Datategy + Nedd Technologies: First Center of AI Excellence in...
Read MoreReducing Carbon Emissions with AI The Role of Machine Learning in Energy Efficiency
Table of Contents
ToggleIn order to prevent the impacts of climate change, the globe faces a significant challenge in reducing carbon emissions. It is crucial to implement sustainable practices and boost energy efficiency in order to meet global climate objectives and prevent a rapid rise in global temperatures.
AI and machine learning can help identify patterns and trends in energy usage, optimize energy systems, and predict and prevent energy waste. In fact, a report by the International Energy Agency estimates that AI could reduce global greenhouse gas emissions by up to 4% by 2030, which is equivalent to nearly 2.4 gigatons of carbon dioxide. This technology has the potential to play a significant role in reducing carbon emissions and mitigating the impacts of climate change. In this context, this article will explore the role of machine learning in energy efficiency and how it can contribute to reducing carbon emissions.
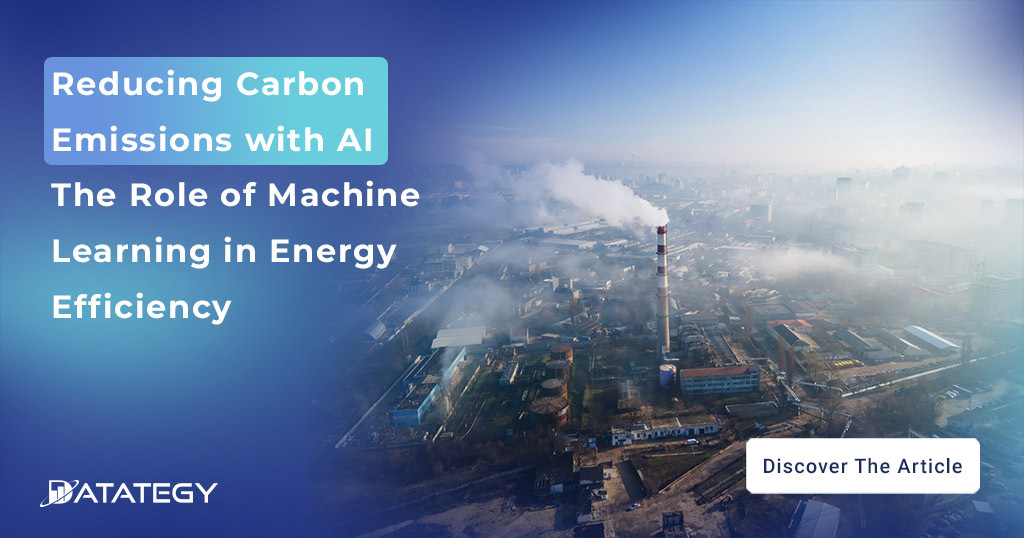
Machine learning algorithms can analyze vast amounts of data to identify patterns and trends that humans may not be able to detect. When it comes to energy efficiency, these algorithms can be used to identify areas where energy is being wasted or used inefficiently. This information can then be used to optimize energy usage and reduce carbon emissions.
Overview of carbon emissions
Global energy-related CO2 emissions grew by 0.9% or 321 Mt in 2022, reaching a new high of over 36.8 Gt. Following two years of exceptional oscillations in energy use and emissions, caused in part by the Covid-19 pandemic, last year’s growth was much slower than 2021’s rebound of more than 6%. Emissions from energy combustion increased by 423 Mt, while emissions from industrial processes decreased by 102 Mt. In a year marked by energy price shocks, rising inflation, and disruptions to traditional fuel trade flows, global growth in emissions was lower than feared, despite gas-to-coal switching in many countries. Increased deployment of clean energy technologies such as renewables, electric vehicles, and heat pumps helped prevent an additional 550 Mt in CO2 emissions. Industrial production curtailment, particularly in China and Europe, also averted additional emissions.
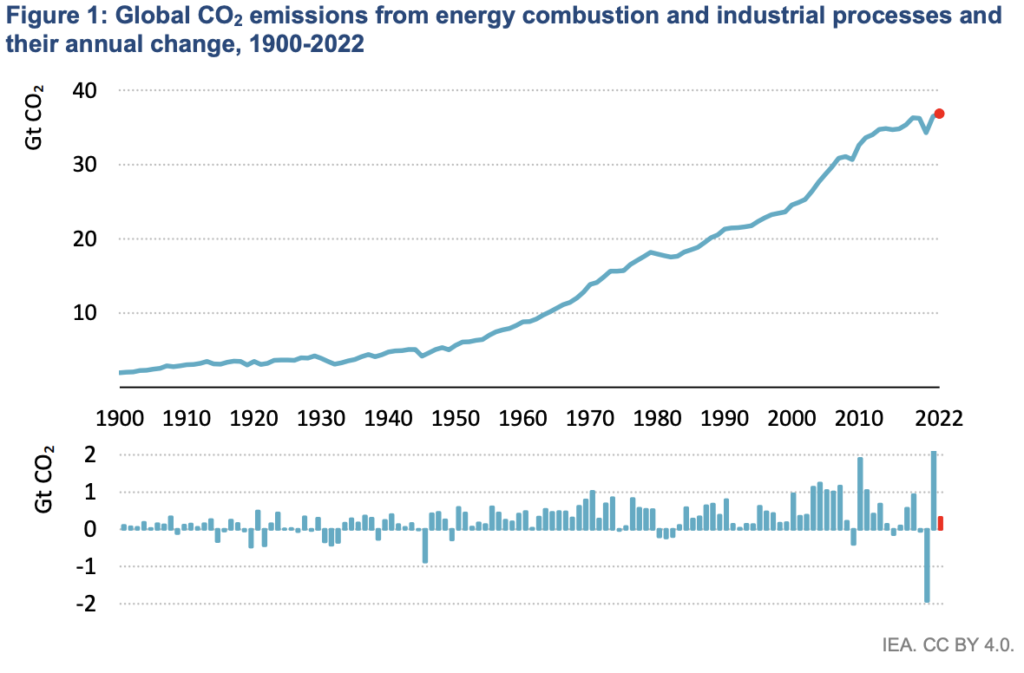
Across areas and sectors, there were conflicting tendencies. Outweighing decreases from Europe and China, CO2 emissions increased in North America and Asia (excluding the People’s Republic of China, or “China” henceforth). Globally, increases in CO2 emissions from electricity and transportation (including international bunkers) outweighed decreases from industry and buildings by 261 Mt and 254 Mt, respectively.
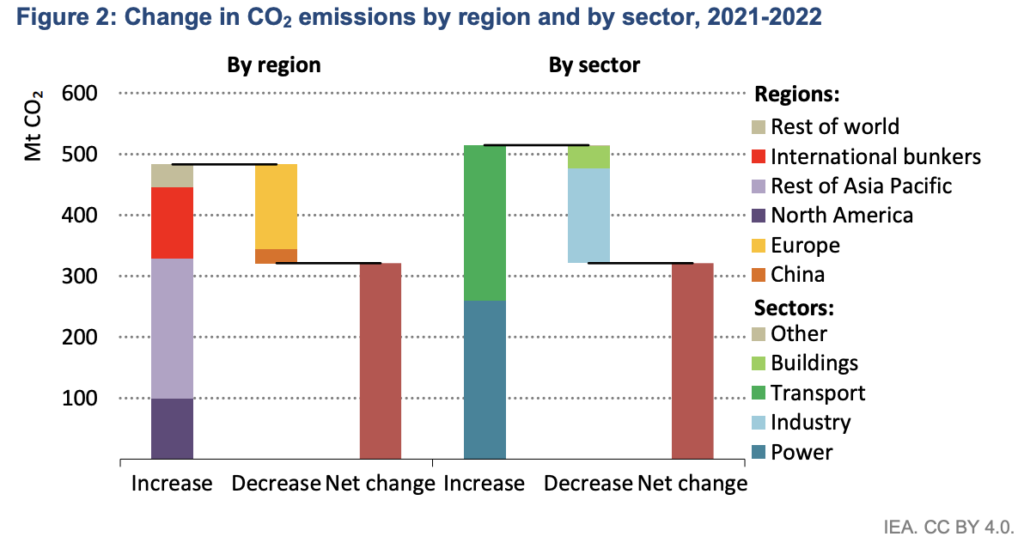
In an exceptionally turbulent year with Russia’s invasion of Ukraine, energy price shocks, rising inflation, and major disruptions to traditional fuel trade flows, global growth in emissions was lower than anticipated. Impressive growth of solar PV and wind generation helped prevent around 465 Mt CO2 in power sector emissions. Other clean energy technologies, including other renewables, electric vehicles, and heat pumps, helped prevent an additional roughly 85 Mt CO2. Without this increased growth in clean energy deployment, the annual increase in energy-related emissions would have been almost triple. Emissions reductions also resulted from economic slowdowns, including 155 Mt CO2 from decreases in energy-intensive industrial production, mainly in China, the European Union, Japan, Korea and North America.
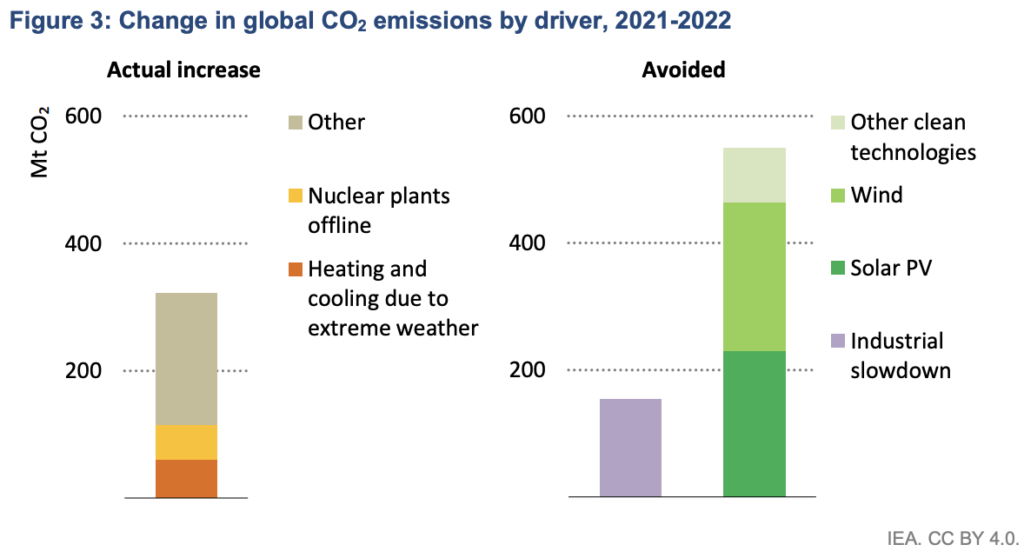
Empowering the Energy Industry through AI Revolution: The Critical Role of CDOs
Empowering the Energy Industry through AI Revolution: The Critical Role of CDOs
Chief Data Officers (CDOs) in the energy industry face several challenges when it comes to adopting AI technologies. There is a cultural shift that must occur within energy companies to embrace AI technologies. CDOs must continue to explore and implement AI technologies to improve efficiency, reduce costs, and meet sustainability goals.
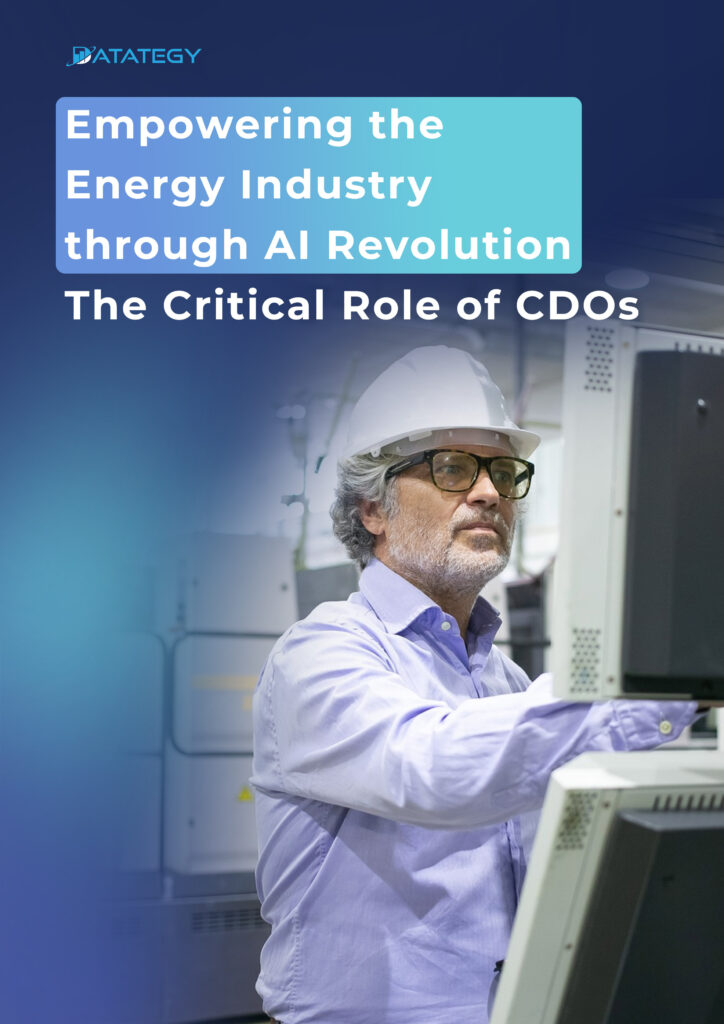
Applications of AI and ML in reducing carbon emissions
By leveraging these technologies, it is possible to develop more efficient and effective approaches to reducing carbon emissions across a range of industries and applications. In this article, we will explore some of the key applications of AI and ML in reducing carbon emissions, and the potential benefits of these approaches.
Energy Management
AI and ML may significantly reduce carbon emissions in the crucial field of energy management. With the growth of smart grids and the rising availability of data on energy consumption, it is conceivable to employ machine learning algorithms to optimise energy usage and eliminate waste.
Demand response is one of the main uses of AI and ML in energy management. Demand response refers to shifting energy demand and pricing signals causing changes in energy use. You may lower total energy use and lower carbon emissions by utilizing machine learning algorithms to estimate energy demand and manage energy use accordingly.
Energy efficiency is a significant use of AI and ML in energy management. It is possible to reduce total energy consumption and reduce carbon dioxide emissions by evaluating data on energy usage and finding places where energy efficiency may be increased. Everything from locating locations where lighting may be made better to maximizing heating and cooling systems to cut down on energy waste can be included in this.
Machine learning can also be used to optimize the operation of power plants and other energy infrastructure. By analyzing data on energy usage and identifying areas where operational efficiency can be improved, it is possible to reduce the carbon footprint of these facilities and cut overall energy consumption.
Transportation
Another crucial sector where AI and ML may significantly reduce carbon emissions is transportation. Machine learning algorithms may be used to improve travel routes and save fuel consumption thanks to the development of smart transportation systems and the growing availability of data on travel habits and vehicle performance.
Route optimization is one of the main uses of AI and ML in the transportation industry. Reducing the length of time that vehicles are on the road can lower fuel consumption and carbon emissions by utilizing machine learning algorithms to evaluate traffic patterns and find the most effective routes.
Another important application of AI and ML in transportation is improving vehicle performance. By analyzing data on vehicle usage and identifying areas where performance can be improved, it is possible to reduce fuel consumption and cut carbon emissions. This can involve everything from optimizing engine performance to reducing idling time to improving aerodynamics.
In order to maximize the utilization of electric and hybrid cars, machine learning may also be applied. It is feasible to maximize the use of these cars and lessen the demand for non-renewable energy sources by anticipating consumption trends and optimizing charging and usage appropriately.
Renewable Energy
One of the most promising sectors where AI and ML may actually reduce carbon emissions is renewable energy. Optimizing their use and integration into the energy system is crucial given the rising uptake of renewable energy sources including solar, wind, and hydropower.
Predicting energy output is one of the main uses of AI and ML in renewable energy. As intermittent renewable energy sources can be unpredictable, integrating them into the system can be tricky. It is feasible to maximize the use of these sources and reduce the demand for non-renewable energy sources by utilizing machine learning algorithms to forecast the energy production from these sources.
Another important application of AI and ML in renewable energy is optimizing energy storage. Renewable energy sources often produce more energy than can be immediately used, making energy storage an important component of a sustainable energy system. By using machine learning algorithms to predict energy demand and optimize storage accordingly, it is possible to minimize the waste of renewable energy and reduce the need for non-renewable energy sources.
Also, the placement and layout of renewable energy infrastructure may be improved using machine learning. The best places for wind turbines and solar panels may be found by examining data on variables like wind patterns and sun radiation. By doing this, you can use renewable energy more effectively and use less non-renewable energy.
Carbon capture technologies
A interesting approach where AI and ML may drastically reduce carbon emissions is carbon capture technology. With the use of these technologies, carbon dioxide (CO2) emissions from power plants and industrial operations may be captured and kept from entering the environment. By analyzing data on factors like temperature, pressure, and flow rates, it is possible to optimize the capture process to maximize the amount of CO2 captured and minimize energy usage.
Monitoring and managing the storage of collected CO2 is a crucial use of AI and ML in carbon capture technology. To guarantee that the CO2 is kept in situ and does not escape into the atmosphere, carbon storage facilities must be closely watched. It is feasible to monitor these storage facilities and find any potential breaches by utilizing machine learning algorithms to assess data on elements like pressure and temperature.
Challenges in Implementing AI and ML for Energy Efficiency
The availability of data is one of the main obstacles to deploying AI and ML solutions for energy conservation. These technologies need a lot of data from sensors and other sources to find patterns and trends in order to be effective. Nevertheless, this information is frequently unavailable or of low quality. Due to this, it may be challenging for AI and ML systems to correctly identify inefficiencies and offer improvement suggestions.
The technical knowledge needed to build and maintain these solutions is another difficulty. The creation and use of AI and ML algorithms are difficult processes that call for specific understanding. Many businesses may find this to be a challenge, especially smaller ones that could lack the funding for specialist staffing or training.
Resource requirements are also a significant challenge. Implementing AI and ML solutions for energy efficiency can be costly, requiring investment in sensors, data storage and processing infrastructure, and specialized software. In addition, ongoing maintenance and upgrades can add to the costs of these solutions.
Finally, there is also the challenge of trust and acceptance. AI and ML algorithms are only as good as the data they are trained on, and there is a risk of bias and errors in the analysis. This can make it difficult for organizations to trust the recommendations made by these algorithms, particularly if they are not transparent about how they reach their conclusions.
Conclusion
In conclusion, reducing carbon emissions is a critical challenge facing our planet, and AI and ML have the potential to be powerful tools in this effort. By optimizing energy usage, transportation, renewable energy, and carbon capture technologies, it is possible to create a more sustainable and efficient energy system.
If you are interested in seeing how AI and ML can help reduce carbon emissions in your organization, we invite you to book a demo of papAI platform. Our platform uses the latest machine learning algorithms to optimize energy usage, transportation routes, renewable energy integration, and carbon capture technologies. By leveraging the power of AI and ML, we can help your organization to reduce its carbon footprint and move towards a more sustainable future.
Interested in discovering papAI?
Our commercial team is at your disposal for any questions.
How AI Drives the Evolution of Industry 4.0
How AI Drives the Evolution of Industry 4.0 Today, Industry...
Read MoreOptimize RAG Systems Like a Pro with Fine-Tuning
Optimize RAG Systems Like a Pro with Fine-Tuning As artificial...
Read MoreSupervised and Unsupervised Learning: A Comparison Guide
Supervised and Unsupervised Learning: A Comparison Guide Artificial intelligence (AI)...
Read More