How does AI enhance and accelerate ESG Strategies? The first...
Read MoreCustomer Churn Prediction using AI: How papAI Improves Customer Churn Prediction in the Insurance Industry?
Table of Contents
ToggleCustomer loyalty is an essential aspect of long-term success in today’s fiercely competitive corporate environment. When it comes to keeping consumers, the insurance industry in particular confronts tough obstacles. Insurance companies must come up with creative strategies to keep their current client base given changing customer expectations, an abundance of alternatives, and strong competition marketing. AI platforms can be a viable solution to solve this problem. According to a survey conducted by Accenture, almost 79% of insurance executives believe that AI will revolutionize the way insurers obtain information about and interact with their customers.
Find out how papAI can improve the deployment of AI projects in the Insurance Sector here.
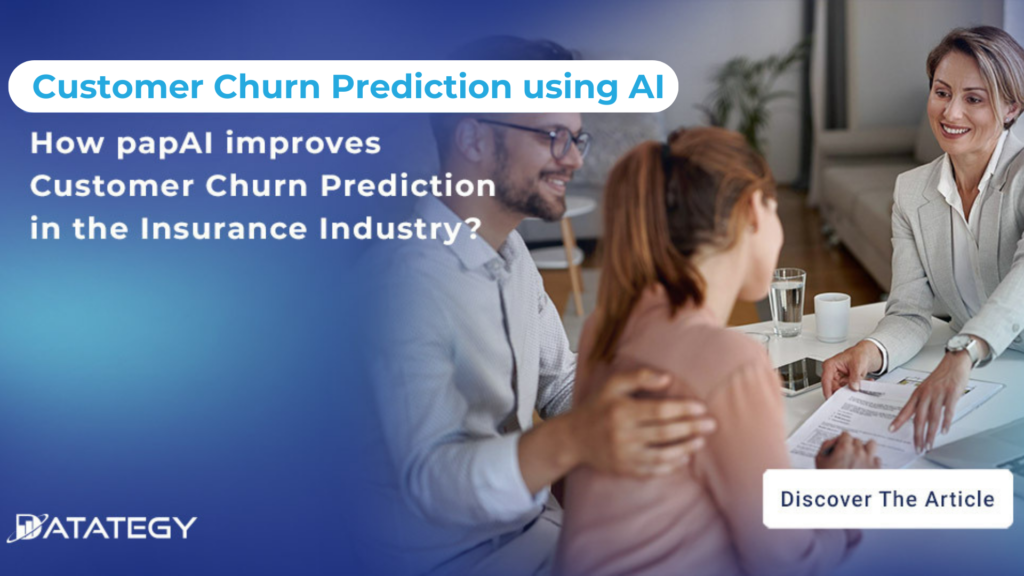
In this article, we’ll look at how papAI solution improves customer churn prediction in the insurance sector, enabling companies to increase retention rates and improve overall business performance.
AI's Adoption Overview in Insurance Industry
North America holds the largest share of the insurance analytics market, as it remains the largest insurance market by premium in addition to early adoption of technology advancements and analytics.
The US and Canada are expected to be the major revenue contributors in the North American insurance analytics market. Most of the insurance analytics vendors, including major players, have a direct or indirect presence in this region through SIs, distributors, and resellers.
The APAC region is expected to grow at the fastest rate in the global insurance analytics market during the period 2020-2023, due to its growing technology adoption rate.
Rapid economic developments, globalization, digitalization, and the increased adoption of cloud-based technologies are expected to drive the insurance analytics market in the APAC region.
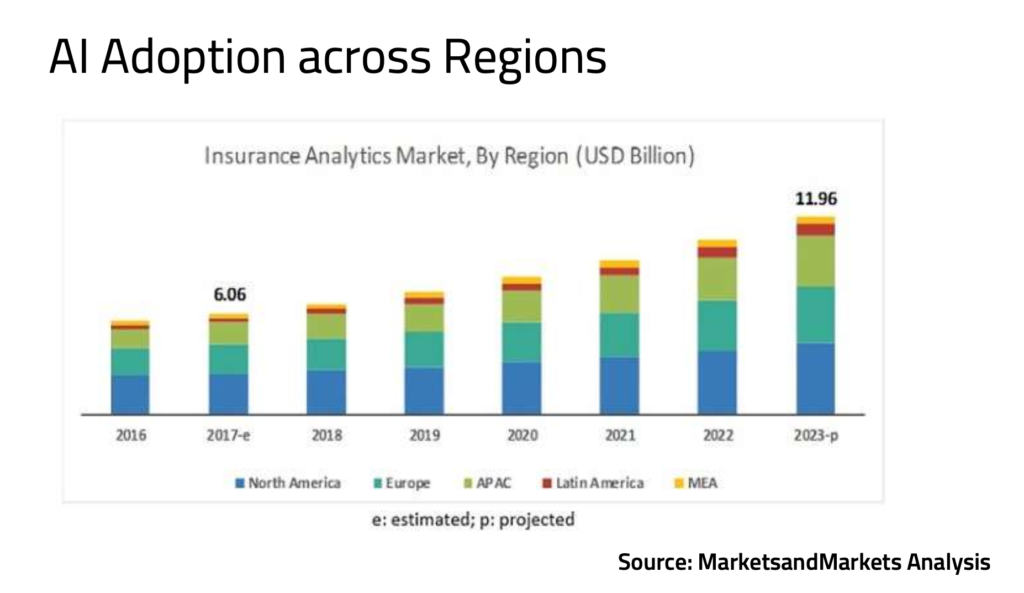
What Does Customer Churn Mean in the Insurance Industry?
- The churn rate is an indicator that enables you to figure out how many clients, users, or subscribers your company loses over a specific time period. In the banking and insurance industries, which historically had relatively low attrition rates, the attrition rate is commonly employed.
How do you determine your churn rate?
Actually, the calculation is as easy as dividing the total number of customers by the number of consumers lost. Although it may be done over any length of time, it is frequently measured over the course of a year.
Your churn rate will be 10% if you have 1000 clients and 100 of them quit. (100/1000)x100 = 10%
Factors Contributing to Customer Churn
Several factors can contribute to customer churn, and understanding these factors is essential for implementing effective customer retention strategies. Here are some key factors contributing to customer churn:
Poor Customer Service: Poor customer service is one of the main causes of customer churn. Slow response times, unhelpful support employees, unsolved problems, or trouble contacting customer service agents are a few examples of this. Customers are more susceptible to going elsewhere when they feel ignored or dissatisfied with the level of service they receive.
Poor Claims Experience: Customer retention and satisfaction are greatly impacted by the claims experience. Customer loyalty can be greatly impacted by slow claim processing timeframes, insufficient coverage, refused claims, or poor customer service. To increase customer retention, insurers must streamline the claims process, communicate clearly and promptly, and provide swift and efficient claim payouts.
Lack of Engagement: Customers are more inclined to leave a brand or business when they feel cut off from them. This sentiment may be exacerbated by a lack of contact, impersonal communication, or inadequate engagement possibilities. Customer engagement may be increased by developing strong relationships through focused marketing efforts, loyalty programs, and individualized experiences.
Competitive Pricing and Discounts: When it comes to insurance, the cost is a crucial consideration for customers. Customers may change insurers if they discover cheaper premiums or enticing offers elsewhere. By giving competitive price alternatives, insurers may help clients stay with them longer. This can be done by regular market analysis, competitive pricing tactics, and offering discounts or loyalty programs.
Principal Impacts of AI on the Insurance Industry
The benefits of implementing AI in insurance seem clear to stakeholders in this ecosystem. According to a study by Capgemini, 94% of insurance executives believe that AI will play a significant role in predicting customer churn and identifying cross-selling opportunities
1- Enhanced Accuracy
An important benefit of adopting AI in the insurance sector is increased accuracy. Advanced data analysis and predictive modeling capabilities of AI algorithms provide more precise forecasts and well-informed decision-making.
The capacity of AI to create prediction models from previous data is a crucial component of its increased accuracy. AI models may learn from past patterns and behaviors using methods like machine learning and deep learning to forecast future events. These models’ accuracy may be improved over time by continually learning from fresh data and adjusting to shifting consumer and industry patterns.
Real-time insights are yet another element that boosts accuracy. Because AI systems can analyze data in real-time, insurers can access current information and make judgments quickly. In fields like risk assessment, fraud detection, and claims processing, this is very helpful. Real-time analytics provide insurers the ability to react rapidly to new risks, spot fraud as it happens, and take preventative action to reduce prospective losses.
2- Early Warning Indicators Detection
In the insurance sector, early warning indications are crucial markers that may assist identify possible client churn, and AI dramatically improves the identification of these signs through sophisticated data analysis and predictive modeling. To find patterns that point to a higher chance of churn, AI algorithms examine extensive sets of client data, including policy specifics, claims history, interactions, and external variables. AI is able to spot outliers and highlights indications of unhappiness by tracking consumer behavior, such as decreased engagement or frequent contact with customer support.
Additionally, sentiment analysis of customer feedback and social media data enables the assessment of consumer sentiment and the identification of unfavorable feelings that may indicate future churn. By using past data to discover early warning indicators and forecast future churn, AI-powered predictive models allow insurers to be proactive in mitigating churn risks and increasing client retention rates. These proactive approaches include personalized interventions and bespoke retention strategies.
3- Reduced Bias and Subjectivity
Reduced subjectivity and bias are important advantages of using AI in the insurance sector since AI algorithms provide more unbiased and objective decision-making than human judgment alone. AI models can lessen the impact of individual biases, emotions, and subjective elements by making use of huge volumes of data and consistent algorithms.
This is crucial in fields where objective and just decision-making are crucial, such as risk assessment, claims processing, and underwriting. By reducing price, insurance coverage, and claims processing inequities, AI ensures that consumers are treated fairly based on data-driven insights rather than subjective opinions. AI helps to create a more transparent, responsible, and equitable insurance environment by lowering subjectivity and prejudice, which promotes consumer trust, legal compliance, and overall industry integrity.
4- Enhance Personalized Customer Retention Strategies
Insurance companies may get an in-depth understanding of the distinctive qualities, preferences, and behaviors of each client thanks to data analysis enabled by AI. Insurers may develop thorough client profiles by combining data from several sources, including as policy details, claims history, customer interactions, and external data. Individualized retention tactics are made possible by these profiles, which offer insights into risk profiles, life events, coverage demands, and communication preferences.
Also, Targeted communication and involvement with consumers are key components of personalized retention tactics. Insurance companies may discover particular client groups with comparable requirements, preferences, or behavioral patterns by using AI-driven customer segmentation. This makes it possible to create customized communication campaigns using a variety of channels, such as social media, email, or mobile apps. Insurance providers may improve consumer engagement, solve particular issues, and emphasize the benefits of their services by sending personalized communications, offers, and content.
Case Study: How papAI Solution can Enhance Customer Churn Prediction?
1- Dataset Presentation
This use case focuses on developing a machine learning model that leverages 16 distinguishing factors (feature_0 to feature_15) to predict customer churn in the insurance industry. By accurately identifying potential churners in advance, insurance companies can devise proactive strategies to prevent customer attrition and enhance long-term customer relationships.
The study aims to highlight the significance of utilizing data-driven approaches in predicting customer churn and its implications for the insurance industry’s growth and profitability.
To prepare the data for analysis, we perform One-hot encoding, which handles categorical variables by creating binary columns that represent the categories. Instead of assigning arbitrary numerical values to categories, it creates binary columns that explicitly indicate the presence or absence of a particular category in each row.
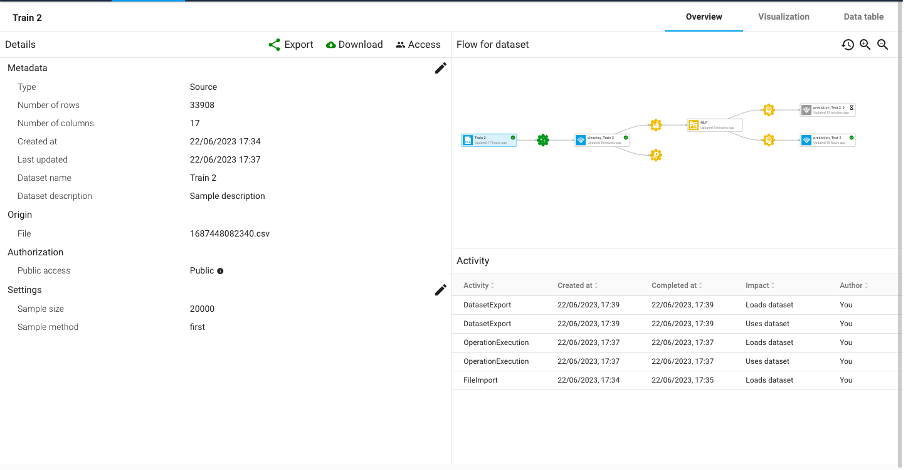
2- Data Analysis and Preparation
To prepare the data for analysis, we perform One-hot encoding, which handles categorical variables by creating binary columns that represent the categories. Instead of assigning arbitrary numerical values to categories, it creates binary columns that explicitly indicate the presence or absence of a particular category in each row. This helps to avoid introducing unintended ordinality assumptions, which could mislead the model.
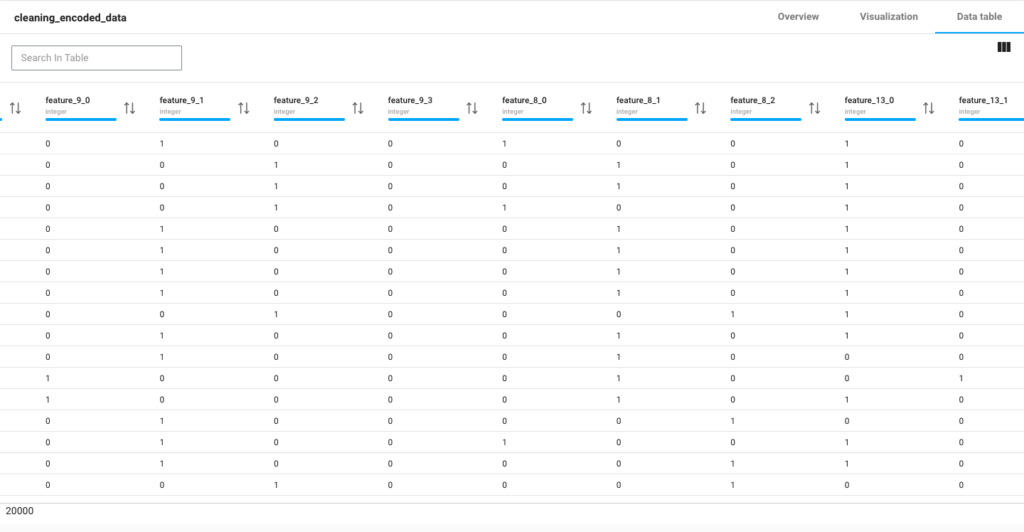
3- Model Training
In the training phase, we utilized various models available in papAI Solution to build and evaluate our Insurance Churn prediction model. Papai provides a wide range of machine-learning algorithms and techniques that are suitable for classification tasks.
To begin, we trained multiple models using the training set obtained from the stratified split. These models included popular classifiers such as logistic regression, decision tree, random forest, and support vector machines… Each model was trained on the input features and the corresponding binary target variable. We also used the data augmentation algorithm SMOTE (Synthetic Minority Over-sampling Technique) which addresses the issue of class imbalance by generating synthetic samples for the minority class, thereby balancing the class distribution.
The data augmentation techniques available in papAI included random over-sampling, SMOTE (Synthetic Minority Over-sampling Technique), ADASYN (Adaptive Synthetic Sampling), Borderline SMOTE, SWIM maha (Synthetic With Interpolation of Minority using Mahalanobis distance), and SWIM RBF (Synthetic With Interpolation of Minority using Radial Basis Function).
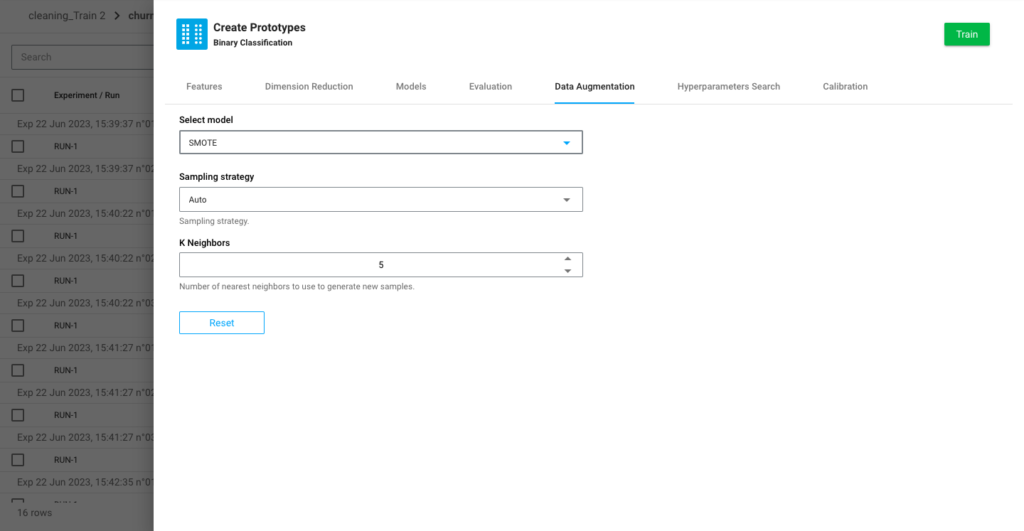
Based on the evaluation results, we selected the model that demonstrated the highest performance. Selecting the model with the best performance ensures that our deployed solution has the highest potential to effectively predict whether the insurance company will lose a customer or not.
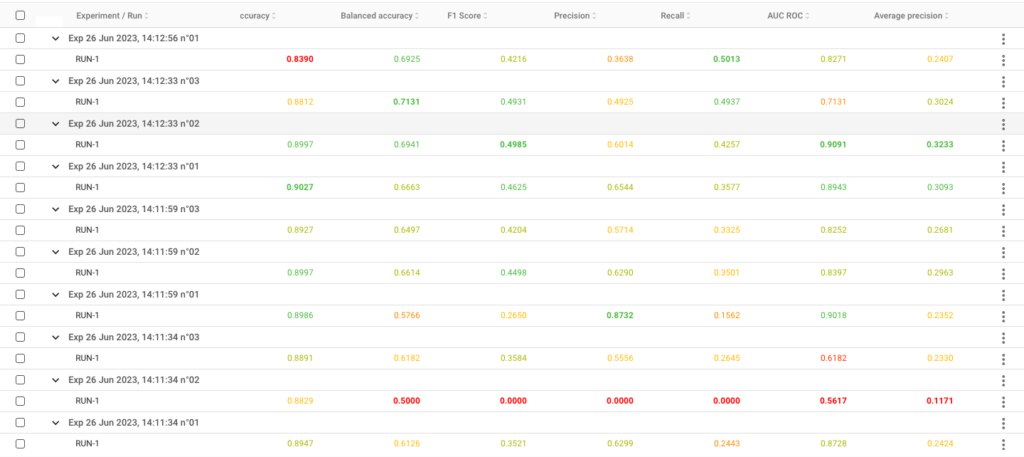
4- Interpret the Predicted Outcome
After deploying the model chosen and making the prediction on the test set we can use the counterfactuals artifact proposed by papAI solution which allows us to choose a row and manipulate the different features to see how the model will react depending on each variable as you can see in the figure below, we changed the value of some feature and turned the prediction from 54,79% false to 89.97 true.
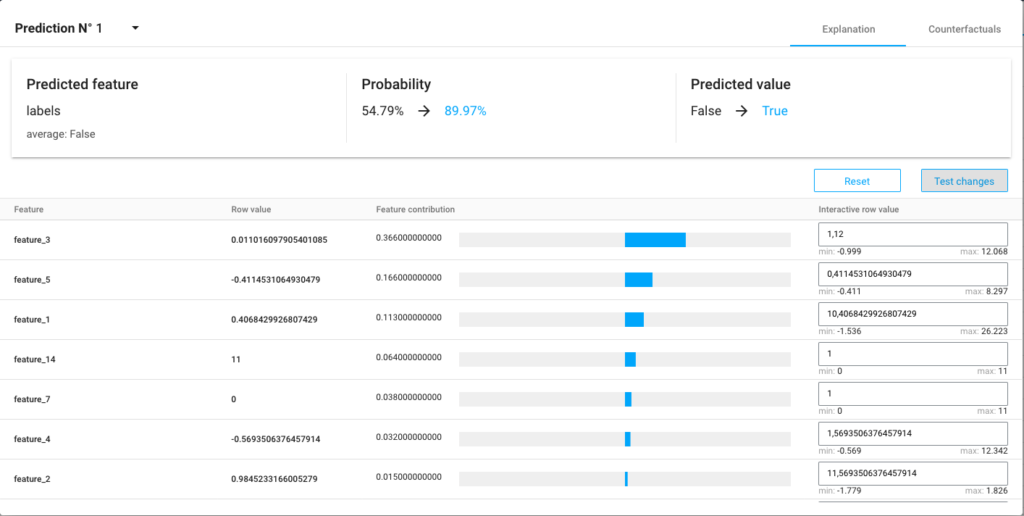
By closely examining the feature impact, we can pinpoint the factors that have the greatest impact on the model’s performance. Variables with higher percentages indicate a significant influence on the model’s prediction, underscoring their crucial role in the learning process. These key variables should be the primary focus for adjustments and subsequent optimizations.
On the other hand, variables with lower percentages can be considered to have a relatively weaker impact on the model’s performance. This could be due to their less informative nature or complex interaction with other variables in the dataset.
Improve the Accuracy of Customer Churn Prediction Projects by Building your Own AI-based Tool with papAI solution
Through a data exploration, cleaning, and visualization procedure that may speed up the deployment of AI initiatives, the papAI solution enables explanation and transparency of outcomes. Book your demo now. Our team of experts can help you build a custom AI-based tool that meets your organisation’s unique needs.
Interested in discovering papAI?
Our commercial team is at your disposal for any questions
How Is AI Transforming Digital Twin in the Industry?
How Is AI Transforming Digital Twin in the Industry? Artificial...
Read More“DATATEGY EARLY CAREERS PROGRAM” With Julien Courteville
“DATATEGY EARLY CAREERS PROGRAM” With Julien Courteville Hello, my name...
Read MoreDatategy + Nedd Technologies: First Center of AI Excellence in USA
Datategy + Nedd Technologies: First Center of AI Excellence in...
Read More