Supervised and Unsupervised Learning: A Comparison Guide Artificial intelligence (AI)...
Read MoreOverview of AI solutions on the market
Table of Contents
ToggleAccording to a PwC study, artificial intelligence is expected to increase global GDP by 14% by 2030. However, it remains difficult today to measure the real impact of AI technologies. Indeed, many projects do not come to fruition due to a lack of means, knowledge, resources or framework to make them evolve. However, many solutions are available to companies seeking to leverage their data. So, how do you find your way around? Here is an overview of existing AI software to guide you in your thinking.
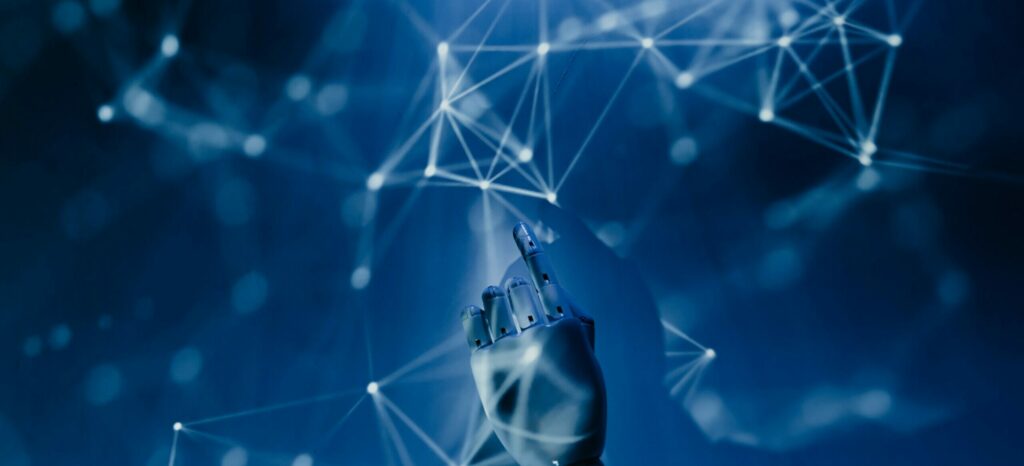
Internal AI developments for more control
Many companies believe that their need for predictive analysis is so specific that an existing AI tool cannot handle it and they start developing a tailor-made solution in-house.
This brings advantages but also limitations:
- Without a doubt, in-house AI developments allow you to keep full control over your workflows and projects. It allows you to relatively adapt to unforeseen events, to the activity and to the evolution of your needs.
- In terms of costs, in-house developments seem more attractive on paper. However, they require dedicated and specialised skills, the presence of profiles mastering the arcana of AI algorithms. These projects can therefore quickly become more expensive than using a software from the market.
- Each development has its share of unforeseen events, which mobilise resources for a longer period of time, not to mention the costs of maintenance after development. This has a direct impact on the efficiency of the project and its costs.
- Technologies evolve rapidly, it is sometimes difficult to keep up with all the trends, to integrate the latest innovations from AI research. This is why it is sometimes necessary to call on external skills, in order to have a modern, efficient and scalable application leading to projects that can be used on a daily basis.
Specialised AI tools: ETL, algorithms, restitution
Many tools are specialised in a specific stage of an AI project. This includes data collection and storage for example, or data qualification. Others are limited to offering AI algorithms. Some focus on the visualisation of data and results. But few offer a complete solution to cover the entire project: from data collection to the restitution and analysis of results. This implies the multiplication of the number of tools to be deployed and used to carry out data projects: an ETL, a machine learning tool, a BI tool, etc. This is often a barrier for decision-makers, as it adds complexity to the process. Not only do several tools have to be deployed, but their integration leads to additional costs and fragmented adoption from its users, decreasing ROIs.
Machine learning tools: do we really cover the whole field of AI?
Many so-called Artificial Intelligence solutions are in fact machine learning solutions. Of course, it is an artificial intelligence technology, but it is not the only one. It is therefore not relevant to equip yourself with a machine learning solution for all AI projects in your company.
Machine learning allows machines to learn and self-correct, but not to properly reason and answer to actual business issues. AI seeks to create behaviours close to those of humans, constantly and quicker, so it goes further than machine learning (with rule engines for example).
AI platforms for customized projects
Artificial intelligence platforms are solutions that offer companies with the possibility of developing several AI projects and covering the whole value chain of a project, both thanks to the variety of algorithms offered and to the centralisation of data in a single tool.
These solutions are increasingly “user centric” and are more ergonomic than other, more technical and less intuitive software. This is therefore a significant advantage in facilitating the interpretation of results and the use of the solution by non-IT profiles. The papAI platform falls into this category.
Vertical tools for specific needs
Some companies choose to deploy AI solutions dedicated to a specific application domain. For example, in identity verification, fraud anticipation (transport, insurance, etc.), health, or predictive maintenance. These specialised AI solutions make it possible to exploit specific data and algorithms adapted to the business sector, thus more precise and more reliable.
Often these AI-based applications are integrated with complete business solutions (such as CMMS in the case of maintenance). The editors are thus counting on AI to enrich, maintain and make their solution more efficient, at the cutting edge of technologies and trends. However, this forces companies equipped with this type of solution to deploy others to take advantage of AI in different areas.
This overview shows that there is no shortage of choice when it comes to AI software. To guide your decision, it is important to weigh the pros and cons of each alternative, to frame your need in relation to your objectives and the skills you have available. Multiplying AI solutions in your IS will not guarantee the success of your data projects.
Choose the best AI software that supports multiple use cases, manages data from collection to visualization and predictive model modeling are key criteria to consider. An AI solution, such as papAI, gives concrete ROIs, and allows exporting data projects from the platform if some companies prefer to stay on an internal solution.
Interested in discovering papAI?
Our commercial team is at your disposal for any question.
Recommended articles
“DATATEGY EARLY CAREERS PROGRAM” With Zineddine Chikhaoui
“DATATEGY EARLY CAREERS PROGRAM” With Zineddine CHikhaoui Hello, my name...
Read MoreAI Governance Pathway: Challenges and Solutions
AI Governance Pathway: Challenges and Solutions Recent advances in artificial...
Read More