Scaling RAG Systems in Financial Organizations Artificial intelligence has emerged...
Read MoreUnlocking the Black Box: How Explainability (XAI) Drives AI Trust in Insurance Industry
Table of Contents
ToggleNowadays, every sector has access to a wealth of data that can be used to get insights and conduct business more effectively. They may utilize that data and make accurate judgments using artificial intelligence (AI) to increase performance and customer happiness. In a similar vein, several applications of AI are being used by insurance firms. AI adoption enhances productivity, profitability, and customer satisfaction. According to a report by PwC, 74% of insurance CEOs believe that AI will significantly impact how they operate, and 52% are exploring the benefits of AI in improving customer trust and loyalty.
Find out how papAI can improve the deployment of AI projects in the Insurance Sector here.
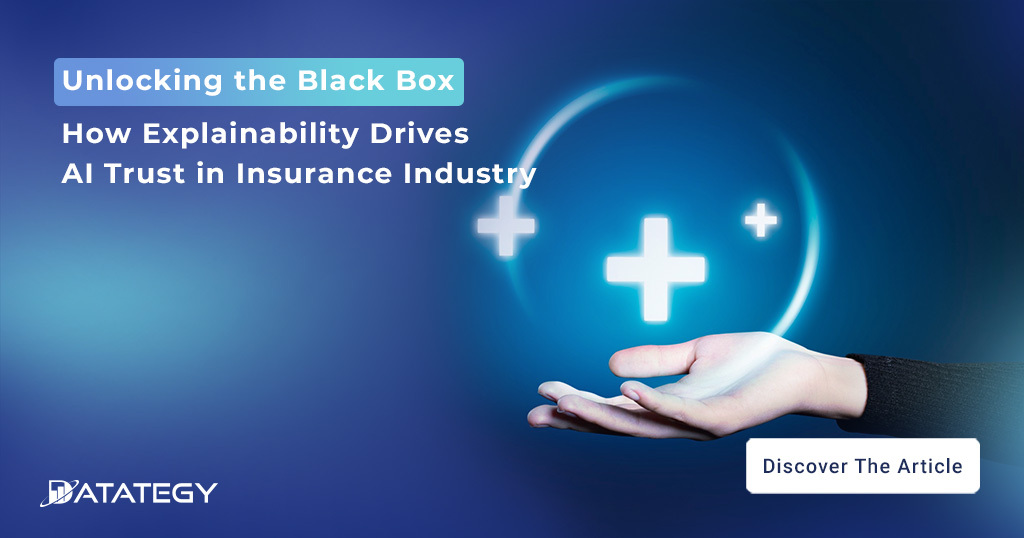
The first AI platforms succeeded in solving a few use cases but did not provide the ability to understand how these results were created, leaving users with a feeling of mistrust. In this article, we will discuss the evolution of the black box concept and the advent of explainable AI (XAI).
What does the term "black box" mean in the field of AI?
The lack of transparency and interpretability in the decision-making processes of AI systems is referred to as the “black box” phenomenon in AI. The reason it is referred described as a “black box” is because people find it difficult to understand how the algorithms operate within. While AI models may accurately forecast the future and make judgments based on a wealth of data, it can be difficult to grasp how they do so.
Deep neural networks and other conventional machine learning algorithms function through intricate mathematical calculations. They examine the unstructured information to discover patterns, then classify or predict things based on those patterns. However, because of the many layers of computations and transformations contained inside these models, it is challenging for people to comprehend the fundamental concepts driving the choices made.
Specifically in the field of insurance, it is essential to understand the black box phenomenon. The owners must understand the criteria taken into account when calculating their premiums, comprehend the justifications for approving or rejecting claims, and have faith in the fairness of the decision-making process. Explanations that help insurance professionals validate AI-driven conclusions and resolve any biases or inaccuracies are helpful. Transparency is required by regulators to guarantee adherence to laws and moral principles.
What does explainability in AI (XAI) mean?
Explainability in AI, or XAI, is the ability of AI systems to clearly and understandably explain how they arrive at their decisions. XAI is essential for fostering confidence in and adoption of AI technology, particularly in highly regulated sectors like insurance. XAI gives stakeholders the ability to understand, validate, and contest the decisions made by AI systems by opening the black box.
Demystifying AI: A Comprehensive Guide to Key Concepts and Terminology
This guide will cover the essential terminology that every beginner needs to know. Whether you are a student, a business owner, or simply someone who is interested in AI, this guide will provide you with a solid foundation in AI terminology to help you better understand this exciting field.
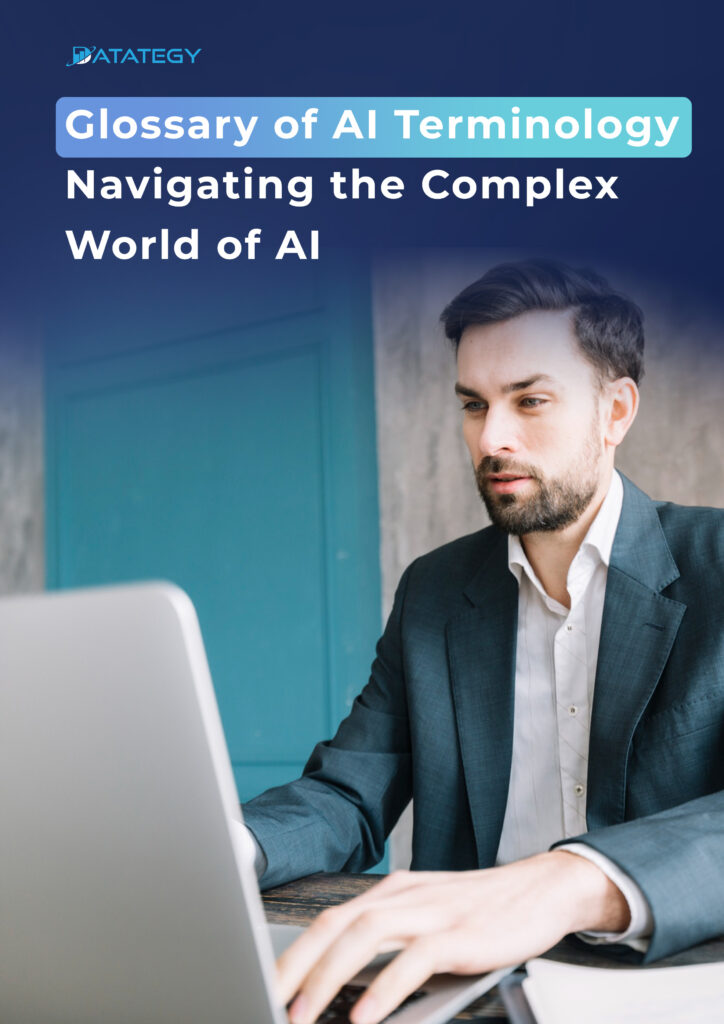
XAI applications in the insurance sector
1- Enhancing Transparency in Claims Processing
Leveraging explainability in AI (XAI) in the insurance sector requires improving transparency in the claims processing process.A report by McKinsey & Company states that insurers leveraging AI and advanced analytics in claims processing can achieve up to a 30% reduction in costs and a 50% improvement in processing time. Insurers may give policyholders a comprehensive grasp of the decision-making process that led to claim results by implementing XAI approaches. Policyholders can learn more about the variables, information, and algorithms employed by AI systems to evaluate claims by having transparent claims processing. This comprehension promotes trust, eliminates uncertainty, and always worries about biased or unjust claim judgments.
Policyholders have access to thorough justifications for claim denials or changes through XAI. They may more clearly understand the particular standards or rules that determined the result, increasing transparency and lowering annoyance. This openness also equips policyholders to have educated conversations with insurers in order to clarify any ambiguities and perhaps find a solution. In the end, being able to comprehend claim judgments enhances the bond between policyholders and insurers, increasing consumer satisfaction and loyalty.
2- Ensuring Fairness and Bias Mitigation
The capacity to guarantee fairness and minimize biases is one of the key benefits of integrating AI and explainability (XAI) approaches in claims processing. The promise of objectivity and consistency in decision-making processes is enhanced by the use of AI algorithms. However, without careful planning and supervision, these algorithms may unintentionally reinforce previous data biases or reflect intrinsic limits.
In a survey conducted by the National Association of Insurance Commissioners (NAIC), 81% of insurance regulators identified fairness and bias mitigation as important considerations when evaluating the use of AI in the insurance industry. Insurance companies may proactively address biases in claims processing by using XAI to detect and correct them. With the use of XAI approaches, insurers may get insight into the variables impacting claim judgments, comprehend the underlying systems, and identify any biases that may exist. Because of the openness, insurers may create plans to reduce prejudice and serve all policyholders fairly.
3- Increasing Underwriting and Risk Evaluation
Underwriting and risk assessment have historically required substantial manual data processing, which may be time-consuming and prone to human mistakes. Contrarily, AI computers can quickly scan enormous volumes of data and spot complicated patterns that a human underwriter could miss. This enables insurers to do more thorough and accurate risk assessments, which enhances pricing options and enhances risk management.
Insurers may acquire thorough justifications for underwriting choices by utilizing XAI and AI. These justifications make the process more open and trustworthy by assisting both underwriters and policyholders in understanding the variables driving risk evaluations. Additionally, using XAI approaches, insurers may spot and address biases that can be present in underwriting models, assuring fairness and minimizing risk discrepancies.
Additionally, AI allows insurers to use non-traditional data sources for risk assessment, moving beyond established criteria like credit scores and claim histories. This entails integrating information from non-traditional sources such as social media, IoT devices, and others. AI systems may give a more thorough and accurate knowledge of individual risks by analyzing a wider variety of data, leading to more individualized underwriting and more equitable pricing.
Features of papAI solution for Explainability: A Deep Dive into the Specific Tools and Features of papAI
A- Feature Importance Analysis
The explainability of AI models depends on knowing how important input features are in relation to one another. papAI solution provides tools for feature importance analysis that show the impact of specific aspects on the decisions made by the AI system. Users may understand what influences the model’s predictions by seeing the effects of each feature. In addition to helping to explain the decision-making process, this information also aids in locating any potential biases and discriminatory tendencies that could be present in the data.
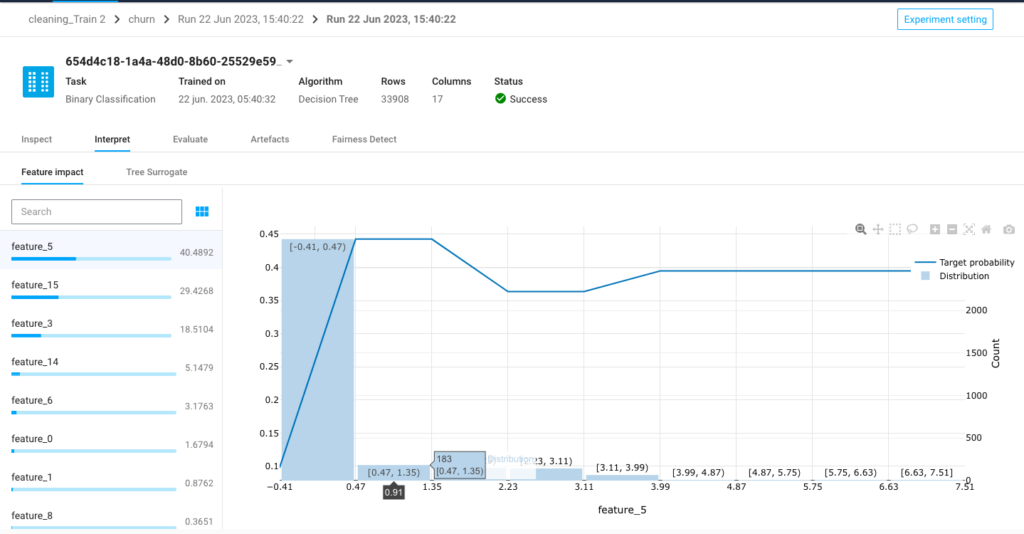
B- Counterfactual Explanations
PapAI offers counterfactual explanations in addition to explanations for AI judgments. Through the manipulation of input variables and observation of the subsequent changes in the AI model’s predictions, this tool enables users to investigate hypothetical possibilities. Users can explore alternate routes that can produce different outcomes and get a greater knowledge of how various aspects impact the outcomes by interactively modifying the inputs. Stakeholders are better equipped to assess the fairness and robustness of AI systems thanks to counterfactual explanations.
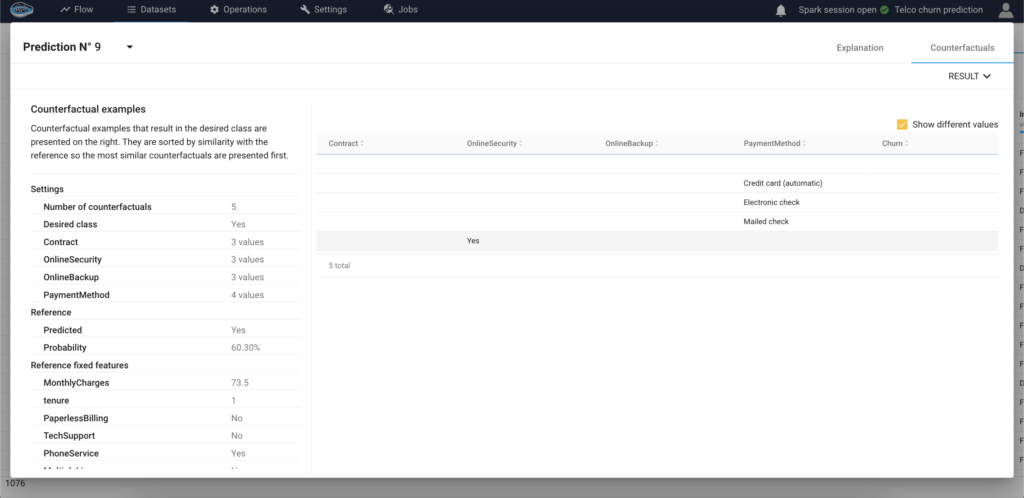
Create your own AI-based tool with papAI solution to deploy, monitor and maintain your AI projects
With papAI solution, you can use AI to improve the explainability of your AI projects in your company. You have the chance to design your own AI-based tool using papAI that is suited to your particular requirements. Utilizing cutting-edge machine-learning techniques will greatly increase the precision and effectiveness of your prediction procedures.
papAI solution helps to explain and make transparent the results through a process of data mining, cleaning, and visualization that can accelerate the deployment of AI projects. Book your demo now. Our team of experts can help you create a custom AI-based tool that meets the unique needs of your organization.
Interested in discovering papAI?
Our commercial team is at your disposal for any questions
How AgenticAI is Transforming Sales and Marketing Strategies
How AgenticAI is Transforming Sales and Marketing Strategies Agentic AI...
Read More“DATATEGY EARLY CAREERS PROGRAM” With Abdelmoumen ATMANI
“DATATEGY EARLY CAREERS PROGRAM” With Abdelmoumen ATMANI Hello, my name...
Read MoreIntroducing the Error Analysis Tree: A Smarter Way to Improve AI Models
Introducing the Error Analysis Tree: A Smarter Way to Improve...
Read More