“DATATEGY EARLY CAREERS PROGRAM” With Julien Courteville Hello, my name...
Read MoreBoosting Sales Performance Using papAI: How to Enhance Pharma Sales Forecasting Accuracy?
Table of Contents
ToggleA rising number of pharmaceutical executives are looking to artificial intelligence (AI) as a potential answer as they struggle with an assortment of complexity, prices, and restrictions. In fact, according to a recently published worldwide market estimate for the pharmaceutical sector, investment in AI will top $3.6 billion by 2026. Applications for drug research, production, diagnostic support, drug commercialization, and business operations are some examples of use cases for the technology.
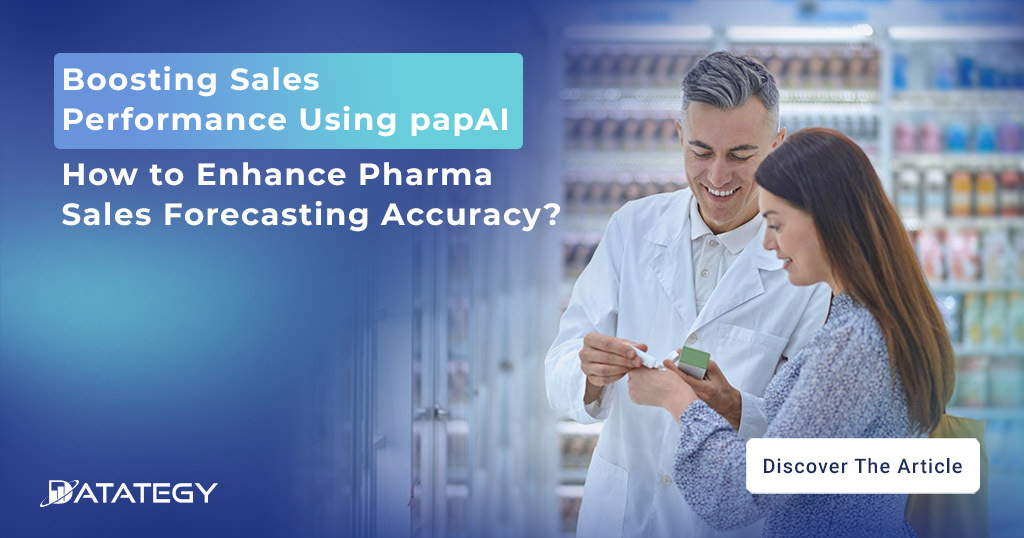
In this article, we will provide an overview of the adoption of AI in the pharmaceutical industry and explore its potential to improve the accuracy of sales forecasts to improve decision making.
AI in Pharmaceutical Market
The global AI in pharmaceutical market was valued at USD 905.91 million in 2021 and is expected to reach over USD 9,241.34 million by 2030, poised to grow at a compound annual growth rate (CAGR) of 29.4% from 2022 to 2030.
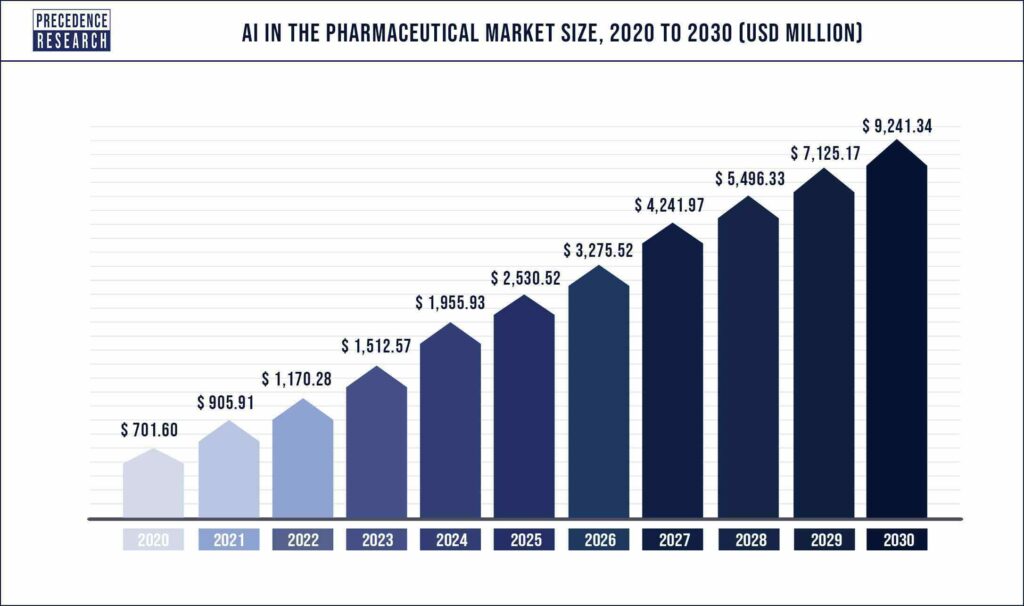
Covid-19, the starting point for mass adoption
Artificial intelligence (AI) in pharmaceuticals refers to automated and networked technologies that can operate autonomously with little to no human interaction. Manufacturing, clinical trial, and R&D activities may all be considerably improved by artificial intelligence. During the forecast period, it is estimated that more than 60% of healthcare organizations worldwide plan to implement artificial intelligence technologies. The booming expansion of the market is anticipated to be significantly influenced by the increasing acceptance of AI in the biotechnology and healthcare sectors.
Leading corporations including Pfizer, Sanofi, GSK, and AstraZeneca are working with or purchasing AI firms and technology. As a result, the leading healthcare corporations’ significant expenditures are significantly boosting the worldwide AI in the pharmaceutical industry.
The rise in healthcare costs, helpful governmental regulations, and robust economic development over the past several years, particularly in emerging nations, are all factors that have contributed to the emergence of AI in the pharmaceutical industry. In 2020, the use of AI in the pharmaceutical industry had a sharp surge as a result of increased funding for the development of new COVID-19 illness treatments. As an illustration, in April 2020 GSK and Vir Biotechnology partnered to improve medication discovery for COVID-19 using AI and CRISPR. Therefore, the COVID-19 disease outbreak has caused the market to grow in 2020, and this growth is anticipated to continue and have a significant impact on the market growth throughout the forecast period.
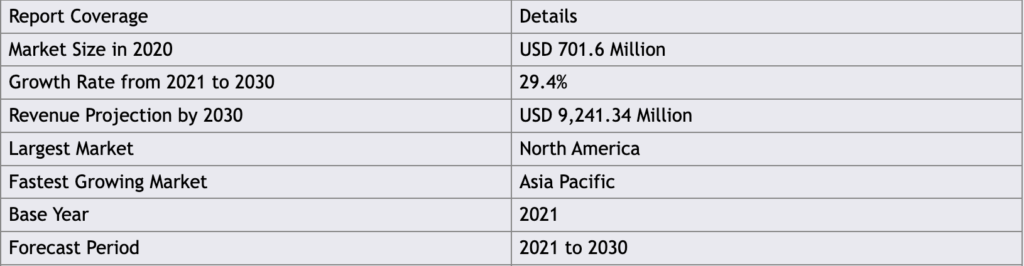
Size of the AI Market for Pharmaceuticals
Artificial Intelligence's Potential for Pharmaceutical Sales Forecasting
Any business must consider sales forecasting as a critical component, and the pharmaceutical sector is no exception. Accurate sales forecasting aids businesses in making better decisions, allocating resources more efficiently, and increasing profitability. However, given the complexity of the market and the wide range of variables that can affect sales, forecasting sales in the pharmaceutical sector is difficult.
Fortunately, improvements in artificial intelligence (AI) offer hopeful answers to raise the accuracy of pharmaceutical sales forecasting. Large volumes of data from several sources, including market trends, product performance, consumer behavior, and external influences like legislative changes, economic situations, and disease outbreaks, may be processed by AI algorithms.
The following are a few ways AI might improve the precision of pharmaceutical sales forecasting:
1- Customer Affinity Prediction
Artificial intelligence (AI) that employs machine learning algorithms to forecast consumer behavior and preferences is known as customer affinity prediction. In the pharmaceutical sector, where salespeople and marketers depend on precise consumer insights to make judgments about their goods and marketing tactics, this technology can be very helpful. AI algorithms can spot trends in consumer data, such as prior purchase history, demographics, and internet activity, and forecast which customers are most likely to make particular purchases or respond favorably to particular marketing initiatives.
This increases the possibility of successful outcomes by enabling pharmaceutical businesses to target individual clients with their marketing and sales activities. Customer affinity prediction can also assist in identifying prospective clients who could be at risk of moving to a competitor. enabling businesses to take preventative action to keep customers. Overall, the accuracy and efficacy of pharmaceutical sales and marketing efforts could be greatly improved with the use of AI for customer affinity prediction.
2- Predictive Modeling
A method for analyzing past data and producing predictions about future occurrences is called predictive modeling. It is a subset of machine learning, a branch of artificial intelligence that focuses on developing algorithms capable of learning from data and making predictions. Predictive modeling may be used in the context of pharmaceutical sales forecasting to analyze historical sales data, consumer behavior, market trends, and other pertinent aspects to produce precise estimates of future sales performance. Making educated judgments on resource allocation, product development, and marketing strategies will aid pharmaceutical businesses, which will ultimately result in improved commercial outcomes.
The most important variables influencing sales success may be found through predictive modeling. enabling businesses to concentrate their efforts in the areas where they will have the biggest impact. Overall, by delivering improved accuracy and insights into future sales performance, the application of predictive modeling in pharmaceutical sales forecasting has the potential to revolutionize the business.
3- Territory Sales Management
The technique of splitting a company’s sales activities into geographical regions or territories is known as sales territory management. By placing sales personnel in areas where they will be most productive, sales territory management aims to maximise sales and profitability. The borders of sales territories can be determined by a number of variables, such as client categories, industry verticals, or product lines.
4- Competitive Analysis
In order to understand a company’s rivals’ positions in the market and to guide business strategy, competitive analysis involves assessing and analyzing their strengths and shortcomings. Pharmaceutical businesses may undertake competitive analysis more effectively and precisely with the use of AI-powered solutions.
To discover significant rivals and their market positions, AI algorithms may gather and analyze enormous volumes of data from several sources, including social media, news articles, and industry studies. This involves keeping an eye on new product introductions, price plans, marketing initiatives, and client feedback. AI solutions that monitor these patterns can help pharmaceutical businesses keep one step ahead of their rivals by spotting chances for development.
Companies may discover possible risks and difficulties, such as new rivals or changes in the competitive environment, using AI-powered competitive analysis. Making strategic decisions with the use of this information will enable businesses to adjust to rapidly shifting market situations.
Case Studies: How papAI Solution can Improve the Accuracy of Pharmaceutical Sales Forecasts
Dataset Presentation
The Pharma Drug Sales dataset is a collection of transactions collected over a six-year period from 2014 to 2019, with hourly frequency. The dataset consists of 14 columns, including drug categories such as Acetic Acid Derivatives, Salicylic Acid Derivatives, and Antihistamines. The group of drugs in the dataset is constructed from 57 different drugs for various medical purposes. Each transaction records the drug type and quantity, providing a comprehensive view of drug sales trends over time. The dataset offers a valuable resource for data analysis and prediction modeling related to the pharmaceutical industry.
Data Analysis and Preparation
The Pharma Drug Sales dataset provides a wealth of information on drug sales. Before training a machine learning model, it is important to perform exploratory data analysis (EDA) and data preparation to ensure that the data is properly cleaned and formatted.
The first step in the process was to create a new column in the data frame called “DateTime”. This column was created by converting the existing “Time” column into a standard date and time format using the Cast function.
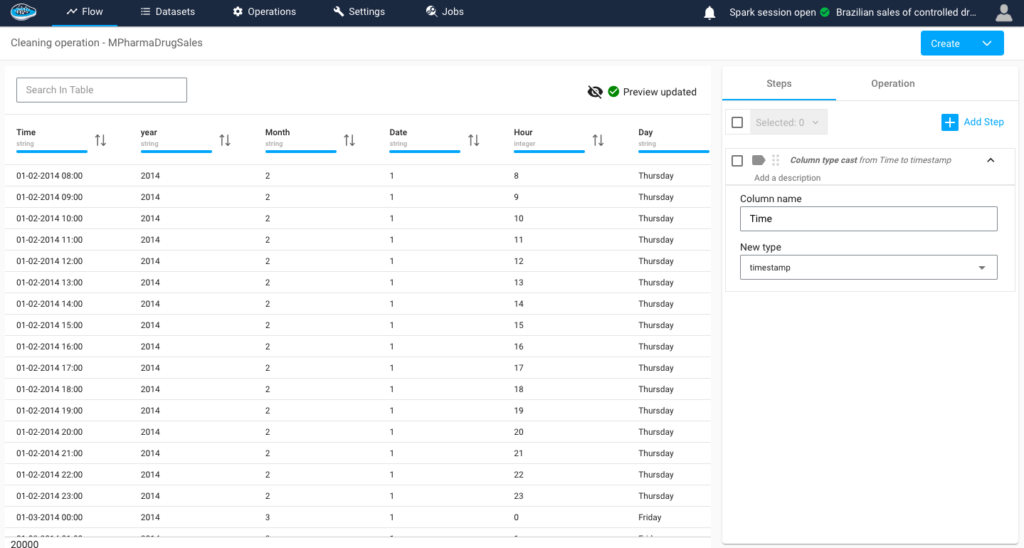
The next step was to transform the data frame into a long format using the “pd.melt” function. The long format is a way of representing data where each row represents a single observation or measurement, and each column represents a variable. This format is often used in data analysis and visualization because it is more flexible and can be easily transformed into other formats if needed. The “pd.melt” function takes the columns of the dataframe and converts them into variables, which are then combined into a single column.
After transforming the data frame into a long format, several unnecessary columns were removed using the “drop” method. The “drop” method is a built-in pandas function that allows you to remove one or more columns or rows from a dataframe. In this case, the columns that were removed were not needed for the subsequent analysis and were therefore dropped.
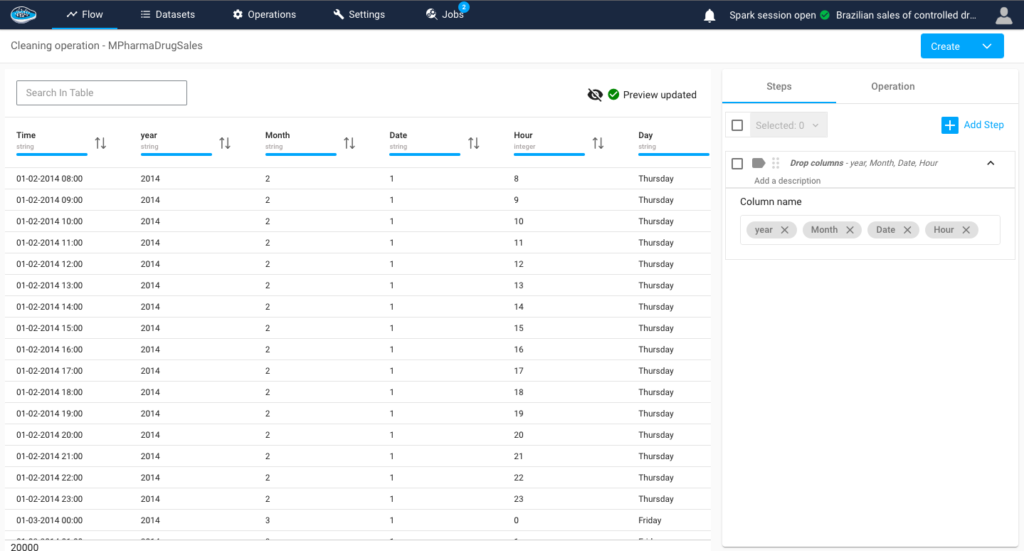
The next step was to define the index column of the dataframe using the “set_index” method. The index column is used to identify each row of the dataframe and is often used for grouping, filtering, and sorting data. In this case, the index column was set to the “DateTime” column, which contains the date and time information for each observation. This step was useful because it allowed us to group the data by week later on.
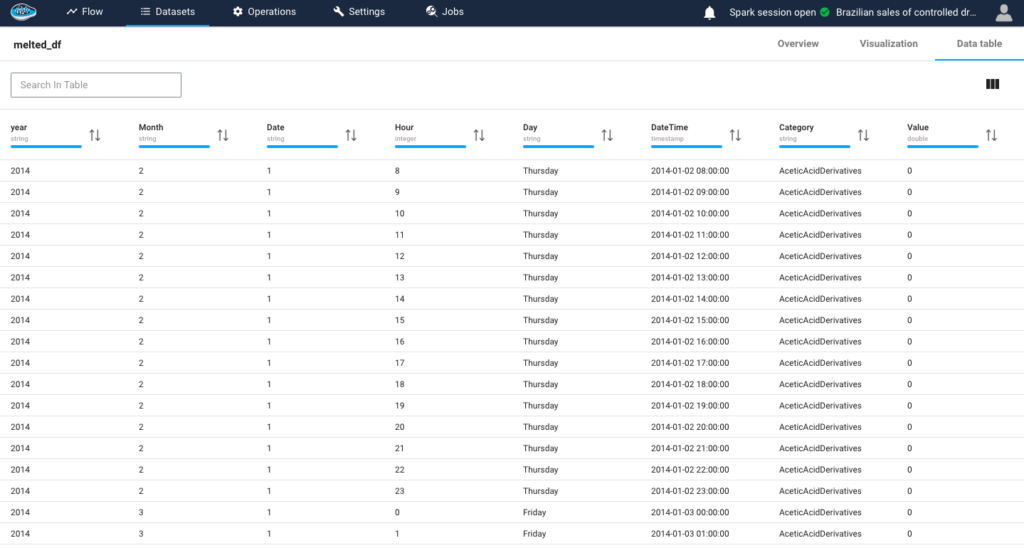
Finally, a new dataframe called “df_daily” was created by grouping the data in the original dataframe by category and by week using the “groupby” and “resample(‘W’).sum()” methods. The “groupby” method is a powerful pandas function that allows you to group data by one or more columns and apply various functions to each group. In this case, the data was grouped by category and week. The “resample(‘W’).sum()” method was used to resample the data at a weekly frequency and calculate the sum of values for each week. The “reset_index()” method was then used to reset the index of the dataframe, which removed the hierarchical index and returned the dataframe to a flat structure. The resulting data frame contained the total value for each category for each week, which was useful for further analysis and visualization.
Overall, the data analysis and preparation process involved several steps to transform and clean the initial dataset. These steps allowed us to prepare the data for further analysis and visualization and provided us with a more flexible and structured dataset that could be easily manipulated and transformed as needed.
Model Training
After completing EDA and data preparation, we trained a machine learning model to predict drug sales based on the time of year and drug category. We decided to use TS-Forecasting MQ-CNN. The TS-Forecasting MQ-CNN algorithm is a deep learning-based method that combines the power of Convolutional Neural Networks (CNNs) and Multichannel Multi-Resolution (MQ) techniques to accurately predict time series data.
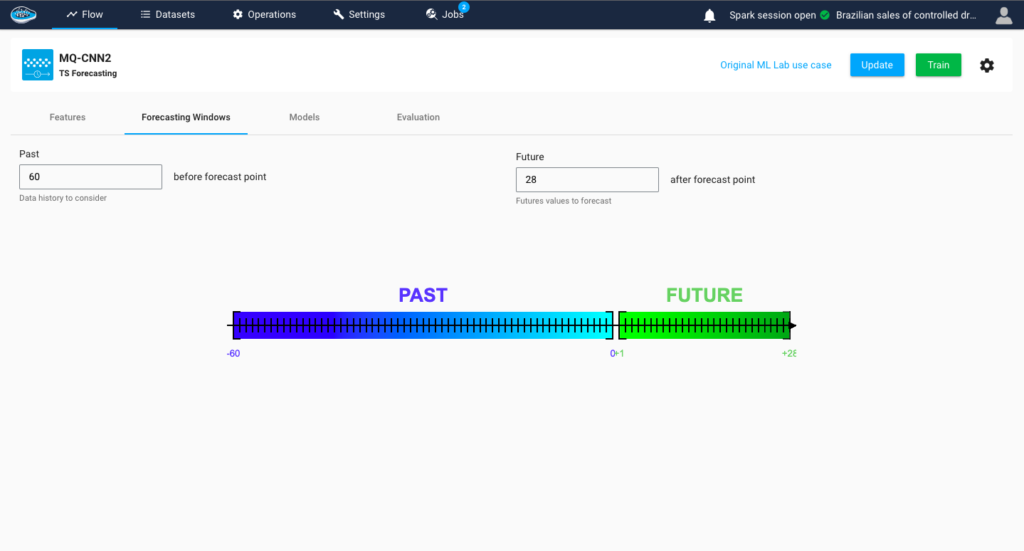
To use the TS-Forecasting MQ-CNN algorithm, we first split the prepared dataset into a training set and a testing set. The training set was used to train the algorithm on the historical data, while the testing set was used to evaluate the performance of the algorithm on unseen data.
The TS-Forecasting MQ-CNN algorithm was then applied to the training data to learn the underlying patterns and relationships in the time series data. Once the model was trained, it was used to make predictions on the testing data. The performance of the algorithm was evaluated using various metrics, such as mean squared error (MSE), mean absolute error (MAE).
MSE measures the average of the squared differences between the predicted values and the actual values. It is calculated by taking the difference between each predicted value and the corresponding actual value, squaring the differences, and then taking the average of the squared differences. MSE gives a higher weight to larger errors, which means that it penalizes large errors more heavily than small errors. Therefore, a lower MSE indicates better performance of the model.
MAE, on the other hand, measures the average of the absolute differences between the predicted values and the actual values. It is calculated by taking the absolute difference between each predicted value and the corresponding actual value, and then taking the average of the absolute differences. Unlike MSE, MAE treats all errors equally, regardless of their size. Therefore, MAE is less sensitive to outliers and is a good metric to use when the distribution of errors is not normal.
Both MSE and MAE are useful metrics for evaluating the performance of a model in time series forecasting. While MSE is more sensitive to large errors, MAE is less sensitive to outliers. Therefore, it is important to consider both metrics when evaluating the performance of a model. A good model should have both a low MSE and a low MAE.
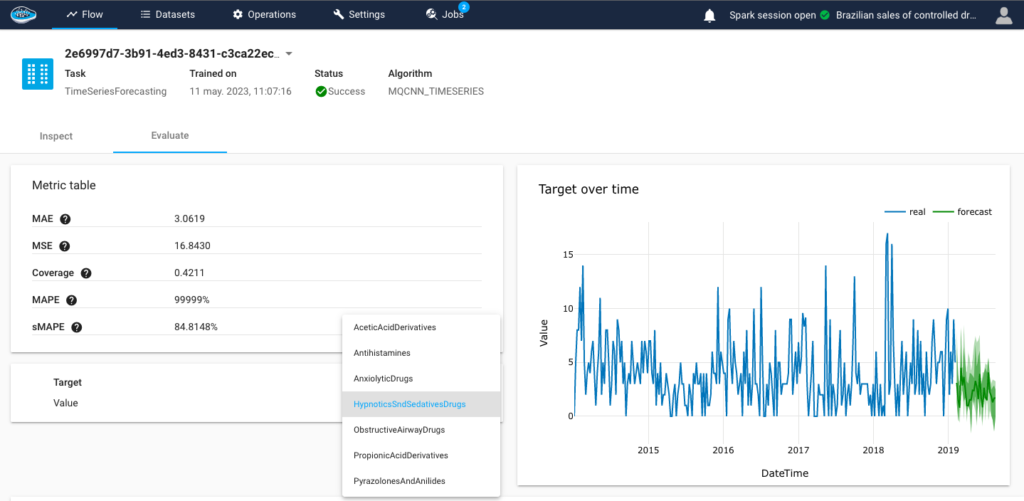
The TS-Forecasting MQ-CNN algorithm was able to accurately predict the future values of the time series data, achieving a high level of accuracy and outperforming other traditional time series forecasting methods. The predictions generated by the algorithm were then used to identify trends and patterns in the data, and to make informed decisions about future actions based on these predictions.
In summary, the use of the TS-Forecasting MQ-CNN algorithm allowed us to predict future values of the time series data with a high degree of accuracy, and to identify patterns and trends in the data that would have been difficult to detect otherwise. This information was valuable for making informed decisions about future actions and optimizing business operations.
Create your own AI-based tool with papAI solution to improve the accuracy of your sales forecast
In conclusion, organizations of all sizes are finding that using AI-based technologies for sales forecasting is more necessary. Businesses may build their own AI-based tools that are uniquely suited to their needs with papAI solution. This might lead to more accurate and trustworthy sales estimates, which would enhance decision-making and boost profitability.
papAI solution helps to explain and make transparent the results through a process of data mining, cleaning, and visualization that can accelerate the deployment of AI projects and drug discovery and reduce the time and cost of bringing new therapies to market.
Book your demo now. Our team of experts can help you create a custom AI-based tool that meets the unique needs of your organization.
Interested in discovering papAI?
Our commercial team is at your disposal for any questions
Datategy + Nedd Technologies: First Center of AI Excellence in USA
Datategy + Nedd Technologies: First Center of AI Excellence in...
Read MoreHow AI Drives the Evolution of Industry 4.0
How AI Drives the Evolution of Industry 4.0 Today, Industry...
Read MoreOptimize RAG Systems Like a Pro with Fine-Tuning
Optimize RAG Systems Like a Pro with Fine-Tuning As artificial...
Read More