How Law Firms Use RAG to Boost Legal Research RAG...
Read MoreEnhancing Risk Management with papAI Solution: AI-Based Financial Distress Prediction
Table of Contents
ToggleFinancial distress is the state in which a business cannot pay its creditors when its debts are due. Bankruptcy may eventually result from lengthy and severe economic difficulties. In the past, experts’ subjective opinions were mostly used to judge if a company was in a financial crisis. The results are inconsistent, cannot be independently verified, and heavily rely on the expertise of the experts, which is only one of the numerous problems with this expert-based strategy. To increase consistency and accuracy, many technologies, such as machine learning, have been developed.
Find out how papAI can improve the deployment of AI projects in the Banking Sector here.
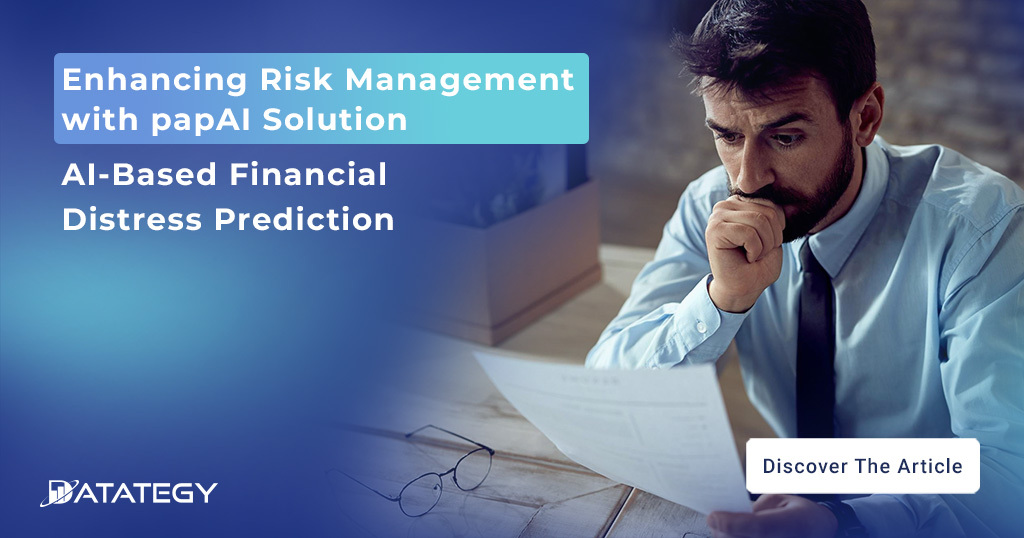
Financial Distress: What It Is and Why?
Definition & Characteristics
A scenario in which a big or small organization is having serious financial problems or is at imminent risk of going insolvent is referred to as financial distress. It shows that the company is unable to produce enough cash flow or get the required capital to meet its liabilities, which is a sign of financial instability and vulnerability. Poor financial management, economic downturns, excessive debt, or disruptive industry changes are just a few examples of internal and external causes that frequently combine to cause financial distress.
Financial Distress Characteristics
Issues with Liquidity: A company’s capacity to swiftly convert its assets into cash is referred to as its liquidity. Financially troubled organizations sometimes struggle with liquidity issues since there aren’t enough cash immediately accessible to cover immediate commitments. Daily operations, stakeholder interactions, and financial crises can all be hampered by a lack of cash.
Decreasing Profitability: Companies in financial trouble frequently show a drop in profitability. Reduced profitability can be caused by a variety of variables, including decreased sales, shrinking market share, and increased expenses. These obstacles may lead to decreasing profit margins, decreased earnings, and trouble producing sustainable profitability.
High debt levels: Debt accumulation is frequently a symptom of financial troubles. When a business finds it difficult to satisfy its obligations, it may turn to borrowing or opening credit lines to make up for sudden cash flow gaps. A negative feedback loop that exacerbates the distressed situation can be created by increasing debt levels, which can further strain the organization’s financial condition.
Low Investment Capacity: the situation of companies in financial difficulty results in a very low investment capacity due to their ineligibility for bank loans and investor investment.
Operational Challenges: A company’s capacity to run efficiently might be affected by financial difficulty. Financial difficulties can have a number of effects, including decreased financing for crucial tasks, layoffs, impaired product or service quality, and insufficient expenditures in innovation. The company’s competitive advantage and viability may be further damaged by these operational difficulties.
Organizational Financial Distress Contributing Factors
Organisations in a variety of industries face serious threats to their stability and existence due to financial instability. Understanding the elements that might lead to financial distress is crucial for managing and mitigating this risk. Companies may proactively address these issues and put policies in place to protect their financial stability by being aware of these aspects. We shall examine a few significant elements that might cause financial trouble in organizations below:
Market volatility and economic downturns: The financial well-being of organizations can be significantly impacted by market volatility and economic downturns. Reduced consumer spending, lower demand for goods and services, and narrowing profit margins can all cause businesses to fall into financial trouble very fast during recessions. Established business models may also be disrupted by market fluctuations, shifts in customer tastes, or disruptive technical developments, making it difficult for organizations to adjust and maintain their competitiveness.
AI-Based Financial Distress Prediction's Advantages
Organizations face various difficulties in the dynamic world of business and finance, including the risk of a financial crisis. Maintaining stability and ensuring long-term success depends on the capacity to proactively recognize and address financial problems. In this aspect, artificial intelligence (AI) solutions have become a potent instrument that is revolutionizing how organizations approach risk management:
Superior Risk Assessment: The ability to forecast financial trouble using AI is improved by giving users a full, all-encompassing view of an organization’s financial situation. These models take into account a wide range of data sources, including market data, industry trends, news sentiment analysis, and social media data, in addition to financial statements and ratios. AI models can find hidden dangers, weaknesses, and possible connections that could affect a company’s financial health by combining such a wide range of data. This makes it possible for businesses to manage resources more wisely and with more knowledge. According to a report by McKinsey, organizations that utilize advanced AI platforms in risk management achieve up to 25% improvement in forecasting accuracy and risk identification.
Banking industry : Lead your Business teams to embrace AI
The banking services industry has long been a leader in AI implementation, discover in this free White paper how you can Lead your Business teams to embrace AI.
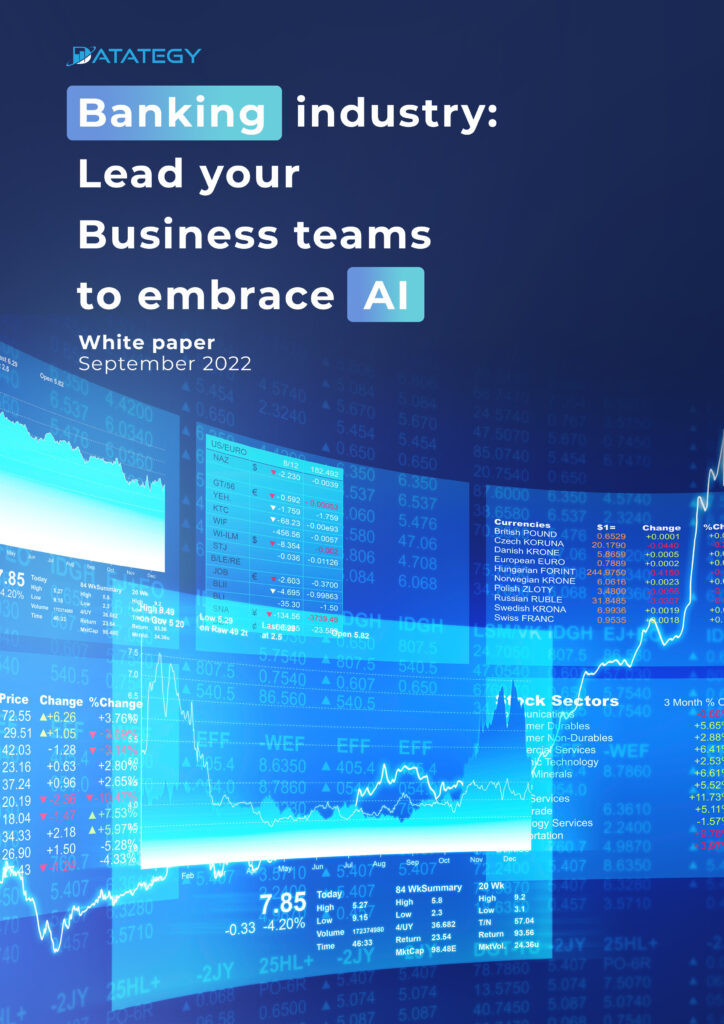
Case Study: How papAI Solution can Enhance Financial Distress Prediction?
1- Dataset Presentation
The dataset used in this analysis revolves around the prediction of financial distress for a sample of companies. It encompasses a range of essential information related to these companies and their financial well-being. The dataset consists of various columns, including the company names, time periods associated with the data, and a target variable called “Financial Distress.”
This target variable gives a probability that distinguishes between financially healthy companies and financially distressed ones. Additionally, the dataset incorporates several features (x1 to x83) that reflect both financial and non-financial characteristics of the companies during the preceding time period. This dataset provides a valuable resource for analyzing and predicting the financial health of companies, making it an ideal foundation for further research and modeling in this domain.
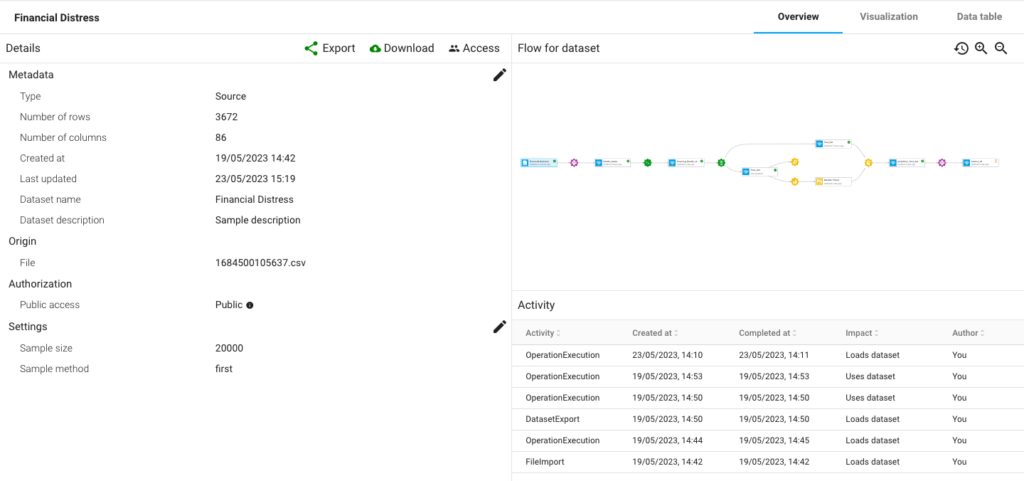
2- Data Analysis and Preparation
To prepare the data for analysis, we perform some preprocessing steps.
Firstly, we cast the “Financial Distress” column into a Boolean format. If the value is greater than -0.50, we consider the company as healthy (False). Otherwise, if it is less than or equal to -0.50, we consider the company as financially distressed (True). This conversion allows us to frame the problem as a binary classification task.
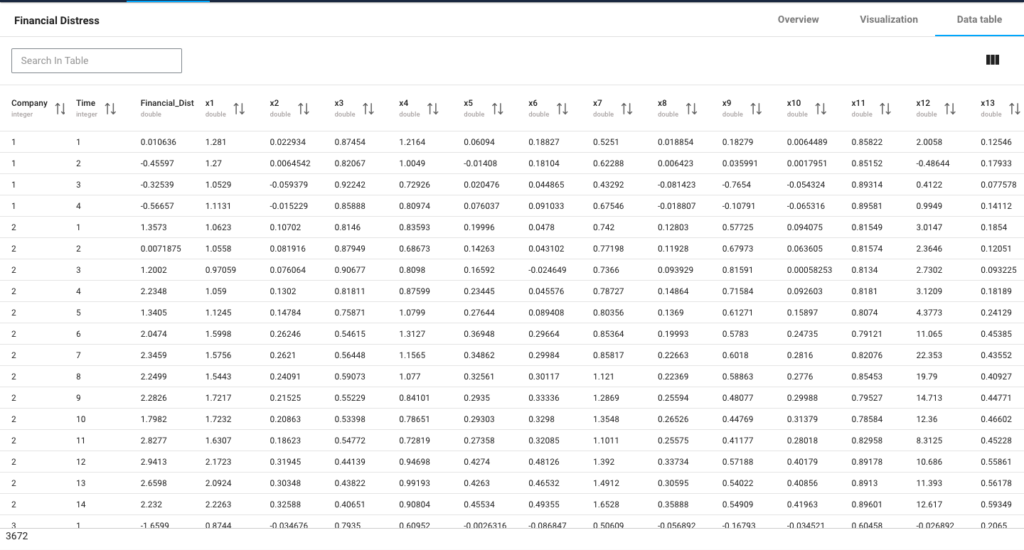
Next, we split the dataset into training and testing sets. We use a stratified splitting method, which ensures that the proportion of financially distressed and healthy companies is maintained in both the training and testing sets. The stratified splitting is crucial in this case due to the imbalance in the dataset. It helps prevent biases that may arise from uneven distribution, ensuring that both sets have representative samples of financially distressed and healthy companies. Specifically, we allocate 75% of the data for training and the remaining 25% for testing.
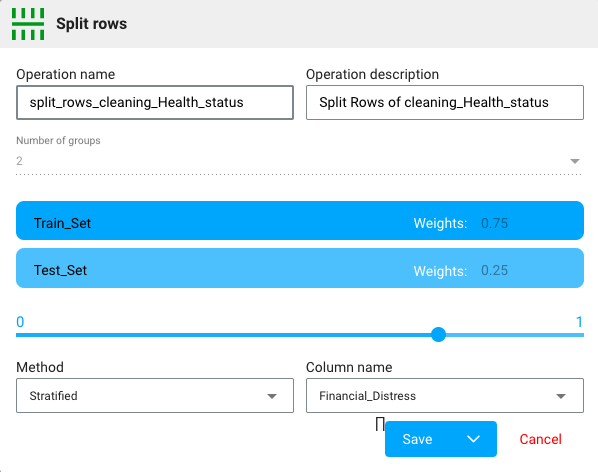
In summary, the data analysis and preparation stage involve transforming the target variable into a binary format for classification, followed by a stratified split of the dataset into training and testing sets. These steps are essential to ensure the integrity of the analysis and the accurate evaluation of the predictive model’s performance.
3- Model Training
In the training phase, we utilized various models available in papAI solution to build and evaluate our financial distress prediction model. papAI provides a wide range of machine-learning algorithms and techniques that are suitable for classification tasks.
To begin, we trained multiple models using the training set obtained from the stratified split. These models included popular classifiers such as logistic regression, decision tree, random forest, and support vector machines… Each model was trained on the input features and the corresponding binary target variable derived from the “Financial Distress” column.
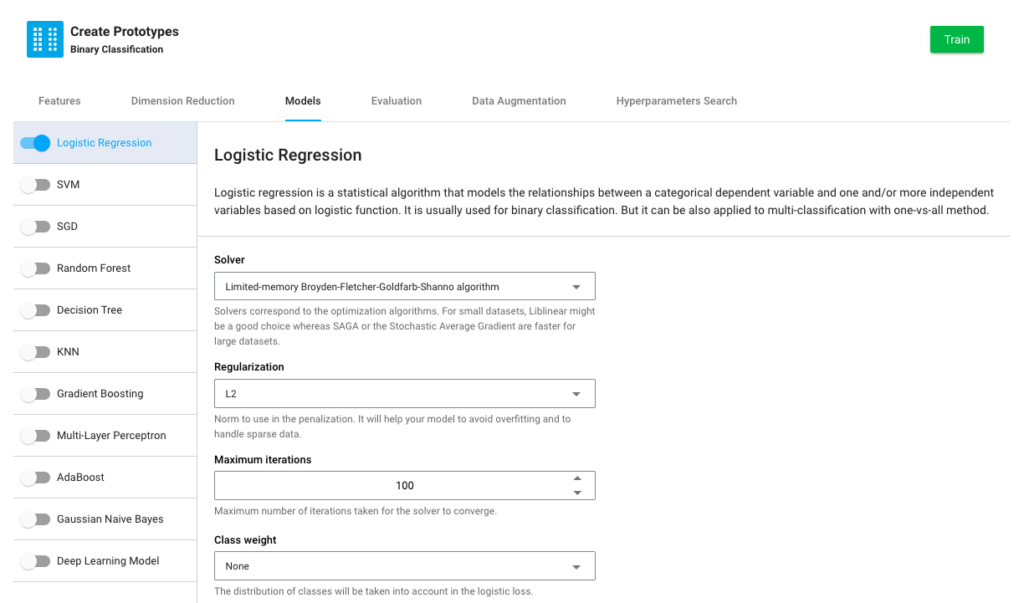
Based on the evaluation results, we selected the model that demonstrated the highest performance in terms of the f-score. This model exhibited the most accurate predictions for both financially distressed and healthy companies. Selecting the model with the best performance ensures that our deployed solution has the highest potential to effectively predict financial distress in companies.
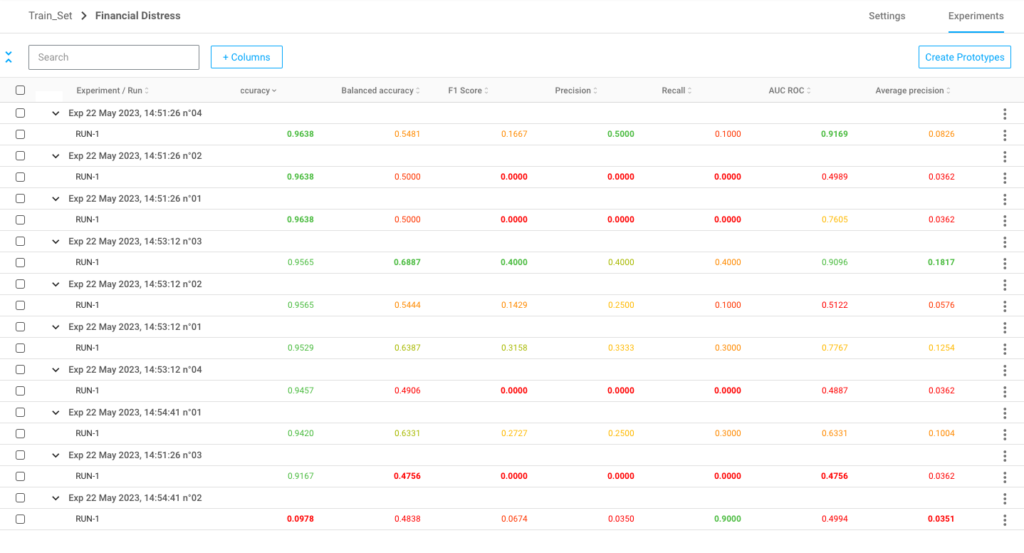
In addition to the training process described above, we employed data augmentation techniques to address the class imbalance issue present in the dataset. Imbalanced datasets can lead to biased models that favor the majority class, resulting in a suboptimal performance for predicting the minority class. To mitigate this challenge, we utilized data augmentation algorithms available in papAI solution.
The data augmentation techniques available included random over-sampling, SMOTE (Synthetic Minority Over-sampling Technique), ADASYN (Adaptive Synthetic Sampling), Borderline SMOTE, SWIM maha (Synthetic With Interpolation of Minority using Mahalanobis distance), and SWIM RBF (Synthetic With Interpolation of Minority using Radial Basis Function).
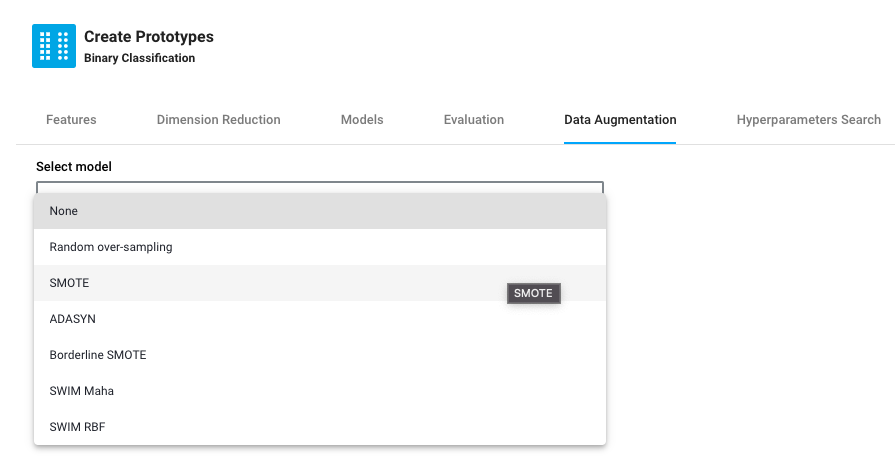
By augmenting the dataset, these algorithms generated synthetic samples for the minority class, thereby balancing the representation of financially distressed and healthy companies. This augmentation process introduced additional variations and diversity to the training data, allowing the model to learn from a more comprehensive and representative set of examples.
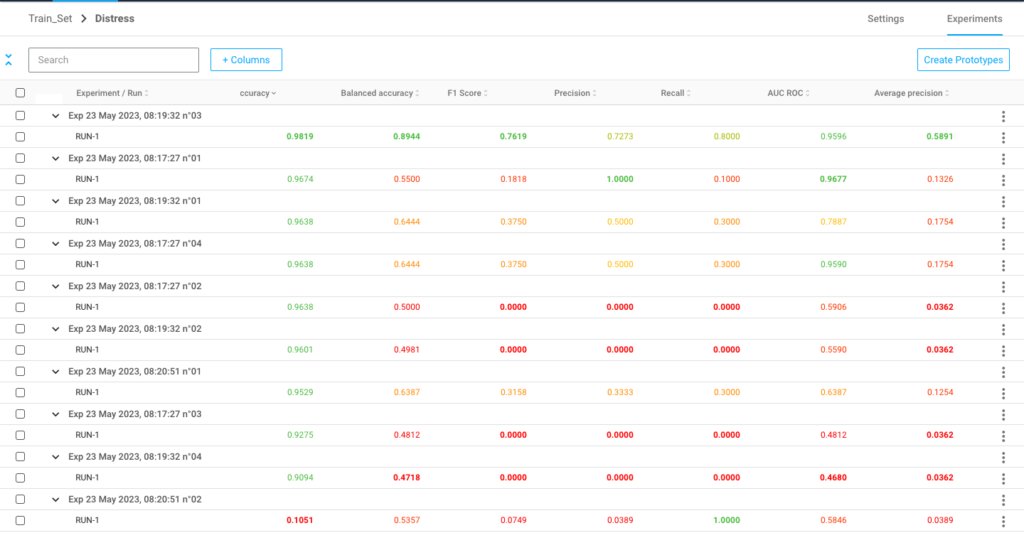
In conclusion, the training phase involved exploring various models available in papAI Solution evaluating their performance using the f-score, employing data augmentation techniques to address the class imbalance, and selecting the model with the highest performance. The chosen model forms the basis of our deployed solution, providing valuable insights and aiding in effective decision-making processes.
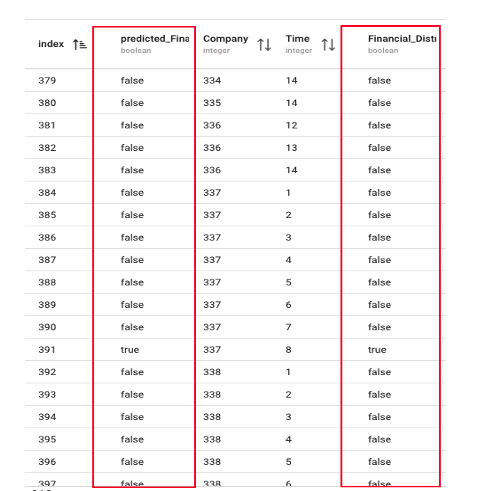
Upon obtaining the predicted results for financial distress and comparing them with the actual financial distress labels, we conducted a thorough analysis to evaluate the performance of our model. The predicted financial distress outcomes were derived from the trained model’s application on the testing dataset. By comparing these predictions with the real financial distress labels, we assessed the model’s ability to accurately classify companies as financially distressed or healthy.
4- Interpret the Predicted Outcome
By closely examining the percentages associated with each variable, we can pinpoint the factors that have the greatest impact on the model’s performance. Variables with higher percentages indicate a significant influence on the model’s prediction, underscoring their crucial role in the learning process. These key variables should be the primary focus for adjustments and subsequent optimizations. On the other hand, variables with lower percentages can be considered to have a relatively weaker impact on the model’s performance. This could be due to their less informative nature or complex interaction with other variables in the dataset.
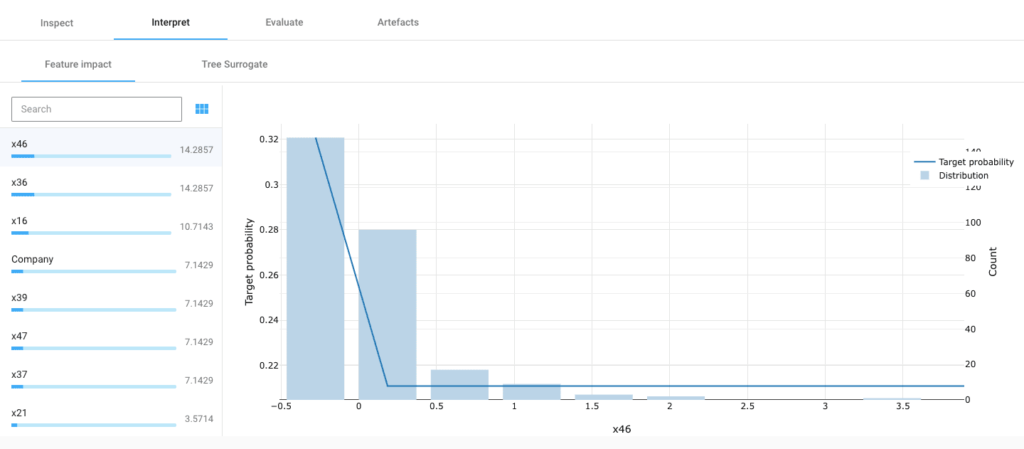
Create Your Own AI-based Tool with papAI solution to Improve Financial Distress Prediction
With papAI solution, you can use AI to improve the financial distress prediction of your company. You have the chance to design your own AI-based tool using papAI that is suited to your particular requirements. Utilizing cutting-edge machine-learning techniques will greatly increase the precision and effectiveness of your prediction procedures.
papAI solution helps to explain and make transparent the results through a process of data mining, cleaning, and visualization that can accelerate the deployment of AI projects. Book your demo now. Our team of experts can help you create a custom AI-based tool that meets the unique needs of your organization.
Interested in discovering papAI?
Our commercial team is at your disposal for any questions
How RAG Systems Improve Public Sector Management
How RAG Systems Improve Public Sector Management The most important...
Read MoreScaling RAG Systems in Financial Organizations
Scaling RAG Systems in Financial Organizations Artificial intelligence has emerged...
Read MoreHow AgenticAI is Transforming Sales and Marketing Strategies
How AgenticAI is Transforming Sales and Marketing Strategies Agentic AI...
Read More