Why AIOps Is Key to Cyber Threat Detection in Defense?...
Read MoreArtificial intelligence: three concrete use cases
Table of Contents
Toggle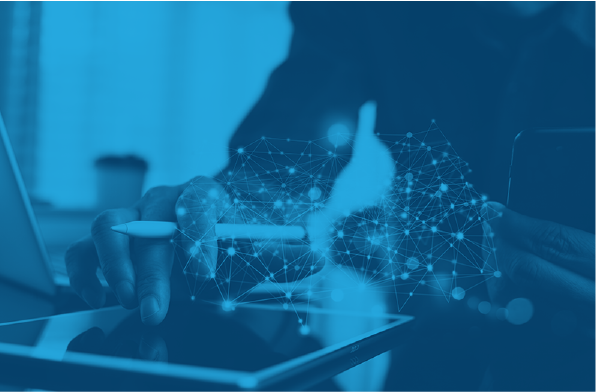
Artificial intelligence projects can be complex, time-consuming and in the worst cases, they can result in failure. It is therefore quite challenging for companies to launch their AI projects without guarantees of success. However, when done well, they bring considerable gains to organizations.
Because we are convinced that facts are worth more than long speeches, we have decided to give you three concrete examples of artificial intelligence projects in the following sectors: industry, telecom and energy.
Artificial intelligence and industry: when predictive maintenance reduces costs
According to McKinsey, predictive maintenance will help companies save $630 billion by 2025, with maintenance costs reduced by 10-40%. The report also estimates that the number of breakdowns could be halved and new machine purchases will decrease by 3-5% by increasing the life of existing equipment.
By definition, predictive maintenance is about detecting faults in machines before they become too serious and production is halted. Data analysis is the key to this prevention, as it allows weak signals to be detected. How can this be done?
Three factors are involved:
- The implementation of sensors on machines, IoT (Internet of Things).
- Modeling of a breakdown pattern: by analyzing the history of breakdowns, it is possible to identify the most likely causes.
- Development of predictive algorithms: to determine alert thresholds (with machine learning for example).
For example: Schindler or ThyssenKrupp lifts have been equipped with sensors that provide data on temperature, door openings, number of floors delivered, etc., with a view to predict technician interventions and thus avoiding breakdowns.
AI projects in telecoms: using scoring to improve customer relations
The telecoms sector is very competitive and customers are not always loyal to their current provider: if a consumer finds a better deal elsewhere with another operator, it will not hesitate to cancel their contract and go to the competition. Offering the right deals at the right time is therefore key to building consumer loyalty and retention. However, some people are not fully receptive to these offers and it is not necessary to devote energy retaining them.
In order to be able to identify the best customers to concentrate efforts on, a scoring system can be set up thanks to artificial intelligence. For this, it is necessary to take into account the following data:
- Telephone calls from customers to the provider
- Different contracts taken out
- Interventions made by the customer service
- Customer satisfaction
- Opinions on social networks…
The more open a customer is to new products and promotions, the easier it will be to retain them. Using a predictive algorithm that scores customers based on their reactions to different solicitations and interactions, telecom operators can focus their efforts on those most likely to accept a new offer. Scoring models are built on the basis of billions of combinations, so it is important to have the technology that can process and consolidate large volumes of data but also evolve with offers, customer requirements and trends.
Energy: using Artificial Intelligence to predict churn
Churn refers to the loss of customers or subscribers. Energy suppliers are confronted with customer dissatisfaction at the time of the annual adjustment. This is because interim bills are calculated on the basis of forecasts and estimates. Consumers are therefore often surprised when the regulation arrives and often seek to change energy supplier at that time.
To remedy this issue, better forecasting is key. And a lot of data is available, such as :
- Several months of consumption history
- Demographic information
- Household equipment
- Weather
- Events such as the current pandemic, which favors home office and therefore significant variations in consumption
Artificial intelligence makes it possible to quickly process large volumes of data in real time, connecting different databases to train and update predictive models. This allows suppliers to make more accurate predictions of consumption – and intermediate bills. The models also help answer questions such as: Why do customers want to leave? What promotion could keep them? Which offer is the most suitable?
Some figures on churn and its consequences in energy:
- A very satisfied customer tells 3 other people
- An unhappy customer tells 12 other people
- A very dissatisfied customer tells 20 other people
- 98% of unhappy customers never complain, they just leave you
Source: Customer Genius by Peter Fisk
Recommended articles
How AI Transforms Predictive Maintenance in Defense Equipment
How AI Transforms Predictive Maintenance in Defense Equipment In a...
Read MoreHow to Scale AI Without Breaking Your Infrastructure in 2025
How to Scale AI Without Breaking Your Infrastructure in 2025...
Read More