Scaling RAG Systems in Financial Organizations Artificial intelligence has emerged...
Read MoreAI in Energy: Revolutionizing Peak Time Prediction and Management
Table of Contents
ToggleThe energy industry has seen a substantial transition recently, with an increased focus on environmental sustainability, efficiency, and cost-effectiveness. Peak time management, when demand for energy jumps at specific times and causes problems like power disruptions, higher costs, and an increased carbon footprint, is one of the biggest difficulties confronting the energy sector. Artificial intelligence (AI) has become a potent tool for forecasting peak energy demand periods in order to deal with this difficulty.
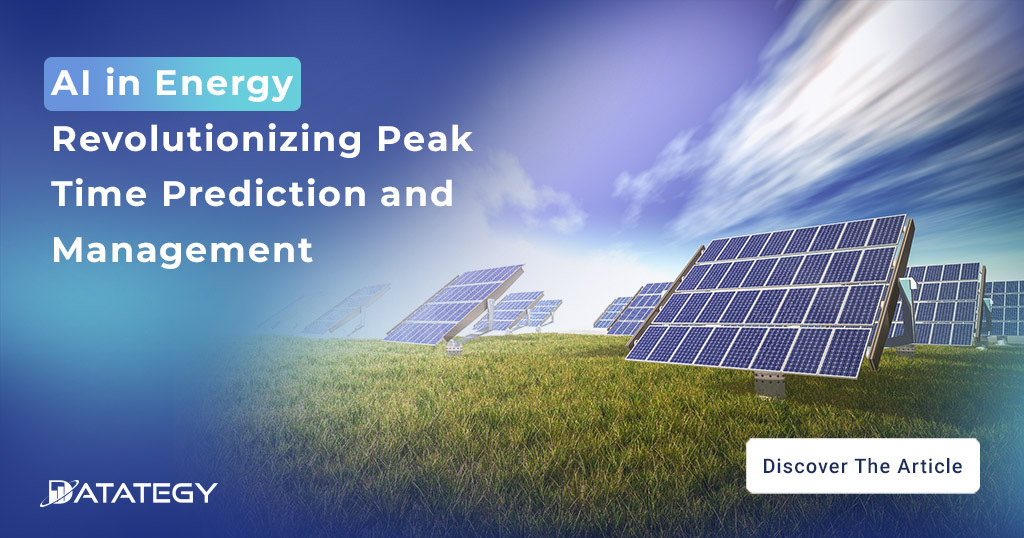
In order to effectively predict peak periods, AI techniques like machine learning and deep learning can examine vast quantities of data from several sources, such as weather predictions, historical energy consumption statistics, and real-time monitoring systems. The present state of the art in AI-based peak time prediction and management in the energy sector is examined in this article, along with the methods, resources, and tools being employed, as well as their advantages and disadvantages.
Rising energy production and consumption worldwide will boost market growth
The global AI in the energy management market is growing tremendously, projected to expand at a CAGR of 21.2% throughout the projected period from 2022 to 2030, starting from US$ 24.4 Bn in 2021. The global AI in the energy management market is majorly driven by the growing energy production & consumption worldwide along with leveraging artificial intelligence (AI) to improve grid stability and the growing need for smart energy management solutions. In 2018, the global primary energy consumption was 1, 57,063.77 TWh raised 2.4% from 1, 53,347.15 TWh in 2017.
Moreover, India, China, and the U.S. together accounted for over 2/3rd of the worldwide increase in energy demand. The surge in the use of renewable energy sources is another factor driving the growth of the market. The introduction of cloud-based software to improve service operations, to deliver real-time insights, and easy product development are some other major factors expected to enhance the market demand over the forecast period.
Traditional Peak Time Prediction Techniques
Peak time prediction is a critical aspect of energy management, where the demand for energy surges during specific periods. Traditional techniques for peak time prediction have been in use for many years, and while they have their limitations, they continue to be used in many energy management systems.
Historical analysis is one of the most often used conventional methods for peak time forecasting. In order to determine peak periods, historical data on energy consumption trends must be analyzed. The basis for historical analysis is the belief that current patterns of energy usage will hold true in the future. This method, however, has limitations because it doesn’t take into account outside variables like weather changes, consumer trends, and technology improvements that might affect energy use.
Forecasting load is another established method for peak period prediction. Using this method, the energy consumption is predicted based on the anticipated load at a specific moment. Mathematical models are used in load forecasting to account for variables like weather, time of day, and customer behavior. Nevertheless, load forecasting has limitations as it does not take into account sudden fluctuations in energy demand, such as those brought on by severe weather.
Peak shaving is a third conventional method for peak time prediction. Moving energy use to off-peak hours, this strategy lowers the demand for energy during peak hours. Several techniques, including load shifting, energy storage, and demand response programs, are used to reduce peak demand. Peak shaving can be a good strategy to cut down on energy use at peak times, but it needs a lot of infrastructure investment and might not be feasible for all energy suppliers.
How to choose the best AI solution for your data project?
In this white paper, we provide an overview of AI solutions on the market. We give you concrete guidelines to choose the solution that reinforces the collaboration between your teams.
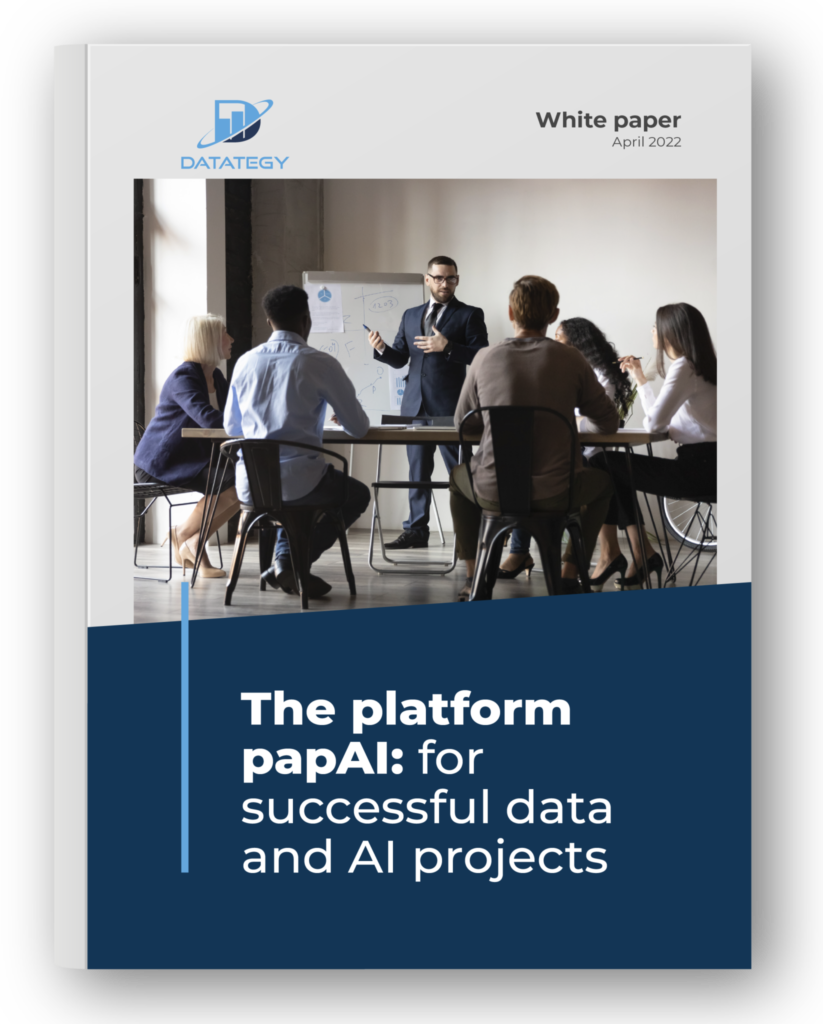
AI Techniques for Peak Time Prediction
1- Machine learning
A technique called machine learning involves looking for patterns and correlations in huge datasets. Machine learning algorithms can use previous energy consumption data, weather forecasts, and other pertinent data to anticipate future patterns of energy usage when it comes to peak times. For instance, a machine learning system may examine historical data on energy use for a particular area, taking into account variables like the time of day, day of the week, and season. The programme might then utilise this information to forecast future energy consumption trends in that area while accounting for changes in environmental elements like weather.
2- Deep learning
In a more sophisticated kind of machine learning known as deep learning, neural networks are trained to identify intricate patterns in vast datasets. Deep learning algorithms are capable of analyzing significant volumes of data from several sources, including social media, weather predictions, and smart meters, in the context of peak time prediction. For instance, a deep learning system may examine data from smart meters in a residential neighborhood to find trends in energy use based on variables like temperature, day of the week, and time of day. The program might then utilize this information to anticipate future trends in the area’s energy usage.
3- Reinforcement Learning
A method known as reinforcement learning includes teaching an agent to base decisions on rewards and punishments. Consumers can be encouraged to use less energy during peak hours by using reinforcement learning in the context of peak time prediction. An energy supplier may, for instance, provide customers discounts or other rewards for lowering their energy use during peak hours. Therefore, using information like weather predictions and previous energy use trends, an algorithm might be taught to provide suggestions to consumers based on these incentives.
4- Hybrid Techniques
For more precise and effective peak time prediction, hybrid approaches combine several AI algorithms. To examine data and find intricate patterns, for instance, a mix of machine learning and deep learning algorithms might be utilized. By combining the advantages of several AI approaches, hybrid algorithms have the benefit of being able to make predictions that are more accurate. For instance, a hybrid algorithm may first discover broad patterns in energy use using machine learning, and then further find more intricate patterns within those broad patterns using deep learning.
Benefits of AI-Based Peak Time Prediction
1- Improved Accuracy
Improved accuracy is one of the main advantages of AI-based peak time prediction. According to a report by the International Energy Agency, AI-based energy management systems can reduce peak demand by up to 10% and reduce overall energy consumption by up to 15%. AI algorithms are made to examine vast volumes of data and spot patterns that people might not see right away. AI algorithms are able to produce precise projections of energy consumption during peak periods by evaluating data from several sources, including weather forecasts, smart meters, and customer behavior data. Energy management may use this to streamline energy use and avoid blackouts.
2- Cost Savings
Another benefit of AI-based peak time prediction is cost savings. By accurately predicting energy demand during peak times, energy managers can optimize energy usage and avoid the need to purchase additional energy at high prices. This can result in significant cost savings, particularly for energy-intensive industries such as manufacturing and transportation.
3- Improved Energy Efficiency
Peak time forecasting using AI can boost energy efficiency as well. According to a report by the US Department of Energy, AI-based energy management systems can reduce energy consumption by up to 20% by optimizing energy usage during peak times.. Energy managers may cut down on wasteful energy use and raise overall energy efficiency by forecasting peak periods and managing energy use. This can assist businesses in lowering their carbon footprint and achieving their environmental objectives.
4- Real-Time Monitoring
AI-based peak time prediction can also provide real-time monitoring of energy usage. By analyzing data from smart meters and other sources in real-time, energy managers can identify potential issues and take corrective action before they lead to blackouts or other disruptions. Real-time monitoring can also help organizations identify areas where they can further improve energy efficiency.
5- Scalability
AI algorithms are perfect for large-scale energy management because they can instantly assess vast volumes of data from several sources. Energy managers may optimize energy use across several sites and save expenses on a worldwide level with the aid of this scalability.
According to a report by McKinsey & Company, AI-based energy management systems can be easily scaled to manage energy usage across multiple locations and reduce costs on a global scale. The report notes that large industrial companies can save up to $1 million per year by using AI-based energy management systems.
Build your own peak time prediction tool using papAI platform
In conclusion, the use of AI-based techniques for peak time prediction offers numerous benefits, including improved accuracy, cost savings, improved energy efficiency, real-time monitoring, and scalability. And with the papAI platform, you can easily build your own Peak Time Prediction tool with high explicability of the results.
papAI platform offers a user-friendly interface that allows energy managers to input data from various sources, such as weather forecasts, smart meters, and consumer behavior data. The platform’s AI algorithms then analyze this data and provide accurate predictions of energy demand during peak times. The results are highly interpretable and provide detailed explanations of the factors that contribute to the predicted demand. By using papAI, energy managers can optimize energy usage, reduce costs, and improve energy efficiency. The platform’s real-time monitoring capabilities also allow for quick identification of potential issues and corrective action to prevent blackouts or other disruptions.
If you’re interested in learning more about the papAI platform and how it can help you build your own peak time prediction tool, you can book a demo with one of our AI experts. Our team can show you how the platform works and help you get started on your own.
Interested in discovering papAI?
Our commercial team is at your disposal for any questions.
How AgenticAI is Transforming Sales and Marketing Strategies
How AgenticAI is Transforming Sales and Marketing Strategies Agentic AI...
Read More“DATATEGY EARLY CAREERS PROGRAM” With Abdelmoumen ATMANI
“DATATEGY EARLY CAREERS PROGRAM” With Abdelmoumen ATMANI Hello, my name...
Read MoreIntroducing the Error Analysis Tree: A Smarter Way to Improve AI Models
Introducing the Error Analysis Tree: A Smarter Way to Improve...
Read More