Social, and Governance are the Three Pillars of ESG
Environment (E): The influence of a firm on the natural environment is the emphasis of this pillar. It includes evaluating environmental aspects including carbon emissions, resource use, waste disposal, and attempts to reduce ecological impact. Under the “E” category, ESG analysis assesses a company’s environmental responsibility, green practices, and carbon footprint management.
Social (S): The “S” pillar explores how a business interacts with its constituents, such as its workers, clients, communities, and suppliers. Social concerns look at things like community involvement, diversity and inclusion, labor practices, and product safety. An organization’s dedication to morality and justice, as well as its contributions to the common good, may be ascertained by evaluating its social performance.
Governance (G): Governance is the study of an organization’s moral and structural foundation. It entails closely examining shareholder rights, CEO remuneration, board makeup, and financial reporting transparency. Companies that practice good governance are more likely to be well-managed, have established checks and balances, and be responsible to the public and their shareholders.
Benefits of using AI in ESG Analysis
1- Enhance Collaboration
A vital component of modern corporate sustainability and ethical responsibility is collaboration. In this procedure, artificial intelligence (AI) is essential because it offers a degree of accuracy and alignment that was previously difficult to attain.
AI is capable of sorting through enormous datasets, including market benchmarks, regulatory frameworks, historical performance records, and more, especially using machine learning algorithms. Organizations are able to create ESG targets that are not only comprehensive but also extremely detailed and quantifiable thanks to this intensive data analysis. These objectives are grounded in real-world possibilities and problems since they are based on data-driven insights.
Furthermore, AI’s strength is in its ability to take into account and incorporate the varied expectations and concerns of many stakeholders, including as local communities, investors, consumers, workers, and regulatory organizations. It can identify and match these stakeholder groups’ objectives and preferences with ESG goals. This alignment shows a sincere commitment to addressing the particular problems of each stakeholder category, going beyond simple goal-setting.
2- Integrating and Unifying data
A basic problem in ESG research is unifying data, which is generally dispersed across several sources and presented in different forms. Using AI-powered data integration solutions to solve this problem has several advantages.
First and foremost, the laborious process of gathering and centralizing data is made easier by ESG data integration via AI. Artificial intelligence (AI) reduces the possibility of errors and inconsistencies in data by integrating data from many departments, systems, and outside sources into a centralized and standardized structure. This standardized data repository serves as a single source of truth for ESG data, making it a useful resource.
Also, the standardized and combined data provide a strong basis for thorough ESG research. The act of comparing data points, recognizing patterns, and finding connections between various ESG measures is made easier by AI-driven data integration. Consequently, businesses are able to derive valuable conclusions from the combined data, facilitating better-informed choices on sustainability programs.
How to choose the best AI solution for your data project?
In this white paper, we provide an overview of AI solutions on the market. We give you concrete guidelines to choose the solution that reinforces the collaboration between your teams.
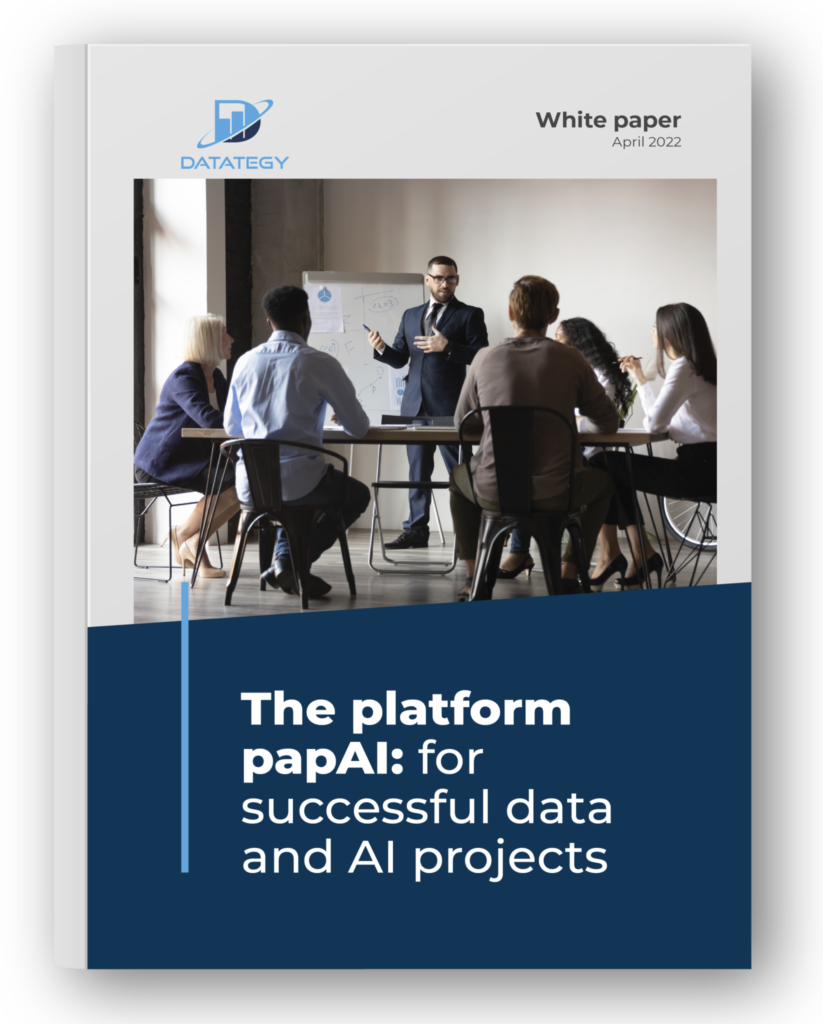
3- Monitor and Improve Project Performance
AI makes it possible to track ESG activities in real-time, resulting in a dynamic system that continuously monitors sustainability initiatives. Organizations have a thorough grasp of their success by consistently gathering and evaluating data pertaining to these efforts. This makes it possible to quickly identify when predetermined sustainability objectives are being exceeded and to take swift corrective action. Ensuring that minor difficulties do not become major ones is essential to preserving an organization’s overall sustainability objectives. This may be achieved by managing and reacting to concerns in real-time.
The automated and real-time performance updates that artificial intelligence (AI) may give improve accountability and transparency in an organization. External and internal stakeholders have access to the most recent information about how sustainability projects are doing. In today’s socially conscious corporate environment, transparency not only establishes confidence and trust but also shows a commitment to sustainability.
Benchmarking ESG performance against rivals and industry norms is made easier using AI. Through ongoing analysis and comparison of performance data, organizations are able to determine their current standing in relation to other players in their industry.
4- Optimize Forecasting Results
The capacity of AI to analyze large datasets, such as past ESG performance, market trends, and a variety of external factors, is one of the main benefits of forecasting. Through this thorough data analysis, organizations are able to forecast with confidence the future condition of their sustainability initiatives. AI is able to identify patterns and connections that human analysts would overlook, which can offer important insights into the anticipated outcomes of ESG projects.
In addition, AI’s forecasting models may incorporate a large range of internal and external inputs. It can take into account changes in the environment, regulations, market dynamics, and socioeconomic conditions. By taking a comprehensive approach to forecasting, companies may become more resilient to shifting business conditions by being ready to adjust to a variety of potential outcomes.
Allocating resources involves forecasting as well. Organizations may allocate resources more effectively by concentrating efforts on initiatives that have a higher chance of success when leveraging AI-driven insights. This optimization helps businesses reach their sustainability goals in an economical way by reducing the chance of squandering money on initiatives with little potential impact.
5- Explainability to More clarity of Decision-Making
Explainability is a crucial component of AI models, especially when it comes to ESG analysis. AI models frequently function as “black boxes,” which means that it might be difficult for humans to understand the inner workings and decision-making procedures of these algorithms. Still, there are attempts being made to improve AI explainability, and this project has a number of important advantages, especially when it comes to ESG research.
Developing trust and confidence in the ESG evaluation process is one of the main benefits of improving AI explainability. Understanding the methodology behind the calculation of ESG measures and ratings is made easier by transparent AI algorithms. Because of this openness, stakeholders—including the public, investors, and regulators—can have more faith in the impartiality and dependability of ESG.
Improving AI explainability also encourages improved organizational decision-making. Internal teams may decide on ESG tactics and advancements more intelligently when they comprehend the reasoning behind the methods used by AI models to arrive at certain suggestions or assessments. Better alignment with stakeholder expectations and international sustainability requirements follows from this.
What is the Future of AI-Powered ESG Solutions?
Generated AI is already guiding the waves in the industry by creating new content and automating the synthesis of knowledge and complex processes. In contrast to traditional models of machine learning that predict analysis and prediction, the generated AI can produce human text, images, code, and even annual reports. For example, ESG can automate sustainability reporting, analyze regulatory changes in real time, and generate strategic recommendations for companies that want to improve effectiveness. The ability to process large amounts of unstructured data makes it a powerful device that ensures compliance, reduces manual workloads and drives data control decisions.
Agentic AI signifies the next phase in the development of artificial intelligence, transitioning from content creation to autonomy. While generative AI focuses on producing content, agentic AI takes action. These AI systems can function independently, making decisions in real time, carrying out tasks, and adjusting to changing conditions without the need for constant human oversight. In the realm of ESG, agentic AI could be employed to oversee supply chains, automatically identifying sustainability risks and even initiating remedial measures. It can also regulate energy use in smart cities, adjusting resources in real time to enhance environmental outcomes.
How can papAI help you in your ESG performance analysis?
papAI is an advanced AI-driven platform designed to help organizations make sense of complex data, automate workflows, and enhance decision-making. When it comes to ESG (Environmental, Social, and Governance) performance, papAI offers cutting-edge tools that streamline data processing, improve transparency, and optimize sustainability strategies.
Automated ESG Data Processing
Managing ESG data from various sources can be overwhelming. papAI automates data collection, cleansing, and structuring, ensuring accuracy while freeing up resources for deeper analysis.
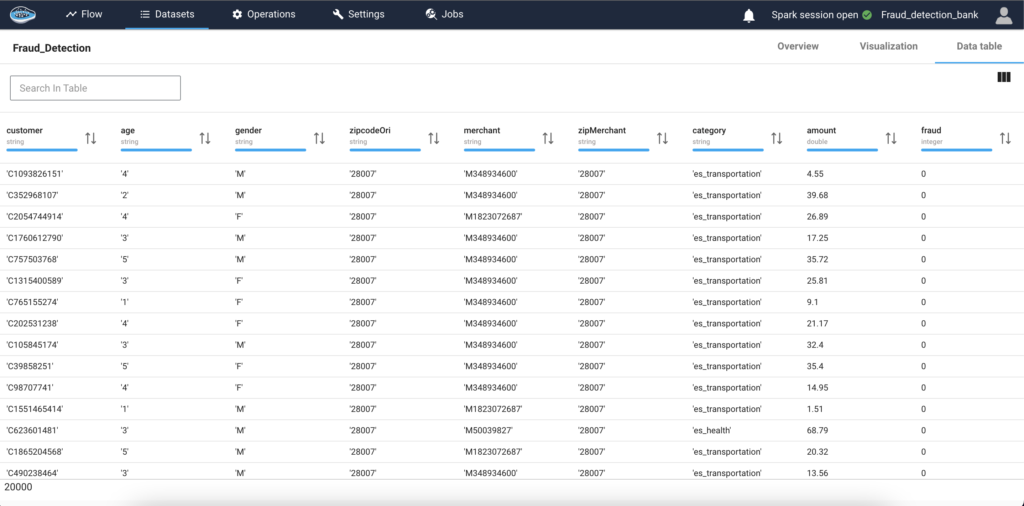
Predictive Analytics for Risk Management
papAI’s AI models identify potential ESG risks by analyzing historical and real-time data, enabling proactive decision-making to prevent compliance or reputational issues.
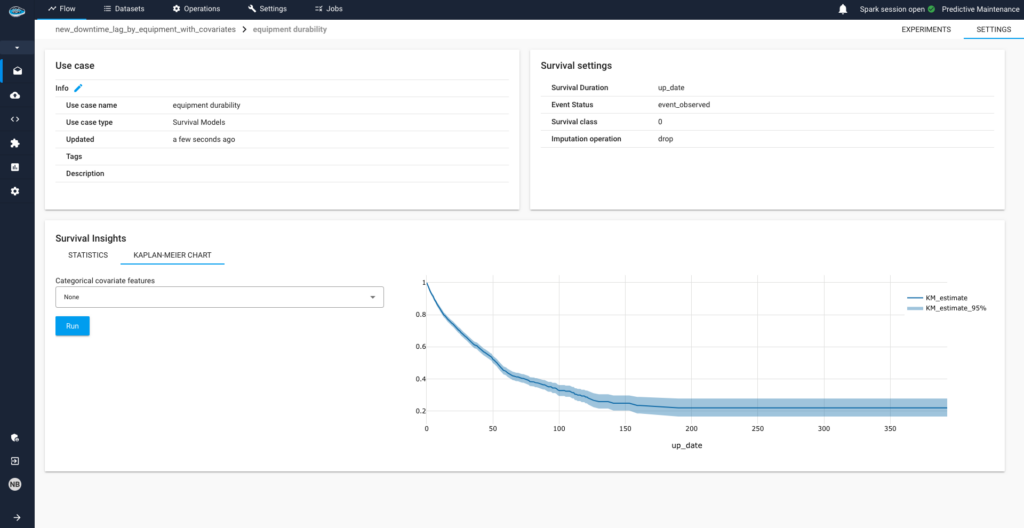
Enhanced Transparency & Reporting
Regulations demand accurate ESG reporting. papAI provides explainable AI models that help companies align with regulatory standards and avoid greenwashing.
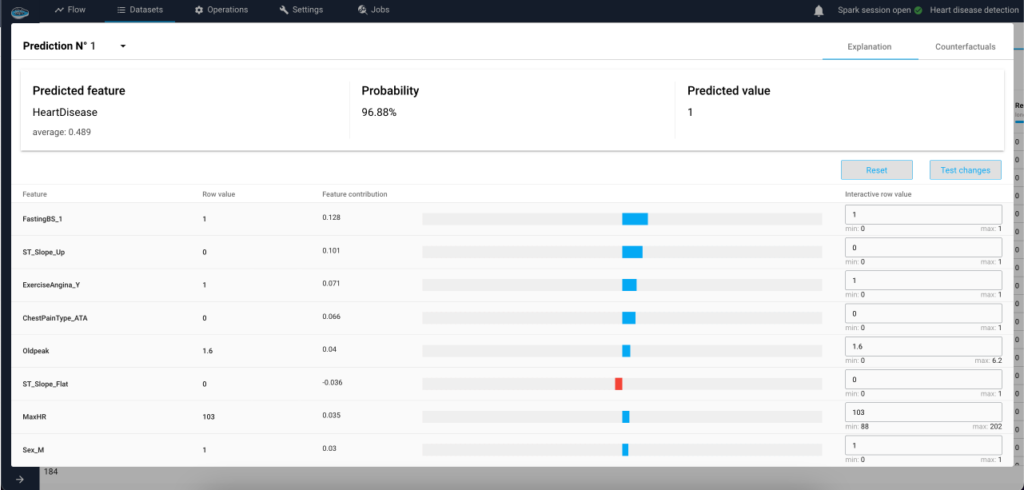
Build your AI-driven ESG analysis using papAI platform
To fully realize AI’s promise for improving ESG analysis, make use of papAI platform’s features. Create a custom AI-driven solution that meets your unique ESG requirements by utilizing cutting-edge machine-learning approaches to improve prediction accuracy and operational efficacy.
papAI makes data collection, cleansing, and visualization easier, which speeds up the deployment of AI projects. Our team of specialists will help you create a customized AI solution that perfectly matches your ESG goals for stakeholder satisfaction. Don’t miss this chance; arrange your demo now.
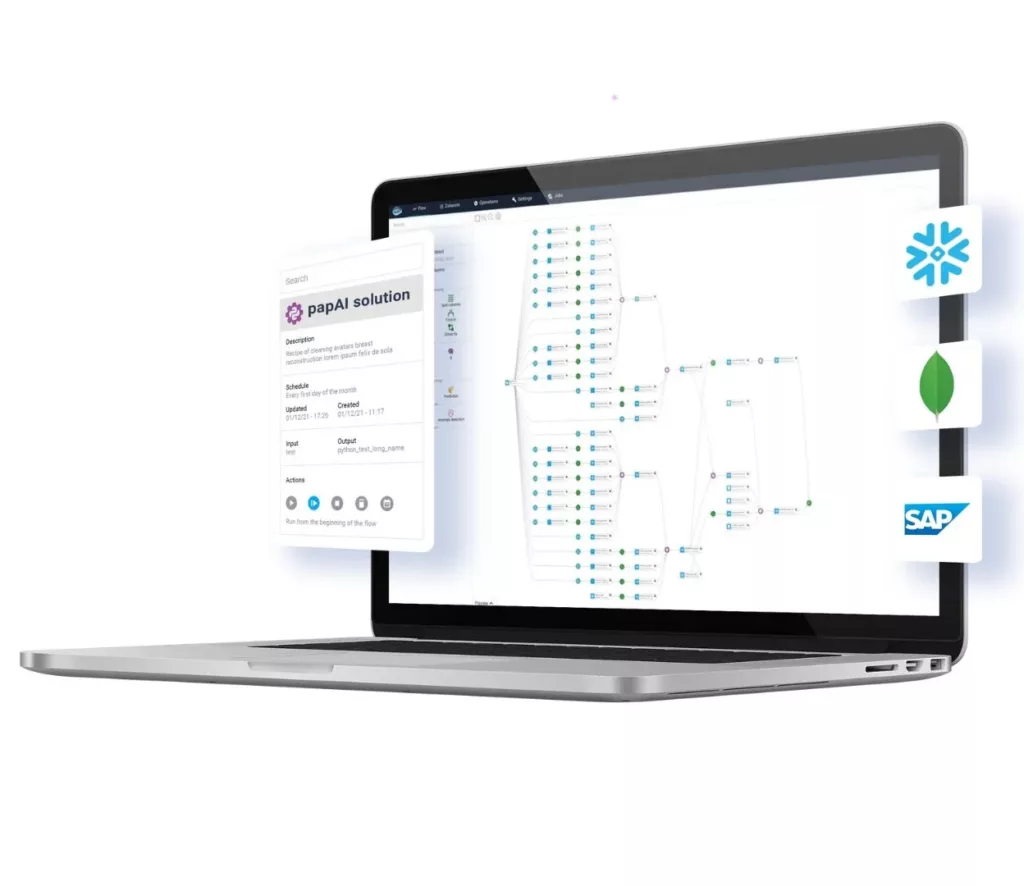
Average result achieved using papAI solution
Interested in discovering papAI
Our team of AI experts will be happy to answer any questions you may have
How Is AI Transforming Digital Twin in the Industry?
How Is AI Transforming Digital Twin in the Industry? Artificial...
Read More“DATATEGY EARLY CAREERS PROGRAM” With Julien Courteville
“DATATEGY EARLY CAREERS PROGRAM” With Julien Courteville Hello, my name...
Read MoreDatategy + Nedd Technologies: First Center of AI Excellence in USA
Datategy + Nedd Technologies: First Center of AI Excellence in...
Read More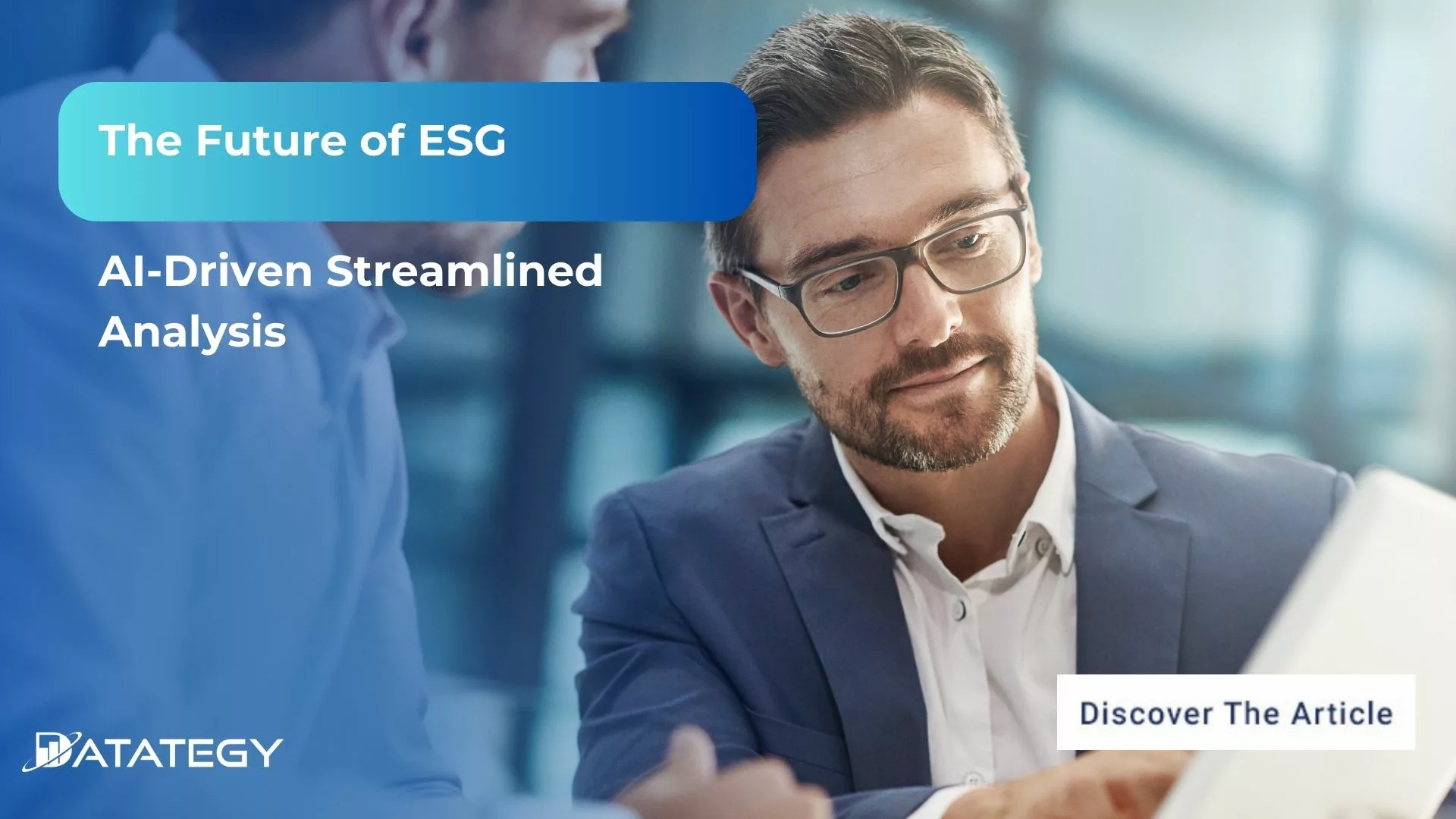
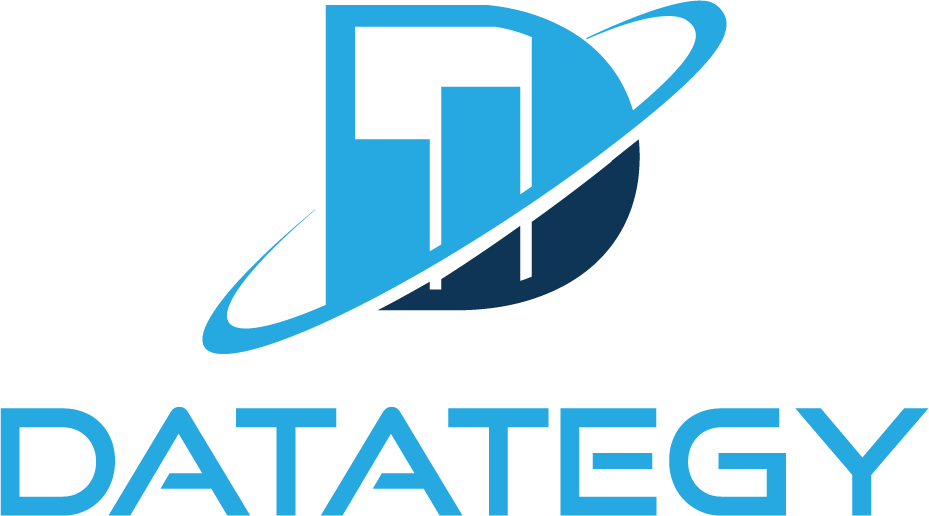