Supervised and Unsupervised Learning: A Comparison Guide Artificial intelligence (AI)...
Read MoreSurvival Analysis Modeling for Customer Churn prediction on papAI
Table of Contents
ToggleSurvival analysis was developed to measure lifespans of individuals. It can be applied to any situation where we have births and deaths (duration). Medical professionals might be interested in the time between childbirths, where a birth in this case is the event of having a child, and a death is becoming pregnant again, Another example is users subscribing to a service: a birth is a user who joins the service, and a death is when the user leaves the service.
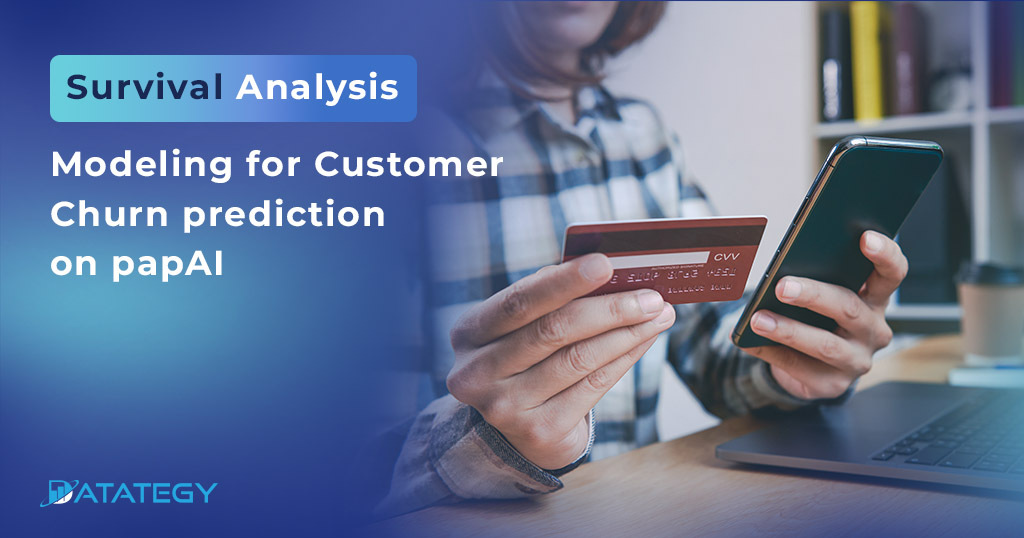
What’s a customer churn ?
Customer churn is a problem that can be modeled using survival analysis. It is a business decision-making process to describe customer behavior during the time period before they stop doing business with us, and decide to move on to another supplier or not purchase anymore from any provider at all.
Customer churn can be defined as every case where a customer decides that she/he wants to end the relationship with a company. There are several factors that can increase customer churn, such as poor service quality or pricing. Customer churn not only means that the customer is moving to another company, but it can also mean losing a customer in terms of reducing purchases or being late with payments.
How to use survival analysis for customer churn problems ?
- identification of the survival time :

- Identification of Data :
- Choose the model :
– Log-Normal AFT model
– Log-Logistic AFT model

We can train multiple models with different combinations of different features at the same time and then compare their performances after being evaluated via papAI to select the best model that will be used later to do predictions on new individuals data.

- Validate and interpret the survival model :

We can also take a look at the features coefficients to interpret the model and see which features impact more the decision of churn to users and compare between different values of a specific feature or combination of more using the partial effects on outcome plot.

Customer churn is a major concern that affects not only the growth of your business, but also the profit.The churn analysis technique can help you to better understand the value of your customers and initiate a retention process based on the different metrics that the papAI platform offers.
Interested in discovering papAI ?
Our commercial team is at your disposal for any question.
“DATATEGY EARLY CAREERS PROGRAM” With Zineddine Chikhaoui
“DATATEGY EARLY CAREERS PROGRAM” With Zineddine CHikhaoui Hello, my name...
Read MoreAI Governance Pathway: Challenges and Solutions
AI Governance Pathway: Challenges and Solutions Recent advances in artificial...
Read More