Supervised and Unsupervised Learning: A Comparison Guide Artificial intelligence (AI)...
Read MoreExplainable AI in Healthcare: Addressing the Need for Transparency and Accountability in Clinical Decision-Making
Table of Contents
ToggleIn recent years, improvements in artificial intelligence (AI) algorithms and access to training data have made it possible to envisage AI augmenting or replacing some of the current functions of physicians. However, the interest of various stakeholders in the use of AI in medicine has not translated into widespread adoption.
As many experts have stated, one of the main reasons for this limited adoption is the lack of transparency associated with some AI algorithms, particularly black box algorithms. Clinical medicine, and especially evidence-based medical practice, relies on transparency in decision-making. If there is no medically explainable AI and the doctor cannot reasonably explain the decision-making process, the patient’s trust in the doctor will erode.
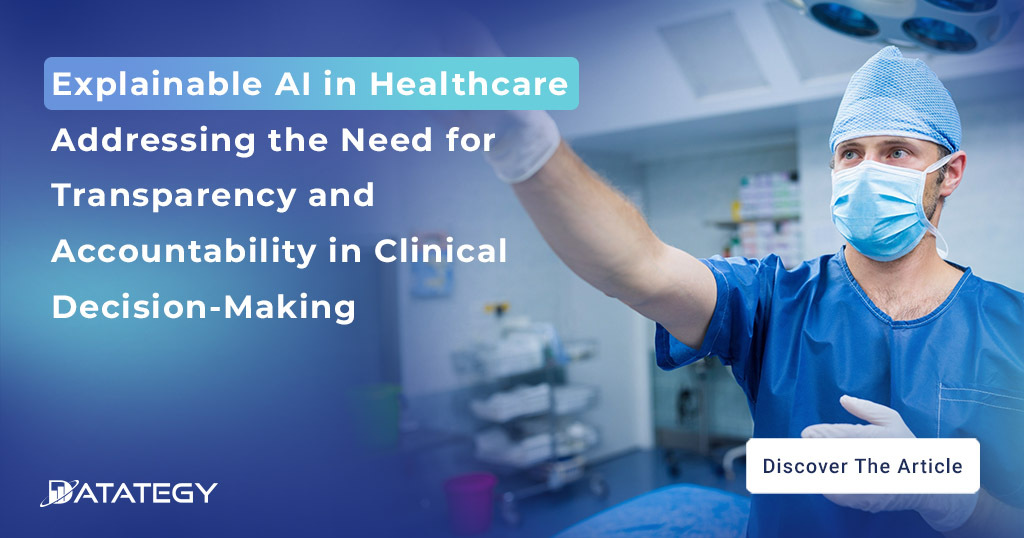
A survey conducted by MIT Technology Review found that 89% of healthcare executives believe that explainable AI is important in order to gain the trust and acceptance of AI-based decision-making in healthcare (source: MIT Technology Review, 2021).
What does explainable AI (XAI) mean?
Explainable artificial intelligence (XAI) is the name given to a group of methods and processes that enable users (in this context, medical professionals) to comprehend how AI systems arrive at their conclusions or forecasts. XAI is especially important in the healthcare industry since more and more clinical decision-making procedures are using AI and machine learning algorithms.
A wide range of healthcare applications for artificial intelligence (AI) systems are available, including patient monitoring, therapy suggestion, and illness diagnosis.Nevertheless, early healthcare systems’ AI-based decision-making was a “black box,” making it challenging to comprehend how the algorithm arrived at a certain result.
The lack of transparency and interpretability caused an important issue for healthcare professionals, who are in charge of making crucial decisions concerning patient care.
Overview of the challenges faced in clinical decision-making
A diagnosis or treatment plan is arrived at by the integration of clinical knowledge, patient preferences, and accessible data. Clinical decision-making is a complicated and multifaceted process. While making clinical decisions, healthcare practitioners must consider a number of factors, including:
Info overload: Healthcare professionals are frequently inundated with voluminous patient data, scholarly research, and therapeutic recommendations, making it challenging to identify the most pertinent and useful information.
Lack of formation: with the emergence of new tools for disease detection and patient management, health professionals need to be increasingly supported and trained.
Clinical fluctuation: Individual people with the same symptoms or diseases may respond to therapies in a variety of ways, making it difficult to choose the most effective treatment.
Time constraints: Healthcare professionals are sometimes forced to make decisions fast, especially in emergency or critical care circumstances, which can result in mistakes or less-than-ideal choices.
Lack of standardization: Clinical decision-making can vary considerably between healthcare organizations and practitioners, which can result in uneven and sometimes poor quality care.
Restricted information access: It’s possible that healthcare professionals don’t always have access to all the pertinent patient information or medical records, which makes it difficult to make recommendations.
Transparency and Explainability, two Concepts that Work Together
Since they foster a climate of trust and confidence between patients and healthcare professionals, transparency and accountability are essential in the healthcare industry. Patients have a right to know how healthcare professionals make clinical choices and should anticipate that their treatment will be supported by reliable data and moral standards.
By offering clear and comprehensible explanations for AI-based decisions, XAI may assist in addressing the demand for transparency and responsibility in the healthcare industry. This can assist medical professionals in spotting any biases or errors in the AI system and guarantee that therapeutic recommendations are supported by reliable data and moral standards.
Benefits of XAI in Healthcare
Improved diagnostic accuracy
XAI can help in increasing the precision of AI-based diagnostic systems by offering clear and comprehensible explanations for why the AI system made a certain diagnosis. A study published in the journal Nature in 2019 found that an XAI-based system improved the accuracy of breast cancer diagnoses compared to human experts. The system was able to accurately identify cancer in 94.5% of cases, compared to 88.3% for human experts. This can assist medical professionals in seeing any biases or flaws in the AI system and guarantee that patients receive correct diagnoses, which can enhance patient outcomes and lower the possibility of misdiagnosis.
Enhanced decision-making
XAI can help healthcare providers make more informed and confident decisions by providing clear and interpretable explanations of how the AI system arrived at a particular decision. This can help healthcare providers identify potential errors or biases in the AI system and ensure that decisions are based on sound evidence and ethical principles, which can improve patient outcomes and reduce the risk of harm.
Increased trust and adoption
By presenting concise and accessible explanations of how the AI system arrived at a certain decision, XAI can contribute in enhancing the trust and acceptability of AI-based systems in healthcare. The relationship between patients and healthcare professionals can be strengthened as a result, increasing patient satisfaction and the uptake of AI-based healthcare solutions.
What does papAI solution provide?
In order to determine if a patient would possibly develop cardiovascular disease, we analysed data from patients’ vital signs and medical history.
1- Identifying the target
Predicting the development of any heart illness in a certain population is the goal in our case. A person needs to be labelled as having a disease risk if a good thing happens. Then, it is possible to identify the risk factors for developing it.
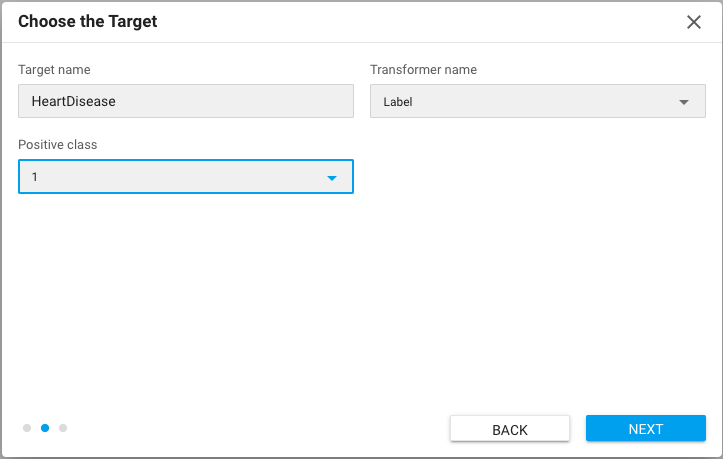
2- Configure the models
The first stage in creating our model is choosing the characteristics, which enables us to understand how they relate to the goal and use that information into the model training.
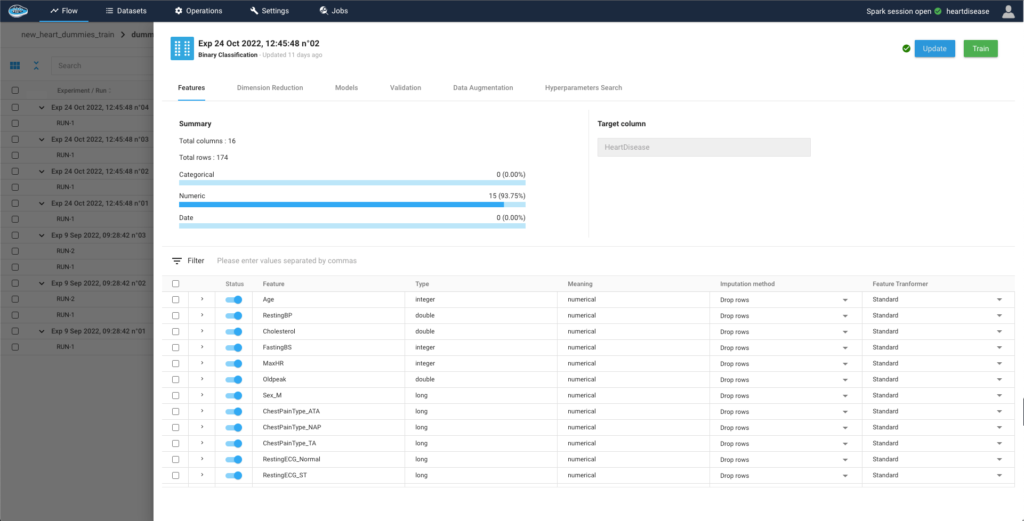
Next we choose the models that could work with the issue we’re trying to address. To develop, train, and deploy an AI pipeline quickly, papAI incorporates a vast library of ready-to-use models. papAI also offers an AutoML option for projects that are more complicated.
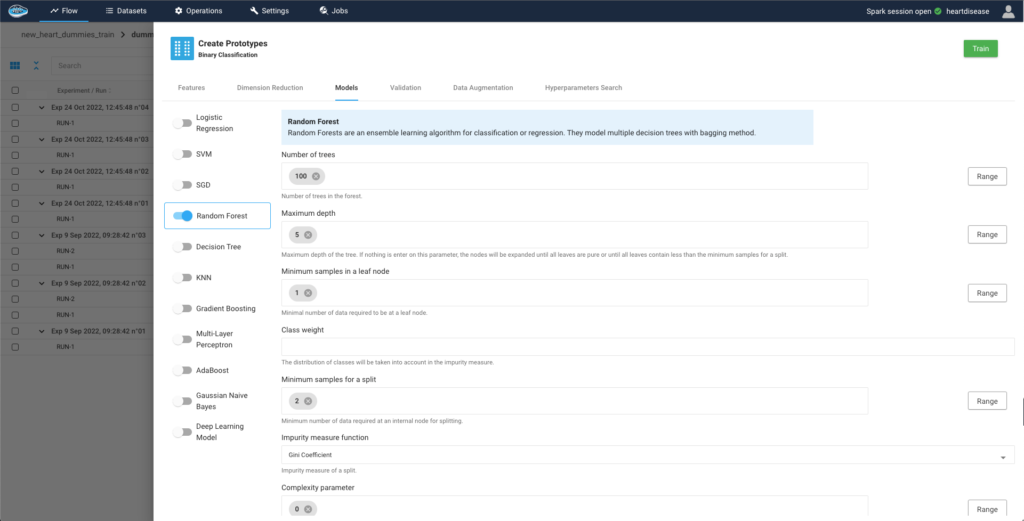
3- Evaluate the trained experiments
The evaluation process follows the execution of an experiment. With a number of measures built into the papAI interface, we must determine its efficacy and performance in forecasting the objective. For instance, assessment measures include confusion matrix, density chart, and accuracy.
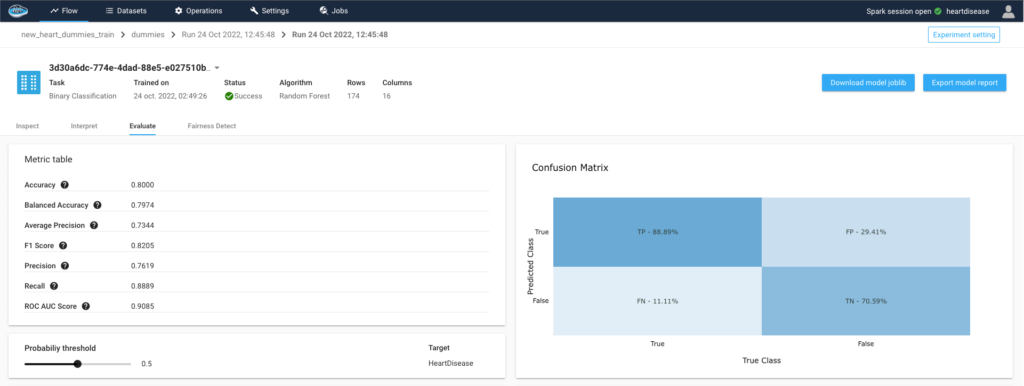
Through the density chart we can clearly see the ability for the model to determine the difference between both classes, people with or without heart disease, at a median threshold of 0.5, meaning that model successfully detected the two population independently.
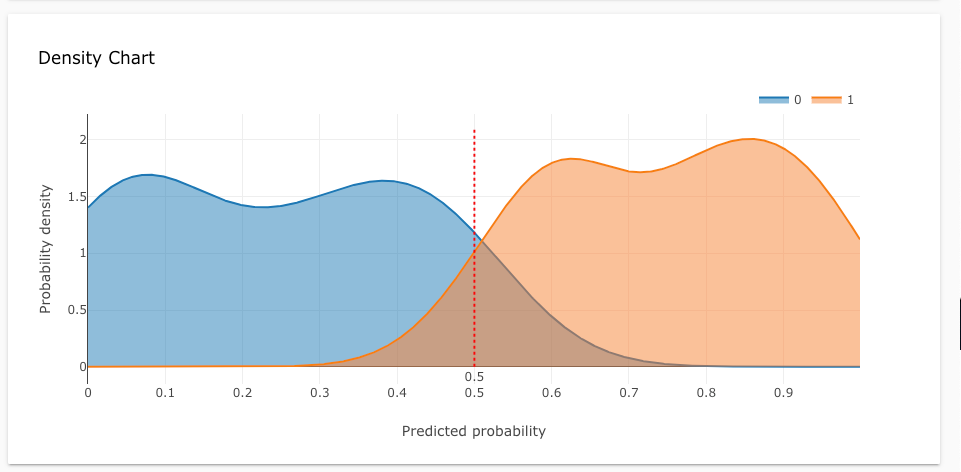
4- Interpret the predicted outcome
The evaluation step works in tandem with the explainability component to comprehend the behaviour of the model and the impact of each variable on the likelihood of the prediction. The potential risk factors for developing heart disease can then be evaluated.
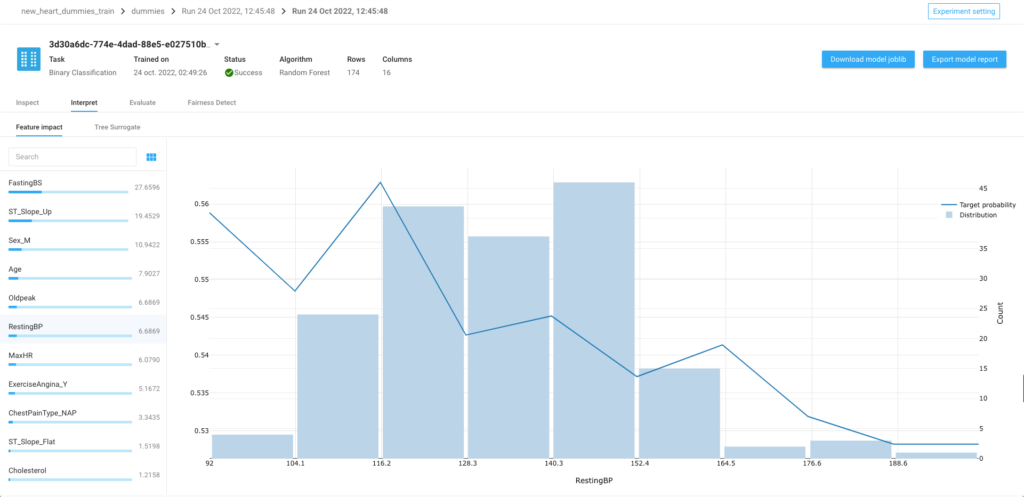
After going through these important processes, a model is chosen and promoted in order to apply it on an testing dataset and obtain individual predictions with a local interpretability. Thanks to this local interpretability, we can deeply understand the decision made by the model and try to develop cases to modify the predicted outcome.
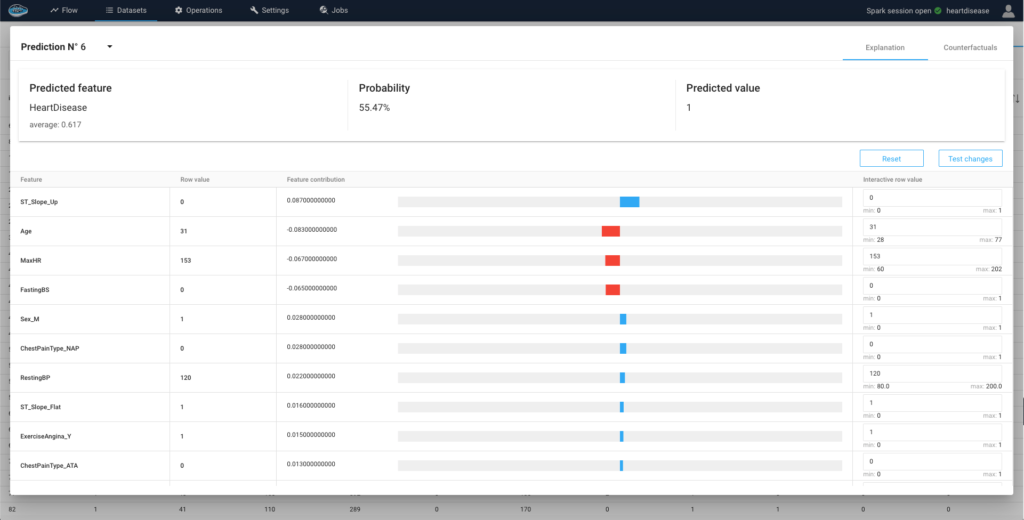
Create your own AI-based tool with papAI solution for more accurate decision making
As a result, Explainable AI (XAI) in healthcare is a potential technology that can offer concise and understandable explanations for how AI systems make decisions, enhancing the precision and reliability of clinical decision-making. It has been simpler for healthcare professionals to create their own XAI-based tools to support their decision-making process with the introduction of AI-based tools as papAI Solution.
Creating your own AI-based tool using papAI Solution can help you make more informed and accurate decisions, resulting in improved patient outcomes and reduced risk of harm. With the increasing amount of data available in healthcare, the use of AI-based tools has become more important than ever. It is essential that healthcare providers embrace this technology and take advantage of its benefits to improve the quality of care they provide.
Book your demo and see papAI solution in action now!
Interested in discovering papAI?
Our commercial team is at your disposal for any questions
“DATATEGY EARLY CAREERS PROGRAM” With Zineddine Chikhaoui
“DATATEGY EARLY CAREERS PROGRAM” With Zineddine CHikhaoui Hello, my name...
Read MoreAI Governance Pathway: Challenges and Solutions
AI Governance Pathway: Challenges and Solutions Recent advances in artificial...
Read MoreHow AI drives Operational Excellence in Manufacturing Industry
How AI drives Operational Excellence in Manufacturing Industry Operational excellence...
Read More